ABSTRACT
Failure of the normal process of cell death pathways contributes to the defection of immune systems and the occurrence of cancers. The key genes, the multimolecular mechanisms, and the immune functions of these genes in pan-cancers remain unclear. Using online databases of The Cancer Genome Atlas, GEPIA2, TISIDB, HPA, Kaplan-Meier Plotter, PrognoScan, cBioPortal, GSCALite, TIMER, and Sangerbox, we identified the key genes from the six primary cell death-related pathways and performed a comprehensive analysis to investigate the multimolecular characteristics and immunological functions of the hub genes in 33 human cancers. We identified five hub genes in the six primary cell death-related pathways (JUN, NFKB1, CASP3, PARP1, and TP53). We found that CASP3, PARP1, and TP53 were overexpressed in 28, 23, and 27 cancers. The expression of the five genes was associated with the development and prognosis of many cancers. Particularly, JUN, NFKB1, CASP3, and TP53 have prognostic values in Brain Lower Grade Glioma (LGG), while PARP1 and CASP3 could predict the survival outcomes in Adrenocortical carcinoma (ACC). In addition, an extensive association between five genes’ expression, DNA methylation, and tumor-immune system interactions was noticed. The five cell death-related hub genes could function as potential biomarkers for various cancers, particularly LGG and ACC. The immunological function analysis of the five genes also proposes new targets for developing immunosuppressants and improving the immunotherapy efficacy of cancers. However, further extensive clinical and experimental research are required to validate their clinical values.
1. Introduction
Cell death is essentially involved in organism development, disease pathogenesis, and tumor inhibition by regulating cell proliferation, stress response, and tissue homeostasis [Citation1,Citation2]. Accumulating evidence indicates that cell death is crucially associated with the immune defense against multiple human diseases, including cancer, and the failure of the normal cell death process contributes to the tumor immune escape and treatment resistance [Citation3]. In recent years, it has been a hot spot to develop different targeted therapy strategies for different ways of cell death, types of cancer, and microenvironment, which could effectively reduce resistance to cell death.
According to whether the process of cell death is energy-dependent, it can be divided into energy-dependent pathways that are highly regulated (autophagy, pyroptosis, apoptosis) and pathways that are energy-independent (necrosis/oncosis) [Citation4–6]. A tumor occurs when the death pathways that play a protective role in normal cells by removing the damaged cells are limited or circumvented [Citation7]. Disfunction and failure of the six primary cell death pathways would contribute to the malignant transformation and cancer development [Citation8–12]. It is well accepted that completing the correct pathway of cell death is a critical cellular function for ensuring the final achievement of proper results in multicellular organisms. Failure to respond to the death stimulus can lead to embryonic development, organ dysfunction, and the tumorigenesis of cancer [Citation13]. However, the precise pathophysiological significance, detailed signal transduction, and specific biomarkers of the cell death pathways in human cancers are unclear.
In the current study, we identified several hub genes from the complex and large interacting genes related to the five major cell death pathways (autophagy, necrosis, apoptosis, pyroptosis, and oncosis) and discussed their multimolecular characteristics and immune function in various cancers; the study design was shown in Figure S1. A deeper exploration of the multimolecular characteristics and immune role of these genes in human tumors from the perspective of cell death could provide novel perspectives for discovering the potential diagnostic and prognostic biomarkers of human cancers and improve the cancer immunotherapy efficacy in the future.
2. Materials and methods
2.1. Identification of candidate genes related to primary cell death pathways
We used Gene Cards (https://www.genecards.org/) database to obtain the gene sets that related to autophagy (6318 genes), necrosis (8580 genes), apoptosis (13,933 genes), pyroptosis (146 genes), and oncosis (33 genes). R packages of “upset” and “ggvenn” were utilized to generate the Venn diagram of the cell death-related genes (131 candidate genes that appear in four of the five cell death pathways and eight common genes that appear in all cell death pathways were identified).
2.2. Expression analysis of the five hub genes in various human tumors from TCGA databases
Multiple databases were utilized to explore the difference in the five (JUN, NFKB1, CASP3, PARP1, and TP53) hub genes’ expression between cancers and normal samples in various cancers. Using the “ggplot2” R package in R software of the 3.6.3 version, we downloaded the transcripts per million reads (TPM) format RNAseq data from TCGA [Citation14] and GTEx, which were processed by UCSC XENA (https://xenabrowser.net/datapages/) through the Toil process [Citation15], to analyze the expression of five hub genes in cancers and normal samples of available 30 cancers (MESO, SARC, and UVM were excluded owing to the lack of normal control data). The RNAseq data in TPM format were log2 transformed and subsequently compared. In addition, using the same way, the differential expression of several famous key genes in other cell death pathways, such as CASP8 (apoptosis), RIPK1, RIPK3, and MLKL (necroptosis), GPX4, SLC7A11 (ferroptosis), CASP1, GSDMD (pyroptosis), BECN1, and ATG5 (autophagy) in various TCGA cancers were also discussed the role of several famous key genes in other cell death pathways in various human cancers
2.3. Survival analysis of the five hub genes in various human tumors from GEPIA2 database
To comprehensively investigate the prognostic role of the five hub genes in different human cancers, we used the GEPIA2 database [Citation16] to compare the survival contribution of gene expression in 33 human cancers after setting the “Significance level” as P < 0.05 and “Group Cutoff” as “median,” and the Overall Survival (OS) and Disease-Free Survival (DFS) of the five genes in each cancer were obtained. Using the “Survival Analysis” function of GEPIA2, the Kaplan-Meier curves were plotted for cancer types with statistical significance of OS.
2.4. Genetic alteration analysis from cBioPortal databases
The co-expression patterns of the five hub genes in several specific cancers were explored under the instructions of the cBioPortal database in the corresponding module (http://www.cbioportal.org/) [Citation17]. The correlation between each gene’s expression and their most correlated 20 genes in specific cancers was also obtained using the R package of “stat” from data of the TCGA database. The TCGA alteration data was downloaded from the cBioPortal database in the “OncoPrint” section to analyze the pan-cancerous genetic changes of the five genes. The types of genetic alteration (e.g. amplification, deep deletion), the study of origin (different cancer types from TCGA Pan-Cancer Atlas), and mutation features were also obtained.
2.5. Immune analysis of the five hub genes from TIMER databases
Using the TIMER (https://cistrome.shinyapps.io/timer/) [Citation18] database, we explored the relationship between the five genes’ expression and the abundance of typical infiltrating immune cells (neutrophils, macrophages, CD4 + T cells, CD8 + T cells, B cells, and dendritic cells) and the major gene markers of specific immune cells. The values of each correlation were recorded and presented as a heatmap.
3. Results
3.1. Hub gene identification of cell death-related genes
As shown in , we applied a Venn diagram among the five cell death pathways-related genes and identified 131 candidate genes (appear in four of the five pathways) and eight common genes (appear in all five pathways). The protein-protein interactions of these candidate genes are presented in , and the highly connected genes in the MCODE module are shown in . Combining the genes in the MCODE cluster and the eight common genes, we get five hub genes that appear in all five primary cell death pathways and have a high degree of connectivity: JUN, NFKB1, CASP3 PARP1, and TP53 (). The functional and pathway enrichment analyses were presented in the supplementary files.
Figure 1. Hub gene selection and cell-death related gene enrichment analysis. (a) Venn diagram of genes common to apoptosis, autophagy, pyroptosis, oncosis, and necrosis (123 candidate genes and 8 common genes were identified). (b) Protein–protein interaction (PPI) network constructed with the candidate genes. (c) Interaction of genes with higher degree of connectivity (MCODE). (d) Venn diagram of common genes and MCODE (five hub-genes were identified). (e) Functions of the candidate genes were predicted by the analysis of gene ontology (GO, and Kyoto Encyclopedia of Gene and Genomes (KEGG) by Metascape tolls). (f) Descriptions of top three significantly enriched GO terms and KEGG pathways of candidate genes. (g) PPI network of the five hub-genes (GeneMANIA).
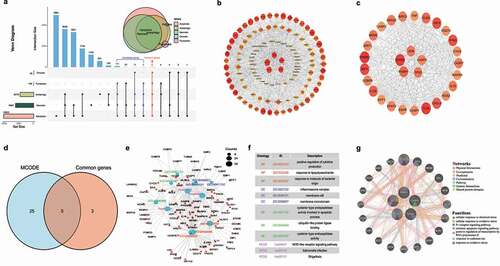
3.2. Expression of the five hub genes in diverse human cancers
We used R software to explore the difference of the five hub genes’ expression between normal samples and cancers across 30 cancer types from TCGA. We found that JUN was less expressed in 14 cancers and more expressed in seven cancers. NFKB1 was more expressed in 15 cancers and less expressed in 11 cancers. CASP3 was more expressed in 28 cancers. Higher expressions of PARP1 and TP53 were noticed in 23 and 27 cancers, respectively (Figure S2a-e). Interestingly, for cancers with various subtypes, such as kidney cancer, we noticed that JUN and TP53 were over-expressed in Kidney renal clear cell carcinoma (KIRC) and less expressed in Kidney Chromophobe (KICH). Besides, the expression of TP53 was also over-expressed in Kidney renal papillary cell carcinoma (KIRP), suggesting a dominant up-regulation role of TP53 in kidney cancers. Such dominant up-regulation role of genes in kidney cancers was also found in NFKB1 and CASP3. While PARP1 was only less expressed in KICH. Concerning lung cancers, we found that PARP1, NFKB1, CASP3, and TP53 were over-expressed, whereas JUN was less expressed both in Lung squamous cell carcinoma (LUSC) and Lung adenocarcinoma (LUAD), suggested each gene plays the same regulation role in different subtypes of lung cancer. The protein expression of the five hub genes in human cancers was assessed using the HPA database and described in the online supplementary files.
3.3. Association between the transcriptional levels of the five genes and the clinical outcomes in diverse human cancers
To explore the prognostic value of the gene’s expression in different cancers, the cases of various cancers were divided into the high and low expression groups according to the expression levels of the five genes. The prognostic role of the five hub genes in different cancers based on the data from various databases was described in online supplementary files. Generally, we found that JUN, NFKB1, CASP3, and TP53 have a detrimental value in Brain Lower Grade Glioma (LGG). NFKB1 and PARP1 have a detrimental value in Adrenocortical carcinoma (ACC). Specifically, JUN has a protective value in Breast invasive carcinoma (BRCA), and NFKB1 has protective values in KIRC. By contrast, PAPR1 has detrimental values in Renal cancers, including KIRC, KIRP, and KICH (Table S3). Among all the cancer types, we selected LGG, KIRC, Colon adenocarcinoma (COAD), and ACC to plot the survival curves using the GEPIA2 database (). Since the expression of multiple genes in LGG and ACC is related to their prognosis, to verify the independent prognostic role of the genes in the two cancers, we constructed prognostic nomograms and calibration curves using the statistically significant parameters from the univariate Cox regression analysis. As presented in , the combination of TP53, JUN, NFKB1, CASP3, and clinical parameters can be used to predict the one-year, three-year, and five-year survival probability of LGG with the c-index of 0.858 (0.804–0.912), while the combination of CASP3, PARP1, and clinical parameters could predict the survival of ACC with the c-index of 0.833 (0.799–0.867). The role of the five cell death-related hub genes in the carcinogenesis of different cancers was presented in the online supplementary files.
Figure 2. Correlation between the five hub genes expression and survival prognosis of different cancers. We used the GEPIA2 tool to perform overall survival (a) and disease-free survival (b) analyses of different tumors in TCGA by the five-hub genes expression. The cancer types with statistical significance (p < 0.05) were highlighted. The Kaplan-Meier curves of the overall survival data of LGG, ACC, COAD, and KIRC are given (c). (d) Heatmap of the overall survival data across 19 human cancers from the Kaplan-Meier plotter. The cancer types with statistical significance (p < 0.05) were highlighted. (e) Correlation between the expression of five genes and survival in different cancers (only cancer types with statistical significance were presented). (f) Prognostic nomograms and calibration curves for the five hub-genes in LGG and ACC.
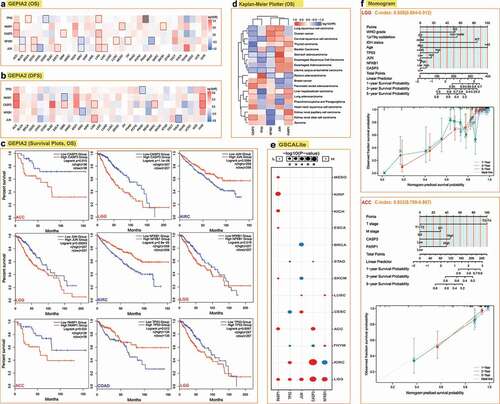
3.4. Extensive genetic alteration of the five hub genes in human cancers
We analyzed the genetic alterations of the five hub genes using the database of cBioPortal for 33 tumors based on the mutation and copy number variation data in TCGA. As shown in Figure S10a, the highest alteration frequency of the five genes was found in TP53 (36%), with an average mutation frequency of 1.4%-4% for other genes. The five cell death-related hub genes also presented widespread CNV amplification in multiple cancers (Figure S10a). The highest alteration frequency of the five genes in different cancers was found in Sarcoma (SARC) for JUN (>6%, with “amplification” as the major type), Uterine Corpus Endometrial Carcinoma (UCEC) for NFKB1 (>6%, with “mutation” as the major type), DLBC for CASP3 (>8%, with “deep deletion” as the major type), BRCA for PARP1 (>8%, with “amplification” as the major type), and UCS for Uterine Carcinosarcoma (TP53) (>80%, with “mutation” as the major type) (Figure S10b). The landscape of the five hub genes mutations in cancers was presented in Figure S10c.
3.5. Cell death-related hub genes are genetically, epigenetically, and transcriptionally associated with diverse human cancers
According to the somatic mutation data from TCGA, we found that the SNV of the five genes extensively existed in multiple cancers, especially for TP53 (with a mutation frequency of 96%). Most mutations of the five genes in tumors with high SNV loads were found in Head and Neck squamous cell carcinoma (HNSC) and Liver hepatocellular carcinoma (LIHC) and (Figure S11a). As shown in Figure S11b, the gene with the highest mutation rate in tumors is TP53, especially in LUSC, where TP53 mutation is found in 405 cases. The impact of CNV and methylation on the five genes’ expression was also analyzed, and the detailed results were described in the online supplementary files. Besides, the association between genes and the 10 famous cancer-related pathways was also described in the online supplementary files.
3.6. Association between immune cell infiltration, typical immune markers, and the five genes’ expression across human cancers
We used the TIMER database to explore the correlation between the typical six immune cells in diverse cancers. As shown in and Table S8, JUN expression was associated with the infiltration levels of Neutrophil in 21 cancers, CD4 + T cells in 18 cancers, Dendritic cells in 19 cancers, Macrophage in 21 cancers, CD8 + T cells in 13 cancers, and B cells in 15 cancers. NFKB1 expression was correlated with the infiltration levels of Macrophage in 20 cancers, Neutrophil in 30 cancers, Dendritic cells in 27 cancers, B cells in 21 cancers, CD4 + T cells in 24 cancers, and CD8 + T cells in 25 cancers (, Table S9). The significant correlations between CASP3 and the infiltration levels of B cells, CD4 + T cells, CD8 + T cells, Dendritic cells, Macrophages, and Neutrophils were noticed in 20, 16, 21, 22, 17, and 21 cancer types, respectively (, Table S10). PARP1 expression was associated with the infiltration levels of Macrophage in 21 cancers, Neutrophil in 18 cancers, B cells in 24 cancers, Dendritic cells in 21 cancers, CD4 + T cells in 17 cancers, and CD8 + T cells in 18 cancers (, Table S11). As presented in and Table S12, TP53 expression was associated with the infiltration levels of Macrophage in nine cancers, Neutrophil in 11 cancers, Dendritic cells in 13 cancers, B cells in nine cancers, CD4 + T cells in 14 cancers, and CD8 + T cells in 11 cancers. The association between the five genes’ expression and the 45 immune markers of 18 immune cells and the MSI, immune score, immune checkpoints, neoantigen counts, TILs, and three immunomodulators were described in the online supplementary files.
Figure 3. Interactions of the five hub genes’ expression and immune cell infiltration. Correlation heatmap of the expression of JUN (a), NFKB1 (b), CASP3 (c), PARP1 (d), TP53 (e), and immune cell infiltration across different cancers. (f) Correlation heatmap of the expression of the five genes and immune cells related gene markers across different cancers. The correlation values were normalized by row scale.
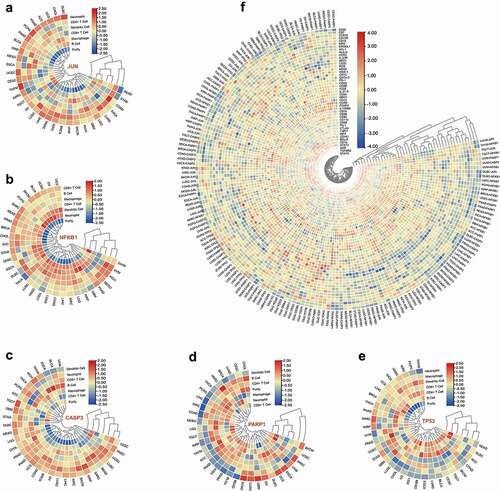
4. Discussion
The current study identified five hub genes from the large-scare and complex interacting gene networks of cell death-related pathways (autophagy, necrosis, apoptosis, pyroptosis, and oncosis). Our results showed that NFKB1, CASP3, PARP1, and TP53 were over-expressed in various cancers, while JUN was less expressed in several cancers, which indicated that JUN might act as a protective factor and other genes serve as detrimental biomarkers in cancers. However, such preliminary assumptions need further corroboration. Based on the results from multiple databases, we found that the expression of JUN, NFKB1, CASP3, and TP53 could act as prognostic biomarkers in various cancers, particularly in LGG, while CASP3 and PARP1 also have a prognostic value in ACC. We also found that the aberrant expression of the five genes was associated with SNV, CNV, and DNA methylation in various cancers. Moreover, such abnormal expression was related to immune cell infiltration, MSI, immune score, expression of immune checkpoint genes, neoantigen counts, TILs, and immunomodulators, which indicated a close interaction between the five genes’ expression and the immune response in different cancers.
Previously, the role of the five genes in tumorigenesis and the development of cancers have been reported [Citation19–28]. The current study observed that JUN has a protective value in several cancers such as LGG and KIRC. Adhered to the previous research, we found that NFKB1 has protective values in various cancers, such as BRCA, KIRC, BLCA, and Rectum adenocarcinoma (READ). In BRCA and KIRC, we also noticed that NFKB1 expression was associated with the clinicopathological stages, which suggests that NKFB1 could act as an index to monitor the progress and prognosis in these cancers. Further extensive clinical and experimental research are required to validate our findings and to explore the underlying mechanisms. In the current study, the protective role of CASP3 in STAD was observed, and we found that high expression of CASP3 indicates a worse survival of some cancers, such as ACC, LGG, KIRC, and GBM. Previously, the aberrant expression of CASP3, which was induced by cisplatin, has been found in many tumor cells, and close associations between the regulation of CASP3 and the survival and chemoresistance were reported in lung cancers [Citation29–32]. Therefore, the detrimental role of CASP3 in certain cancers may also be due to its intense association with chemoresistance. We also found that PARP1 can be a detrimental biomarker in many cancers, and the association between PARP1 expression and the development of cancers was also noticed, indicating the potential of PARP1 in monitoring tumor progression in these cancers. TP53 is a mutated gene commonly seen in human cancers, which participates in response to death stimuli such as oncogenic hyperproliferation, oxidative, and DNA damage [Citation20]. As a tumor suppressor, we found TP53 has protective values in several cancers such as Colon adenocarcinoma (COAD), STAD, Bladder Urothelial Carcinoma (BLCA), and Cervical squamous cell carcinoma (CESC). However, the detrimental values of TP53 were also noticed in multiple cancers, such as LGG, BRCA, and KIRC. Since accumulating evidence suggests a high correlation between TP53 mutation and the worse survival of numerous cancers [Citation33], the detrimental role of TP53 in these cancers may be owing to its high mutation in those tumors. Consistently, among the five genes, we observed a high alteration frequency of TP53 in many cancers.
Many studies have reported that the alteration of JUN, NFKB1, CASP3, and PARP1 is associated with the prognosis of specific cancers [Citation34–37]. Besides TP53, the alteration of JUN, NFKB1, CASP3, and PARP1 in various cancers were also observed, which could partially explain the prognostic values of these four genes in specific cancer. One of the famous epigenetic factors that regulate gene expression is DNA methylation, which is found crucially involved in the occurrence and development of many cancers [Citation38,Citation39]. We found numerous significant correlations between DNA methylation and the expression levels of the five genes in many cancers, suggesting the epigenomic role of the five hub genes in the progress and prognosis of various cancers. Moreover, statistical correlations between the expression of five genes and drug sensitivity were observed, which could assist in the decrease of drug resistance in cancer treatment and thus improve the outcome of cancer patients.
Recently, the dense connection between immunity and the cell death-related pathways has been reported, and many of the overlapping pathways were found to participate in both the immune response and the cell death process [Citation40]. To identify the immunological role of the five cell death-related hub genes and their potential to improve the immunotherapy efficacy in various tumors, we explored the interaction between the five genes’ expression and immune system in different cancers from several aspects using multiple aspects online tools. One of the hallmarks of cancer is the immune cell infiltration of tumors [Citation41]. Previously, the strong correlation between immune cell infiltration and the progress of many tumors has been identified, such as bladder cancer, prostate cancer, and breast cancer [Citation42–44]. Of interest, Prizment et al. [Citation45] reported that the immune infiltrations of B cells and T cells existed in HNSC, and the degree of the infiltrating levels is associated with better survival of HNSC. In our study, we observed an extensive association between the five hub genes’ expression and the typical six immune cells’ immune infiltration levels (B cells, CD4 + T cells, CD8 + T cells, Dendritic cells, Macrophage, and Neutrophil) and multiple immune gene markers. This suggests that the five genes might interact with the immune cell infiltrations in regulating the carcinogenesis and prognosis of cancers.
As one of the promising biomarkers in immune-checkpoint inhibitors, MSI has an independent predictive value in predicting the immunotherapy efficacy of several cancers, such as colorectal and stomach cancers [Citation45–48]. This study observed statistical significance between each gene’s expression and MSI in specific cancers, such as COAD and LGG, which indicates that the five genes may affect patients’ response to immune checkpoint suppression therapy by affecting the MSI of the tumors. TME crucially participates in the carcinogenesis and development of multiple cancers, and the features of TME could act as the index for evaluating the response of cancer cells to immunotherapy [Citation49]. Based on the ESTIMATE immune scores, we found the expression of the five genes was significantly related to the immune cell content in the TME of several cancers. Moreover, in the era of precision medicine, immune checkpoint therapy is seen as a promising and pillar strategy for treating cancer [Citation50]. In our research, we also noticed massive significant correlations between the expression of all the five genes and the expression of immune checkpoint genes in various cancers. Besides, it has been reported that the efficacy of immune checkpoint therapy could also be affected by the neoantigen heterogeneity [Citation51]. A similar statistical association between the five gene’s expression and the neoantigen counts, which could serve as an attractive target for cancer therapy due to its high cell specificity, was also observed in several specific cancers. According to numerous previous studies, TILs are associated with a better prognosis for many tumors [Citation52]. We also found an extensive association between the five hub genes’ expression and most of the TILs in various cancers, which indicates that these five genes may play an important part in cancer specific immune response by regulating TILs. Of notice, intense interaction was noticed between the five genes and TILs as well as three immunomodulators in LGG and ACC, suggesting they have value in immunotherapy. Taken together, we can conclude that the transcription levels of the cell death-related genes are significantly correlated to the immune infiltration of cancer cells. It may influence the progress and prognosis of various cancers by interacting with the immune microenvironment. However, further research is needed to verify this hypothesis. Based on the above findings, the expression of the five genes may propose new targets for developing immunosuppressants and improving the immunotherapy efficacy of cancers. Although the five hub genes were identified from the large and complex gene networks of six primary cell death-related pathways, some other cell death-related pathways were not included, such as ferroptosis. Therefore, we also discussed the role of 10 famous key genes (CASP8, RIPK1, RIPK3, MLKL, GPX4, SLC7A11, CASP1, GSDMD, BECN1, and ATG5) in other cell death pathways in 30 human tumors. Similarly, differential prognosis-related expression of the key genes in many cancers were observed, including LGG and ACC. And multiple co-expression between the five major cell death-related hub genes and other famous key genes in other cell death pathways in LGG and ACC suggested the important role of cell death-related genes in these cancers.
Several limitations should be recognized in the current study. First, since the current comprehensive analysis was performed based on multiple online databases, the data heterogeneity would inevitably exist, which could reduce the reliability of our results. Second, the current research is a pan-cancer analysis based on data from 33 human tumors, including prognostic and immunological aspects, it is challenging to verify our results with experiments simultaneously. Further extensive clinical studies and experimental research in vitro /in vivo are required to validate their clinical values in cancers.
5. Conclusions
Our concentrative and comprehensive pan-cancer study of the five cell death-related hub genes indicates these factors are differentially expressed between cancer and normal samples and disclose significant correlations of the five gene’s expression with genetic alterations and DNA methylation. Our research also suggests that the five genes have the potential to be prognostic biomarkers in various cancers. Particularly, we found that JUN, NFKB1, CASP3, and TP53 have prognostic values in LGG, while PARP1 and CASP3 have prognostic values in ACC. Close associations exist between the five genes’ expression and the immune cell infiltration, MSI, immune scores, immune checkpoint genes’ expression, neoantigen counts, TILs, and immunomodulators in a variety of cancers, including LGG and ACC. These results could help us understand the multimolecular characteristics and immune role of the five cell death-related hub genes across human cancers and provide a novel insight for developing a more precise and personalized immunosuppressants therapy strategy.
Authors’ contributions
FW conceived the study idea. DH collected the data. TZ, ZY, LW, YW, NM, BT, YT, ZL, XL, YL, XR, YZ, and FW contributed to the analysis of the data. DH, TZ, ZY, LW, and YW wrote the initial draft with all authors providing critical feedback and edits to subsequent revisions. All authors approved the final draft of the manuscript. All authors are accountable for all aspects of the work in ensuring that questions related to the accuracy or integrity of any part of the work are appropriately investigated and resolved. FW is the guarantor. The corresponding author attests that all listed authors meet authorship criteria and that no others meeting the criteria have been omitted.
Ethics statements
Ethical approval for our study was granted by The Committee on Medical Ethics of The First Affiliated Hospital of Anhui Medical University (Reference number: Quick-PJ2021-09-14). Since all the data used in the current study was available online, and no individual patient was involved, it could be confirmed we have obtained all the written informed consent.
Supplemental Material
Download Zip (38.5 MB)Acknowledgments
We thank all the people who offer help for this study
Disclosure statement
No potential conflict of interest was reported by the author(s).
Data availability statement
The authors confirm that the data supporting the findings of this study are available within the article and its supplementary materials.
Supplementary material
Supplemental data for this article can be accessed online at https://doi.org/10.1080/15384101.2022.2101337
Additional information
Funding
References
- Fang Y, Tian S, Pan Y, et al. Pyroptosis: a new frontier in cancer. Biomed Pharmacother. 2020;121:109595.
- Yu J, Zhong B, Xiao Q, et al. Induction of programmed necrosis: a novel anti-cancer strategy for natural compounds. Pharmacol Ther. 2020;214:107593.
- Fulda S. Regulation of cell death in cancer-possible implications for immunotherapy. Front Oncol. 2013;3:29.
- D’Arcy MS. Cell death: a review of the major forms of apoptosis, necrosis and autophagy. Cell Biol Int. 2019;43(6):582–592.
- Shi J, Gao W, Shao F. Pyroptosis: gasdermin-mediated programmed necrotic cell death. Trends Biochem Sci. 2017;42(4):245–254.
- Fernandes-Alnemri T, Wu J, Yu JW, et al. The pyroptosome: a supramolecular assembly of ASC dimers mediating inflammatory cell death via caspase-1 activation. Cell Death Differ. 2007;14(9):1590–1604.
- Cerella C, Teiten MH, Radogna F, et al. From nature to bedside: pro-survival and cell death mechanisms as therapeutic targets in cancer treatment. Biotechnol Adv. 2014;32(6):1111–1122.
- Kerr JF, Searle J. A mode of cell loss in malignant neoplasms. J Pathol. 1972;106(1): Pxi.
- Levine B, Kroemer G. Autophagy in the pathogenesis of disease. Cell. 2008;132(1):27–42.
- Mizushima N, Levine B, Cuervo AM, et al. Autophagy fights disease through cellular self-digestion. Nature. 2008;451(7182):1069–1075.
- Nixon RA, Yang DS. Autophagy failure in Alzheimer’s disease–locating the primary defect. Neurobiol Dis. 2011;43(1):38–45.
- Ouyang L, Shi Z, Zhao S, et al. Programmed cell death pathways in cancer: a review of apoptosis, autophagy and programmed necrosis. Cell Prolif. 2012;45(6):487–498.
- Fink SL, Cookson BT. Apoptosis, pyroptosis, and necrosis: mechanistic description of dead and dying eukaryotic cells. Infect Immun. 2005;73(4):1907–1916.
- Tomczak K, Czerwinska P, Wiznerowicz M. The Cancer Genome Atlas (TCGA): an immeasurable source of knowledge. Contemp Oncol (Pozn). 2015;19(1A):A68–A77.
- Vivian J, Rao AA, Nothaft FA, et al. Toil enables reproducible, open source, big biomedical data analyses. Nat Biotechnol. 2017;35(4):314–316.
- Tang Z, Li C, Kang B, et al. GEPIA: a web server for cancer and normal gene expression profiling and interactive analyses. Nucleic Acids Res. 2017;45(W1):W98–W102.
- Gao J, Aksoy BA, Dogrusoz U, et al. Integrative analysis of complex cancer genomics and clinical profiles using the cBioPortal. Sci Signal. 2013;6(269):l1.
- Li T, Fan J, Wang B, et al. Timer: a web server for comprehensive analysis of tumor-infiltrating immune cells. Cancer Res. 2017;77(21):e108–e110.
- Angel P, Hattori K, Smeal T, et al. The jun proto-oncogene is positively autoregulated by its product, Jun/AP-1. Cell. 1988;55(5):875–885.
- Boettcher S, Miller PG, Sharma R, et al. A dominant-negative effect drives selection of TP53 missense mutations in myeloid malignancies. Science. 2019;365(6453):599–604.
- Donoghue S, Baden HS, Lauder I, et al. Immunohistochemical localization of caspase-3 correlates with clinical outcome in B-cell diffuse large-cell lymphoma. Cancer Res. 1999;59(20):5386–5391.
- Estrov Z, Thall PF, Talpaz M, et al. Caspase 2 and caspase 3 protein levels as predictors of survival in acute myelogenous leukemia. Blood. 1998;92(9):3090–3097.
- Faderl S, Thall PF, Kantarjian HM, et al. Caspase 2 and caspase 3 as predictors of complete remission and survival in adults with acute lymphoblastic leukemia. Clin Cancer Res. 1999;5(12):4041–4047.
- Kaltschmidt B, Kaltschmidt C, Hofmann TG, et al. The pro- or anti-apoptotic function of NF-kappaB is determined by the nature of the apoptotic stimulus. Eur J Biochem. 2000;267(12):3828–3835.
- Lee MH, Na H, Kim EJ, et al. Poly(ADP-ribosyl)ation of p53 induces gene-specific transcriptional repression of MTA1. Oncogene. 2012;31(49):5099–5107.
- Sun Y, Chen XY, Liu J, et al. Differential caspase-3 expression in noncancerous, premalignant and cancer tissues of stomach and its clinical implication. Cancer Detect Prev. 2006;30(2):168–173.
- Winter RN, Kramer A, Borkowski A, et al. Loss of caspase-1 and caspase-3 protein expression in human prostate cancer. Cancer Res. 2001;61(3):1227–1232.
- Zhao X, Li D, Huang D, et al. Risk-associated long noncoding RNA FOXD3-AS1 inhibits neuroblastoma progression by repressing PARP1-mediated activation of CTCF. Mol Ther. 2018;26(3):755–773.
- Croce CM. Causes and consequences of microRNA dysregulation in cancer. Nat Rev Genet. 2009;10(10):704–714.
- Basu S, Ma R, Mikulla B, et al. Apoptosis of human carcinoma cells in the presence of inhibitors of glycosphingolipid biosynthesis: i. treatment of colo-205 and SKBR3 cells with isomers of PDMP and PPMP. Glycoconj J. 2004;20(3):157–168.
- Yoo J, Kim CH, Song SH, et al. Expression of caspase-3 and c-myc in non-small cell lung cancer. Cancer Res Treat. 2004;36:303–307.
- Okouoyo S, Herzer K, Ucur E, et al. Rescue of death receptor and mitochondrial apoptosis signaling in resistant human NSCLC in vivo. Int J Cancer. 2004;108:580–587.
- Robles AI, Harris CC. Clinical outcomes and correlates of TP53 mutations and cancer. Cold Spring Harb Perspect Biol. 2010;2(3):a1016.
- Pettitt SJ, Krastev DB, Brandsma I, et al. Genome-wide and high-density CRISPR-Cas9 screens identify point mutations in PARP1 causing PARP inhibitor resistance. Nat Commun. 2018;9:1849.
- Voce DJ, Schmitt AM, Uppal A, et al. Nfkb1 is a haploinsufficient DNA damage-specific tumor suppressor. Oncogene. 2015;34:2807–2813.
- Soung YH, Lee JW, Kim SY, et al. Somatic mutations of CASP3 gene in human cancers. Hum Genet. 2004;115:112–115.
- Lukey MJ, Greene KS, Erickson JW, et al. The oncogenic transcription factor c-Jun regulates glutaminase expression and sensitizes cells to glutaminase-targeted therapy. Nat Commun. 2016;7:11321.
- Anteneh H, Fang J, Song J. Structural basis for impairment of DNA methylation by the DNMT3A R882H mutation. Nat Commun. 2020;11:2294.
- Van Baak TE, Coarfa C, Dugue PA, et al. Epigenetic supersimilarity of monozygotic twin pairs. Genome Biol. 2018;19:2.
- Weiss EM, Wunderlich R, Ebel N, et al. Selected anti-tumor vaccines merit a place in multimodal tumor therapies. Front Oncol. 2012;2:132.
- Kalafati L, Kourtzelis I, Schulte-Schrepping J, et al. Innate immune training of granulopoiesis promotes anti-tumor activity. Cell. 2020;183:771–785.
- Shao N, Tang H, Mi Y, et al. A novel gene signature to predict immune infiltration and outcome in patients with prostate cancer. Oncoimmunology. 2020;9:1762473.
- Efstathiou JA, Mouw KW, Gibb EA, et al. Impact of immune and stromal infiltration on outcomes following bladder-sparing trimodality therapy for muscle-invasive bladder cancer. Eur Urol. 2019;76:59–68.
- Ali HR, Chlon L, Pharoah PD, et al. Patterns of immune infiltration in breast cancer and their clinical implications: a gene-expression-based retrospective study. Plos Med. 2016;13:e1002194.
- Prizment AE, Vierkant RA, Smyrk TC, et al. Cytotoxic T cells and granzyme B associated with improved colorectal cancer survival in a prospective cohort of older women. Cancer Epidemiol Biomarkers Prev. 2017;26:622–631.
- Kim CG, Ahn JB, Jung M, et al. Effects of microsatellite instability on recurrence patterns and outcomes in colorectal cancers. Br J Cancer. 2016;115:25–33.
- An C, Choi IS, Yao JC, et al. Prognostic significance of CpG island methylator phenotype and microsatellite instability in gastric carcinoma. Clin Cancer Res. 2005;11:656–663.
- Lee DW, Han SW, Bae JM, et al. Tumor mutation burden and prognosis in patients with colorectal cancer treated with adjuvant fluoropyrimidine and oxaliplatin. Clin Cancer Res. 2019;25:6141–6147.
- Lei X, Lei Y, Li JK, et al. Immune cells within the tumor microenvironment: biological functions and roles in cancer immunotherapy. Cancer Lett. 2020;470:126–133.
- Sharma P, Allison JP. The future of immune checkpoint therapy. Science. 2015;348:56–61.
- Sato H, Jeggo PA, Shibata A. Regulation of programmed death-ligand 1 expression in response to DNA damage in cancer cells: implications for precision medicine. Cancer Sci. 2019;110:3415–3423.
- Galon J, Costes A, Sanchez-Cabo F, et al. Type, density, and location of immune cells within human colorectal tumors predict clinical outcome. Science. 2006;313:1960–1964.