ABSTRACT
Extracellular matrix (ECM), as an important framework for tumor microenvironment, plays important roles in many critical processes, including tumor growth, invasion, immune suppression, and drug resistance. However, few biomarkers of ECM-related genes (ERGs) have been developed for prognosis prediction and clinical treatment of bladder cancer (BC) patients. Bioinformatics analysis and LC-MS/MS analysis were used to screen differentially expressed ERGs in BC. Multivariate Cox regression analysis and Lasso regression analysis were used to construct and validate an ERGs-based prognostic prediction model for BC. Immunohistochemistry was used to detect the protein expression of hub gene-COL6A1 in BC patients. Using bioinformatics analysis from The Cancer Genome Atlas (TCGA) database and proteomic analysis from our BC cohort, we constructed and validated an effective prognostic prediction model for BC patients based on four differentially expressed ERGs (MAP1B, FBN1, COL6A1, and MFAP5). Moreover, we identified human collagen VI-COL6A1 was a hub gene in this prognostic prediction model and found that COL6A1 was closely related to malignancy progression, prognosis, and response to PD-1 inhibitor immunotherapy in BC. Our findings highlight the satisfactory predictive value of ECM-related prognostic models in BC and suggested that COL6A1 may be a potential biomarker in predicting malignant progression, prognosis, and efficacy of immunotherapy in BC.
1. Introduction
Bladder cancer is one of the most common malignant tumors in the urinary system, which directly threatens human health and survival [Citation1]. In recent years, immune checkpoint inhibitor (ICI) immunotherapy has brought a new dawn to patients with advanced and metastatic BC, while still facing the difficulties of low response rates and a lack of reliable prognostic predictors [Citation2]. Therefore, it is urgent and challenging to seek novel biomarkers for the prediction of disease prognosis and response to the immunotherapy in BC.
Recent studies have shown that the interaction between tumor cells and the tumor microenvironment (TME) is a necessary condition for the development and progression of malignant tumors [Citation3]. TME is a local internal environment in tumors composed of extracellular matrix (ECM), soluble molecules, and tumor stromal cells [Citation4]. Among them, ECM, as an important framework of TME, plays important roles in many critical processes, including tumor growth, invasion, immune suppression, and drug resistance [Citation5]. However, few biomarkers of ECM-related genes (ERGs) have been developed for prognosis prediction and clinical treatment of BC patients.
Here in this study, we innovatively combined proteomic analysis from our BC cohort with bioinformatics analysis from The Cancer Genome Atlas (TCGA) database, constructed and validated an effective prognostic prediction model for BC patients based on differentially expressed ERGs, making the research results more accurate and reliable. Moreover, to our knowledge, we first identified human collagen VI-COL6A1 was a hub gene in the ERGs-based prognostic signature of BC and found that COL6A1 was closely related to malignancy progression, prognosis, and response to PD-1 inhibitor immunotherapy in BC.
2. Materials and methods
2.1 Data collection and differentially expressed ERGs identification
RNA-seq expression and clinical information such as gender, age, stage, T, N, M, overall survival (OS), and survival status of BC patients were downloaded from The Cancer Genome Atlas (TCGA) database (https://tcga‐data.nci.nih.gov/tcga/). The TCGA cohort contains 414 BC patients and detailed clinical characteristics are described in supplementary . ERGs were identified by screening from Molecular Signature Database (MsigDB), and R software was used to identify differentially expressed genes, with false discovery rate (FDR) < 0.05 and |log2FC| > 1 as the selection criteria.
Table 1. Correlation between clinicopathological features and COL6A1 expression of the 71 BC patients in our cohort.
2.2 Proteomic analysis by LC-MS/MS analysis
To further distinguish differentially expressed ERGs at the protein level, tissue samples from 10 BC patients who received radical cystectomy without radiotherapy, chemotherapy, and neoadjuvant therapy before surgery in the Affiliated Hospital of Qingdao University were collected. All patients provided written informed consent before participation and the research protocol was approved by the Ethical Committee of the Affiliated Hospital of Qingdao University.
Proteomic analysis was carried out by liquid chromatography-mass spectrometry and mass spectrometry (LC-MS/MS) analysis to analyze the expression levels of various proteins in BC tissues and normal tissues. It includes protein extraction, trypsin digestion, HPLC separation, TMT/iTRAQ labeling, LC-MS/MS analysis, database searches, and bioinformatics methods. The threshold ratio was set to 1.2 to distinguish between the up- and down-regulated proteins in BC.
2.3 Construction of ERGs-based prognostic prediction model
R software package “survival” was used to perform univariate Cox regression analysis to determine the prognosis-related ERGs. These genes were included in a Lasso-Cox model for cross-validation to establish an ERGs-based prognostic signature. The risk score was calculated with “glmnet” R-package, and patients in the cohort were divided into high- and low-risk groups based on the median risk score. Kaplan–Meier analysis was used to compare the overall survival (OS) of high- and low-risk BC patients. The accuracy of the signature was determined by the area under ROC curve (AUC) values. Furthermore, patients with complete information on clinical characteristics were selected to assess the independence of the signature in prognosis prediction. Differential analyses of the risk score between subgroups of each clinicopathological parameter were performed using the Wilcoxon test to examine the effect of the signature on tumor progression.
2.4 Identification and validation of the hub gene
Differentially expressed ERGs were further put into the Search Tool for the Retrieval of Interacting Gene (STRING, https://string-db.org/) to construct protein–protein interaction (PPI) networks. Cytoscape was used to screen the PPI network and identify the hub gene in the PPI network. Then, the TCGA BC cohort (TCGA-BLCA) and GEO BC cohort (GSE13507) were further used to validate the correlation between the hub gene expression and prognosis in BC patients.
2.5 Immunohistochemistry (IHC)
Tissue samples were collected from 71 patients with primary BC who underwent surgery from January 2017 to December 2021 and 58 patients with advanced or metastatic BC who received immunotherapy of PD-1 inhibitor (Tislelizumab) from January 2020 to December 2021 in the Affiliated Hospital of Qingdao University. Informed consent was acquired from all patients, and the research protocol was approved by the Ethics Committee of the Affiliated Hospital of Qingdao University. In the cohort of 71 BC patients, no patients received adjuvant chemotherapy, radiotherapy, or immunotherapy before surgery, and follow-up was performed every 3 months in the first year, every 6 months for the next 2 years and once per year thereafter. All clinical information is summarized in . In the cohort of 58 patients treated with Tislelizumab, Tislelizumab monotherapy (200 mg, every 3 weeks) were used for treatment in 28 patients, whereas 30 patients received a combination of Tislelizumab plus platinum-based chemotherapy. Tumor responses were assessed according to the immune-modified Response Evaluation Criteria in Solid Tumors (iRECIST 1.1) and included complete response (CR), partial response (PR), stable disease (SD), and progressive disease (PD). ORR was defined as the proportion of the sum of CR and PR in the total. DCR was defined as the proportion of the sum of CR, PR, and SD in the total. All clinical information is summarized in .
Table 2. Clinical information, efficacy evaluation, and COL6A1 expression of the 58 BC patients treated with Tislelizumab in our cohort.
Paraffin-embedded tissue sections were incubated with COL6A1 polyclonal antibody (Proteintech Company, Chicago, USA) overnight after deparaffinization and microwave antigen retrieval. Immunostaining was performed by the avidin-biotin peroxidase method and counterstained with hematoxylin. Each sample was scored by two independent pathologists. Five fields of view were randomly selected and classified according to the degree of staining (0: negative staining, 1: pale yellow, 2: light brown, 3: dark brown) and the percentage of staining (0: 0%, 1: 1–25%, 2: 26–50%, 3: 51–75%, 4: 76–100%). The final score was the multiplication of the two scores and score ≥8 was considered positive.
3. Results
3.1 Identification of differentially expressed ERGs and construction of ERGs-based prognostic model for BC
To investigate the correlation between ERGs and prognosis of BC patients, we preliminarily screened out 225 differentially expressed ERGs between 414 BC tissues and 19 normal tissues from TCGA database and finally identified 71 differentially expressed ERGs in the protein expression level by LC-MS/MS proteomic analysis and the protein expression information is summarized in supplementary . Univariate Cox regression analysis showed that 24 differentially expressed ERGs(NCAM1、MAP1B、FBN1、COL6A1、HSPG2、CSPG4、COL6A3、GPX3、COL6A2、MFAP5、LUM、LAMA4、VCL、TNXB、DCN、OGN、CES1、ITGA1、SGCE、DPYSL3、SPON1、DPT、ANXA6、COL14A1)had significant prognostic value in BC (P < 0.05). Multivariate Cox and lasso regression analysis was used to construct a prognostic model, which contained four ERGs (MAP1B, FBN1, COL6A1, and MFAP5) and the coefficient of prognostic risk score as follows:(0.0239×MAP1B’s expression level)+(0.0045×FBN1”s expression level)+(5.11199876083267e-05×COL6A1”s expression level)+(0.0017×MFAP5’s expression level). The K-M survival curve showed that the ERGs-based prognostic model was closely related to the OS of BC patients (p < 0.001) (). Patients with high-risk scores had a significantly shorter 5-year survival rate than patients with low-risk scores. ROC curve analysis showed that the AUC was 0.641, which indicated a superior predictive accuracy of the prognostic model ().
Figure 1. The risk survival curve of the model based on the 4 ECM differentially expressed genes was closely related to the overall survival rate, and the survival rate of the high-risk group was worse than that of the low-risk group (p = 1.369e-05)(a). The ROC curve was established based on the model constructed by the 4 ECM differentially expressed genes, and the area under the curve (AUC) was 0.641(b).
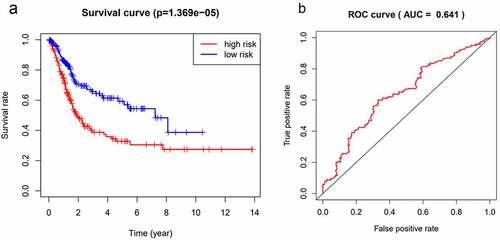
The heat map indicated that the risk scores of the model were closely related to tumor grade, T, and N in BC patients (). We then assess the prognostic values of ERGs-based prognostic model and clinical characteristics using univariate and multivariate Cox regression. Univariate Cox regression analysis showed that age, grade, T, N, and risk score were significantly associated with BC survival (). Multivariate Cox regression analysis confirmed that the ERGs-based prognostic model was an independent prognostic factor for BC (P < 0.001) ().
Figure 2. Based on the ECM differential expression gene, the model risk grouping and clinical correlation thermograms showed that the 4 genes with high expression had high risk and were closely related to T stage, N stage and grades of BC. *P <0.05; **P <0.01; ***P <0.001(a). Univariate Cox regression analysis showed that age, grade, T stage, N stage and model risk score were significantly correlated with survival rate of BC: age (HR = 1.029, CI:1.001–1.058, p < 0.05), stage (HR = 1.778, CI:1.243–2.543, p < 0.05), T stage (HR = 1.696, CI:1.149–2.504, p < 0.05), N stage (HR = 1.547, CI:1.177–2.033, p < 0.05), model risk score (HR = 2.325, CI:1.542–3.506, p < 0.05) (b). Multivariate Cox regression analysis showed that the score was an independent risk factor for predicting the prognosis of BC (HR = 2.462, CI:1.537–3.944, P < 0.05) (c).
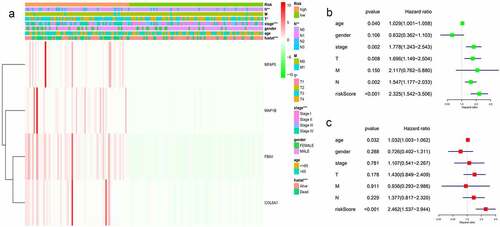
3.2 COL6A1 was a hub gene in the differentially expressed ERGs of BC
To explore the interaction of 24 differentially expressed ERGs in BC, the STRING database was used to construct the PPI network and Cytoscape was used to screen and identify the hub gene. We found that COL6A1 was the hub gene in the PPI network of 24 differentially expressed ERGs in BC (). We then validated the correlation between COL6A1 and prognosis in the TCGA-BLCA and GSE13507 cohort. The results showed that COL6A1 was highly expressed in BC tissue compared with normal bladder tissue () and patients with high expressions of COL6A1 had a significantly worse OS rate compared to patients with low expressions of COL6A1 (p = 0.044) ().
Figure 3. The STRING database showed that COL6A1 was a hub gene in protein–protein interaction network analysis (PPI) (a). COL6A1 was highly expressed in BC tissues, and COL6A1 expression was significantly higher in tumor tissues than in normal tissues in TCGA-BLCA cohort and GSE13507 cohort (p = 1.355e-04) (b). Survival analysis showed that the overall survival rate of patients with high COL6A1 expression in TCGA-BLCA cohort and GSE13507 cohort was poor (P = 0.044) (c).
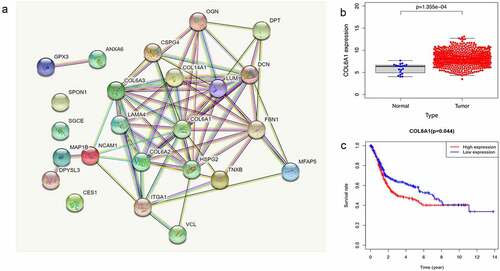
3.3 COL6A1 expression was correlated with clinicopathologic outcomes and prognosis of BC
We further validated the protein expressions of COL6A1 in 71 BC patients from our cohort by IHC and observed that COL6A1 was aberrantly expressed in 70.42% (50/71) of BC tissues (). To evaluate the role of COL6A1 expression in BC, the correlations between COL6A1 expression and clinicopathological features were analyzed and summarized in . We found that COL6A1 expression had no association with age, gender, and T and N stages. However, the expression of COL6A1 in metastatic BC tissues was significantly higher than that in non-metastatic BC tissues, indicating that COL6A1 may play an important role in the metastasis of BC (). Furthermore, after dividing 71 BC patients into high- and low-expression groups according to the median COL6A1 expression level, the K-M survival analysis showed a worse OS in the high-expression group than that in the low-expression group (p = 0.034), demonstrating that COL6A1 has potential value in predicting the prognosis of BC ().
3.4 High expression of COL6A1 predicted poor response to PD-1 inhibitor immunotherapy in BC
To evaluate the possible predictive value of COL6A1 on clinical response to PD-1 inhibitor immunotherapy in BC, COL6A1 protein expressions were detected in 58 patients with advanced or metastatic BC who received Tislelizumab immunotherapy. There were 2 cases of CR, 14 cases of PR, 29 cases of SD, and 13 cases of PD. Interestingly, we found that the ORR was 53.8% and DCR was 84.6% in the low-expression group of COL6A1, while the ORR was 20% and DCR was 75.6% in the high-expression group of COL6A1. Fisher’s precision probability test showed that there was a significant statistical difference in ORR between the two groups (p = 0.031), but not in DCR (p = 0.711), suggesting that BC patients with high expression of COL6A1 had poor response to PD-1 inhibitor immunotherapy in the short-term curative effect and COL6A1 could be used as a potential predictor for clinical response to PD-1 inhibitor immunotherapy in BC.
4. Discussion
In recent years, studies have shown that ECM is a complex microenvironment composed of many biomolecular frameworks, which regulates the growth and function of tumor cells in different ways. At the same time, tumor cells can also remodel and modify ECM by abnormally expressing oncogenes to facilitate its growth, invasion, and metastasis [Citation6–9]. Thus, ECM remodeling is considered to be one of the characteristics of aggressive malignant tumors [Citation10]. In this study, we innovatively combined proteomic analysis with bioinformatics analysis and constructed a prognostic prediction model for BC patients based on differentially expressed ERGs. Our findings highlighted the satisfactory predictive value of ECM-related biomarkers and prognostic models in BC.
The proteins in the ECM are mainly composed of collagen, fibronectin, laminin, hyaluronic acid [Citation11]. Human collagen VI(COL6A1) is one of the most important ECM proteins, which consists of three main polypeptide chains (α1, α2, and α3) encoded by different genes, and the COL6A1 gene encodes the α1 polypeptide chain, often involved in tumor cell growth and metastasis [Citation12]. COL6A1 can contribute to the construction of local ECM microenvironment by forming a discrete network of bead-like microfilaments that interact with other ECM molecules, thus enhancing the extension, penetration, and invasion of tumor cells [Citation13]. It has been demonstrated that COL6A1 can activate multiple downstream signal pathways including JAK-STAT signal pathway, MAPK signal pathway, NOTCH signal pathway, p53 signal pathway, VEGF signal pathway, and WNT signal pathway, to promote tumor cell proliferation by interaction with other ECM receptors [Citation14–17]. Previous studies have shown that a high expression of COL6A1 was closely related to the metastasis and poor prognosis of patients with lung cancer, clear cell renal cell carcinoma, prostate cancer, cervical cancer, and so on [Citation18–21]. In this study, we identified COL6A1 as a hub gene in the ERGs-based prognostic signature of BC and confirmed that COL6A1 was an important prognostic risk gene in BC, which was closely related to the malignant progression and prognosis of BC. However, further studies are still warranted to illuminate the specific role and mechanisms of COL6A1 in BC.
Another interesting finding in this study was that high expression of COL6A1 predicted poor response to PD-1 inhibitor immunotherapy in BC. It has been demonstrated that ECM can participate in tumor immunosuppression by inhibiting tumor cell death and interfering with tumor antigen presentation, recognition, activation, migration, and killing activity of effector T cells [Citation17]. Moreover, recent studies have shown that tumor-associated ECM is one of the major barriers to successful cancer immunotherapy and targeting tumor-associated ECM has great potential in the growing field of combination immunotherapy for cancer [Citation22,Citation23]. Thus, our study highlights the potential value of COL6A1 in predicting clinical response to PD-1 inhibitor immunotherapy for BC.
There are still some limitations in our study. Firstly, the number of patients in this study cohort is insufficient, especially those receiving immunotherapy, which needs to be further verified in more data. Secondly, our study only focused on the expression and prognostic value of COL6A1 in patients with BC. Further studies are needed to clarify the mechanism of COL6A1 involved in malignancy progression, metastasis, and response to PD-1 inhibitor immunotherapy in BC.
5. Conclusion
COL6A1 may be a potential biomarker in predicting malignant progression, prognosis, and response to PD-1 inhibitor immunotherapy in BC.
Key messages
COL6A1 was highly expressed in BC tissue compared with normal bladder tissue and patients with high expressions of COL6A1 had a significantly worse OS rate compared to patients with low expressions of COL6A1;
The expression of COL6A1 in metastatic BC tissues was significantly higher than that in non-metastatic BC tissues, indicating that COL6A1 may play important role in the metastasis of BC;
Fisher’s precision probability test showed that there was a significant statistical difference in ORR between the two groups (p = 0.031), suggesting that BC patients with high expression of COL6A1 had poor response to PD-1 inhibitor immunotherapy in the short-term curative effect and COL6A1 could be used as a potential predictor for clinical response to PD-1 inhibitor immunotherapy in BC.
Authors’ contributions
XZZ: Conceptualization, Methodology, Data curation, Resources, Writing original draft, and Final approval. JL: Data curation, Formal analysis, Validation, Writing, Reviewing, Editing, and Final approval. XCY: Validation, Visualization, Resources, Writing, Reviewing, Editing, and Final approval. WJ: Resources, Writing, Supervision, Reviewing, Editing, and Final approval. CQS: Software, Resources, Writing, Reviewing, Editing, and Final approval. XZZ: Resources, Software, Writing, Reviewing, Editing, and Final approval. YHW: Conceptualization, Supervision, Project administration, Writing, Reviewing, Editing, and Final approval. All authors agree to be accountable for all aspects of the work.
Ethics approval
The studies involving human participants were reviewed and approved by the Ethical Review Committee of The Affiliated Hospital of Qingdao University. The patients/participants provided their written informed consent to participate in this study. Written informed consent was obtained from the individual(s) for the publication of any potentially identifiable images or data included in this article.
Supplemental Material
Download Zip (23.8 KB)Disclosure statement
No potential conflict of interest was reported by the author(s).
Data availability statement
Data and materials supporting the results are available upon reasonable request from the corresponding author Yonghua Wang ([email protected]).
Supplementary material
Supplemental data for this article can be accessed online at https://doi.org/10.1080/15384101.2022.2154551
Additional information
Funding
References
- L SR, D MK, E FH, et al. Cancer statistics, 2022[J]. Ca A Cancer J Clinicians. 2022;72(1):7–33. DOI:10.3322/caac.21708
- M DN, T LA, Holden S, et al. Immunotherapy for the treatment of urothelial carcinoma[j]. J Urol. 2017;197(1):14–22. DOI:10.1016/j.juro.2016.02.3005
- Cassetta L, Kitamura T. Macrophage targeting: opening new possibilities for cancer immunotherapy[j]. Immunology. 2018;155(3):285–293.
- R CO, Q CN, L SA, et al. Functional polarization of tumour-associated macrophages by tumour-derived lactic acid[j]. Nature. 2014;513(7519):559–563. DOI:10.1038/nature13490
- Chaudhuri O, Cooper-White J, A JP, et al. Effects of extracellular matrix viscoelasticity on cellular behaviour[j]. Nature. 2020;584(7822):535–546. DOI:10.1038/s41586-020-2612-2
- Harjunp H, L AM, Guenther C, et al. Cell adhesion molecules and their roles and regulation in the immune and tumor microenvironment[j]. Front Immunol. 2019;10:10.
- Pires A, Burnell S, Gallimore A. Exploiting ECM remodelling to promote immune-mediated tumour destruction. Curr Opin Immunol. 2022;74:32–38.
- Di Martino JS, Akhter T, Bravo-Cordero JJ. Remodeling the ECM: implications for metastasis and tumor dormancy[J]. Cancers (Basel). 2021;13(19):4916.
- Kaushik N, Kim S, Suh Y, et al. Proinvasive extracellular matrix remodeling for tumor progression[j]. Arch Pharm Res. 2019;42(1):40–47. DOI:10.1007/s12272-018-1097-0
- Nelson CM, Bissell MJ. Of extracellular matrix, scaffolds, and signaling: tissue architecture regulates development, homeostasis, and cancer[j]. Annual Review of Cell & Developmental Biology. 2006;22(1):287–309.
- Narunsky L, Oren R, Bochner F, et al. Imaging aspects of the tumor stroma with therapeutic implications[j]. Pharmacology & Therapeutics. 2014;141(2):192–208. DOI:10.1016/j.pharmthera.2013.10.003
- Tanaka T, Ikari K, Furushima K, et al. Genomewide linkage and linkage disequilibrium analyses identify COL6A1, on chromosome 21, as the locus for ossification of the posterior longitudinal ligament of the spine [j]. Am J Hum Genet. 2003;73(4):812–822. DOI:10.1086/378593
- Martoni E, Petrini S, Trabanelli C, et al. Characterization of a rare case of Ullrich congenital muscular dystrophy due to truncating mutations within the COL6A1 gene C-Terminal domain: a case report[j]. BMC Med Gene. 2013;14(1):14. DOI:10.1186/1471-2350-14-59
- Lin H, Yang Y, Hou C, et al. Identification of COL6A1 as the key gene associated with antivascular endothelial growth factor therapy in glioblastoma multiforme[j]. Genet Test Mol Biomarkers. 2021;25(5):334–345. DOI:10.1089/gtmb.2020.0279
- Fujita A, R SJ, Festa F, et al. Identification of COL6A1 as a differentially expressed gene in human astrocytomas[j]. Genet Mol Res. 2008;7(2):371–378. DOI:10.4238/vol7-2gmr432
- A SS, G AM, Jussila L, et al. Lymphangiogenesis and cancer metastasis[j]. Nat Rev Cancer. 2002;2(8):573–583. DOI:10.1038/nrc863
- L CJ, Shahi P, V TS, et al. FGFR1-WNT-TGF-β signaling in prostate cancer mouse models recapitulates human reactive stroma [j]. Cancer Research. 2014;74(2):609. DOI:10.1158/0008-5472.CAN-13-1093
- H CK, H CY, S WY, et al. Quantitative secretome analysis reveals that COL6A1 is a metastasis-associated protein using stacking gel-aided purification combined with iTRAQ labeling[j]. J Proteome Res. 2011;10(3):1110–1125. DOI:10.1021/pr1008724
- Wan F, Wang H, Shen Y, et al. Upregulation of COL6A1 is predictive of poor prognosis in clear cell renal cell carcinoma patients[j]. Oncotarget. 2015;6(29):27378. DOI:10.18632/oncotarget.4860
- P ZY, N WF, J SY, et al. Reactive stroma component COL6A1 is upregulated in castration-resistant prostate cancer and promotes tumor growth[j]. Oncotarget. 2015;6(16):14488. DOI:10.18632/oncotarget.3697
- Hou T, Tong C, Kazobinka G, et al. Expression of COL6A1 predicts prognosis in cervical cancer patients[j]. Am J Transl Res. 2016;8(6):2838.
- P HD, Jensen C, W KSL, et al. Blood-based extracellular matrix biomarkers are correlated with clinical outcome after PD-1 inhibition in patients with metastatic melanoma[j]. Journal for ImmunoTherapy of Cancer. 2020;8(2): DOI:10.1136/jitc-2020-001193.
- H PD, L RB, Diao L, et al. Collagen promotes anti-PD-1/PD-L1 resistance in cancer through LAIR1-dependent CD8+ T cell exhaustion[j]. Nat Commun. 2020;11(1):1–18. DOI:10.1038/s41467-020-18298-8