Abstract
Chronic obstructive pulmonary disease is a complex pulmonary disease that causes airflow obstruction in humans. To identify the core genes in COPD pathogenesis, seven diverse microarray datasets (GSE475, GSE1122, GSE1650, GSE3212, GSE8823, GSE37768, and GSE22148) were downloaded from the gene expression omnibus database. All the datasets were analyzed independently with the R/Bioconductor package to screen the differentially expressed genes (DEGs). The gene ontology and pathway enrichment analysis were performed for the acquired DEGs using DAVID (Database for Annotation, Visualization, and Integrated Discovery). Further protein-protein interaction network was constructed for the DEGs and their potential hub genes and sub-networks were identified using Cytoscape software. From the selected seven datasets, 188 overlapped DEGs were perceived eventually based on considering the repetitive genes between at-least one dataset. Gene Ontology analysis reveals that most of the DEGs were significantly enriched in immune response, inflammatory response, extracellular region, lipid binding, cytokine, and chemokine activity. Moreover, genes from the sub-network analysis were again submitted to the DAVID server to validate the results which uncover the Toll-like receptor signaling pathway was significantly enriched and all the genes present in this pathway were likewise detected as hub genes from Cytoscape software. CXCL9, CXCL10, CXCL11, CCL4, TLR7, and SPP1 hub genes in the toll-like receptor signaling pathway were explored in this study as potential biomarker genes associated with COPD pathogenesis.
Introduction
Chronic obstructive pulmonary disease (COPD) is a complex and progressive lung disease that causes airflow limitation in humans. COPD is the fourth leading cause of death globally but anticipated to be the third driving reason for death by the year 2020 [Citation1]. Six percent of deaths occurred during the year 2012 globally due to 3 million death rates of COPD. In low and middle-income countries, 328 million people were estimated to have deadly disease COPD [Citation2]. The Chronic airflow limitation is caused by two main conditions which are chronic bronchitis (mucus hypersecretion) and emphysema (parenchyma destruction). COPD is a multi-factorial disease, which is influenced by hereditary as well as environmental factors associated with it. Chronic bronchitis and emphysema involved in COPD cause mucus hypersecretion and parenchyma destruction in the lungs [Citation3]. Tobacco smoking is the major significant risk factor involved in the development of COPD [Citation4]. Though smoking cigarettes is the main cause of COPD, some nonsmokers also develop COPD due to the inhalation of noxious particles/gases into the lungs. Other risk factors in COPD incorporates outdoor air pollution, occupational exposure to dust and fumes, and exposure to second hand smoke [Citation5]. The genetic risk factor associated with COPD is alpha-1 antitrypsin deficiency. This alpha-1 antitrypsin protein protects the lung from neutrophil elastase which is a powerful enzyme causes damage in the lung tissues [Citation6]. Inflammation in COPD patients [Citation7,Citation8] is characterized by an increase in the number of inflammatory cells (neutrophils, macrophages, and T-lymphocytes) and the release of multiple inflammatory mediators (lipids, chemokines, cytokines, and growth factors).
The Global Initiative for Chronic Obstructive Lung Disease (GOLD) classifies COPD airflow constraint in four different stages I (Mild), II (Moderate), III (Severe), and IV (Very severe) COPD and suggests the treatment decisions for each category with a stepwise increase in therapy [Citation9]. Spirometry is the most commonly recognized test used to diagnose COPD. It measures the amount of air inhaled profoundly which is forced vital capacity (FVC) and the amount of air we exhale in one second which is forced expiratory volume (FEV1). Airflow limitation severity in chronic obstructive pulmonary disease pathology [Citation10] was shown in . Formerly COPD severity was measured purely on forced expiratory volume in one second (FEV1) which is a marker of airflow limitation however patients with similar FEV1 may have different phenotypes [Citation11]. Hence in 2011, the GOLD strategy developed the combined COPD assessment which assorts patients based on airflow limitation (FEV1), symptoms using modified Medical Resource Council (mMRC) dyspnea scale or COPD Assessment Test (CAT) score as well as exacerbation history [Citation9].
Smoking cessation is a key factor used to prevent COPD exacerbations [Citation12]. Bronchodilators, inhaled corticosteroids (ICS) and LABA/LAMA [Citation13] are the medications given for COPD patients to improve the health status and quality of life. COPD patients often experience viral exacerbations, among which rhinovirus, a single-stranded RNA virus considered as the most significant trigger responsible for COPD viral exacerbations [Citation14]. Antiviral drug targets of single-stranded ribonucleic acid (RNA) viruses cause chronic diseases in humans [Citation15]. RNA viruses associated with this chronic human diseases exhibit a major threat in public health globally with increased mortality [Citation16]. High-throughput RNA-sequencing based transcriptomics is the most popular statistical approach which can be utilized for biomarker detection of chronic human diseases. For drug discovery of chronic human diseases, biological network theory, and methodology were applied based on a protein–protein interaction network, drug-target network, and disease-gene network studies [Citation17].
There are several different causative components involved in COPD mechanism thus identifying specific biomarkers to aid the diagnosis of this progressive disease is important. This study aims to acquire the core genes involved in the pathogenesis of COPD at all possible extents. Microarray gene expression profiling of human diseased tissues may give new insights into the molecular pathogenesis of COPD and eventually lead to the identification of novel therapeutic targets [Citation18]. Identification of differentially expressed genes (DEGs) from gene expression omnibus (GEO) datasets may help to improve COPD diagnosis [Citation19] and treatments. In this study, we analyzed the seven diverse gene expression profiles of etiological samples which act as the significant cause for developing COPD. By utilizing the seven distinctive GEO datasets, DEGs were identified separately for each dataset. Out of which the overlapped genes were recognized and further gene ontology and pathway enrichment analysis were performed. Furthermore, using the constructed protein–protein interaction network, critical hub genes were also recognized for the obtained DEGs to elute out the potential core genes involved in the pathogenesis of COPD mechanism.
Materials and methods
Differentially expressed gene screening from gene expression omnibus datasets
Seven different gene expression series file (GSE475, GSE3212 [Citation20], GSE8823 [Citation21], GSE1122 [Citation22], GSE1650 [Citation23], GSE22148 [Citation24], and GSE37768) were selected for our study and downloaded from NCBI GEO database (https://www.ncbi.nlm.nih.gov/geo/). The sample types and platform information of each selected datasets are described in . The detailed clinical information of the samples of all seven datasets is given in . Raw microarray data in CEL format were loaded into the affy package of R and the chip annotations were downloaded. The data were preprocessed, background corrected, standardized and the expression data were generated using the GCRMA algorithm and executed in the Bioconductor (version 3.6) affy package of R (version 3.5.1; https://www.r-project.org/). The probe intensities were plotted by boxplots before and after normalization to check the effects of GC-RMA standardization. Low variance genes were removed from the data during the stage of uninformative probesets filtration. Limma package was used to mine the differentially expressed genes with a threshold of logFC > 1 and p-value < 0.05. The probes without corresponding gene symbols and the duplicates were removed. The above procedure was followed for every seven datasets separately.
Table 1. Selection of GEO datasets for chronic obstructive pulmonary disease.
Functional enrichment analysis of differentially expressed genes
Gene ontology analysis was applied in this study to investigate the biological function of the DEG’S [Citation25]. Gene ontology (GO) terms and Kyoto Encyclopedia of Genes and Genomes (KEGG) pathway analysis of the screened as well as repeated DEG’s were performed by the Functional Annotation Tool in the Database for Annotation, Visualization and Integrated Discovery (DAVID version 6.8) [Citation26,Citation27] which is a powerful tool for gene-function classification. The major GO terms (Biological Process, Cellular component and Molecular function) and enriched KEGG pathways were identified based on the cutoff criterion hypergeometric distribution count > 2 and p-value < 0.05.
Construction of protein-protein interaction network
All the repeated DEGs from seven datasets were submitted to the Search Tool for the Retrieval of Interacting Genes/Proteins (STRING) online database and a medium interaction score >0.04 was used as the cutoff basis to predict the interaction between differentially expressed genes. The constructed protein-protein interaction (PPI) network was exported in .tsv format from STRING and imported into Cytoscape (3.7.1 version) for visualization [Citation28] and hub gene identification. Hub proteins interact with a large number of partners play key roles in the diseases. The top 20 hub proteins were selected from repeatedly appearing proteins classified by cytoHubba plugin based on maximal clique centrality (MCC), degree, closeness, and betweenness measures. Network Analyzer plugin of Cytoscape was used to process the topological parameters of the PPIs to sort the protein functionality. Topological metrics like average clustering coefficient, degree of distribution of proteins, shortest path length, and topological coefficients were computed for the whole PPI network to find the molecular mechanisms of diseases [Citation29].
Sub-network clustering analysis
Sub-network analysis helps in determining the biological relationships between multiple genes present in the network. The biological process interacts with the genes to play a regulatory role. In common, those genes execute a similar or same biological function in the sub-networks [Citation30]. Highly interconnected regions in the PPI network were identified by the MCODE plugin. The node cutoff of 0.2, K-Core of 2, fluff-density cutoff of 0.2 and a maximum depth of 100 was initialized in the MCODE plugin. The sub-networks were then validated by subjecting them to gene ontology identification and gene-functional clustering using the DAVID server [Citation29].
Survival analysis of hub genes
The online survival analysis tool, Kaplan Meier-plotter [Citation31] was utilized in this study to evaluate the prognostic value of the hub genes. To assess the prognostic value of the specific gene, the patient samples were categorized into high and low expression groups according to the median expression value of the hub gene. The hazard ratio with 95% confidence intervals and log rank p-value of each hub gene were calculated [Citation32].
Results
Identification of differentially expressed genes of COPD from different etiological samples
Differentially expressed gene screening from gene expression omnibus datasets provides new insights into the identification of disease-specific genes and complex regulatory networks. The work plan of the study design is shown in . Our aim of this study was to identify the core genes associated with the pathogenetic mechanism of COPD. We investigated seven distinctive GEO datasets (GSE475, GSE1122, GSE1650, GSE3212, GSE8823, GSE37768, and GSE22148) in R using Bioconductor package. The datasets were normalized using the GCRMA package and further subjected for differential expression analysis using limma. Two threshold criterion was applied for all the datasets which are logFc > 1, p-value < 0.05. Genes with positive logFC values were taken as upregulated genes whereas genes with negative logFC values were considered as downregulated genes. Likewise, all the DEG were segregated independently for all seven datasets. Duplicates and expression values lacking specific gene symbols were removed from the study. By comparing the different sample types, a total of 32, 47, 19, 64, 582, 55, 1219 potential DEGs were identified in GSE475, GSE1122, GSE1650, GSE3212, GSE8823, GSE37768, and GSE22148 datasets, respectively. The up- and down-regulated genes identified from seven diverse datasets are shown in Supplementary Table S3. Subsequently, when the DEGs were investigated for overlap between seven different datasets, a total of 386 DEGs () were obtained. Even though all the datasets were not highlighting particular DEG, many DEGs were overlapped between the smoker’s sample. Hence, DEG’s repeated between at-least one dataset were considered in this study to find the core genes involved in COPD. For finding commonly co-expressed genes, Bioinformatics and Evolutionary Genomics [Citation33] tool was utilized and the text output was saved. Using Bioinformatics and Evolutionary Genomics tool, we recognized 188 overlapped DEGs () between the different combinations of datasets, among which most noteworthy of 136 DEG were overlapped between GSE8823 and GSE22148 datasets. The results show that smoking plays a pivotal and effective role in COPD disease progression because the dataset GSE8823 comprises the smoker’s sample and GSE22148 comprise of the sputum sample at stage II, III, and IV COPD from COPD ex-smokers. Finally, the obtained 188 overlapped genes were found crucial and taken further for gene ontology and protein-protein interaction analysis.
Figure 1. Flow diagram of the study design. COPD: Chronic Obstructive Pulmonary Disease; GEO: Gene Expression Omnibus.
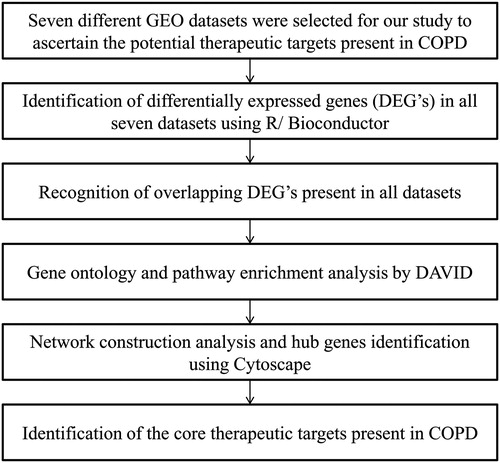
Table 2. The 188 overlapping genes were identified between each of the seven datasets.
Table 3. List of consistently repeated genes recognized between different combinations of GEO datasets.
Gene ontology and pathway enrichment analysis of DEG’s in COPD
Gene ontology analysis was performed for the 188 overlapped DEG with p value < 0.05 as the cutoff criterion to find out the biological functions involved in it. The obtained 188 aberrant genes were given as input in the functional annotation tool, DAVID and significantly enriched GO terms and KEGG pathways were categorized. GO terms were grouped into three functional groups: biological process (BP), cellular component (CC), and molecular function (MF) and their top 10 GO terms were investigated and presented in . In the biological process, the top three significantly enriched terms were signal transduction, immune response, and inflammatory response. Integral component of membrane, plasma membrane, and extracellular exosome were noted as the most significant terms in the cellular component. Meanwhile, molecular function of the DEGs was significantly enriched in cytokine activity, protein homodimerization activity and heparin binding. Furthermore, KEGG pathway analysis was performed to investigate the aberrant gene associated pathways based on the DEGs identified. There are five significant KEGG pathways acquired which are Cytokine–cytokine receptor interaction, extracellular matrix- receptor interaction, toll-like receptor signaling pathway, proteoglycans in cancer and hematopoietic cell lineage. To validate the significantly enriched pathways, we have also checked the KEGG pathways separately for the DEGs from seven datasets and found cytokine-cytokine receptor interaction and Toll-like receptor signaling pathways were present in GSE1122 and GSE8823 datasets. These outcomes suggest that along with smoking, emphysema and alpha-1 antitrypsin deficiency-related emphysema may play a crucial role in developing COPD.
Protein-protein interaction network and hub gene screening
The protein-protein interaction network is helpful in understanding the physiological activities of the cell and its internal and external response to environment. Cytoscape software was utilized to determine the biological relationships among the 188 differentially expressed genes [Citation30]. By using the STRING database, the protein-protein interaction of overlapped DEGs was established. The protein-protein interaction network was constructed using Cytoscape and comprised of 174 nodes and 296 edges among which 14 disconnected nodes were removed from the network. The differentially expressed genes used for protein-protein interaction network construction is 174. The PPI network was imported and visualized in Cytoscape and the hub genes were selected with the cutoff measure of degree ≥10. The top 20 hub genes were identified based on different algorithms with high centrality statistics like MCC, degree, closeness, and betweenness measures present in cytoHubba plugin and listed in . A total of 23 hub genes appeared almost in two categories demonstrating their significant function in maintaining the global network structure and communication [Citation29]. The frequently appeared hub genes are highlighted in . The majority of the hub genes play an active role in the immune and inflammatory response, cytokine, and chemokine activity, plasma and integral component of membrane, cell-cell signaling, signal transduction, and chemokine receptor binding. The modules were identified from the whole protein-protein interaction network comprised of 174 genes (nodes). The two highly interconnected sub-networks (modules) were extracted from the protein-protein interaction network using the MCODE plugin. Module 1 contains 22 hub genes in the network whereas module 2 contains 8 hub genes. In common, we found 7 hub genes (APOE, ESR1, SOD2, SPP1, THBS1, LAMB1, and SDC2) in both clusters 1 and 2 in the network which strongly suggests that those genes play a putative role in COPD. Genes present in these modules were mainly associated with negative regulation of inflammatory response, signal transduction, inflammatory response, extracellular matrix organization, chronic inflammatory response, cell adhesion, extracellular region, plasma membrane, extracellular exosome, cytokine activity, and lipoprotein particle binding. KEGG pathways involved in these aberrant genes are extracellular matrix receptor interaction and toll-like receptor signaling pathways. The protein-protein interaction network of 188 differentially expressed genes was displayed in . The topological parameters distribution using Cytoscape’s Network Analyzer plugin was shown in , where the number of interacting partners was measured by the degree of distribution of proteins. The average number of neighbors present in the PPI network is 4.9. The closely interlinked proteins were determined using the shortest path length parameter. Low average clustering co-efficient value shows the sparsely connected proteins as neighbors of densely associated proteins in the PPI network. Topological coefficient evaluates the range of each protein shares its neighbor with others [Citation29].
Figure 3. Protein–protein interaction network of filtered differentially expressed genes using Cytoscape software.
Two significant sub-networks (Module 1 & 2) were identified using the MCODE plugin. Nodes in dark blue color represent the DEG-encoded proteins of module 1, a node in red color represents the DEG-encoded protein of module 2, and nodes in green color represent the overlapped proteins between module 1 and 2.
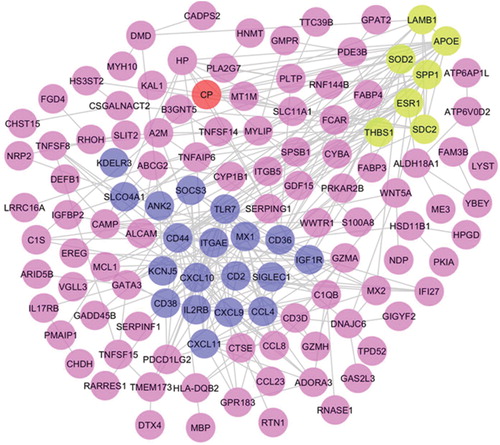
Figure 4. Topological properties of the central network (a) Average clustering coefficient, (b) Degree distribution of proteins, (c) Shortest path length distribution, (d) Topological coefficients.
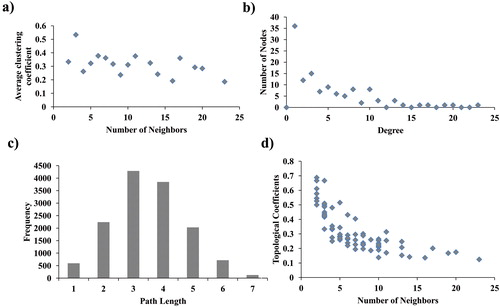
Table 4. Top 20 hub proteins identified using different algorithms by cytoHubba plugin.
Prognostic value of the obtained hub genes
To validate the obtained hub genes as potential biomarkers, Kaplan-Meier survival analysis was performed. Kaplan-Meier plotter online survival analysis tool for lung cancer is developed to identify the prognostic value of biomarkers related to survival which further helps us to understand the molecular mechanism of the disease [Citation34]. Non-small-cell lung cancer (NSCLC) samples were utilized in this study and a quality control check was performed for all the arrays to avoid potential bias. This tool is developed by following all the standard procedures and the Kaplan-Meier survival plot were employed in the tool using “Survival” package in R. The obtained COPD hub genes CXCL9 [Citation35], CXCL10 [Citation35], CXCL11 [Citation35], CCL4 [Citation36], TLR7 [Citation37], and SPP1 [Citation38] were also evidenced as potential biomarkers in various lung cancer studies. In Kaplan-Meier plotter, the mRNA lung cancer dataset was selected and the hub genes with the matching Affymetrix identifiers (matched with our COPD differentially expressed genes) were given as input. There are 1926 NSCLC patients were enrolled in the study for all the six hub genes. Non-small-cell lung cancer patients were divided into low and high-risk groups based on the median level. Kaplan-Meier method was employed to compare the overall survival ratio between two groups for all the six hub genes. The log rank p-value and hazard ratio (HR) with 95% confidence intervals (CI) were determined. The high expression levels of CXCL9, CXL10, CXCL11, CCL4, TLR7, and SPP1 in lung cancer patients were associated with poor overall survival. Out of the six hub genes, the log p-value of TLR7 and SPP1 gene was <0.05 and might be considered as a potential biomarker for lung cancer patients.
Discussion
Microarrays have been extensively applied to gene expression studies of various respiratory diseases, describing the genetic profiles of the disease [Citation39]. In the present study, gene expression data of seven diverse datasets were obtained from the GEO database for the screening of core genes associated with COPD. The previous studies on gene expression in COPD incorporate smokers dataset and other COPD related datasets but this study focuses on seven diverse etiological datasets that reveal more overlapped DEGs. Using multiple expression datasets, in combination with integrative bioinformatics methods becomes an innovative way to solve this problem. Therefore, a multiple-cohort integrative analysis with a relatively stringent sample filtering was applied in the present study [Citation39]. Gene expression omnibus (GEO) datasets were chosen from different sample types and platforms however all the experiment types are expression profiling by array. To obtain the potential key genes from all different COPD causative factors we have included different etiological sample types in our study. The selection criteria of GEO datasets in the study are as follows: The major important cause for COPD is cigarette smoking so we have picked GSE3212, GSE8823, and GSE37768 datasets, the control, and COPD diseased samples were deposited in GSE475, Emphysema plays a crucial role in developing COPD so we have chosen GSE1122 and GSE1650, COPD occurs in humans at different stages, treatment on each stage relies upon the severity of the disease. To check the differentially expressed genes (DEGs) in Stage II, III, & IV we have picked the GSE22148 dataset. GSE1122 dataset contains the genetic disorder alpha-1 antitrypsin deficiency-related emphysema samples which were also considered in our study. The differentially expressed genes were identified in each dataset independently using the R Affy/Bioconductor package. As an outcome, an overlap of 188 genes was identified. Gene overlapped in at-least two datasets were considered as the aberrant DEGs in this study.
Gene ontology and pathway enrichment analysis were performed for the 188 overlapped DEGs to decipher the biological functions associated with the respective genes. In gene ontology annotation, the most significant terms were well associated with immune response, inflammatory response, cell adhesion, cell surface, extracellular region, lipid binding, cytokine, and chemokine activity. Regarding the pathway enrichment, the majority of remarkably enriched terms were associated with the Toll-like receptor signaling pathway, cytokine-cytokine receptor interaction and extracellular matrix (ECM) receptor interaction in concurrence with the results of the gene ontology enrichment. Toll-like receptor (TLR) signaling pathway contains most of the recognized DEGs, which is a critical pathway in initiating immune responses upon stimulation [Citation40] and moreover assumes a significant role in inflammation, immune cell regulation, cell survival and proliferation [Citation41]. Toll-like receptor signaling activation is responsible for the development of several diseases such as autoimmune, chronic inflammatory, and allergic diseases [Citation42]. TLRs in humans participate in the activation of immune responses and each TLRs perceive specific antigens. TLRs are also expressed in resident lung cells such as alveolar macrophages and other invading cells. Numerous investigations confirm that impaired innate immunity contributes to the pathogenesis of COPD. The role of specific TLRs during inflammation has suggested their involvement in the pathogenesis of COPD [Citation43]. The toll-like receptor signaling pathway firmly linked to the pathophysiology of COPD was additionally affirmed due to its contribution to the disease [Citation44]. TLR2, TLR4, and TLR9 in COPD appeared to participate in the cigarette smoke-induced inflammatory responses [Citation45–47]. Therefore, our predicted result further proves that the Toll-like receptor signaling pathway has been involved in the pathogenic mechanism of COPD and it is considered as a crucial aberrant pathway in COPD pathophysiology.
To elucidate the complex biological processes, the interacting partners of the DEG may be useful to mine out the potential biomarkers of COPD. The string database helps curate known and predicted PPIs for constructing protein functional association networks [Citation39]. The protein-protein interaction network comprising of 174 nodes and 296 edges was established. Hub genes repeated in more than one parameter were considered in this study. CXCL10, TLR7, CXCL9, MX1, CXCL11, CD44, ITGAE, CCL4, CD38, SIGLEC1, SPP1, APOE, IL2RB, SDC2, LAMB1, CD2, ESR1, SOD2, CD36, CP, SOCS3, IGF1R, THBS1 were recognized from the protein-protein interaction network as major hub genes since it is rehashed in more than one algorithm. These 23 hub genes are also present in both modules 1 and 2. Furthermore using MCODE plugin, two significant modules were identified. Genes obtained from both the modules were again submitted to the DAVID server for validation of gene ontology and pathway analysis. As expected, an extracellular matrix receptor interaction pathway was represented in both modules 1 & 2 and also predominantly found in previously acquired 188 overlapped gene lists. The genes from module 1 retained the same toll-like receptor signaling pathway. In the toll-like receptor signaling pathway, all the six hub genes (CXCL9, CXCL10, CXCL11, CCL4, SPP1, and TLR7) have directly participated which confirms that these aberrant genes might play a critical role in COPD pathogenesis.
Chemokines are a group of small, secreted and heparin-binding proteins that are not only active as chemotactic factors but also as end-point effectors of immune protective functions. These proteins are classified into Cys-Xaa-Cys (CXC) and several other chemokines dependent on the position of characteristic structure determining cysteine residues within their N-terminal part [Citation48–51]. Chemokine ligands CXCL9, CXCL10, and CXCL11 is a small cytokine belonging to the CXC chemokine family exerting their action by means of CXC chemokine receptor-3 (CXCR3), a receptor highly expressed on activated T cells [Citation52,Citation53]. These IFN-γ induced chemokines are believed to be crucial in directing activated T cells to sites of inflammation where they assume an important role in Th1-mediated immune responses. These responses have been recently implicated in COPD pathogenesis.
CXCL9 and CXCL10 act on lung macrophages to secrete matrix metalloproteinase-12 which contributes to the lung inflammation and tissue destruction in COPD patients was also previously reported [Citation54]. Increased number of CXCL9, CXCL10, and CXCL11 in COPD lungs may contribute to the lung inflammation through the release of IFN-γ and furthermore by the release of proteolytic enzymes such as perforin and granzyme B which causes emphysematous changes in lungs. CXCL9 and CXCL10 are the most promising inflammatory signals recognized at higher levels in the serum of COPD patients. CXCL9, 10, and 11 acts on CXCR3+ cells to recruit inflammatory cells into the lungs, such as T lymphocytes, but CXCL11 seems to be also involved in lymphocyte clearance, emphasizing the possible dual role of chemokines in COPD [Citation55].
Monocytes/macrophages migration is mainly intervened by CC chemokines [Citation56]. CCL4 otherwise called as macrophage inflammatory protein-1β (MIP-1β) is a CC chemokine which aides in activating CCR5 receptor [Citation57]. CCL4 level is raised extensively during acute exacerbations in sputum samples of COPD patients [Citation58]. COPD severity increases with an increase in plasma concentration of CCL4 [Citation59]. An increase in macrophage inflammatory protein-1β in bronchoalveolar lavage fluid (BALF) and the level of airflow limitation indicates an involvement of MIP-1β in the progression of chronic obstructive pulmonary disease. MIP-1β levels are increased more in smokers affected with chronic bronchitis [Citation60]. Impact of chemokine receptors in COPD was illustrated in .
Figure 5. Role of chemokine receptors in COPD.
Innate immune cells such as macrophages, neutrophils, dendritic cells, CD4+ and CD8+ T lymphocytes express chemokines. The function of the chemokines is divided into two parts: Inflammatory and Homeostatic. The cc chemokine (CCL4) ligand attracts immune cells to inflammatory sites by involving in inflammatory response whereas the CXCR3 chemokine receptors (CXCL9, CXCL10, and CXCL11) participates in the cell migration during tissue development. The chemokine ligands CXCL9, CXCL10, and CXCL11 binds to the CXCR3 receptor and induced on activated T-helper (Th) 1 cell and CD8+ T lymphocytes at the sites of inflammation and leads to lung damage which eventually results in COPD. The CCL4 ligand from CCR5 receptor stimulates IL-2 and IFN-γ which causes severe airflow limitation and leads to COPD.
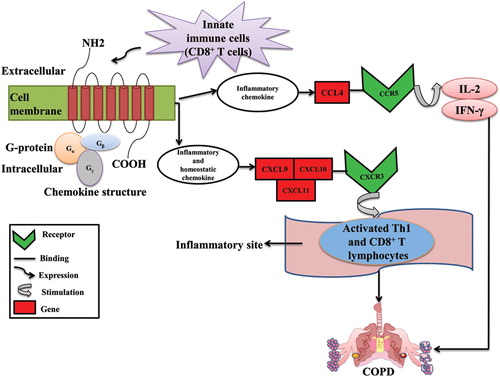
Secreted phosphoprotein 1 (SPP1) formerly known as osteopontin (OPN) is a multifunctional protein that intervenes in various biological functions and has been implicated in the pathogenesis of several chronic inflammatory diseases. OPN also functions as a Th1 cytokine, promotes cell-mediated immune responses and plays a key role in autoimmune and chronic inflammatory diseases [Citation61]. Increased SPP1 levels correlated with decreased lung function in smokers contrasted with nonsmokers [Citation62]. Elevated SPP1 levels in alveolar macrophages of smokers having normal lung function compared with nonsmokers indicates the inflammatory potential of SPP1, MMP-12, CCL2, CCR5 [Citation20,Citation62] in smokers.
Viral genomes are recognized by pattern recognition receptors including Toll-like receptors (TLRs) such as TLR3, TLR7, and TLR8 which are expressed on the cell surface and in the endosomes of immune and structure cells. The single-stranded RNA activates TLR7 which acts through MYD88 and TRAF6, leading to NF-kappa-B activation, cytokine secretion, and inflammatory response. TLR7 interacts with MYD88 via their respective TIR domains [Citation63]. TLR7/8 uses MyD88 molecular adaptor to activate the downstream signaling pathway [Citation64]. In COPD, whole lung tissue explants showed a greater pro-inflammatory response to TLR7/8 activation than control smokers [Citation65].
Toll-like receptors (TLRs) play a pivotal role in the pathophysiology of COPD and hindrance of TLR signaling is considered as a significant therapeutic approach in chronic inflammatory diseases [Citation66]. TLRs are class of proteins involved in innate immune responses and a family of pattern recognition receptors that respond to distinct molecules referred to as pathogen-associated molecular patterns (PAMPs) and damage-associated molecular patterns (DAMPs) [Citation67,Citation68]. These conserved receptors belong to type I transmembrane glycoproteins and made of three structurally significant components: the leucine-rich repeat motifs for ligand recognition at N-terminus; contains a single transmembrane helix; and a conserved cytoplasmic Toll/Interleukin I (IL-1) receptor (TIR) at C-terminus for intracellular signaling transduction [Citation69,Citation70]. There are 13 TLRs recognized as of now, among which TLR1-10 are expressed in humans [Citation68]. The expression of TLRs can be found in various immune (e.g. dendritic cells, monocytes, macrophages, and B lymphocytes) and nonimmune cells (e.g. epithelial cells, endothelial cells, and fibroblasts) [Citation71]. The TLR signaling pathway are categorized [Citation42,Citation72] in two cascades: through myeloid differentiation factor 88 (MyD88) adaptor protein and TIR-domain-containing adaptor-inducing interferon-β (TRIF), otherwise called MyD88-independent pathway, to activate interferon (IFN) regulatory factors (IRFs) and NF-κB for the production of type I IFNs. Elevated levels of TLRs in the lung, including monocyte and neutrophil infiltration may contribute to the development of COPD. Synergistic interactions of TLRs between airway smooth muscle and monocytes cause up-regulation of cytokine release through IL-1 which are crucial in the maintenance of inflammation in COPD [Citation73]. Few studies confirmed that TLR2 [Citation45], TLR4 [Citation46], and TLR9 [Citation47] proteins participated in cigarette smoke-induced inflammatory responses. TLR7 signaling and OPN function in COPD is displayed in .
Figure 6. Toll-like receptor 7 signaling and role of osteopontin in COPD.
Plasmacytoid dendritic cells express TLR7 from the endosomal compartment which recognizes single-stranded RNA from viruses and signals the adaptor molecule MyD88 in the presence of a ligand. TLR7-MYD88 complex activates IRAK4 in its death domain which phosphorylates and activates IRAK1. The activated IRAK1 recruits TRAF6 and mediated IRF7 activation upon phosphorylation by initiating innate immune responses. IKK-α and OPN are critically involved in IRF7 activation and type I interferon production because insufficient osteopontin expression leads to defective nuclear translocation of IRF7 in plasmacytoid dendritic cells. These TLR7 signaling finally leads to the release of inflammatory cytokines/type I interferons and results in COPD.
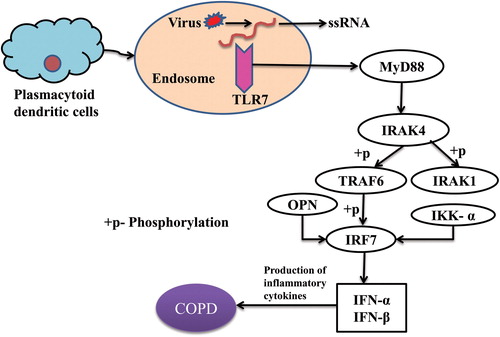
The outcomes from survival analysis further prove that all the six hub genes were associated with poor overall survival. It is worth noting that the log rank p-value of TLR7 and SPP1 gene was within the cutoff criterion (p < 0.05) and these genes may serve as a potential prognostic biomarker for lung cancer patients. Overall survival analysis of the six hub genes was displayed in . All these genes were also identified as aberrant hub genes in COPD and may likewise act as potential biomarkers in COPD patients as well since COPD and lung cancer [Citation74] are closely linked diseases.
Figure 7. Kaplan-Meier survival analysis of the obtained six hub genes. Prognostic value of (a) CXCL9, (b) CXCL10, (c) CXCL11, (d) CCL4, (e) TLR7, and (f) SPP1 were evaluated using the Kaplan-Meier plotter. Black represents genes with low expression values and red represents genes with high expression values.
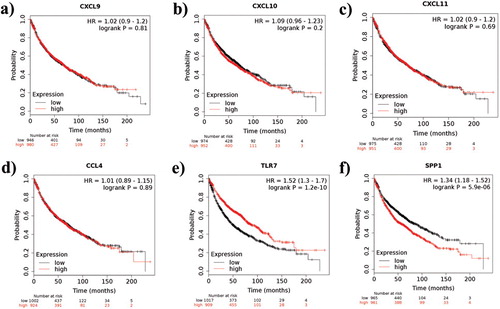
Together, the outcomes support the reliability of the functional analysis of DEGs and propose these hub genes as promising biomarkers for COPD. Although the DEG list derived from the seven datasets were not identical, the uniqueness is explicable. First, different detecting samples and platforms may partly represent the contrasts between the outcomes from the seven datasets in the microarray data. Second, the absence of probes in certain datasets may bring about less recognized DEGs in the present investigation. The 188 overlapped DEGs were found common in all seven datasets and furthermore no specific DEGs were identified in all seven datasets. However, DEGs overlapped in more than one dataset were considered. This might be because of the diverse genetic background, different samples, and different platforms used on multiple gene expression profiles.
Conclusion
Bioinformatics analysis of microarray gene expression data reveals COPD biomarker genes in this study by utilizing the systems biology approach. The present study using multiple GEO datasets mined out CXCL9, CXCL10, CXCL11, CCL4, TLR7, and SPP1 genes as the potential biomarker genes for COPD. These genes may contribute to COPD disease-specific diagnosis and therapy. All these genes have directly participated in the toll-like receptor signaling pathway which confirms their potential role in developing COPD. Chemokine receptors, toll-like receptor 7 and the osteopontin gene were recognized in this study as significant targets associated with COPD pathogenesis.
Abbreviations | ||
NCBI | = | National Center for Biotechnology Information |
GEO | = | Gene Expression Omnibus |
GCRMA | = | Gene Chip Robust Multi Array Averaging |
MCODE | = | Molecular Complex Detection |
CD4 | = | Cluster of Differentiation 4 |
CD8 | = | Cluster of Differentiation 8 |
CXCR3 | = | C-X-C motif Chemokine Receptor 3 |
CXCL9 | = | C-X-C motif Chemokine Ligand 9 |
CXCL10 | = | C-X-C motif Chemokine 10 |
CXCL11 | = | C-X-C motif Chemokine 11 |
IFN-γ | = | Interferon Gamma |
CCR5 | = | CC Chemokine receptor 5 |
CCL4 | = | Chemokine (C-C motif) Ligand 4 |
IL-2 | = | Interleukin-2 |
IRF7 | = | Interferon Regulatory Factor 7 |
IRAK1 | = | Interleukin-1 Receptor-Associated Kinase 1 |
IRAK4 | = | Interleukin-1 Receptor-Associated Kinase 4 |
TLR2 | = | Toll-like Receptor 2 |
TLR3 | = | Toll-like Receptor 3 |
TLR4 | = | Toll-like Receptor 4 |
TLR6 | = | Toll-like Receptor 6 |
TLR7 | = | Toll-like Receptor 7 |
TLR8 | = | Toll-like Receptor 8 |
TLR9 | = | Toll-like Receptor 9 |
MYD 88 | = | Myeloid Differentiation Primary Response 88 |
TRAF6 | = | Tumor Necrosis Factor Receptor-Associated Factor 6 |
TIR | = | Toll/Interleukin-1 receptor |
LABA | = | Long acting beta 2 agonist |
LAMA | = | Long acting anti-muscarinic agent |
IKK-α | = | Inhibitor of nuclear factor kappa-B kinase subunit alpha |
Supplemental Material
Download PDF (1.2 MB)Acknowledgment
We thank Vellore Institute of Technology for providing the computational facility.
Disclosure statement
The authors report no conflicts of interest. The authors alone are responsible for the content and writing of the paper.
References
- Lozano R, Naghavi M, Foreman K, et al. Global and regional mortality from 235 causes of death for 20 age groups in 1990 and 2010: a systematic analysis for the Global Burden of Disease Study 2010. Lancet Lond Engl. 2012;380(9859):2095–2128. DOI:10.1016/S0140-6736(12)61728-0
- Eisner MD, Anthonisen N, Coultas D, et al. An official American Thoracic Society public policy statement: novel risk factors and the global burden of chronic obstructive pulmonary disease. Am J Respir Crit Care Med. 2010;182(5):693–718. DOI:10.1164/rccm.200811-1757ST
- MacNee W. ABC of chronic obstructive pulmonary disease: pathology, pathogenesis, and pathophysiology. BMJ. 2006;332(7551):1202–1204. DOI:10.1136/bmj.332.7551.1202
- Qian Y, Wei L, Xu D, et al. Comprehensive analysis of gene-expression profile in chronic obstructive pulmonary disease. Int J Chron Obstruct Pulmon Dis. 2015;10:1103–1109. DOI:10.2147/COPD.S68570
- Decramer M, Janssens W, Miravitlles M. Chronic obstructive pulmonary disease. Lancet Lond Engl. 2012;379(9823):1341–1351. DOI:10.1016/S0140-6736(11)60968-9
- Srikanth C, Bharadwaj R. Docking studies of serine protease inhibitor with various SPI activators to treat COPD. Helix. 2017;8:1473–1478.
- Barnes PJ. Mechanisms in COPD: differences from asthma. Chest. 2000;117(2 Suppl):10S–14S. DOI:10.1378/chest.117.2_suppl.10s
- Chung KF. Cytokines as targets in chronic obstructive pulmonary disease. Curr Drug Targets. 2006;7(6):675–681. DOI:10.2174/138945006777435263
- Safka KA, Wald J, Wang H, et al. GOLD stage and treatment in COPD: a 500 patient point prevalence study. Chronic Obstr Pulm Dis Miami Fla. 2016;4(1):45–55.
- Daheshia M. Pathogenesis of chronic obstructive pulmonary disease (COPD). Clin Appl Immunol Rev. 2005;5(5):339–351. DOI:10.1016/j.cair.2005.09.003
- Glaab T, Vogelmeier C, Buhl R. Outcome measures in chronic obstructive pulmonary disease (COPD): strengths and limitations. Respir Res. 2010;11(1):79. DOI:10.1186/1465-9921-11-79
- Viniol C, Vogelmeier CF. Exacerbations of COPD. Eur Respir Rev. 2018;27(147):170103. DOI:10.1183/16000617.0103-2017
- Candela M, Costorella R, Stassaldi A, et al. Treatment of COPD: the simplicity is a resolved complexity. Multidiscip Respir Med. 2019;14(1):18. DOI:10.1186/s40248-019-0181-8
- Wedzicha JA. Role of viruses in exacerbations of chronic obstructive pulmonary disease. Proc Am Thorac Soc. 2004;1(2):115–120. DOI:10.1513/pats.2306030
- Dinesh DC, Tamilarasan S, Rajaram K, et al. Antiviral drug targets of single-stranded RNA viruses causing chronic human diseases. CDT. 2020;21(2):105–124. DOI:10.2174/1389450119666190920153247
- Selvaraj G, Selvaraj C, Wei DQ. Computational Advances in Chronic Diseases Diagnostics and Therapy - II. Curr Drug Targets. 2020;21(2):103–104. DOI:10.2174/138945012102200101144250
- Selvaraj G, Selvaraj C, Wei DQ. Computational advances in chronic diseases diagnostics and therapy - I. Curr Drug Targets. 2020;21(1):1–2. DOI:10.2174/138945012101191206142040
- Chen Z-H, Kim HP, Ryter SW, et al. Identifying targets for COPD treatment through gene expression analyses. Int J Chron Obstruct Pulmon Dis. 2008;3(3):359–370. DOI:10.2147/COPD.S1758
- Bhattacharya S, Srisuma S, Demeo DL, et al. Molecular biomarkers for quantitative and discrete COPD phenotypes. Am J Respir Cell Mol Biol. 2009;40(3):359–367. DOI:10.1165/rcmb.2008-0114OC
- Heguy A, O’Connor TP, Luettich K, et al. Gene expression profiling of human alveolar macrophages of phenotypically normal smokers and nonsmokers reveals a previously unrecognized subset of genes modulated by cigarette smoking. J Mol Med. 2006;84(4):318–328. DOI:10.1007/s00109-005-0008-2
- Kazeros A, Harvey B-G, Carolan BJ, et al. Overexpression of apoptotic cell removal receptor MERTK in alveolar macrophages of cigarette smokers. Am J Respir Cell Mol Biol. 2008;39(6):747–757. DOI:10.1165/rcmb.2007-0306OC
- Golpon HA, Coldren CD, Zamora MR, et al. Emphysema lung tissue gene expression profiling. Am J Respir Cell Mol Biol. 2004;31(6):595–600. DOI:10.1165/rcmb.2004-0008OC
- Spira A, Beane J, Pinto-Plata V, et al. Gene expression profiling of human lung tissue from smokers with severe emphysema. Am J Respir Cell Mol Biol. 2004;31(6):601–610. DOI:10.1165/rcmb.2004-0273OC
- Singh D, Fox SM, Tal-Singer R, et al. Induced sputum genes associated with spirometric and radiological disease severity in COPD ex-smokers. Thorax. 2011;66(6):489–495. DOI:10.1136/thx.2010.153767
- Huang S, Sun C, Hou Y, et al. A comprehensive bioinformatics analysis on multiple Gene Expression Omnibus datasets of nonalcoholic fatty liver disease and nonalcoholic steatohepatitis. Sci Rep. 2018;8(1):7630. DOI:10.1038/s41598-018-25658-4
- Huang DW, Sherman BT, Lempicki RA. Systematic and integrative analysis of large gene lists using DAVID bioinformatics resources. Nat Protoc. 2009;4(1):44–57. DOI:10.1038/nprot.2008.211
- Huang DW, Sherman BT, Lempicki RA. Bioinformatics enrichment tools: paths toward the comprehensive functional analysis of large gene lists. Nucleic Acids Res. 2009;37(1):1–13. DOI:10.1093/nar/gkn923
- Shannon P, Markiel A, Ozier O, et al. Cytoscape: a software environment for integrated models of biomolecular interaction networks. Genome Res. 2003;13(11):2498–2504. DOI:10.1101/gr.1239303
- Sundarrajan S, Lulu S, Arumugam M. Insights into protein interaction networks reveal non-receptor kinases as significant druggable targets for psoriasis. Gene. 2015;566(2):138–147. DOI:10.1016/j.gene.2015.04.030
- Xunlu Y, Minshan F, Liguo Z, et al. Integrative bioinformatics analysis reveals potential gene biomarkers and analysis of function in human degenerative disc annulus fibrosus cells. Biomed Res Int. 2019;2019:9890278–9890279. DOI:10.1155/2019/9890279
- Balázs G. KM-plot [Internet]. Kaplan-Meier plotter. [cited 2020. Jun 2]. Available at: http://www.kmplot.com/analysis.
- Hou G-X, Liu P, Yang J, et al. Mining expression and prognosis of topoisomerase isoforms in non-small-cell lung cancer by using Oncomine and Kaplan-Meier plotter. PLoS One. 2017;12(3):e0174515. DOI:10.1371/journal.pone.0174515
- Draw Venn Diagram [Internet]. [cited 2019. Jul 25]. Available at: http://bioinformatics.psb.ugent.be/webtools/Venn/.
- Gyorffy B, Surowiak P, Budczies J, et al. Online survival analysis software to assess the prognostic value of biomarkers using transcriptomic data in non-small-cell lung cancer. PloS One. 2013;8(12):e82241.
- Spaks A, Jaunalksne I, Spaka I, et al. Diagnostic value of circulating CXC chemokines in non-small cell lung cancer. Anticancer Res. 2015;35(12):6979–6983.
- Li L, Liu Y, Zhan Y, et al. High levels of CCL2 or CCL4 in the tumor microenvironment predict unfavorable survival in lung adenocarcinoma. Thorac Cancer. 2018;9(7):775–784. DOI:10.1111/1759-7714.12643
- Chatterjee S, Crozet L, Damotte D, et al. TLR7 promotes tumor progression, chemotherapy resistance, and poor clinical outcomes in non-small cell lung cancer. Cancer Res. 2014;74(18):5008–5018. DOI:10.1158/0008-5472.CAN-13-2698
- Zhang H, Liu H, Yuan D, et al. Prognostic value of secreted phosphoprotein-1 in pleural effusion associated with non-small cell lung cancer. BMC Cancer. 2014;14:280. DOI:10.1186/1471-2407-14-280
- Wang R, Zhou X, Wang H, et al. Integrative analysis of gene expression profiles reveals distinct molecular characteristics in oral tongue squamous cell carcinoma. Oncol Lett. 2019;17(2):2377–2387. DOI:10.3892/ol.2018.9866
- Hansbro PM, Haw TJ, Starkey MR, et al. Toll-like receptors in COPD. Eur Respir J. 2017;49(5):1700739. DOI:10.1183/13993003.00739-2017
- Toll-like Receptor Signaling | Cell Signaling Technology [Internet]. [cited 2019. Sep 3]. Available at: https://www.cellsignal.com/contents/science-cst-pathways-immunology-inflammation/toll-like-receptor-signaling/pathways-tlr.
- Kawasaki T, Kawai T. Toll-like receptor signaling pathways. Front Immunol. 2014;5(461):1–8. DOI:10.3389/fimmu.2014.00461
- Lafferty EI, Qureshi ST, Schnare M. The role of toll-like receptors in acute and chronic lung inflammation. J Inflamm (Lond). 2010;7:57. DOI:10.1186/1476-9255-7-57
- Bezemer GF, Sagar S, van Bergenhenegouwen J, et al. Dual role of toll-like receptors in asthma and chronic obstructive pulmonary disease. Pharmacol Rev. 2012;64(2):337–358. DOI:10.1124/pr.111.004622
- Droemann D, Goldmann T, Tiedje T, et al. Toll-like receptor 2 expression is decreased on alveolar macrophages in cigarette smokers and COPD patients. Respir Res. 2005;6(1):68DOI:10.1186/1465-9921-6-68
- Karimi K, Sarir H, Mortaz E, et al. Toll-like receptor-4 mediates cigarette smoke-induced cytokine production by human macrophages. Respir Res. 2006;7:66. DOI:10.1186/1465-9921-7-66
- Mortaz E, Adcock IM, Ito K, et al. Cigarette smoke induces CXCL8 production by human neutrophils via activation of TLR9 receptor. Eur Respir J. 2010;36(5):1143–1154. DOI:10.1183/09031936.00062209
- Zlotnik A, Morales J, Hedrick JA. Recent advances in chemokines and chemokine receptors. Crit Rev Immunol. 1999;19(1):47. DOI:10.1615/CritRevImmunol.v19.i1.10
- Baggiolini M. Chemokines in pathology and medicine. J Intern Med. 2001;250(2):91–104. DOI:10.1046/j.1365-2796.2001.00867.x
- Luster AD. Chemokines-chemotactic cytokines that mediate inflammation. N Engl J Med. 1998;338(7):436–445. DOI:10.1056/NEJM199802123380706
- Murphy PM, Baggiolini M, Charo IF, et al. International union of pharmacology. XXII. Nomenclature for chemokine receptors. Pharmacol Rev. 2000;52(1):145–176.
- Qin S, Rottman JB, Myers P, et al. The chemokine receptors CXCR3 and CCR5 mark subsets of T cells associated with certain inflammatory reactions. J Clin Invest. 1998;101(4):746–754. DOI:10.1172/JCI1422
- Loetscher M, Gerber B, Loetscher P, et al. Chemokine receptor specific for IP10 and mig: structure, function, and expression in activated T-lymphocytes. J Exp Med. 1996;184(3):963–969. DOI:10.1084/jem.184.3.963
- Grumelli S, Corry DB, Song L-Z, et al. An immune basis for lung parenchymal destruction in chronic obstructive pulmonary disease and emphysema. PLoS Med. 2004;1(1):e8. DOI:10.1371/journal.pmed.0010008
- Henrot P, Prevel R, Berger P, et al. Chemokines in COPD: from implication to therapeutic use. Int J Mol Sci. 2019;20(11):2785. DOI:10.3390/ijms20112785
- Pelaia G, Vatrella A, Gallelli L, et al. Biological targets for therapeutic interventions in COPD: clinical potential. Int J Chron Obstruct Pulmon Dis. 2006;1(3):321–334. DOI:10.2147/copd.2006.1.3.321
- Bafadhel M, McKenna S, Terry S, et al. Acute exacerbations of chronic obstructive pulmonary disease: identification of biologic clusters and their biomarkers. Am J Respir Crit Care Med. 2011;184(6):662–671. DOI:10.1164/rccm.201104-0597OC
- Barnes PJ. The cytokine network in chronic obstructive pulmonary disease. Am J Respir Cell Mol Biol. 2009;41(6):631–638. DOI:10.1165/rcmb.2009-0220TR
- Perret J, McDonald C, Apostolopoulos V. Elevated serum interleukin-5 levels in severe chronic obstructive pulmonary disease. Acta Biochim Biophys Sin (Shanghai). 2017;49(6):560–563. DOI:10.1093/abbs/gmx030
- Capelli A, Stefano AD, Gnemmi I, et al. Increased MCP-1 and MIP-1beta in bronchoalveolar lavage fluid of chronic bronchitics. Eur Respir J. 1999;14(1):160–165. DOI:10.1034/j.1399-3003.1999.14a27.x
- Lund SA, Giachelli CM, Scatena M. The role of osteopontin in inflammatory processes. J Cell Commun Signal. 2009;3(3–4):311–322. DOI:10.1007/s12079-009-0068-0
- Woodruff PG, Koth LL, Yang YH, et al. A distinctive alveolar macrophage activation state induced by cigarette smoking. Am J Respir Crit Care Med. 2005;172(11):1383–1392. DOI:10.1164/rccm.200505-686OC
- Gantier MP, Tong S, Behlke MA, et al. TLR7 is involved in sequence-specific sensing of single-stranded RNAs in human macrophages. J Immunol. 2008;180(4):2117–2124. DOI:10.4049/jimmunol.180.4.2117
- Yamamoto M, Sato S, Hemmi H, et al. Role of adaptor TRIF in the MyD88-independent toll-like receptor signaling pathway. Science. 2003;301(5633):640–643. DOI:10.1126/science.1087262
- Pomerenke A, Lea SR, Herrick S, et al. Characterization of TLR-induced inflammatory responses in COPD and control lung tissue explants. Int J Chron Obstruct Pulmon Dis. 2016;11:2409–2417. DOI:10.2147/COPD.S105156
- Gao W, Xiong Y, Li Q, et al. Inhibition of toll-like receptor signaling as a promising therapy for inflammatory diseases: a journey from molecular to nano therapeutics. Front Physiol. 2017;8:508. DOI:10.3389/fphys.2017.00508
- Kawai T, Akira S. The role of pattern-recognition receptors in innate immunity: update on Toll-like receptors. Nat Immunol. 2010;11(5):373–384. DOI:10.1038/ni.1863
- Moresco EM, LaVine D, Beutler B. Toll-like receptors. Curr Biol. 2011;21(13):R488–R493. DOI:10.1016/j.cub.2011.05.039
- Botos I, Segal DM, Davies DR. The structural biology of Toll-like receptors. Structure. 2011;19(4):447–459. DOI:10.1016/j.str.2011.02.004
- Bryant CE, Symmons M, Gay NJ. Toll-like receptor signalling through macromolecular protein complexes. Mol Immunol. 2015;63(2):162–165. DOI:10.1016/j.molimm.2014.06.033
- Takeda K, Kaisho T, Akira S. Toll-like receptors. Annu Rev Immunol. 2003;21(1):335–376. DOI:10.1146/annurev.immunol.21.120601.141126
- Akira S, Takeda K. Toll-like receptor signalling. Nat Rev Immunol. 2004;4(7):499–511. DOI:10.1038/nri1391
- Chaudhuri N, Dower SK, Whyte MKB, et al. Toll-like receptors and chronic lung disease. Clin Sci. 2005;109(2):125–133. DOI:10.1042/CS20050044
- Durham AL, Adcock IM. The relationship between COPD and lung cancer. Lung Cancer Amst Neth. 2015;90(2):121–127. DOI:10.1016/j.lungcan.2015.08.017