Abstract
The purpose of this study was to investigate the relationship between glucose to lymphocyte ratio (GLR) and the outcome of acute exacerbation chronic obstructive pulmonary disease (AECOPD) patients admitted to the intensive care unit (ICU). This study included 3573 patients from the eICU Collaborative Research Database (eICU-CRD) and 926 AECOPD patients admitted to ICU from the Medical Information Mart for Intensive Care-IV (MIMIC-IV) database. The optimal cutoff value for GLR was 5.6. Kaplan-Meier analysis demonstrated that patients in lower GLR (< 5.6) group showed a better overall survival than patients in higher GLR (≥ 5.6) group in all sets. Multivariate Cox regression analysis demonstrated that age, Sequential Organ Failure Assessment (SOFA) score, SpO2, albumin and GLR are independent predictors of poor overall survival in the training cohort and were incorporated into the nomogram for in-hospital mortality as independent factors. The nomogram exhibited excellent discrimination with C-indexes in training cohort, internal validation and external validation cohort were (0.801, 95%CI: 0.769-0.863), (0.805, 95%CI: 0.759-0.851) and (0.811, 95%CI: 0.772-0.850), respectively. The calibration plot indicated an adequate fit of the nomogram for predicting the risk of in-hospital mortality in all sets. Moreover, the ROC analyses demonstrated that the discrimination abilities of GLR were better than other blood-based inflammatory biomarkers. As an easily available biomarker, GLR can independently predict the in-hospital mortality in AECOPD patients admitted to ICU. The nomogram combining GLR with other significant indicators exhibited excellence predictive performance for in-hospital mortality.
Introduction
Chronic obstructive pulmonary disease (COPD) is a markedly global burden to the health care systems despite advances in medical therapy, and is the third leading cause of death worldwide [Citation1–3]. Acute exacerbation of COPD (AECOPD) is one of the leading causes of hospitalization and is associated with considerable mortality [Citation4]. About 10% COPD patients undergoing an acute exacerbation during hospitalization, which needed critical care interventions particularly the support of ventilator, and hence require admission to the intensive care unit (ICU) [Citation5]. A meta-analysis analyzed the mortality of AECOPD patients admitted to ICU and found the mortality rate was extremely high with 17.6%-48.8% during hospital stay [Citation6]. Therefore, it is of significantly important for clinicians to explore useful and easily obtained biomarkers for prognostic evaluation.
Numerous studies have highlighted the critical role of inflammation in the severity and prognosis of AECOPD patients. Increased inflammatory response aggravates clinical symptoms and worsens lung function among these patients [Citation7,Citation8]. Lots of serum inflammatory biomarkers were reported to exhibit good prognostic values for AECOPD patients, such as neutrophil-lymphocyte ratio (NLR) [Citation9], platelet-lymphocyte ratio (PLR) [Citation10] and systemic immune-inflammation index (SII) [Citation11]. As one of the components of the systemic inflammatory response, lymphocytes are well-known inflammatory markers involved in AECOPD. Sun et al. demonstrated that noninvasive mechanical ventilation (NIMV) failure of AECOPD patients had lower lymphocyte counts than those patients with NIMV success [Citation9]. Moreover, a study found lower lymphocyte counts in eosinophilic AECOPD patients [Citation12]. Another study evaluated the in-hospital mortality predictive role of NLR and PLR in AECOPD patients and found that lower lymphocyte counts were significantly associated with increased in-hospital mortality [Citation13].
Moreover, accumulating evidences indicated that hyperglycemia was closely associated with prognosis in AECOPD patients. Monsour et al. examined the outcome of AECOPD with or without diabetes mellitus (DM) and found that DM is independently associated with increased ICU transfers amongst patients hospitalized with AECOPD, patients with DM had higher length of stay and increased need for ICU transfer [Citation14]. Another study also indicated that DM comorbidity leading to lower PaO2, longer hospital stays, and increased risk of death in AECOPD patients [Citation15].
Based the evidences above, researchers tried to explore whether the combination of blood glucose and lymphocyte count could be a prognostic biomarker in predicting prognosis in AECOPD patients. Previous studies assessed the ratio of glucose to lymphocytes (GLR), an indicator which included both glucose metabolism and systemic inflammation in different types of malignancies including pancreatic ductal adenocarcinoma [Citation16], pancreatic cancer patients [Citation17] and resected pT2 gallbladder cancer [Citation18]. However, the role of GLR to predict prognosis in AECOPD patients admitted to ICU remains unclear. Hence, this study was designed to evaluate the prognostic value of GLR in AECOPD patients admitted to ICU.
Methods
Data source
All data were extracted from the eICU Collaborative Research Database (eICU-CRD) [Citation19] and the Medical Information Mart for Intensive Care-IV (MIMIC-IV version 1.0) database [Citation20]. Due to the data used in this study were extracted from public databases, it was exempt from the requirement for informed consent from patients and approval of the Institutional Review Board (IRB). All procedures were performed according to the ethical standards of the 1964 Helsinki Declaration and its later amendments or comparable ethical standards. After finishing the web-based training courses and the Protecting Human Research Participants examination, we obtained permission to extract data from the eICU-CRD and MIMIC-IV.
Cohort selection
Patients diagnosed as AECOPD over 18 years older at their first ICU admission were included in the present study. The definition of AECOPD was based on the International Classification of Diseases (ICD) code. The exclusion criteria were as followings: (1) less than 18-year-old; (2) fasting blood glucose and lymphocyte counts data at the first day of admission to the ICU were missing; (3) patients with repeated ICU admissions. Finally, 3573 patients with AECOPD from eICU-CRD and 926 patients with AECOPD from MIMIC-IV were included in this study. In addition, patients with AECOPD from eICU-CRD were randomly divided into the training cohort (n = 2502) and the internal validation cohort (n = 1071) in a ratio of 7:3. And patients with AECOPD from MIMIC-IV (n = 926) were used as external validation set. The detailed process of selection is shown in .
Data collection and outcomes
In the present study, we collected patient’s baseline parameters including age, sex, vital signs, total bilirubin, albumin, serum creatinine (SCr), hemoglobin, white blood cell (WBC) count, lymphocyte counts, monocyte counts, platelet counts, heart rate, SpO2, MAP, hypertension, diabetes, congestive heart failure (CHF) and chronic kidney disease (CKD), Sequential Organ Failure Assessment score (SOFA) and oxford acute severity of illness score (OASIS). The use of vasopressor and mechanical ventilation during the first day of ICU admission were also extracted. The GLR was defined as fasting blood glucose/lymphocyte counts. NLR was calculated by neutrophil counts/lymphocyte count. PLR was calculated by platelet counts/lymphocyte counts. SII was calculated by neutrophil counts × platelet counts/lymphocyte counts.
The primary outcome was in-hospital mortality.
Statistical analysis
Baseline characteristics are expressed as mean ± standard deviation (SD) and categorical variables are expressed as number (percentage), descriptive statistics are presented as medians with interquartile ranges (IQR). The baseline characteristics between different groups were compared using analyzed with one-way ANOVA followed by the Tukey’s post hoc test or chi-square test. The X-tile software was conducted to evaluate the optimal cutoff GLR.
Univariate and multivariate Cox regression were conducted to determine the significant variables predictive of in-hospital mortality, the factors that had P values <0.05 in the univariate analysis were entered into a multivariate analysis. The Kaplan-Meier analysis was used to estimate overall survival, and the differences between the curves were compared using the log-rank test. We compared the predictive abilities between GLR and NLR, PLR and SII by conducting the receiver operating characteristic (ROC) curve analysis. C-index was used to assess the nomogram performance for predicting in-hospital mortality in AECOPD patients admitted to ICU. All analyses were conducted using R software and SPSS Statistics. p < 0.05 was considered statistically significant.
Results
Patient characteristics and clinical features
3573 patients with AECOPD from eICU-CRD and 926 patients with AECOPD from MIMIC-IV were finally included in this study. Patients from eICU-CRD were randomly divided into the training cohort (n = 2502) and the internal validation cohort (n = 1071) in a ratio of 7:3. And patients with AECOPD from MIMIC-IV (n = 926) were used as external validation set. The detailed process of selection is shown in . exhibited the baseline parameters in the training set, internal validation set and external validation set. Age (p < 0.001), vasopressors usage (p < 0.001), congestive heart failure (p < 0.001), chronic kidney disease (p < 0.001), liver disease (p < 0.001), SOFA score (p < 0.001), OASIS score (p < 0.001), heart rate (p < 0.001), SPO2 (p < 0.001), white blood cell (p < 0.001), hemoglobin (p < 0.001), albumin (p < 0.001), total bilirubin (p < 0.001), creatinine (p < 0.001), NLR (p < 0.001), PLR (p = 0.027), GLR (p < 0.001), length of ICU (p < 0.001), length of hospital (p < 0.001) and in-hospital mortality (p < 0.001) differed markedly between the three groups.
Table 1. Clinicopathological characteristics of all patients.
GLR was an independent prognostic factor
Univariate analysis indicated that age, MV usage, SOFA score, SPO2, PLR, SII, albumin and GLR were predictors for in-hospital mortality in the training cohort (p < 0.05, ). And multivariate analyses demonstrated that age (HR:1.03, 95%CI: 1.02-1.0, p < 0.001), SOFA score (HR:1.15, 95%CI: 1.10-1.20, p < 0.001), SPO2 (HR:0.97, 95%CI: 0.94-0.99, p = 0.01), albumin (HR:1.04, 95%CI: 1.03-1.05, p < 0.001) and GLR (HR:0.37, 95%CI: 0.27-0.52, p < 0.001) were independent predictors for in-hospital mortality in AECOPD patients admitted to ICU (). Kaplan-Meier analysis demonstrated that patients in lower GLR (< 5.6) group showed a better overall survival than patients in higher GLR (≥ 5.6) group both in the training cohort (p < 0.001, ), in the internal validation cohort (p < 0.001, ) and in the external validation cohort (p = 0.00016, ).
Figure 2. Kaplan-Meier curves of AECOPD patients admitted to ICU stratified by the optimal cutoff value of GLR in the (A) training cohort and (B) internal validation cohort and (C) external validation cohort.
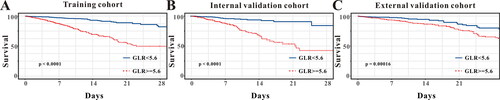
Table 2. Cox analyses of factors associated with in-hospital mortality in training cohort.
Prognostic value of GLR as a predicted biomarker in nomogram for in-hospital mortality
A nomogram was created according to the independent predictors based on the multivariate Cox regression analysis to predict the in-hospital mortality of the AECOPD patients admitted to ICU. A higher score in the nomogram means a shorter in-hospital mortality. As depicted in , GLR contributed the most to the predictive effect to prognosis in the nomogram, followed by platelet, albumin, etc. (). The nomogram showed relatively good model discriminative capacity for in-hospital mortality in AECOPD patients admitted to ICU which reflected by C-index. C-index were (0.801, 95%CI: 0.769-0.863) in the training cohort, (0.805, 95%CI: 0.759-0.851) in the internal validation cohort and (0.811, 95%CI: 0.772-0.850) in the external validation cohort. The calibration curve for the predictive nomogram depicted a high agreement between the actual probability and predicted probability in the training set, in the internal validation set and in the external validation set (). Finally, decision curve analysis (DCA) was performed to determine the clinical utilities of the predictive nomogram. The DCA curve also manifested that the survival nomogram derived from the training set was clinically useful in the training set as well as in the internal validation set and in the external validation set ().
Figure 3. The survival nomogram for predicting in-hospital mortality of AECOPD patients admitted to ICU. When using it, drawing a vertical line from each variables upward to the points and then recording the corresponding points (i.e. “albumin ≥ 3.0 g/dL” = 4 points). The point of each variable was then summed up to obtain a total score that corresponds to a predicted probability of in-hospital mortality at the bottom of the nomogram.
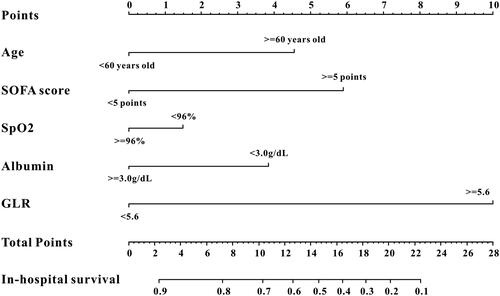
Figure 4. (A) The calibration curve for predicting in-hospital mortality in the training cohort. (B) The calibration curve for predicting in-hospital mortality in the internal validation. (C) The calibration curve for predicting in-hospital mortality in the external validation cohort. (D) Decision curve analysis DCA of the nomogram to predict in-hospital mortality in the training cohort. (E) DCA of the nomogram to predict in-hospital mortality in the internal validation. (F) DCA of the nomogram to predict in-hospital mortality in the external validation cohort.
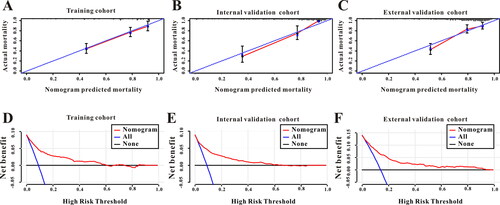
Comparison the predictive role between GLR and NLR, PLR, LMR, SII
Previous studies focused on the development of rapid systemic inflammatory biomarkers based on accessible laboratory tests for reliable prognosis prediction of AECOPD patients, such as NLR [Citation9], PLR [Citation10] and SII [Citation11]. These systemic inflammatory biomarkers were all reported to be associated with prognosis of AECOPD patients. As a novel systemic inflammatory biomarker of GLR, we intended to compared the predictive abilities between NLR, PLR, SII and GLR by conducting the ROC curve analysis. When predicting the in-hospital mortality for AECOPD patients admitted to ICU, the GLR exhibited good predictive abilities in the training set (AUC: 0.750, 95%CI: 0.732-0.767), in the internal validation set (AUC: 0.738, 95%CI:0.710-0.764) and in the external validation set (AUC: 0.755, 95%CI:0.726-0.783). Moreover, the AUC of GLR was greater when compared with the AUC of NLR, PLR and SII both in the training set, in the internal validation set and in the external validation set (p < 0.05, respectively, ). Consequently, these data showed that the novel systemic inflammatory response biomarker of GLR was superior to other systemic inflammatory response biomarkers (NLR, PLR and SII) when predicting the in-hospital mortality for AECOPD patients admitted to ICU.
Table 3. Receiver operating curve anaysis.
Discussion
In this study, we tried to examine the potential application of blood laboratory-based parameters GLR, which combined blood glucose with lymphocyte, to predict in-hospital mortality in AECOPD patients admitted to ICU. The results indicated that elevated GLR was significantly linked to an increased in-hospital mortality. A predictive nomogram model incorporating GLR and other prognostic factors exhibited excellent discrimination and calibration abilities in predicting in-hospital mortality. And the validation data also showed consistent results. In addition, GLR was a superior predictor for in-hospital mortality when compared to NLR, PLR and SII.
AECOPD patients admitted to ICU are commonly associated with poor prognosis, including a worsened in lung function, declined quality of life, an increased risk of recurrent exacerbations and elevated risk of mortality. Despite significant progress in treatment, patients with advanced AECOPD have poor prognosis. A meta-analysis analyzed the mortality of AECOPD patients admitted to ICU and found the mortality rate was extremely high with 17.6%-48.8% during hospital stay [Citation6]. Another study reported that the mortality rate was 27.2% in AECOPD patients admitted to ICU [Citation21]. In the present study, the mortality rate of AECOPD patients admitted to ICU was 9% in eICU-CRD database and 13.9% in MIMIC-IV database, which was consistent with Mohan et al. [Citation22] reported (13.7%) but lower than Warwick et al. reported [Citation23]. The potential reason maybe the lower mechanical ventilation usage in this study compared to Warwick et al. [Citation23] reported (25% vs. 55.1%). Identification of parameters related to in-hospital mortality yielded several important findings. As expected, increasing age predicted in-hospital mortality, which was similar to previous reported [Citation24]. In addition, SOFA score was found to predict in-hospital mortality. SOFA score is a well-known scoring system to predict short-term mortality in sepsis and other life threatening conditions, especially in ICU condition [Citation25,Citation26]. Yang et al. found that the SOFA score was significantly higher in non-survivors compared to survivors [Citation27]. Unsurprisingly, we also found the SpO2 could predict in-hospital mortality in AECOPD patients admitted to ICU. Moreover, albumin (ALB) was identified as a prognostic factor in-hospital mortality. Serum ALB values were used for nutritional assessment which has been shown to decrease in many acute and chronic diseases particularly in ICU condition. Lower ALB values were reported to predict in-hospital mortality in AECOPD patients and were incorporated into many scoring system models [Citation28,Citation29].
However, the inflammatory biomarkers including NLR, PLR and SII were not predictors for in-hospital mortality in AECOPD patients admitted to ICU in the present study. And NLR, SII were reported to exhibit good predictive effect in AECOPD patients [Citation9, Citation11], however, the prognostic role of PLR was controversial, Luo et al. [Citation10] demonstrated that PLR could predict mortality in AECOPD patients but Emami Ardestani et al. [Citation13] indicated that PLR couldn’t predict mortality in AECOPD patients and these results deserve further research. In the present study, we found a novel inflammatory biomarker GLR which combined blood glucose and lymphocytes counts, exhibited excellent predictive ability for in-hospital mortality. Patients with GLR ≥ 5.6 depicted poor outcome compared to those GLR < 5.6, and a predictive nomogram model incorporating GLR and age, SOFA score, SpO2, albumin which exhibited excellent discrimination and calibration abilities in predicting in-hospital mortality. Furthermore, we compared the predictive abilities between NLR, PLR, SII and GLR by conducting the ROC curve analysis and found that GLR was superior to other systemic inflammatory response biomarkers (NLR, PLR and SII) when predicting the in-hospital mortality for AECOPD patients admitted to ICU. Previous studies reported that GLR depicted good prognostic value in different types of malignancies [Citation16–18]. To our knowledge, the present study is the first to report the prognostic value of GLR for in-hospital mortality in AECOPD patients admitted to ICU.
Although the precise mechanisms remains unclear, some clues may explain the association between higher GLR and increased risk of in-hospital mortality in AECOPD patients admitted to ICU. A study demonstrated diabetes mellitus (DM) is independently associated with increased ICU transfers amongst patients hospitalized with AECOPD and patients with DM had higher length of stay and increased need for ICU transfer [Citation14]. Another study also indicated that AECOPD patients with DM showed lower PaO2, longer hospital stays, and increased risk of death [Citation15]. Moreover, Chen et al. reported that stable COPD patients with DM received oral antihyperglycaemic drugs therapy showed lower risk of acute exacerbations of COPD [Citation30]. These findings revealed that higher blood glucose levels were associated with poor outcome in AECOPD patients. Moreover, lymphocytes are well-known inflammatory markers which have been widely reported to be linked with prognosis in AECOPD patients. Sun et al. demonstrated that noninvasive mechanical ventilation (NIMV) failure of AECOPD patients had lower lymphocyte counts than those patients with NIMV success [Citation9]. In addition, lower lymphocyte counts were also found in eosinophilic AECOPD patients [Citation12]. Another study evaluated the predictive role of NLR and PLR for in-hospital mortality in AECOPD patients and revealed that lower lymphocyte counts were significantly associated with increased in-hospital mortality [Citation13]. Lymphocytes are indicators of immunity and decrease lymphocytes counts demonstrates immunity injury, and reduction in immune function, and leading to poor outcomes in COPD patients [Citation31]. Geerdink et al. [Citation32] found that frequent AECOPD were accompanied by reduced lymphocytes, including CD8+ T effector memory cells and CD4+ T central memory cells. Therefore, GLR may reflect the synergistic effect of hyperglycemia and depressed immunity in AECOPD patients admitted to ICU.
There were several limitations to our study. First, this was a retrospective study based on the eICU-CRD and MIMIC-IV databases, the results in this study require further validation by prospective studies in the future. Moreover, GLR was calculated only from the data measured when the patients first admitted to the ICU. Dynamic assessment of GLR during patients’ stay in ICU can decrease bias of the results. Finally, although the sample size of this study was not small, future larger multicenter prospective studies are warranted to validate these findings.
Conclusions
The present study demonstrated that elevated GLR was significantly associated with increased risk of in-hospital mortality in AECOPD patients admitted to ICU. The nomogram combined GLR showed excellence discrimination and calibration abilities for predicting in-hospital mortality. It may possibly provide clinicians with useful clues to make accurate decision in AECOPD patients admitted to ICU in clinical. Future prospective studies are warranted to validate these findings.
Authors’ contributions
Tianyang Hu conceived and designed the study, Xiaoqiang Liu extracted the data, Xiaoqiang Liu analyzed the data and drafted the work. Yanan Liu takes responsibility for the content of the manuscript including the data and analysis. All authors have approved the final version of the manuscript for submission and agree to be accountable for all aspects of the work.
Ethics approval and consent to participate
This study was approved by the Anyue County People’s Hospital Medical Ethics Committee (See Supplementary file).
Disclosure statement
No potential conflict of interest was reported by the author(s).
Funding
The author(s) reported there is no funding associated with the work featured in this article.
Availability of data and materials
The datasets used are available from the corresponding author on reasonable request.
References
- Vogelmeier CF, Criner GJ, Martinez FJ, et al. Global strategy for the diagnosis, management, and prevention of chronic obstructive lung disease 2017 report. GOLD executive summary. Am J Respir Crit Care Med. 2017;195(5):557–582. DOI:10.1164/rccm.201701-0218PP
- Buist AS, McBurnie MA, Vollmer WM, BOLD Collaborative Research Group, et al. International variation in the prevalence of COPD (the BOLD study): a population-based prevalence study. Lancet. 2007;370(9589):741–750. DOI:10.1016/S0140-6736(07)61377-4
- Hoyert DL, Xu JQ. Deaths: preliminary data for 2011. Natl Vital Stat Rep. 2012;61(6):1–65.
- Groenewegen KH, Schols AM, Wouters EFM. Mortality and mortality-related factors after hospitalization for acute exacerbation of COPD. Chest. 2003;124(2):459–467. DOI:10.1378/chest.124.2.459
- Mulpuru S, McKay J, Ronksley PE, et al. Factors contributing to high-cost hospital care for patients with COPD. Int J Chron Obstruct Pulmon Dis. 2007; 24(12):989–995.
- Singanayagam A, Schembri S, Chalmers JD. Predictors of mortality in hospitalized adults with acute exacerbation of chronic obstructive pulmonary disease. Ann Am Thorac Soc. 2013;10(2):81–89. DOI:10.1513/AnnalsATS.201208-043OC
- Zhou X, Li Q, Zhou X. Exacerbation of chronic obstructive pulmonary disease. Cell Biochem Biophys. 2015;73(2):349–355. DOI:10.1007/s12013-015-0605-9
- Groenewegen KH, Postma DS, Hop WC, COSMIC Study Group, et al. Increased systemic inflmmation is a risk factor for COPD exacerbations. Chest. 2008;133(2):350–357. DOI:10.1378/chest.07-1342
- Sun W, Luo Z, Jin J, et al. The neutrophil/lymphocyte ratio could predict noninvasive mechanical ventilation failure in patients with acute exacerbation of chronic obstructive pulmonary disease: a retrospective observational study. COPD. 2021;16:2267–2277. DOI:10.2147/COPD.S320529
- Luo Z, Zhang W, Chen L, et al. Prognostic value of neutrophil:Lymphocyte and platelet:Lymphocyte ratios for 28-Day mortality of patients with AECOPD. Int J Gen Med. 2021;14:2839–2848. DOI:10.2147/IJGM.S312045
- Liu X, Ge H, Feng X, et al. The combination of hemogram indexes to predict exacerbation in stable chronic obstructive pulmonary disease. Front Med (Lausanne). 2020;7:572435. DOI:10.3389/fmed.2020.572435
- Dai G, Ran Y, Wang J, et al. Clinical differences between eosinophilic and noneosinophilic acute exacerbation of chronic obstructive pulmonary disease: a multicenter cross-sectional Study. Mediators Inflamm. 2020;2020:1059079. DOI:10.1155/2020/1059079
- Emami Ardestani M, Alavi-Naeini N. Evaluation of the relationship of neutrophil-to lymphocyte ratio and platelet-to-lymphocyte ratio with in-hospital mortality in patients with acute exacerbation of chronic obstructive pulmonary disease. Clin Respir J. 2021;15(4):382–388. DOI:10.1111/crj.13312
- Monsour E, Rodriguez LM, Abdelmasih R, et al. Characteristics and outcomes of diabetic patients with acute exacerbation of COPD. J Diabetes Metab Disord. 2021;20(1):461–466. DOI:10.1007/s40200-021-00766-7
- Lin L, Shi J, Kang J, et al. Analysis of prevalence and prognosis of type 2 diabetes mellitus in patients with acute exacerbation of COPD. BMC Pulm Med. 2021;21(1):7. DOI:10.1186/s12890-020-01371-9
- Zhang Y, Xu Y, Wang D, et al. Prognostic value of preoperative glucose to lymphocyte ratio in patients with resected pancreatic cancer. Int J Clin Oncol. 2021;26(1):135–144. DOI:10.1007/s10147-020-01782-y
- Zhong A, Cheng CS, Kai J, et al. Clinical significance of glucose to lymphocyte ratio (GLR) as a prognostic marker for patients with pancreatic Cancer. Front Oncol. 2020;10:520330. DOI:10.3389/fonc.2020.520330
- Navarro J, Kang I, Hwang HK, et al. Glucose to lymphocyte ratio as a prognostic marker in patients with resected pT2 gallbladder Cancer. J Surg Res. 2019;240:17–29. DOI:10.1016/j.jss.2019.02.043
- Pollard TJ, Johnson AEW, Raffa JD, et al. The eICU collaborative research database, a freely available multi-center database for critical care research. Sci Data. 2018;5:180178. DOI:10.1038/sdata.2018.178
- Liu T, Zhao Q, Du B. Effects of high-flow oxygen therapy on patients with hypoxemia after extubation and predictors of reintubation: a retrospective study based on the MIMIC-IV database. BMC Pulm Med. 2021;21(1):160. DOI:10.1186/s12890-021-01526-2
- Stolz D, Barandun J, Borer H, et al. Diagnosis, prevention and treatment of stable COPD and acute exacerbations of COPD: the swiss recommendations 2018. Respiration. 2018;96(4):382–398. DOI:10.1159/000490551
- Mohan A, Premanand R, Reddy LN, et al. Clinical presentation and predictors of outcome in patients with severe acute exacerbation of chronic obstructive pulmonary disease requiring admission to intensive care unit. BMC Pulm Med. 2006;6(1):27. DOI:10.1186/1471-2466-6-27
- Warwick M, Fernando SM, Aaron SD, Resource Optimization Network, et al. Outcomes and resource utilization among patients admitted to the intensive care unit following acute exacerbation of chronic obstructive pulmonary disease. J Intensive Care Med. 2021;36(9):1091–1097. DOI:10.1177/0885066620944865
- Seneff MG, Wagner DP, Wagner RP, et al. Hospital and 1-year survival of patients admitted to intensive care units with acute exacerbation of chronic obstructive pulmonary disease. JAMA. 1995;274(23):1852–1857.
- Raith EP, Udy AA, Bailey M, for the Australian and New Zealand Intensive Care Society (ANZICS) Centre for Outcomes and Resource Evaluation (CORE), et al. Australian and New Zealand intensive care society (ANZICS) Centre for outcomes and resource evaluation (CORE). prognostic accuracy of the SOFA score, SIRS criteria, and qSOFA score for in-Hospital mortality among adults with suspected infection admitted to the intensive care unit. JAMA. 2017;317(3):290–300. DOI:10.1001/jama.2016.20328
- Jentzer JC, Bennett C, Wiley BM, et al. Predictive value of individual sequential organ failure assessment Sub-scores for mortality in the cardiac intensive care unit. PLoS One. 2019;14(5):e0216177. DOI:10.1371/journal.pone.0216177
- Yang J, Yang J. Association between blood eosinophils and mortality in critically ill patients with acute exacerbation of chronic obstructive pulmonary disease: a retrospective cohort study. Int J Chron Obstruct Pulmon Dis. 2021;16:281–288. DOI:10.2147/COPD.S289920
- Yu X, Zhu GP, Cai TF, et al. Establishment of risk prediction model and risk score for in-hospital mortality in patients with AECOPD. Clin Respir J. 2020;14(11):1090–1098. DOI:10.1111/crj.13246
- Gu Y, Ye X, Liu Y, et al. A risk-predictive model for invasive pulmonary aspergillosis in patients with acute exacerbation of chronic obstructive pulmonary disease. Respir Res. 2021;22(1):176. DOI:10.1186/s12931-021-01771-3
- Chen KY, Wu SM, Tseng CH, et al. Combination therapies with thiazolidinediones are associated with a lower risk of acute exacerbations in new-onset COPD patients with advanced diabetic mellitus: a cohort-based case-control study. BMC Pulm Med. 2021;21(1):141. DOI:10.1186/s12890-021-01505-7
- Guo R, Li J, Ma X, et al. The predictive value of neutrophil-to-lymphocyte ratio for chronic obstructive pulmonary disease: a systematic review and Meta-analysis. Expert Rev Respir Med. 2020;14(9):929–936. DOI:10.1080/17476348.2020.1776613
- Geerdink JX, Simons SO, Pike R, et al. Differences in systemic adaptive immunity contribute to the ‘frequent exacerbator’ COPD phenotype. Respir Res. 2016;17(1):140. DOI:10.1186/s12931-016-0456-y