ABSTRACT
In this study, a novel photovoltaic power forecasting system that utilizes a deep Convolutional Neural Network (CNN) structure and an input signal decomposition algorithm is proposed. The proposed CNN architecture extracts deep features to forecast short-term power using transfer learning-based AlexNet. The historical power, solar radiation, wind speed, and temperature data are selected as the input. The signal decomposition algorithm called Empirical Mode Decomposition (EMD) is utilized to decompose the historical power signal into sub-components. In order to extract deep features, all input parameters are converted to 2D feature maps and feed to the input of the CNN. The experiments are realized on a grid-tied Photovoltaic Power Plant (PVPP) that has 1000 kW installed capacity located in Turkey. The experiments are performed under four weather conditions as partial cloudy, cloudy-rainy, heavy-rainy, and sunny days to show the effectiveness of the proposed method. The obtained results are compared with the benchmark regression algorithms. When the results are analyzed, the proposed method gives the highest Correlation Coefficient (R) and the lowest Root Mean Square Error (RMSE), Mean Absolute Error (MAE), and SMAPE values under all horizons and weather conditions. For 1-h to 5-h ahead, the average R values of the proposed method are obtained as 97.28%, 95.77%, 94.49%, 93.61%, and 92.62%, respectively. The average RMSE values are observed as 4.90%, 6.30%, 7.50%, 8.00%, and 9.17% for 1-h to 5-h ahead. The experimental results confirm that the proposed method outperforms the conventional regression algorithms and reveals effective results with its competitive performance.
Additional information
Notes on contributors
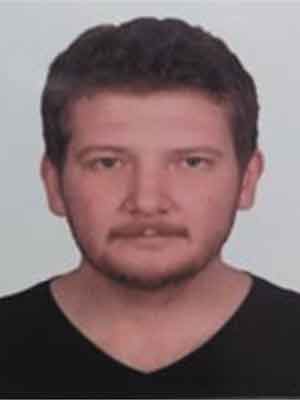
Deniz Korkmaz
Deniz Korkmaz received his Ph.D. degree in Electrical and Electronics Engineering from Fırat University in 2018. He is working as an Assistant Professor in the Department of Electrical and Electronics Engineering at Malatya Turgut Ozal University. He has published nearly 30 papers in journals and proceedings. His research interests are intelligent control techniques, artificial intelligence, deep learning, robotics, and mechatronics.
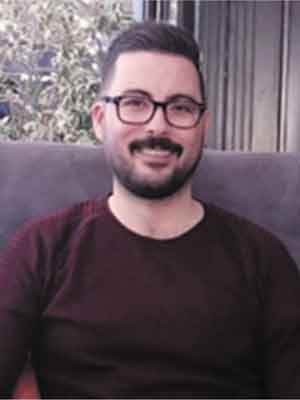
Hakan Acikgoz
Hakan Acikgoz received his Ph.D. degree in Electrical and Electronics Engineering from Kahramanmaraş Sutcu Imam University in 2018. He is working as an Assistant Professor in the Department of Electrical and Electronics Engineering at Gaziantep Islam Science and Technology University. He has ten years of work experience in the field of Academic. His research interests are power electronic converters, intelligent controller systems, identification and control of nonlinear systems, and forecasting methods.
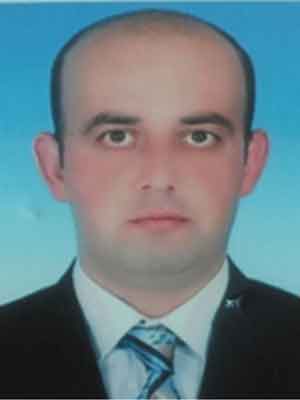
Ceyhun Yildiz
Ceyhun Yildiz received his Ph.D. degrees from the Electrical and Electronics Engineering from Kahramanmaraş Sutcu Imam University, Kahramanmaraş, Turkey in 2017. He worked as an engineer for eight years in the Ministry of Energy and Natural Resources of Turkey. He is currently a Lecturer in the Department of Electricity and Energy of Kahramanmaraş İstiklal University. His research interests include stochastic optimization, renewable power forecasting systems, wind energy, and hydroelectricity.