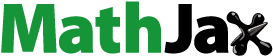
ABSTRACT
Natural fiber composites are a potential material in a range of engineering applications because of their excellent properties, which include reduced weight, better strength, and economic affordability. Aside from these features, these materials are biodegradable and renewable. The intent of this study is to look through into mechanical properties of aluminum oxide (Al2O3), boron carbide (B4C), and silicon carbide (SiC) particle-filled jute fiber polymer composite. The response surface methodology (RSM) with three levels/three factors is used to achieve the different combinations of process variables required to fabricate the desired polymer composites. In this regard, the effect of process variables on tensile characteristics, increase in weight %, and flexural characteristics is examined in detail. Further, the best combination of process parameters is chosen to produce composites with the desired mechanical qualities. The significance of such variables on each output variable is assessed using analysis of variance. A hybrid grey-based adaptive neuro-fuzzy inference system model is constructed for establishing multiple performance indexes. From the validation outcomes obtained, it is proved that the evolved model is proficient for precise prediction.
摘要
天然纤维复合材料由于其优异的性能,包括重量减轻、强度更好和经济实惠,是一种潜在的工程应用材料.除了这些特点,这些材料是可生物降解的和可再生的.本研究旨在深入研究氧化铝(Al2O3)、碳化硼(B4C)和碳化硅(SiC)颗粒填充黄麻纤维聚合物复合材料的力学性能.具有三个水平/三个因素的响应面方法(RSM)用于实现制造所需聚合物复合材料所需的工艺变量的不同组合.在这方面,详细检查了工艺变量对拉伸特性、重量增加(%)和弯曲特性的影响.此外,选择工艺参数的最佳组合以生产具有所需机械质量的复合材料.使用方差分析评估这些变量对每个输出变量的重要性.为了建立多个性能指标,构建了一种基于混合灰色的自适应神经模糊推理系统(ANFIS)模型.从所获得的验证结果来看,证明了进化模型能够准确预测.
Introduction
Natural fiber–reinforced composites (NFRCs) have attracted considerable attention from manufacturers and academics in recent decades for leveraging these materials’ applications for several industrial uses due to their lower cost, lightweight, and biodegradability. The exaction for and use of natural fiber is increasing due to environmental concerns. In this context, few researchers have explored the implications of life cycle and environmental impact evaluations on the processing of NFRCs in a literature study on the same. Researchers have found that NFRCs have superior mechanical properties such as flexural, tensile, impact, and interlaminar strengths. Further, they exhibit the best heat, water absorption, and tribological properties, making them a better choice for various structural applications (Sanjay et al. Citation2018; Girijappa et al. Citation2019; Ramesh et al. Citation2020; Vinod et al. Citation2020). Delamination may appear in natural fibers due to the lessened interference bonding amid the fiber and the polymer which affects the mechanical properties. Hence, there is a need to create hybrid laminated composites with the use of natural and synthetic fibers to enhance the required mechanical properties where the stacking order has been discovered to have a indicative effect on the desired characteristics (Balakrishnan et al. Citation2016; Feng et al. Citation2019; Karthi et al. Citation2019; Khan, Srivastava, and Gupta Citation2018; Sabeel Ahmed et al. Citation2012; Das et al. Citation2021). In specific, biofibers are viewed as an associable alternative to carbon fiber–reinforced composites in automobile applications. Jute fiber is an eminent and popular biofiber, considering its typical characteristics such as dimensional stability, ease of availability, and exceptional mechanical properties (Krishnasamy et al. Citation2020; Kumar, Nandal, and Gupta Citation2021; Lotfi et al. Citation2021). Jute fiber is a dominant material that significantly improves the quality of components in several applications, particularly in construction of buildings. Researchers have found that the fiber boosts tensile and compressive strengths (Maraveas Citation2020; Maslinda et al. Citation2017; Monteiro et al. Citation2016; Paul, Gouda, and Bhowmik Citation2021). Also, jute fabrics coated with bitumen emulsion and unsaturated polyester, which were exposed to γ-radiation, exhibited superior properties (Akter et al. Citation2018, Citation2020). So far, researchers investigated the properties of fabricated composites. However, few researchers studied the tribological behavior of a jute fiber composites according to the ASTM standards. The investigation revealed that fiber bonding significantly impacts tribological properties (Chaudhary, Kumar Bajpai, and Maheshwari (Citation2018); (Raju et al. Citation2013; Singh and Zafar Citation2020; Yallew, Kumar, and Singh Citation2015). Jute fiber is a dominant material that significantly improves the properties in a variety of applications, particularly in building construction.
Consequently, the composites were reinforced to improve the desirable properties. However, fillers significantly minimize the downside of the reinforced fibers and further enhance the desired properties. (Jagadeesh et al. Citation2020; Akter et al. Citation2020)). Various in-organic fillers such as aluminum oxide, boron carbide, silicon carbide, and graphene were studied in this regard. Compared to other fillers, boron carbide exhibited the best elastic properties (Sayer Citation2014), and studies on wear phenomenon on inclusion of aluminum oxide portrayed a remarkable decrease in wear rate (Arpitha et al. Citation2017). Also, flexural and tensile strength was enhanced with significant proportions of fillers (Vinay et al. Citation2021; Hemath et al. Citation2020; Narayana and Gopalan Citation2022). Likewise, the inclusion of graphene powder exhibited a considerable increase in composite strength (Ganapathy et al. Citation2021). However, natural materials such as rosewood dust and padauk wood dust (Dinesh et al. Citation2020), carbonized coconut shell powder (Jenish et al; Akter et al. Citation2020), wood apple shell powder (Nagaraja Setty et al. Citation2020, Citation2021), wood charcoal (Das et al. Citation2021), fish bone, and egg shell powders as a filler materials were also endeavored (Abhishek et al. Citation2018; Ganesan et al. Citation2018). The properties of the prepared laminates were evaluated using the response surface methodology (RSM). With the use of statistical and mathematical approaches, a model was developed for analyzing a large number of input variables (Venkatachalam et al. Citation2021).
Determining the suitable process variable to achieve better performance in manufacturing is difficult for all industries. While investigating the multiple performance measures, the enhancement of one parameter may influence the other parameter. Multi-criteria decision-making (MCDM) is one of the best alternative approaches for determining the solutions in various applications. Grey relational grade (GRA) is one among the MCDM approach used in solving these kinds of problems (Kumar et al. Citation2021; Rajak et al. Citation2019; Rao et al. Citation2019). Even though GRA has various merits, the information obtained from this approach is unclear. To avoid such demerits, a decision-making tool supported by artificial intelligence was developed and implemented, resulting in a significant improvement in the process. ANFIS (adaptive neuro-fuzzy inference system) is a tool for accomplishing the desired objectives by amalgamating the benefits of neural network and fuzzy systems (Kharwar, Kumar Verma, and Singh Citation2020; Manikandan et al. Citation2019; Prakash et al. Citation2022; Thejasree and Krishnamachary Citation2022; Manikandan et al. Citation2019).
Developing and analyzing the process variables in the preparation of jute fiber composites need considerable attention. In this regard, variables (particle type, size, and percentage weight) as well as dependent variables (tensile stress and modulus, increase in weight %, flexural stress, and modulus) were given much attention in this work. Furthermore, an attempt is made to construct a decision-making tool (Hybrid Intelligent Decision-Making Tool-Grey ANFIS), which is being used to foresight the desired output measure.
Materials and methods
Jute woven fiber, LY-556 epoxy resin, and XY-54 hardener were procured from Vruksha Natural products, Guntur, while the ceramic particles were obtained from Nice Chemicals, Trichy, Tamil Nadu. The woven fiber is chopped according to the mold’s dimensions. Composites made of woven jute fiber and ceramic particles are produced using a mold box with dimensions of 300 × 300 × 3 mm3. These composite laminates are fabricated with the precise measurements needed. The entire process starts by introducing the resin into the dye. The mold is then sealed, and pressure is applied on the top side of the other plate which is used to enclose the bottom dye.
RSM is an inimitable technique used for modeling. RSM analyzes the data mathematically, statistically, and graphically, and it produces one or many outputs that are predisposed by different input variables as well. Also, the RSM approach works to develop mathematical relationships. The experiments in this work were created using the Box–Behnken Design (BBD) method. Accordingly, the design is obtained and the composites are fabricated. The various levels of process variables that are chosen are mentioned in . The Instron machine-make 8801 was used for the tensile and flexural testing, and the specimens were prepared in compliance with ASTM standards as D3039-15 and D790-15, respectively, as shown in . The water absorption test is carried out in line with ASTM D 570 specifications.
Table 1. Process parameters.
outlines the sequence of experiments that must occur in order for the samples to be prepared.
Table 2. Combination of process variables for composite preparation with coded/uncoded values.
ANFIS model
Despite its numerous advantages, the output measure in the GRA method is better and commonly used for multi-aspect optimization, containing some unclear and uncertain information. With cutting-edge intelligent decision-making tools, the conventional MCDM approach may be supported. Combining these tools enhances process control, which leads to better performance. A strong model with the least amount of error was combined to create the ANFIS, a smart evaluation tool. Some soft computing strategies are more practical when precise mathematical statistics are not available. The soft computing approach deviates from the conventional one in that it yields accurate results and lowers ambiguity.
Results and discussions
The RSM-BBD methodology is used to prepare composites with different combinations. A total of 15 composite specimens were prepared, and the samples are mechanically tested to determine the desired mechanical characteristics. This section explores the effects of process combination upon preferable mechanical properties.
Influence of critical variables on tensile characteristics
The effects of critical variables on tensile characteristics of fabricated specimens are depicted in . The graph demonstrates that at medium levels of particle type and size, and lower levels of weight % of the particle, the tensile characteristics of fiber composite are higher. It should also be observed that the “type of particle,” a process variable, affects the tensile properties of the prepared composite specimens. At medium levels of particle type and size, increased filler content reduces the flexibility of the composite, which is a significant finding. As a result, the composite loses its capability to withstand loads and becomes more brittle.
Influence of critical variables on flexural characteristics
The response of process parameters on the flexural characteristics of the tested fiber composites is shown in . According to the illustration, the flexural properties of fiber composite are higher for medium levels of particle type and size and lower level for particle weight %. Herein, the particle type is a significant parameter that influences the flexural characteristics of the composite samples. The composite’s flexural characteristics increase with the addition of B4C particle of medium size. The elasticity and brittleness of the composite diminish as particle weight % increases.
Process variables’ effect on weight gain as a percentage (%)
shows how processing conditions affect the growth in weight % of the samples. The illustration shows that the intermediate level of particle type, greater level of particle size and particle weight % show better results for the water absorption capacity of the fabricated composites.
ANOVA for evaluating the significance of process variables
shows the results of a quantitative approach on the considered process variables for selected performance parameters during the production of fiber composites. According to the statistical analysis, the particle weight % is the critical process parameter for the majority of the identified performance metrics. The results of analysis of variance (ANOVA) highlight a correlation between the response and the analysis.
Table 3. Analysis of variance results.
Multiple regression models to achieve the desired outcome
A regression analysis is performed for the desired output, and the relationship between the desirable performance variables and the model parameters is conferred. For selected performance measures, the evolved linear, quadratic, interaction, and second-order full model equations are depicted in Equations (1–5).
Contour plot analysis on the effect of parameters
Contour plot is utilized to find the location of highest or lowest response predicted from the process. It is especially useful when a maximum or minimum response is anticipated in the contour region inside or near the data range. Contour plots are extremely useful for investigating the combined effects of several control parameters on output characteristics. The contour plots are shown in .
present the contour plots showing the combined effects of the particle type, particle size, and particle weight % on the tensile characteristics. According to the plots, the tensile stress and modulus are maximum at the medium particle type and particle size level.
The contour plots, presented in , are extracted to study the influence of particle type, particle size, and weight % on flexural properties. It is found from the plots that the maximum flexural strength and modulus are achieved for the amalgamations of the medium level of particle type and particle size and low level of particle weight %.
The water absorption capacity of the prepared composites is analyzed, which is shown in . During the study on the influence of process parameters on water absorption, it is found that water absorption capacity is improved when the particle type is medium, the particle size is large, and the particle weight % is high.
Evolution of ANFIS model for jute fiber composites
The ANFIS framework is evolved using the MATLAB toolbox to predict the intended multi-performance indicator. This work’s ANFIS model has one output parameter and five input parameters. The built model is trained and thereafter utilized to imagine the required measurements. The training details involved in this study are mentioned in .
Table 4. Training parameters for ANFIS model.
The 243 rules in the ANFIS model were generated with “trimf,“ through the input. Grey theory is one of the conventional approaches engaged in attaining the Grey Relational Coefficient and is given as input data. For further processing, the outcomes that are evaluated are normalized. The ANFIS editor for jute fiber composites is shown in . The same is involved in estimating the chosen MPIs (ANFIS-GRG). represent the FIS editor and rule viewer, respectively.
Interpretation on ANFIS analysis to envisage GRG
Typical surface plots for determining process variables’ effect on jute fiber composites using ANFIS-GRG are shown in . The impact of GRC of flexural strength with GRC of flexural modulus, tensile strength, tensile modulus, and increase in weight % based on ANFIS-GRG is studied. It is evident from that the mid level of Grey relational coefficient of flexural strength and flexural modulus is better and improved from ANFIS-GRG. depicts that similar findings are made at intermediate level of tensile modulus. It is noted from showing ANFIS-GRG that a higher level of Grey relational coefficient of flexural strength and a midlevel of tensile strength are finer and improved and likewise, the higher level of % increase in weight.
Analysis on performance of the developed ANFIS model
Here, is the observed value,
is the predicted value from the model,
is the average of the observed values, and n is the number of experiments. The error values obtained from Equations (6–13) are represented in . Performance analysis highlighting minimum error is shown in .
Table 5. Performance analysis of Grey-ANFIS model error.
The error values obtained from Equations (6–13) are represented in .
Analyzed model efficiency
The model’s efficiency is also analyzed using Equations (14) and (15), and the values obtained from this analysis are depicted in .
Table 6. Performance analysis of Grey-ANFIS model efficiency.
The assessment of the fabricated composites is evaluated using the proposed ANFIS model, and the results are compared with those obtained through experimentation. A significant relation was found between actual and desired results. EquationEquations (14)(14)
(14) and (Equation15
(15)
(15) ) are used to assess the performance of the developed model as shown in .
The foreseen values of jute fiber composites obtained using the created model are compared with the corresponding experimental results. It was found that a considerable relationship exists between experimental findings and the values obtained from the considered model. A visual comparison of the observed values between GRG and ANFIS-GRG is shown in . The comparison demonstrated a close association between the tested and developed models.
Conclusions
BBD technique is used in this work to obtain possible combinations of operating parameters. The performance measures used in this study are tensile characteristics, flexural properties, and increase in weight %. A three-level/three-parameter model is adopted. The compression molding machine has been employed to fabricate the composites. The mechanical characteristics of the fabricated composites are investigated. ANOVA is used to identify the relevance of individual process variables. According to the ANOVA assessment, particle type is the influential process variable. Mathematical correlations are established, and the R2 values of the produced models are within limits, indicating that the predicted model is suitable for future assessment. The Grey system theory is considered to evaluate the multi-performance index and the GRC values generated by this study and to construct a hybrid ANFIS-GRG model. The model’s competency is demonstrated by its performance evaluation.
Highlights
Box–Behnken Design approach is used for designing the experiments.
Compression molding machine is used to fabricate the composites.
ANOVA is used to identify the relevance of individual process variables.
Particle type is the most influential process variable.
Hybrid Intelligent Decision-Making Tool-Grey ANFIS is used to predict the desired output measure.
Disclosure statement
No potential conflict of interest was reported by the author(s).
References
- Abhishek, S., M. R. Sanjay, R. George, S. Siengchin, J. Parameswaranpillai, and C. Iulian Pruncu. 2018. Development of new hybrid phoenix pusilla/carbon/fish bone filler reinforced polymer composites. Journal of the Chinese Advanced Materials Society. 6 (4): 553–23. doi:10.1080/22243682.2018.1522599.
- Akter, N., S. Chandra Das, S. A. Grammatikos, J. Saha, and M. Ahmad Khan. 2020. Development of sustainable jute geotextiles by bitumen emulsion and polyester resin: Effect of gamma radiation. Journal of Engineered Fibers and Fabrics 15:15. doi:10.1177/1558925020957969.
- Akter, N., J. Saha, S. Chandra Das, and M. Ahmad Khan. 2018. Effect of bitumen emulsion and polyester resin mixture on the physico-mechanical and degradable properties of jute fabrics. Fibers 6:3. doi:10.3390/fib6030044.
- Arpitha, G. R., M. R. Sanjay, P. Senthamaraikannan, C. Barile, and B. Yogesha. 2017. Hybridization effect of sisal/glass/epoxy/filler based woven fabric reinforced composites. Experimental Techniques. 41 (6): 577–84. doi:10.1007/s40799-017-0203-4.
- Balakrishnan, P., M. J. John, L. Pothen, M. S. Sreekala, and S. Thomas. 2016. Natural fibre and polymer matrix composites and their applications in aerospace engineering. Advanced Composite Materials for Aerospace Engineering Elsevier Ltd. doi:10.1016/b978-0-08-100037-3.00012-2.
- Chaudhary, V., P. Kumar Bajpai, and S. Maheshwari. 2018. Studies on mechanical and morphological characterization of developed jute/hemp/flax reinforced hybrid composites for structural applications. Journal of Natural Fibers 15 (1):80–97. doi:10.1080/15440478.2017.1320260.
- Das, S. C., S. Ashek-E-Khoda, M. Abu Sayeed, D. P. Suruzzaman, S. Aninda Dhar, S. A. Grammatikos, and S. A. Grammatikos. 2021. On the use of wood charcoal filler to improve the properties of natural fiber reinforced polymer composites. Materials Today Proceedings 44:926–29. doi:10.1016/j.matpr.2020.10.808.
- Das, S. C., D. Paul, S. A. Grammatikos, M. A. B. Siddiquee, S. Papatzani, P. Koralli, J. M. M. Islam, M. A. Khan, S. M. Shauddin, R. A. Khan, et al. 2021. Effect of stacking sequence on the performance of hybrid natural/synthetic fiber reinforced polymer composite laminates. Composite Structures 276(August):114525. doi:10.1016/j.compstruct.2021.114525.
- Dinesh, S., P. Kumaran, S. Mohanamurugan, R. Vijay, D. Lenin Singaravelu, A. Vinod, M. R. Sanjay, S. Siengchin, and K. Subrahmanya Bhat. 2020. Influence of Wood Dust Fillers on the Mechanical, Thermal, Water Absorption and Biodegradation Characteristics of Jute Fiber Epoxy Composites. Journal of Polymer Research 27:1. doi:10.1007/s10965-019-1975-2.
- Feng, P., H. Jiyao, S. Peng, C. Gao, Z. Zhao, S. Xiong, and C. Shuai. 2019. Characterizations and interfacial reinforcement mechanisms of multicomponent biopolymer based scaffold. Materials Science and Engineering: C 100:809–25. Elsevier B.V. doi:10.1016/j.msec.2019.03.030.
- Ganapathy, T., R. Sathiskumar, M. R. Sanjay, P. Senthamaraikannan, S. S. Saravanakumar, J. Parameswaranpillai, and S. Siengchin. 2021. Effect of graphene powder on banyan aerial root fibers reinforced epoxy composites. Journal of Natural Fibers 18 (7): 1029–36. doi:10.1080/15440478.2019.1675219.
- Ganesan, K., C. Kailasanathan, M. R. Sanjay, P. Senthamaraikannan, and S. S. Saravanakumar. 2018. A new assessment on mechanical properties of jute fiber mat with egg shell powder/nanoclay-reinforced polyester matrix composites. Journal of Natural Fibers 17 (4): 482–90. doi:10.1080/15440478.2018.1500340.
- Girijappa, T., Y. Gowda, S. Mavinkere Rangappa, J. Parameswaranpillai, and S. Siengchin. 2019. Natural fibers as sustainable and renewable resource for development of eco-friendly composites: A comprehensive review. Frontiers in Materials 6 (September):1–14. doi:10.3389/fmats.2019.00226.
- Hemath, M., S. Mavinkere Rangappa, V. Kushvaha, H. Nath Dhakal, and S. Siengchin. 2020. A comprehensive review on mechanical, electromagnetic radiation shielding, and thermal conductivity of fibers/inorganic fillers reinforced hybrid polymer composites. Polymer Composites 41 (10):3940–65. doi:10.1002/pc.25703.
- Jagadeesh, P., Y. Gowda Thyavihalli Girijappa, M. Puttegowda, S. Mavinkere Rangappa, and S. Siengchin. 2020. Effect of natural filler materials on fiber reinforced hybrid polymer composites: An overview. Journal of Natural Fibers 1–16. doi:10.1080/15440478.2020.1854145.
- Karthi, N., K. Kumaresan, S. Sathish, S. Gokulkumar, L. Prabhu, and N. Vigneshkumar. 2019. An overview: Natural Fiber reinforced hybrid composites, chemical treatments and application areas. Materials Today: Proceedings 27:2828–34. doi:10.1016/j.matpr.2020.01.011.
- Khan, M. Z., S. K. Srivastava, and M. K. Gupta. 2018. Tensile and flexural properties of natural fiber reinforced polymer composites: A review. Journal of Reinforced Plastics and Composites 37 (24):1435–55. doi:https://doi.org/10.1177/0731684418799528.
- Kharwar, P. K., R. Kumar Verma, and A. Singh. 2020. Simultaneous optimisation of quality and productivity characteristics during machining of multiwall carbon nanotube/epoxy nanocomposites. Journal of Mechanical Engineering 1–19. doi:10.1080/14484846.2020.1794511.
- Krishnasamy, P., G. Rajamurugan, S. Aravindraj, and P. Edwin Sudhagar. 2020. Vibration and wear characteristics of aloevera/flax/hemp woven fiber epoxy composite reinforced with wire mesh and BaSO4. Journal of Natural Fibers 1–17. doi:10.1080/15440478.2020.1835782.
- Kumar, S., S. Kumar Ghoshal, P. Kumar Arora, and L. Nagdeve. 2021. Multi-variable optimization in die-sinking EDM process of AISI420 stainless steel. Materials and Manufacturing Processes 36 (5): 572–82. doi:10.1080/10426914.2020.1843678.
- Kumar, P., V. Nandal, and A. Gupta. 2021. Erosion behavior of marble dust filled jute fiber reinforced composite. International Journal of Fracture and Damage Mechanics 7 (1):27–33.
- Lotfi, A., L. Huaizhong, D. Viet Dao, and G. Prusty. 2021. Natural fiber–reinforced composites: a review on material, manufacturing, and machinability. Journal of Thermoplastic Composite Materials 34 (2):238–84. doi:10.1177/0892705719844546.
- Manikandan, N., D. Arulkirubakaran, D. Palanisamy, and R. Raju. 2019. Influence of wire-EDM textured conventional tungsten carbide inserts in machining of aerospace materials (Ti–6al–4v Alloy). Materials and Manufacturing Processes 34 (1):103–11. doi:10.1080/10426914.2018.1544712.
- Manikandan, N., K. Balasubramanian, D. Palanisamy, P. M. Gopal, D. Arulkirubakaran, and J. S. Binoj. 2019. Machinability analysis and ANFIS modelling on advanced machining of hybrid metal matrix composites for aerospace applications. Materials and Manufacturing Processes 34 (16):1866–81. doi:10.1080/10426914.2019.1689264.
- Maraveas, C. 2020. Production of sustainable construction materials using agro-Wastes. Materials 13:2. doi:10.3390/ma13020262.
- Maslinda, A. B., M. S. Abdul Majid, M. J. M. Ridzuan, M. Afendi, and A. G. Gibson. 2017. Effect of water absorption on the mechanical properties of hybrid interwoven cellulosic-cellulosic fibre reinforced epoxy composites. Composite Structures 167:227–37. doi:10.1016/j.compstruct.2017.02.023.
- Monteiro, A. S., B. Leonardi, H. Savastano, and J. Baruque-Ramos. 2016. Tururi palm fibrous material (manicaria saccifera gaertn): Physicochemical characterization. Green Materials 3 (4): 120–31. doi:10.1680/jgrma.15.00024.
- Nagaraja Setty, S., V. Kumar, G. Goud, S. Peramanahalli Chikkegowda, S. Mavinkere Rangappa, and S. Siengchin. 2020. Characterization of chemically treated limonia acidissima (wood apple) shell powder: Physicochemical, thermal, and morphological properties. Journal of Natural Fibers 19:4093–104. doi:10.1080/15440478.2020.1853925.
- Nagaraja Setty, S., V. Kumar, G. Govardhan, S. Mavinkere Rangappa, and S. Siengchin. 2021. Raw and chemically treated bio-waste filled (limonia acidissima shell powder) vinyl ester composites: Physical, mechanical, moisture absorption properties, and microstructure analysis. Journal of Vinyl and Additive Technology 27 (1):97–107. doi:10.1002/vnl.21787.
- Narayana, S. L., and V. Gopalan. 2022. Investigations on mechanical properties of jute fibre reinforced with aluminium oxide fortified epoxy composite. Advances in Materials and Processing Technologies 1–26. doi:10.1080/2374068X.2022.2096836.
- Paul, R., K. Gouda, and S. Bhowmik. 2021. Effect of different constraint on tribological behaviour of natural fibre/filler reinforced polymeric composites: A review. Silicon 13 (8): 2785–807. doi:10.1007/s12633-020-00613-z.
- Prakash, C., P. Senthil, N. Manikandan, and D. Palanisamy. 2022. Investigations on machinability characteristics of cast aluminum alloy based (LM 26+ graphite+ fly ash) hybrid metal matrix composites for automobile components. Materials and Manufacturing Processes 37 (7):748–63.
- Rajak, D. K., D. D. Pagar, P. L. Menezes, and E. Linul. 2019. Fiber-reinforced polymer composites: manufacturing. Properties, and Applications 11:10. doi:10.3390/polym11101667.
- Raju, B., R. BheemappaSuresha, B. Kanthraju, and B. S. G. Kanthraju. 2013. Investigations on mechanical and tribological behaviour of particulate filled glass fabric reinforced epoxy composites. Journal of Minerals and Materials Characterization and Engineering 01:160–67. doi:10.4236/jmmce.2013.14027.
- Ramesh, M., C. Deepa, L. Rajesh Kumar, M. R. Sanjay, and S. Siengchin. 2020. Life-cycle and environmental impact assessments on processing of plant fibres and its bio-composites: A critical review. Journal of Industrial Textiles 51:1–25. doi:10.1177/1528083720924730.
- Rao, Y. S., N. Subbarao Mohan, N. Shetty, and B. Shivamurthy. 2019. Drilling and structural property study of multi-layered fiber and fabric reinforced polymer composite: A review. Materials and Manufacturing Processes 34 (14):1549–79. doi:10.1080/10426914.2019.1686522.
- Sabeel Ahmed, K., V. M. Syed Sha Khalid, S. J. Amith Kumar, and S. J. Amith Kumar. 2012. Dry sliding wear behavior of SiC/Al2O3 filled jute/epoxy composites. Materials and Design 36 (April):306–15. doi:10.1016/j.matdes.2011.11.010.
- Sanjay, M. R., P. Madhu, M. Jawaid, P. Senthamaraikannan, S. Senthil, and S. Pradeep. 2018. Characterization and properties of natural fiber polymer composites: A comprehensive review. Journal of Cleaner Production 172 (January): 566–81. doi:10.1016/J.JCLEPRO.2017.10.101.
- Sayer, M. 2014. Elastic properties and buckling load evaluation of ceramic particles filled glass/epoxy composites. Composites Part B: Engineering 59:12–20. doi:10.1016/j.compositesb.2013.11.016.
- Singh, M. K., and S. Zafar. 2020, “Effect of layering sequence on mechanical properties of woven kenaf/jute fabric hybrid laminated microwave-processed composites. Journal of Industrial Textiles 51:2731S–52S. doi:10.1177/1528083720911219.
- Thejasree, P., and P. C. Krishnamachary. 2022. Weldability analysis and ANFIS modelling on laser welding of inconel 718 thin sheets. Materials and Manufacturing Processes 37 (10):1190–202. doi:10.1080/10426914.2022.2039694.
- Venkatachalam, G., V. Suthenthiraveerappa, J. Stanley David, A. R. A. Jeyanthi Subramanian, C. Ping Jen, and C. -P. Jen. 2021, Experimental and numerical analyses on the buckling characteristics of woven flax/epoxy laminated composite plate under axial compression. Polymers 13:7. doi:10.3390/polym13070995.
- Vinay, S. S., M. R. Sanjay, S. Siengchin, and C. V. Venkatesh. 2021. Effect of Al2O3 nanofillers in basalt/epoxy composites: Mechanical and tribological properties. Polymer Composites 42 (4):1727–40. doi:10.1002/pc.25927.
- Vinod, A., M. R. Sanjay, S. Suchart, and P. Jyotishkumar. 2020. Renewable and sustainable biobased materials: An assessment on biofibers, biofilms, biopolymers and biocomposites. Journal of Cleaner Production 258. doi:10.1016/j.jclepro.2020.120978.
- Yallew, T. B., P. Kumar, and I. Singh. 2015. Sliding Behaviour of Woven Industrial Hemp Fabric Reinforced Thermoplastic Polymer Composites. International Journal of Plastics Technology 19 (2):347–62. doi:10.1007/s12588-015-9121-4.