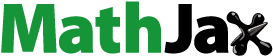
ABSTRACT
The present research is an extension of a previous paper published by the authors. In the first part of the research, the flexural properties of Washingtonia filifera (WF) fiber-reinforced plaster composite treated with sodium bicarbonate were explored using response surface method statistics. In the current study, the data was analyzed using artificial neural network tool. The main objective of the current research is to model the flexural properties of an environmentally friendly gypsum biocomposite reinforced with treated and untreated WF fibers using response surface method and artificial neural networks. For this purpose, the study reports a comparative approach between models predicted by response surface methodology (RSM) and artificial neural networks (ANNs). The statistical results as root mean square error and coefficient of determination reveal that ANN and RSM are effective techniques for bending properties prediction of plaster/WF biocomposites. In addition, ANN and RSM models correlate highly with the experimental data. However, artificial neural network model displayed more accuracy.
摘要
本研究是作者先前发表的论文的延伸. 在研究的第一部分中,使用响应面法统计研究了碳酸氢钠处理的Washingtonia filifera(WF)纤维增强石膏复合材料的弯曲性能. 在当前的研究中,使用人工神经网络工具分析数据. 当前研究的主要目的是使用响应面方法和人工神经网络来模拟由经处理和未经处理的WF纤维增强的环保石膏生物复合材料的弯曲性能. 为此,该研究报告了响应面方法(RSM)和人工神经网络(ANN)预测模型之间的比较方法. 均方根误差和确定系数的统计结果表明,ANN和RSM是预测石膏/WF生物复合材料弯曲性能的有效技术. 此外,ANN和RSM模型与实验数据高度相关. 然而,人工神经网络模型显示出更高的准确性.
1. Introduction
Mineral-bonded panels offer many applications in flooring, interior wall coverings, partitions, and ceilings. Compared to other composite wood materials, these boards (such as fiberboard bonded to gypsum) are more durable and display greater strength when subjected to fire exposure. However, the addition of certain quantity of natural fibers provides a reinforcement effect, whereas above that quantity, they can have a negative effect. Gypsum is moderately important in the construction field relative to other aggregates, despite its many benefits such as abundant product availability, reduced cost, rapid setting, and lighter weight. Its principal limitation is its brittleness and poor tensile strength, which reduce its potential applications. Currently, there is a tendency to investigate sustainable materials for building construction, using natural fibers as a reinforcement material for lime plasters (Le Bella et al. Citation2014; Troëdec et al. Citation2011; Di). The use of natural fibers in construction materials is attractive in general due to their high accessibility worldwide, and their low cost, density, thermal conductivity, and recyclability (Boumaaza, Belaadi, and Bourchak Citation2022b; Cheng et al. Citation2023a, Citation2023b; Iucolano et al. Citation2015), making them a good alternative to synthetic fibers.
Different researchers have investigated the influence of incorporating various types of natural fibers in the plaster matrix, concrete, or cement mortar on the mechanical properties of the composite material. Some fibers used as reinforcement in the plaster matrix were sisal fiber (Di Bella et al. Citation2014), ramie (Cheng et al. Citation2021), kenaf fibers, jute (Adda et al. Citation2021; Tabet et al. Citation2021), hemp, olive fibers, flax (Iucolano et al. Citation2018; Makhlouf et al. Citation2020) and date palm fiber (Belakroum et al. Citation2018; Lekrine et al. Citation2022).
Ravikumar et al. examined the impact of eco-friendly sodium bicarbonate treatment using response surface methodology on the delamination and surface roughness of jute fiber reinforced polyester composites (Ravikumar et al. Citation2022). Sumesh et al. focused on the impact of bio-waste loading from banana, pineapple, and coconut plants in epoxy-based composites for mechanical applications (Sumesh et al. Citation2021). Recently, Cheng et al. investigated the penetration behaviors of 3D printed continuous ramie yarns woven and non-woven polylactic acid (PLA) biocomposites reinforced. To evaluate the effect of the 3D printed architecture according to a novel interlaced print path and a conventional (unidirectional and orthogonal) print path, the authors performed quasi-static penetration tests (Cheng et al. Citation2023a, Citation2023b).
The hydrophilic properties of natural fibers and their inadequate adherence to the matrix is a drawback (Belaadi et al. Citation2020; Zuber et al. Citation2012), resulting in a limited ability to transfer the matrix stresses to the fiber. The dimensional changes in the fibers can cause micro-cracking of the biocomposite, decreasing its performance and properties (Belaadi et al. Citation2022; Dembri et al. Citation2022). In order to enhance fiber adhesion to the matrix and increase the biocomposite strength, fiber surface modification is usually effected by chemical treatments that modify the fiber surface. The most commonly adopted chemical approach is alkali treatment, in which the fibers are treated with alkaline solution (NaOH) to obtain better properties. Many studies have been conducted on the effects of this treatment on the properties of biocomposites, such as solution concentration, temperature, and immersion time (Cai et al. Citation2015; Saaidia, Belaadi, and Haddad Citation2022).
According to some experimental results, a variety of parameters, such as fiber type, treatment type, and concentration percentage significantly influence flexural properties. It is, thus, necessary to search for the best fabrication conditions, guaranteeing a compromise between production cost and product characteristics. Evaluating the contribution of different parameters to flexural property improvement takes time and money. Therefore, the focus of most recent studies about biocomposite materials engineering is to predict the biocomposite properties through numerical models with reduced experimentation. Modeling and predicting the mechanical properties of engineering materials requires considerable effort. The natural fiber composites have been tried with ANN, GRA, Taguchi approaches for optimization. Sumesh and Kanthavel applied the Taguchi method and gray relational analysis to find the optimized process parameter for the impact hammer test on the damping behavior of epoxy-based sisal/black/alumina nanocomposites (Sumesh and Kanthavel Citation2020). In addition recently, the authors investigated the factors contributing to the mechanical stability of epoxy-based composites reinforced with sisal and banana natural fibers using Taguchi optimization based on the gray relational approach for the compression molding technique (Sumesh and Kanthavel Citation2022).
RSM and ANN are widely used to develop approximation models to optimize the response (output variable), which is affected by many independent parameters (input variables). ANN and RSM were applied in civil engineering to model the behavior of materials, optimize construction structures, and predict mixing proportions of concrete composition based on experimental investigations. Cai et al. adopted machine learning methods (Cai et al. Citation2022) and response surface methodology based on experimental results to predict 3D printed material properties and interfacial properties (Cai et al. Citation2022). Nazerian et al. (Citation2018) used ANN and RSM to model the bending strength of gypsum board reinforced with fibers; others researchers (Nambiar and Ramamurthy Citation2006) suggested models correlating the mixture proportion with concrete strength and density using RSM. Recently, Boumaaza et al. examined the performance of gypsum mortar biocomposites reinforced with three natural fibers, specifically sisal, flax, and jute, treated chemically with NaOH by changing the fiber length (5, 10, 20 mm) and NaOH concentrations (0%, 1.5%, 2%, and 4%) (Boumaaza, Belaadi, and Bourchak Citation2022a). A variety of parameters affecting the mechanical properties of gypsum biocomposites were examined in the literature, but limited information has been reported concerning fiber effect on the bending properties of biocomposite materials based on gypsum.
To the best of our knowledge, this is the first report proposing RSM and ANN models in predicting the flexural properties of untreated and sodium bicarbonate treated WF-reinforced gypsum biocomposites. To this end, the study reports a comparative approach between the models predicted by RSM and ANN as a function of immersion time fiber and percentage of NaHCO3 treatment solution to produce environmentally friendly and economical biocomposites.
2. Materials and methods
2.1. Materials used
The plaster used was a local Algerian product, commercially available for interior purposes. Washingtonia filifera (WF) is locally grown in the Guelma region (Algeria). The fibers were manually collected, immediately placed in distilled water to clean the surface, and then dried at room temperature in air to avoid moisture. Thereafter, the fibers were chemically treated using NaHCO3 sodium bicarbonate to generate high-performance fibers with a reduced diameter, which improved matrix/fiber adhesion and mechanical properties. In this process, the WF fibers were tempered in a 5%, 10%, and 20% NaHCO3/water solution for 24, 72, 168, and 240 h. The treated WF fibers were cleaned with distilled water to eliminate the sodium impurities and then cured at 50°C for 24 h in an air oven, before being cooled and stored (Benzannache et al. Citation2021).
2.2. Characterization of the raw materials
The binder chemical composition was performed by thermal simultaneous analysis (DSC-TGA), by means of a NETZSCH STA 449 F3 Jupiter brand. The weighted specimens were specifically heated on alumina plates between 25 and 1000°C with a 10°C/min heating rate in an azote environment.
The binder mineralogical composition was analyzed by X-ray diffraction (XRD), with a Bruker D8 ECO type instrument equipped with a copper anticathode tube with a wavelength Kα1 = 0.15406 nm. The WF fiber density was estimated using a helium pycnometer (MultiVolume Pycnometer 1305, Micromeritics).
2.3. Fabrication process of samples
The biocomposite, consisting of powdered plaster and short WF fibers, was initially homogenized and mixed with water for about 60 s. The 2% fibers incorporated into the gypsum matrix were cut into lengths ranging from 20 mm to 40 mm. The W/P ratio of water to plaster was 0.7. The compound was poured into 4 × 4 × 16 cm open molds and compacted for 40 s using a traditional vibrator. The samples were demolded after 5 h of plaster setting, dried for 72 h at T = 45°C and then stocked at 23–25°C and RH = 48–50% in a chamber until testing. For each sample type, three specimens were tested. The parameters of the developed biocomposites are shown in . The 3-point bending test was performed according to ASTM C348 standard using a 5kN universal testing machine with an axial displacement speed of 1 mm/min at 140 mm span. The displacements at mid-span and the load were controlled with a data acquired system.
Table 1. Experimental results for stress, displacement, and flexural modulus.
2.4. Experimental design approach of plaster biocomposite
The studied orthogonal design outputs were chosen to predict the bending properties of biocomposites through the artificial neural networks and response surface methodology for analysis the influence of input factors on reinforced plaster bending properties. Mix ratios in the investigations were generated by applying a statistical experimental design technique in plaster biocomposites. Three responses were determined: flexural strength (σf), displacement (y), and flexural modulus (Ef). This experimental design included 13 experiments. In this study, two control variables were considered: (1) fiber immersion time and (2) percent concentration of NaHCO3, labeled A and B, respectively, as shown in .
3. Statistical analysis
In previous study, the Analysis of Variance (ANOVA) to validate the resulting experimental data was applied to determine the appropriate model at α = 0.05 level of significance (i.e., for 95% probability). In addition, parameters such as fiber immersion time and NaHCO3 concentration were optimized using RSM to obtain the optimal values of the bending properties. In this study, ANNs inspired by biological neural systems were used to model the nonlinear processes. This approach is based on the weighted sum of inputs obtained by the neuron through (usually nonlinear) activation functions producing outputs (Altun, Kişi, and Aydin Citation2008; Kewalramani and Gupta Citation2006; Yilmaz and Yuksek Citation2009). ANNs typically consist of multiple layers, an input layer, hidden layers, and an output layer. Neurons in the input layer acquire feedback from the outside environment to be transferred to neurons in the hidden layer that are fully weight-connected among themselves (Mansour et al. Citation2004; Qu, Cai, and Chang Citation2018). These neurons may comprise many hidden computing units as represented in the ANN architecture (Sarıdemir Citation2009).
To achieve the ultimate model, R2 was chosen to serve as the primary criterion for choosing which network can generate the best model. Training the neural network was considered a major procedure to acquire accurate results; insufficient training renders ANNs inefficient and can yield imprecise predictions. These experimental inputs were therefore divided into three subsets: 70% were used to train the network, 15% to validate it, and the last 15% for testing purposes. The input numbers determine the neurons in the input layer, and the output numbers determine the neurons in the output layer. The number of neurons in the hidden layer was determined by running multiple neural network tests until the output value (MSE) was at a minimum. For this investigation, the Levenberg-Marquardt algorithm was selected to train the ANN model for predicting the WF reinforced gypsum biocomposite flexural properties.
The accuracy of the generated ANN model was checked through the correlation coefficient (R2) and root mean square error (RMSE) values provided by EquationEquations 1(1)
(1) and Equation2
(2)
(2) (Adewale George, Ighalo, and Marques Citation2021).
4. Results and discussion
4.1. DSC and XRD analysis
The results of the thermal analysis, reported in , revealed the presence of three distinct endothermic and one exothermic peak. The first endothermic peak, around 25–100°C was attributed to moisture, 2nd peak in the range 100–200°C around T = 135°C, attributed to dehydration of the hemihydrate (see EquationEq. (3)(3)
(3) ). Another endothermic peak was detected in the range 600–800°C around T = 700°C, attributed to the decomposition of calcite (see EquationEq. (4)
(4)
(4) ). In addition, an exothermic peak was detected around T = 355°C, and attributed to the polymorphic transition.
Figure 1. (a) XRD of the WF fiber and plaster matrix (H = CaSO4_0.5 H2O; H/A = CaSO4; C = CaCO3) and (b) TG/DSC of the plaster matrix.
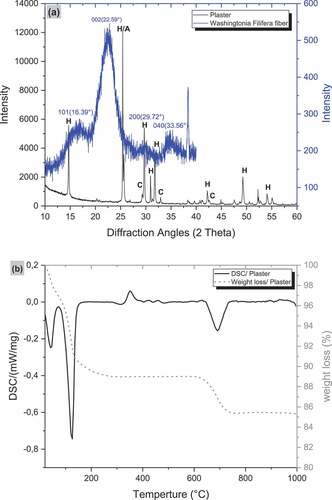
The mineralogical composition performed by XRD confirmed the previous results. In fact, the scheme reported in showed a large peak for anhydrite, seven peaks to hemihydrate, and three to calcite corresponding to the two-theta diffraction. The peak attributed to anhydrite is around (26), for calcite is around (42–29), and for hemihydrate is around (29–54).
4.2. Results of ANN modeling methods
More information on the RSM was discussed in Benzannache et al. (Citation2021), in which the resulting data were explored on the basis of RSM statistics. There was a significant effect of NaHCO3 concentration (A) for σf and Ef (Prob ≤0.05) although it was less significant for y. For the time of treatment (B), it was not significant for σf but more significant for Ef. Their interaction (AB), however, showed a significant effect for σf and less significant for y and Ef. An effect of the NaHCO3 treatment is obviously noticeable and provides a significant improvement in the mechanical properties of all treated specimens. However, the increase is dependent on the NaHCO3 concentration and treatment period. The cracks are less accentuated in the specimens reinforced with 20% NaHCO3 treated fiber for 168 h compared to the other specimens, which obviously explains the improvement of its mechanical properties in bending (Benzannache et al. Citation2021).
The treatment of Doum fiber with 1% NaOH () causes the removal of amorphous materials from the fiber surface leading to a cleaner surface, rough and to the formation of irregular scratches on the surface of the fiber, which increases the fiber-matrix adhesion.
In the current work, the data will be analyzed using ANN statistics. The ANN architecture used in this work is shown in . shows the correlation coefficient during training, validation, testing and all predicted values (output) to actual values (target) (98.98%, 99.44%, and 99.56%) corresponding to all predicted values for σf, y, and Ef, respectively. A well correlation of the experimental response and measured R2 values with the predicted ANN response was observed; therefore, the developed ANN model results based on the experimental data perfectly predicted the tested responses (σf, y, and Ef) among the various models. As a result of having the high value of R2, 2-8-1, 2-7-1, and 2-6-1 were adopted as appropriate architectures to model σf, y, and Ef. Two input nodes (fiber immersion time and percentage of NaHCO3), 8, 7, and 6 nodes to the hidden layer, and 1 node for the output were considered for (σf, y, and Ef), respectively, and the model architectures are shown in and . To evaluate the adequacy of the model, experimental (“real”) values of σf, y, and Ef were compared to the predicted ANN model values represented by the perfect y = x fit line with the predicted and experimental data presenting a high correlation. The majority of the intersection points were clearly observed to be close to the midline. A plot of the ANN outputs from the modeling process is provided in in comparison to the actual data for the gypsum/WF biocomposite across the training, validation, and testing steps. The experiment data variability of the model was reported by the coefficient of determination R2, which showed the model’s prediction accuracy (Macedo et al. Citation2015). The correlation precision for training, testing, and total is (0.9999, 1, 0.9944), (1, 1, 0.9956), and (0.9977, 1, 0.9798) for σf, y, and Ef, respectively, leading to a high level of confidence regarding the output model. The ANN predicted values approached parity level and fit nicely with the experimentally determined ones. Consequently, a confident prediction of the bending properties of gypsum/WF biocomposites can be performed via the ANN approach.
Figure 2. ANN architecture used in this study for experimental (a) strength and (b) displacement and (c) Young modulus data.
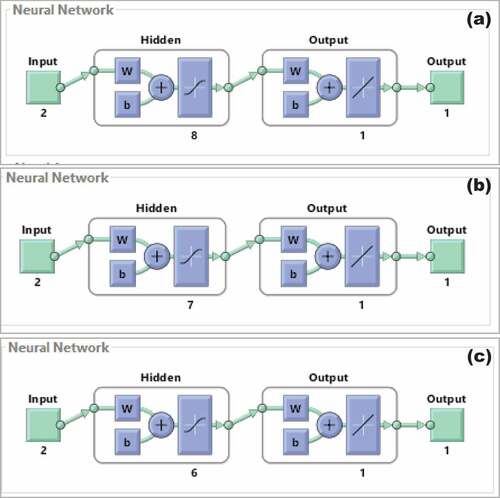
Figure 3. ANN modeling results for biocomposites WF/Plaster (a-c) strength (d-f) displacement and (g-i) Young modulus data.
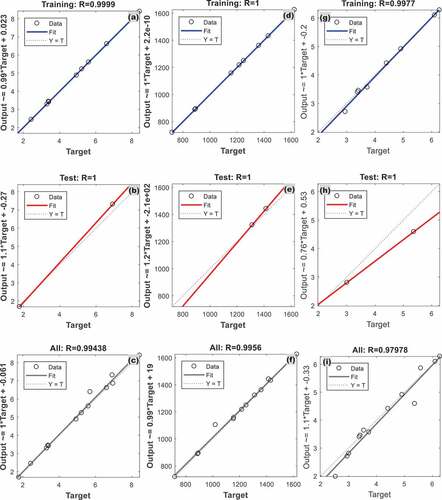
Table 2. ANN tested architectures MSE and R-values for training, validation, and testing of Plaster/WF.
Table 3. Mathematical models obtained with ANN method for different biocomposites.
The histogram of the errors distributed over an interval of 20 bins generated was produced within the model network by means of the errors in the training, validation, and testing as shown in , where the errors were clearly located around the zero line. The training and validation processes were stopped at the third and sixth iteration for σf, y, and Ef models, respectively (). After an optimal validation was registered at 1.4625E–12, 4.0393E–13, and 1.8433E–10 by the gradient at the 6th, 9th, and 7th iteration for σf, y, and Ef respectively, ANN evaluated the stop point to be an ideal one, beyond which the validation bias exceeded accepted tolerances (). The combined interactions of the effects among the inputs and response variables can also be examined with other plotted illustrations, such as the surface contour and 3D plots (Ositadinma, Tagbo, Boumaaza, Belaadi, and Bourchak Citation2021; Ositadinma, Chamberlain, and Elijah Citation2019). These graphs are presented in and applied to show the effect of varying parameters on the flexural properties. The surface graphics were obtained by varying two parameters in the experimental trial range in the model. An interactive effect of NaHCO3 concentration level and fiber immersion time was exhibited. The bending stress, displacement, and Young’s modulus were found to increase to their optimum values with increasing NaHCO3 concentration levels (NaHCO3 = 20); however, these values increased to an optimum immersion time (t = 168 h) and then decreased. This is in good agreement with the results obtained by RSM. According to the surface contour and the 3D plot type, an interactive effect of fiber treatment concentration and immersion time was significant.
Figure 4. ANN distribution of errors results for (a) strength and (b) displacement and (c) Young modulus data.
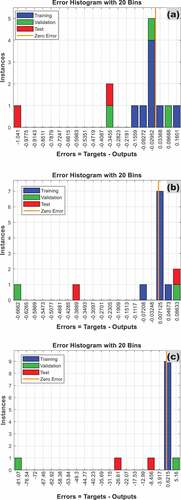
4.3. ANN and RSM analysis of experimental results
An optimization of variables such as NaHCO3 concentration and fiber immersion time with RSM was performed by Benzannache et al. (Citation2021) to attain the optimum values of σf, y, and Ef for the plaster/WF biocomposite. To validate the accuracy of the mathematical models, the correlation between the actual and predicted values for σf, y, and Ef was verified. showed the comparison of the experimentally obtained results and those predicted by the RSM and ANN models for σf, y, and Ef, along with their absolute error percentages. It was found that the predicted results obtained for σf, y, and Ef using both models were in perfect correlation with the actual data. The well relationship between these outcomes verified that the mathematical models were successful in reflecting the predicted outcomes. Also, the performance of the developed RSM and ANN models is statistically measured and is shown in . The determination coefficient (R2) in ANN training, validation, and test for σf takes the value of 99.79, 99.86%, and 1.00%, respectively. The same thing was observed for y and Ef (). The R2 calculated by ANN methods shows more accuracy than RSM models shown in the previous work (Benzannache et al. Citation2021).
show the variations of the residuals of the two prediction approaches. Indeed, the residuals are quite low and regular for the ANN compared to the RSM. The latter model shows a larger deviation than the ANN model. These residuals confirm that the learning procedure, the validation, and the ANN test were performed satisfactorily, with a very low error level, and that the mathematical model is relevant. This is in good agreement with the results obtained by (Boumaaza et al. Citation2022) which indicated that the ANN models performed better than those obtained by the RSM models. However, it is important to mention that the ANN models efficaciously estimated the experimental findings.
5. Conclusions
In the current investigation, the ANN and RSM statistics on the flexural property data of gypsum/WF were reported. The plaster reinforcement with untreated and NaHCO3-treated fibers provided the plaster biocomposite with higher flexural strength, displacement, and elastic modulus than those obtained by raw fibers. The optimal condition for maximum flexural strength, displacement, and flexural modulus showed that treatment with 20% NaHCO3 for a duration of 168 h provided the optimal composition of the gypsum/WF biocomposite. This is probably due to the improvement in the surface condition of chemically treated fibers by this formulation leading to good adhesion between the fibers and the matrix. The formulated ANN predictive models (2:8:1), (2:7:1) and (2:6:1) showed greater flexibility and the ability to exhibit a non-linear relationship for predicting flexural properties. The variations of the residuals are quite small and regular for ANN compared to RSM. This last model shows higher deviation than the ANN one. The comparison of the two RSM and ANN models revealed that the ones predicted by ANN are significantly more robust than those of RSM, as confirmed by their correlation coefficient (R2), close to 1 and their low MSE error. Therefore, the ANN method can be an effective strategy to anticipate values in experimental studies, saving time and cost when performing new experiments.
Biocomposites developed through this process have a significant role to offer new materials for the civil engineering industry. Such as for building walls and other construction elements, and for the production of reinforced gypsum boards.
Highlights
WF fibers treated with NaHCO3 had good adhesion to plaster matrix.
The ANN was used to model and optimize the flexural properties of the plaster/WF biocomposite.
The ANN model was more accurate in its predictive ability than RSM.
Acknowledgements
This research work was funded by Institutional Fund Projects under grant no. (IFPIP:1368-135-1443). The authors gratefully acknowledge technical and financial support provided by the Ministry of Education and King Abdulaziz University, DSR, Jeddah, Saudi Arabia.
Disclosure statement
No potential conflict of interest was reported by the authors.
Additional information
Funding
References
- Adda, B., A. Belaadi, M. Boumaaza, and M. Bourchak. 2021. Experimental investigation and optimization of delamination factors in the drilling of jute fiber–reinforced polymer biocomposites with multiple estimators. International Journal of Advanced Manufacturing Technology 116 (9):2885–18. doi:10.1007/s00170-021-07628-9.
- Adewale George, A., J. O. Ighalo, and G. Marques. 2021. Utilisation of machine learning algorithms for the prediction of syngas composition from biomass bio-oil steam reforming. International Journal of Sustainable Energy 40 (4):310–25. doi:10.1080/14786451.2020.1803862.
- Altun, F., Ö. Kişi, and K. Aydin. 2008. Predicting the compressive strength of steel fiber added lightweight concrete using neural network. Computational Materials Science 42 (2):259–65. doi:10.1016/j.commatsci.2007.07.011.
- Belaadi, A., S. Amroune, Y. Seki, O. Y. Keskin, S. Köktaş, M. Bourchak, A. Dufresne, H. Fouad, and M. Jawaid. 2022. Extraction and characterization of a new lignocellulosic fiber from yucca treculeana l. leaf as potential reinforcement for industrial biocomposites. Journal of Natural Fibers 19 (15):1–16. doi:10.1080/15440478.2022.2054895.
- Belaadi, A., M. Boumaaza, S. Amroune, and M. Bourchak. 2020. Mechanical characterization and optimization of delamination factor in drilling bidirectional jute fibre-reinforced polymer biocomposites. International Journal of Advanced Manufacturing Technology 111 (7–8):2073–94. doi:10.1007/s00170-020-06217-6.
- Belakroum, R., A. Gherfi, M. Kadja, C. Maalouf, M. Lachi, N. El Wakil, and T. H. Mai. 2018. Design and properties of a new sustainable construction material based on date palm fibers and lime. Construction and Building Materials 184:330–43. doi:10.1016/j.conbuildmat.2018.06.196.
- Bella, G., V. F. Di, G. Galtieri, C. Borsellino, and A. Valenza. 2014. Effects of natural fibres reinforcement in lime plasters (kenaf and sisal vs. polypropylene). Construction and Building Materials 58:159–65. doi:10.1016/j.conbuildmat.2014.02.026.
- Benzannache, N., A. Belaadi, M. Boumaaza, and M. Bourchak. 2021. Improving the mechanical performance of biocomposite plaster/Washingtonian filifira fibres using the RSM method. Journal of Building Engineering 33:33. doi:10.1016/j.jobe.2020.101840.
- Boumaaza, M., A. Belaadi, and M. Bourchak. 2021. The effect of alkaline treatment on mechanical performance of natural fibers-reinforced plaster: Part II optimization comparison between ANN and RSM statistics. Journal of Natural Fibers 19 (14):1–16. doi:10.1080/15440478.2021.1964129.
- Boumaaza, M., A. Belaadi, and M. Bourchak. 2022a. THe effect of alkaline treatment on mechanical performance of natural fibers-reinforced plaster: Optimization using RSM. Journal of Natural Fibers 18 (12):2220–40. doi:10.1080/15440478.2020.1724236.
- Boumaaza, M., A. Belaadi, and M. Bourchak. 2022b. Systematic review on reinforcing mortars with natural fibers: Challenges of environment-friendly option. Journal of Natural Fibers 19 (16):1–25. doi:10.1080/15440478.2022.2060408.
- Boumaaza, M., A. Belaadi, M. Bourchak, M. Jawaid, and H. Satha. 2022. Comparative study of flexural properties prediction of Washingtonia filifera rachis biochar bio-mortar by ANN and RSM models. Construction and Building Materials 318:125985. doi:10.1016/j.conbuildmat.2021.125985.
- Cai, M., H. Takagi, A. Norio Nakagaito, K. Kusaka, M. Katoh, and Y. Li. 2015. Influence of alkali concentration on morphology and tensile properties of abaca fibers. Advanced Materials Research 1110:302–05. doi:10.4028/scientific.net/AMR.1110.302.
- Cai, R., K. Wang, W. Wen, Y. Peng, M. Baniassadi, and S. Ahzi. 2022. Application of machine learning methods on dynamic strength analysis for additive manufactured polypropylene-based composites. Polymer Testing 110:107580. doi:10.1016/j.polymertesting.2022.107580.
- Cai, R., W. Wen, K. Wang, Y. Peng, S. Ahzi, and F. Chinesta. 2022. Tailoring interfacial properties of 3D-printed continuous natural fiber reinforced polypropylene composites through parameter optimization using machine learning methods. Materials Today Communications 32:103985. doi:10.1016/j.mtcomm.2022.103985.
- Cai, R., W. Wen, K. Wang, Y. Peng, S. Ahzi, and F. Chinesta. 2022. Tailoring interfacial properties of 3d-printed continuous natural fiber reinforced polypropylene composites through parameter optimization using machine learning methods. Materials Today Communications 32:103985. doi:10.1016/j.mtcomm.2022.103985.
- Cheng, P., Y. Peng, L. Shixian, Y. Rao, A. Le Duigou, K. Wang, and S. Ahzi. 2023a. 3D printed continuous fiber reinforced composite lightweight structures: A review and outlook. Composites Part B: Engineering 250:110450. doi:10.1016/j.compositesb.2022.110450.
- Cheng, P., Y. Peng, K. Wang, A. Le Duigou, S. Yao, and C. Chen. 2023b. Quasi-static penetration property of 3D printed woven-like ramie fiber reinforced biocomposites. Composite Structures 303:116313. doi:10.1016/j.compstruct.2022.116313.
- Cheng, P., K. Wang, X. Chen, J. Wang, Y. Peng, S. Ahzi, and C. Chen. 2021. Interfacial and mechanical properties of continuous ramie fiber reinforced biocomposites fabricated by in-situ impregnated 3D printing. Industrial Crops and Products 170:113760. doi:10.1016/j.indcrop.2021.113760.
- Dembri, I., A. Belaadi, M. Boumaaza, and M. Bourchak. 2022. Tensile behavior and statistical analysis of Washingtonia filifera fibers as potential reinforcement for industrial polymer biocomposites. Journal of Natural Fibers 19 (16):1–16. doi:10.1080/15440478.2022.2069189.
- Iucolano, F., D. Caputo, F. Leboffe, and B. Liguori. 2015. Mechanical behavior of plaster reinforced with abaca fibers. Construction and Building Materials 99:184–91. doi:10.1016/j.conbuildmat.2015.09.020.
- Iucolano, F., B. Liguori, P. Aprea, and D. Caputo. 2018. Evaluation of bio-degummed hemp fibers as reinforcement in gypsum plaster. Composites Part B: Engineering 138:149–56. doi:10.1016/j.compositesb.2017.11.037.
- Kewalramani, M. A., and R. Gupta. 2006. Concrete compressive strength prediction using ultrasonic pulse velocity through artificial neural networks. Automation in Construction 15 (3):374–79. doi:10.1016/j.autcon.2005.07.003.
- Lekrine, A., A. Belaadi, A. Makhlouf, S. Amroune, M. Bourchak, H. Satha, and M. Jawaid. 2022. Structural, thermal, mechanical and physical properties of Washingtonia filifera fibres reinforced thermoplastic biocomposites. Materials Today Communications 31:103574. doi:10.1016/j.mtcomm.2022.103574.
- Macedo, M. N. Q., J. J. M. Galo, L. A. L. de Almeida, and A. C. D. C. Lima. 2015. Demand side management using artificial neural networks in a smart grid environment. Renewable and Sustainable Energy Reviews 41:128–33. doi:10.1016/j.rser.2014.08.035.
- Makhlouf, A., A. Belaadi, S. Amroune, M. Bourchak, and H. Satha. 2020. Elaboration and characterization of flax fiber reinforced high density polyethylene biocomposite: Effect of the heating rate on thermo-mechanical properties. Journal of Natural Fibers 19 (10):1–14. doi:10.1080/15440478.2020.1848737.
- Mansour, M. Y., M. Dicleli, J. Y. Lee, and J. Zhang. 2004. Predicting the shear strength of reinforced concrete beams using artificial neural networks. Engineering Structures 26 (6):781–99. doi:10.1016/j.engstruct.2004.01.011.
- Nambiar, E. K. K., and K. Ramamurthy. 2006. Influence of filler type on the properties of foam concrete. Cement and Concrete Composites 28 (5):475–80. doi:10.1016/j.cemconcomp.2005.12.001.
- Nazerian, M., M. Kamyabb, M. Shamsianb, M. Dahmardehb, and M. Kooshaa. 2018. Comparison of response surface methodology (RSM) and artificial neural networks (ANN) towards efficient optimization of flexural properties of gypsum-bonded fiber boards. CERNE 24 (1):35–47. doi:10.1590/01047760201824012484.
- Ositadinma, I., N. J. T. Chamberlain, and O. C. Elijah. 2019. Optimum process parameters for activated carbon production from rice husk for phenol adsorption. Current Journal of Applied Science and Technology 36 (6):1–11. doi:10.9734/cjast/2019/v36i630264.
- Qu, D., X. Cai, and W. Chang. 2018. Evaluating the effects of steel fibers on mechanical properties of ultra-high performance concrete using artificial neural networks. Applied Sciences 8 (7):1120. doi:10.3390/app8071120.
- Ravikumar, P., G. Rajeshkumar, P. Manimegalai, K. R. Sumesh, M. R. Sanjay, and S. Siengchin. 2022. Delamination and surface roughness analysis of jute/polyester composites using response surface methodology: consequence of sodium bicarbonate treatment. Journal of Industrial Textiles 51 (1_suppl):360S–77S. doi:10.1177/15280837221077040.
- Saaidia, A., A. Belaadi, and A. Haddad. 2022. Moisture absorption of cork-based biosandwich material extracted from quercussuber l. plant: ann and fick’s modelling. Journal of Natural Fibers 19 (16):1–18. doi:10.1080/15440478.2022.2072996.
- Sarıdemir, M. 2009. Predicting the compressive strength of mortars containing metakaolin by artificial neural networks and fuzzy logic. Advances in Engineering Software 40 (9):920–27. doi:10.1016/j.advengsoft.2008.12.008.
- Sumesh, K. R., and K. Kanthavel. 2020. Synergy of fiber content, Al2O3 nanopowder, NaOH treatment and compression pressure on free vibration and damping behavior of natural hybrid-based epoxy composites. Polymer Bulletin 77 (3):1581–604. doi:10.1007/s00289-019-02823-x.
- Sumesh, K. R., and K. Kanthavel. 2022. Grey relational optimization for factors influencing tensile, flexural, and impact properties of hybrid sisal banana fiber epoxy composites. Journal of Industrial Textiles 51 (3\_suppl):4441S–59S. doi:10.1177/1528083720928501.
- Sumesh, K. R., V. Kavimani, G. Rajeshkumar, S. Indran, and G. Saikrishnan. 2021. EFfect of banana, pineapple and coir fly ash filled with hybrid fiber epoxy based composites for mechanical and morphological study. Journal of Material Cycles & Waste Management 23 (4):1277–88. doi:10.1007/s10163-021-01196-6.
- Tabet, Z., A. Belaadi, M. Boumaaza, and M. Bourchak. 2021. Drilling of a bidirectional jute fibre and cork-reinforced polymer biosandwich structure: ANN and RSM approaches for modelling and optimization. International Journal of Advanced Manufacturing Technology 117 (11–12):3819–39. doi:10.21203/rs.3.rs-503708/v1.
- Troëdec, M. L., P. Dalmay, C. Patapy, C. Peyratout, A. Smith, and T. Chotard. 2011. Mechanical properties of hemp-lime reinforced mortars: influence of the chemical treatment of fibers. Journal of Composite Materials 45 (22):2347–57. doi:10.1177/0021998311401088.
- Yilmaz, I., and G. Yuksek. 2009. Prediction of the strength and elasticity modulus of gypsum using multiple regression, ANN, and ANFIS models. International Journal of Rock Mechanics and Mining Sciences 46 (4):803–10. doi:10.1016/j.ijrmms.2008.09.002.
- Zuber, M., K. Mahmood Zia, I. Ahmad Bhatti, Z. Ali, M. U. Arshad, and M. J. Saif. 2012. Modification of cellulosic fibers by UV-irradiation. Part II: After treatments effects. International Journal of Biological Macromolecules 51 (5):743–48. doi:10.1016/j.ijbiomac.2012.07.001.