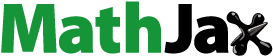
ABSTRACT
As part of the ongoing renaissance of phage biology, more phage genomes are becoming available through DNA sequencing. However, our understanding of the transcriptome architecture that allows these genomes to be expressed during host infection is generally poor. Transcription start sites (TSSs) and operons have been mapped for very few phages, and an annotated global RNA map of a phage – alone or together with its infected host – is not available at all. Here, we applied differential RNA-seq (dRNA-seq) to study the early, host takeover phase of infection by assessing the transcriptome structure of Pseudomonas aeruginosa jumbo phage ɸKZ, a model phage for viral genetics and structural research. This map substantially expands the number of early expressed viral genes, defining TSSs that are active ten minutes after ɸKZ infection. Simultaneously, we record gene expression changes in the host transcriptome during this critical metabolism conversion. In addition to previously reported upregulation of genes associated with amino acid metabolism, we observe strong activation of genes with functions in biofilm formation (cdrAB) and iron storage (bfrB), as well as an activation of the antitoxin ParD. Conversely, ɸKZ infection rapidly down-regulates complexes IV and V of oxidative phosphorylation (atpCDGHF and cyoABCDE). Taken together, our data provide new insights into the transcriptional organization and infection process of the giant bacteriophage ɸKZ and adds a framework for the genome-wide transcriptomic analysis of phage–host interactions.
Introduction
Knowledge about transcriptome architecture and key elements is important to achieve a global understanding of gene regulation in a bacterium of interest. This applies not only to the bacteria, but also to their natural predators: (bacterio)phages. Yet, while global transcriptomic technology, in particular RNA-seq, have accelerated the assignment of gene structure on the whole-genome level for many bacteria, our understanding of the architecture of phage transcriptomes has been trailing. An improved characterization of phage transcriptomes is necessary for several reasons, which include the growing appreciation of temporal regulation of phage gene expression during an infection, a frequently observed concomitant usurpation of the host’s gene expression machinery, and a paucity of functional knowledge for the vast majority of phage genes [Citation1,Citation2].
Our current understanding of the transcriptome architecture of phages is largely based on the dissection of individual promoters in a handful of model phages, primarily phages λ, T7, P22 and T4. These analyses typically used time-consuming, gene-by-gene approaches such as primer extension, RNase protection assays, or 5´RACE (rapid amplification of cDNA ends) [Citation3–5]. Such classical approaches were used for the recent identification of promoters in the Pseudomonas phage ɸKZ [Citation6–8]. The giant phage ɸKZ is particularly interesting since this virus encodes two sets of RNA polymerase (RNAP) β and β´-like subunits, resulting in a transcription scheme that functions independently of the host transcription machinery [Citation7,Citation9,Citation10]. One set of RNAP subunits is associated with the virion (vRNAP), and when it accesses the host cell together with the viral DNA, it leads to transcription initiation of 28 early viral promoters with a conserved AT-rich consensus element [Citation7].
Global maps of transcription initiation events in the viral genome are lacking. Ideally, one would want to obtain transcription initiation maps during different stages of infection for both the phage and its host, because the host response is a critical discriminator in the early stage of infection, as apparent by CRISPR-CAS defence systems. Over the past decade, specific methods have been developed to map bacterial transcriptomes at single-nucleotide resolution. Of these, differential RNA-seq (dRNA-seq) [Citation11] has been successfully applied for transcription start site (TSS) mapping to dozens of different microbes in a global scale [Citation12]. This global TSS knowledge permits to annotate 5´untranslated regions (5´UTRs), promoter regions, and monocistronic versus polycistronic (operon) transcriptional units. It also allows experimental identification of (non)-coding genes, missed by DNA-based genome annotations.
TSS mapping by dRNA-seq utilizes the property that primary transcripts in bacteria possess a 5´-triphosphate (5´PPP) end, whereas processed RNA transcripts mostly carry a 5´-monophosphate (5´P) group. Discrimination is achieved through terminator exonuclease (TEX) treatment that degrades the processed transcripts – prior to cDNA synthesis and sequencing. This causes a relative enrichment of reads at primary 5´PPP ends (compared to the untreated sample), which can be used for TSS annotation [Citation11,Citation13]. The analysis of dRNA-seq TSS mapping data has recently become more straightforward with the development of ANNOgesic [Citation14], a bioinformatics pipeline that integrates the analysis and annotation of TSSs as well as other transcriptional features.
In the present work, we have mapped TSSs by dRNA-seq for the first time to a phage, studying infection of P. aeruginosa by ɸKZ to reveal TSSs in both the viral and host genomes simultaneously, specifically during redirection of the transcription machinery and metabolism towards viral replication. At this time point of infection, we discover bacterial TSSs as a direct response to viral entry and reveal differentially regulated genes involved in oxidative phosphorylation and host virulence. This pioneering application of high-throughput analysis of phage and host transcriptomes establishes a pipeline for similar analysis of many other phage–host interactions.
Material and methods
Bacterial strain, growth conditions and bacteriophage preparation
P. aeruginosa strain PAO1 (DSM 22644) was grown in standard Lysogeny Broth (LB) medium at 37°C and 220 rpm, without any supplementation for all experiments. Bacteriophage ɸKZ (HER number 153) was amplified by liquid cultivation: Fresh LB was inoculated with an overnight culture of PAO1 and grown at 37°C to an optical density of OD600 0.3, followed by infection with a high titre lysate of ɸKZ (PFU/mL). After incubation for 5 min at room temperature, the mixture was incubated at 37°C and continuously shaking at 220 rpm until lysis was visible. Overnight incubation at 4°C was followed by centrifugation (6,000
g for 20 min) and phage lysate was purified by filtration (0.45 µm). The titre was estimated by soft-agar overlay to ensure a high titre phage stock.
Phage ɸKZ was kindly provided by ‘Félix d´Herelle Reference Center for Bacterial Viruses’, University Laval.
Infection condition and RNA extraction
Early exponentially grown PAO1 cells (OD600 = 0.3) were infected with ɸKZ with a multiplicity of infection [Citation15] (MOI) of 15 to ensure a synchronous infection of the culture. At MOI 15, over 95% of bacterial cells were infected within 10 min (Supplementary Fig. 1). After incubation for 5 min at room temperature, the infection continued at 37°C and 220 rpm for 5 min. Collected samples were treated with stop mix (95% EtOH, 5% phenol, pH 5.5) and immediately snap-frozen in liquid nitrogen to halt all cellular processes and preserve the early infection state. Afterwards, the samples were thawed on ice, and pelleted by centrifugation for 20 min at 4°C at 4,500 rpm. Cell lysis was caused by the activation of lysozyme and total RNA was isolated by performing Hot Phenol extraction, followed by ethanol precipitation. DNase I treatment (Fermentas) was carried out and the integrity of RNA samples was accessed on a 1.0% agarose gel. To ensure a high infection rate of PAO1 cells, cell counts of samples taken 10 min post-infection should contain less than 5% surviving cells compared to control culture after overnight incubation.
dRNA-seq library construction and sequencing
Total RNA was extracted from ɸKZ-infected and uninfected P. aeruginosa PAO1 cells. The RNA was then divided into two pools, named TEX- and TEX+. Samples were examined by capillary electrophoresis and fragmented using ultrasound (4 pulses of 30 sec at 4°C), followed by T4 Polynucleotide Kinase (NEB) treatment. TEX+ samples were subjected to Terminator-5´-phosphate-dependent exonuclease to enrich primary transcripts whereas TEX- samples remained untreated. For cDNA synthesis RNA samples were poly(A)-tailed and remaining 5´PPP structures were removed. After ligation of an RNA adapter to the 5´P end, first-strand cDNA synthesis was performed using an oligo(dT)-adapter primer and M-MLV reverse transcriptase. PCR amplification of cDNAs was conducted using a high-fidelity DNA polymerase and cDNAs were purified using the Agencourt AMPure XP kit (Beckman Coulter Genomics). Quality control was carried out by capillary electrophoresis. All described procedures were performed by Vertis Biotechnology (Germany). Libraries were sequenced as 75 bp strand-specific single-end Illumina libraries on the Illumina Nextseq 500 system at the Core Unit SysMed, University Würzburg.
Analysis of dRNA-seq data and prediction of genomic features
The raw data are available at GEO under the accession GSE153067. Obtained Illumina reads in FASTQ format were used as input to perform adapter clipping and quality trimming via the FASTX toolkit version 0.10.1. The tool READemption (version 0.4.3) was used to perform the alignment and differential expression analysis of the processed data. In this process, poly(A)-tails were removed and all sequences shorter than 12 nt were discarded. Genome sequences and annotations for P. aeruginosa PAO1 (AE004091.2) and ɸKZ (AF399011.1) were acquired from NCBI. The differential expression analysis was performed using DEseq 2 included in the READemption pipeline. Further, analysis of the untreated and TEX treated samples was performed using ANNOgesic (version 1.0.0) using the pipelines features for TSSs, transcript detection, sRNA prediction, terminator prediction, UTR detection, sORF prediction and promoter motif prediction. In short, all features were run with standard settings with following modifications: Primary TSSs were allowed to be 500 nt upstream of the associated genes, while TSSs not more than 8 nt apart were annotated as one TSS. Further manual curation was performed to remove false positives by removing PAO1 and ɸKZ TSSs with values <50 and <30. 5´UTRs were allowed to be up to 500 nt and the transcripts detected could be elongated up to 25 nt in order to join an annotated gene. sORFs were predicted with the size criteria to be between 20 nt and 500 nt of length. In this range, the predicted transcript could be elongated 20 nt upstream and 50 nt downstream to include a start codon (ATG, TTG, GTG) or stop codon (TTA, TAG, TGA).
Sequence analysis for the detection of promotor motifs
Identification of motifs in the regions upstream of the TSSs was performed using MEME (Bailey & Elkan, 1994). For the dataset generated from the uninfected PAO1 cells, regions of 50 base pairs upstream of the TSSs were extracted and direct motif discovery with MEME was carried out. Concerning the dataset of ɸKZ-infected cells: First, σ70 promoters were filtered by screening all upstream sequences (50 nt) for the presence of motifs corresponding to specifically applied position-specific scoring matrices for the −10 and −35 boxes for subsequent clustering and filtration. Motif scores were used to filter putative σ70 promoters by clustering, using a Gaussian mixture model with two components and full covariance matrices. The set of remaining sequences was subjected to MEME analysis for additional motif discovery (non-σ70 motifs). Discovered motifs could be assigned to RpoN, FliA or AlgU σ factors by comparison to motifs identified in a previous ChIP-seq study [Citation16].
Results and discussion
The primary transcriptome of uninfected P. aeruginosa PAO1
Prior to mapping phage TSSs during infection, we performed dRNA-seq on ɸKZ´s uninfected host, P. aeruginosa. This bacterium harbours a 6.3 Mb genome with 5,688 proteins [Citation17], endowing it with huge metabolic capabilities and adaptability to a wide range of environments. The genome encodes no fewer than 24 σ factors and 550 additional transcription factors, representing one of the largest reported bacterial transcriptional regulatory networks [Citation18–20]. Several studies on this opportunistic pathogen have leveraged RNA-seq techniques [Citation21–24]. There have also been studies that led to the annotation of TSSs in this organism although, in the absence of phage infection [Citation25,Citation26].
Our dRNA-seq analysis was performed on P. aeruginosa PAO1 (DSM 22644) grown in LB medium to the early exponential phase (OD600 of 0.3) (), a growth phase in which the phage infection proceeds rapidly based on the proper physiological state of the host and therefore serves as a standard condition of this system [Citation7,Citation27]. TEX- and TEX+ libraries were prepared from biological duplicates which, after cDNA sequencing and mapping to the PAO1 genome, yielded ~2.1–2.7 and ~9.1–9.5 million uniquely aligned reads, respectively (, samples 1.1 and 1.2). ANNOgesic analysis, followed by manual curation, identified a total of 3,108 TSSs (). This number is in agreement with the total of 3,159 TSSs annotated in a previous RNA-seq by others who analysed PAO1 grown to late exponential phase in LB media [Citation26].
Table 1. Summary of the number of uniquely aligned reads (excluding rRNA reads) mapped to the P. aeruginosa PAO1 (DSM22644) reference sequence and the ɸKZ sequence. For all libraries, PAO1 cells were grown to an OD of 0.3 in LB media. Samples infected in this growth state and cultures were harvested after 10 min of infection
Figure 1. Experimental workflow of dRNA-seq and TSS annotation in PAO1. (A) Total RNA of P. aeruginosa PAO1 was extracted, during or without ɸKZ infection. To distinguish between processed and primary transcripts, one library was treated with terminator exonuclease (+TEX) whereas the other was not (-TEX). After sequencing and genome-analysis by ANNOgesic, specific enrichment patterns were visualized and annotated according to those TSSs. (B) TSS categories and their overlaps are displayed in a Venn diagram. The majority of detected TSSs are associated with multiple categories. However, 1,821 out of 3,108 were annotated as primary TSSs. (C) Schematic representation of TSS categorization in primary (P), secondary (S), internal (I), antisense (as) and orphan (O). (D) Partition of 5´UTR lengths of 2,187 TSSs (primary and secondary) of PAO1 transcripts by their size. Transcripts with a 5´UTR lengths < 10 nt were defined as leaderless transcripts. Most transcripts possess a 5´UTR length between 21–30 nt whereas no transcripts were detected with 5´UTR lengths >300 nt
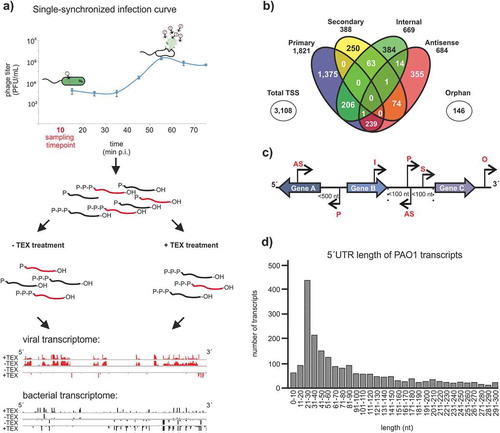
We assigned TSSs to five classes, depending on their genomic location and expression levels, as described previously [Citation11]. pTSS (primary TSS of a gene or operon), sTSS (secondary TSS showing lower expression level compared to pTSS for the same gene), iTSS (internal TSS located inside a gene), aTSS (antisense TSS to a gene within 100 nt distance), and oTSS (orphan TSS, no nearby gene) (). The 1,821 pTSSs annotated here cover 32.0% of annotated genes within the PAO1 genome. We benchmarked TSS assignments with data from Gill et al., 2018 [Citation26] and the PseudoCAP database [Citation17], which contains 51 experimentally validated TSSs for this strain. 49.3% of the detected TSSs were in precise correspondence to the same starting nucleotide with previous data [Citation26] including those for pslA (PA2231), ohrR (PA2849) and PA3720 that have additionally been determined by 5´RACE or primer extension analysis [Citation28–30]. TSS assignments and categorization are listed in Supplementary Table 1.
To facilitate subsequent analysis of phage-induced changes in the transcriptional landscape of the host, we used these TSS data to annotate 5´UTRs of mRNAs. We also reannotated non-coding genes of putative small regulatory RNAs (sRNAs) where possible. 5´UTRs were found to be up to 300 nt in length (average and median lengths: 71 nt and 46 nt, respectively). The majority of 5´UTRs were found to span 21–30 nt (). These numbers echo the average 5´UTR length previously determined in E. coli (20–40 nt) or P. aeruginosa strain PA14 (median length: 47 nt) [Citation25,Citation31]. We also predicted a total of 55 proteins to be synthesized from leaderless mRNAs (pTSS <10 bp of the start codon), which may require a distinct type of translation initiation [Citation32].
P. aeruginosa is rife with sRNAs, many of which associate with the sRNA chaperone Hfq [Citation33,Citation34] and act by base-paring mechanisms to either repress or activate the translation of trans-encoded target mRNAs [Citation35–38]. Although many more have been predicted [Citation39], PseudoCAP [Citation17] currently lists only 43 ncRNAs for this strain, most of which were expressed during late exponential/stationary phase [Citation40]. Our dRNA-seq analysis identified 173 putative sRNAs expressed during early exponential phase; 22 of these sRNAs had been reported to be expressed during other growth conditions, for example, SPA0071, P8, sRNA1466 and PA2633.1 (Supplementary Table 2). Taken together, our PAO1 data provided an excellent benchmark for the quality of the dRNA-seq analysis and constituted a building block for investigating the host response to ɸKZ infection, as further described below.
Single-nucleotide resolution transcriptome map of bacteriophage ɸKZ
ɸKZ is an obligatory lytic giant bacteriophage whose genome possess 369 open reading frames (ORFs) and six tRNA genes, organized in 134 operons [Citation7]. Its infection cycle is completed in 56 min () and the present transcriptional schema of ɸKZ, inferred from general expression analysis and gene-by-gene validation, consists of early, middle, and late genes. These genes were proposed to be transcribed from three specific promoters associated with 28 early, 6 middle and 16 late viral genes, respectively; for three early genes, a TSS had been manually determined by primer extension analysis [Citation7].
To study the phage as it infects its host, RNA samples were generated from exponentially grown P. aeruginosa PAO1 cells (OD600 = 0.3) infected by a high-titre lysate of ɸKZ with an MOI of 15 (see Material and Methods; Supplementary Fig. 1). This MOI was chosen to ensure most cells will be in the same infection state. Infection was stopped by snap freezing the samples in liquid nitrogen after 10 minutes to ensure the capture of RNA samples from an early state of infection [Citation7,Citation41]. We assumed that the remodelling of the host transcriptome and the transition of the host cell to a phage-producing cell by viral compounds is mainly carried out at this time point based on the expression of early regulatory phage genes, like the Dip protein [Citation7,Citation42].
Out of the 2,283,888 uniquely aligned reads (excluding rRNA reads) originating from host and phage (, samples 2.1 and 2.2), 874,410 unique reads were assigned to the ɸKZ genome, representing 38.0% of the total. This percentage is in agreement with previous RNA-seq [Citation7], reporting 36.9% of all reads to be of phage origin.
The dRNA-seq approach allowed us to distinguish between primary, secondary, internal, and antisense TSSs in the viral genome for the first time. We identified 65 TSSs (58 pTSSs, 2 sTSSs) associated with 73 genes in the viral genome, showing that phage TSSs can also have multiple associations (, Supplementary Table 3). The majority of the 65 TSS detected in this study are associated with annotated early and middle genes of the virus. In addition, eight out of nine previously described TSSs [Citation7] were also identified by our dRNA-seq approach. Both observations serve as a validation of the accuracy of our global approach. Two sTSSs were associated with uncharacterized gene PHIKZ054 (predicted scaffold protein, encoded within a six-gene operon of unknown function) and with PHIKZ298, encoding a structural protein of the virus particle (). Based on orphan TSS prediction, we were able to annotate sORF007 as a new hypothetical gene in ɸKZ, associated with a primary and a secondary TSS. This gene is annotated for close relatives like Pseudomonas phage KTN4 (KU521356.1; KTN4_059), SL2 (NC042081.1; gp317) and fnug (MT133560.1; fnug_53). A comparison with the PFAM database did not yield any conserved protein domain for the gene. Our analysis also defined 5´UTRs with typical lengths in the range of 31–40 nt, thus very similar to the situation in the host bacterium (see above, ).
Figure 2. TSS annotation of ɸKZ and motif searches. (A) Two examples of 5´-end enrichment patterns observed by TEX treatment of ɸKZ transcripts. For PHIKZ260, a primary TSS was detected whereas a primary and secondary TSS could be distinguished for PHIKZ054. The distance between the TSS and the AUG start codon is indicated. (B) Venn diagram provides a detailed view on detected viral TSSs and shows their overlaps. The majority of all 65 viral TSSs is categorized as primary, whereas just a few can be associated to the other four categories. (C) 5´UTR lengths of ɸKZ mRNAs are depicted. The majority possess a length between 31 and 40 nt, which is longer than the median length of host transcripts. Interestingly, no leaderless transcript could be detected. (D) Giant bacteriophage ɸKZ early promoter sequence found for 32 sequences. Motif searches were conducted by MEME analysis, using 50 nt sequences upstream of all primary TSSs. (E) For 28 TSSs, one more promoter motif was found using MEME analysis and characterized by a TACT motif followed by an A-repeat
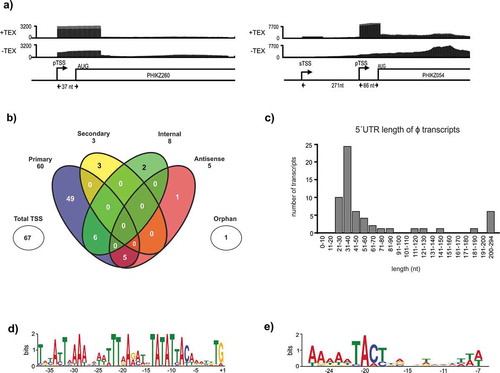
Figure 3. Transcriptional map of giant bacteriophage ɸKZ. Combined depiction of the viral genome of phage ɸKZ and annotation of transcriptional start sites (TSSs) and terminators by dRNA-seq data. TSSs are found mainly on the leading strand and at the beginning of ORFs. The classification scheme of TSSs follows Sharma et al., 2010 and is visualized in Figure 2b in detail. The legends depicts already annotated gene types denoted by colour (dark grey: structural head proteins, light grey: structural proteins, yellow: non-virion RNAP-associated subunits (PHIKZ71/73/74/55/123), dark blue: virion-associated RNAP subunits (PHIKZ080/149/178/180), cyan: scaffold protein (PHIKZ054), light blue: major capsid protein (PHIKZ120), orange: Dip protein (PHIKZ037), black: tRNAs-Thr/Asn/Asp/Met/Pro/Leu, purple: AMG proteins (PHIKZ155/188/214/217/235/305/306), dark green: nuclear-shell associated proteins (PHIKZ039/105), light green: tail tip protein (PHIKZ131) and tail-associated endolysin (PHIKZ181), arrows: TSS, blue line: terminator, red arrow: new annotated gene (predicted as sORF007), light grey box: operon
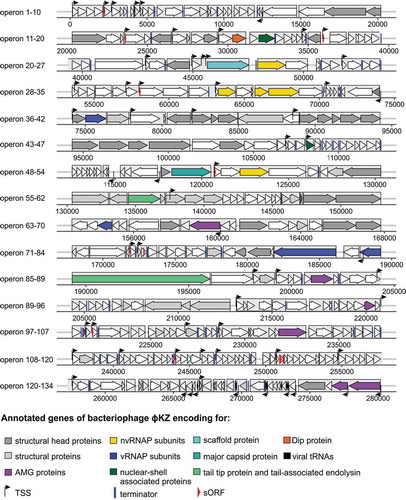
We note an intriguing strand bias: 49 (75%) TSSs were located on the leading strand, reflecting the unidirectional distribution of early genes of ɸKZ, as reported by Ceyssens et. al., 2014. Interestingly, two TSSs where shown to be associated with viral tRNAs which are transcribed on the lagging strand. This association had not been observed previously but seems to make sense given that these tRNAs are necessary for a rapid mRNA translation and consequently for efficient phage reproduction [Citation43].
ɸKZ encodes two subsets of β/β´subunits needed for virion and non-virion-associated RNAPs that drive the transcription of early and middle/late viral genes, respectively. To predict promoter sequences for binding of the vRNA polymerase, nucleotides −1 to −50 located upstream of all TSSs were analysed using MEME. This revealed a viral early promoter motif encompassing an extended −10 box and a T-rich motif upstream of position −19 in 32 sequences (). This motif further refines the previous motif extracted from 28 promoters of the early genes, three of which were experimentally validated by 5´RACE [Citation7].
To ensure that no motifs in the remaining 33 sequences were overlooked, we performed a second MEME analysis on these. This led to the identification of a second promoter motif in 28 sequences that share similarity to the promoter motif for middle genes of ɸKZ [Citation7]. It is defined by a TACT motif, centred at 22 nt upstream of a TSS and is preceded by an A-rich repeat. For four sequences this motif was slightly shifted. This motif was observed for 14 genes each, on the leading (PHIKZ133) and lagging (PHIKZ305, PHIKZ306 and PHIKZ301) strands, respectively (). This highlights the contrasting expression dynamics associated with early and middle phage-encoded genes. Interestingly, PHIKZ054 was found to have the early gene promoter motif associated with a pTSS and the middle gene promoter motif with an sTSS. This implies that the expression of this gene is regulated both during the early and middle stages of viral gene expression.
Transcriptome architecture and non-coding RNAs of ɸKZ
Bacteriophage ɸKZ ORFs are organized in 134 operons, of which 103 are located on the leading strand [Citation6,Citation7]. To investigate potential new regulatory elements and improve the annotation of this phage, we analysed our TEX- data set in more detail. ANNOgesic predicted 54 terminators, 37 of which are of high confidence. This expands the number of known terminator sites +60.8 % (23 known by the previous study) in this bacteriophage [Citation44] (Supplementary Table 4). Compared to the 286 kb genome of giant virus vB_Pae_PA5oct, which has 36 putative terminators [Citation45], this number seems plausible.
While small proteins in bacteria have recently been discovered as constituent parts of different signalling pathways and for in the interplay with phages [Citation46–49], the detection and functional characterization of viral small proteins is limited [Citation50,Citation51]. ANNOgesic ´s sORF prediction resulted in eight potential small ORFs (sORFs) in this giant bacteriophage of which one was intergenic (Supplementary Table 5). Here, we are defining an sORF as a potential ORFs of a size of 30–150 bp and a ribosome binding site upstream of the start codon [Citation14]. However, a more detailed analysis of the individual sORF predictions showed that these were either already part of an existing gene in ɸKZ and related phages (e.g. Pseudomonas phage SL2, Pseudomonas phage PA7) or lacked a start codon and were hence discarded.
Comparative analysis of gene expression of PAO1 in uninfected vs. infected condition
Lytic bacteriophages, as obligate parasites, are dependent on the redirection of the transcription machinery and metabolism of their host towards phage replication [Citation41,Citation52]. Giant bacteriophages such as ɸKZ attain this objective by phage-encoded RNAP subunits, auxiliary metabolic phage genes (AMGs) [Citation53,Citation54] and other host-targeting factors, including the RNA degradosome interacting protein, Dip [Citation7,Citation41,Citation42]. In this regard, our dRNA-seq of the joint phage-host transcriptome, also allowed us to investigate the remodelling of the host transcriptome early (10 min) after ɸKZ attack.
As an output of the dRNA-seq approach, quantifiable RNA-seq data are generated from uninfected and infected P. aeruginosa PAO1 cells. In the previous sections, we showed that these data are reliable based on the overlap to previous studies, including TSS annotations. In order to investigate what transcription factor might govern the response to the phage infection, we analysed the promoter regions in the uninfected data set. To this end, we extracted 50 (−1 to −50) bases upstream of the 1,812 primary TSSs for MEME analysis [Citation55]. The σ70 promoters were found to represent the majority of all promoter sequences, as expected (Supplementary Table 1, Supplementary Fig. 2). Furthermore, three different other promoter motifs were detected besides σ70 sequences in the uninfected data set: RpoN, FliA and AlgU (Supplementary Table 1, Supplementary Fig. 2). The RpoN sigma factor is known to be involved inter alia in the transcription of genes necessary for nitrogen assimilation and motility [Citation56,Citation57], whereas the FliA factor activates genes required for flagellin biosynthesis and adherence [Citation18,Citation58,Citation59].
In the data set generated from ɸKZ-infected PAO1 cells, σ70 promoter sequences again proved the most detected sequences. However, searching for motifs in the 50 nt long sequences upstream of the 423 TSSs found exclusively during ɸKZ infection (Supplementary Table 6), a strong enrichment of AlgU motifs was detected (78 out of 423 sequences) (Supplementary Table 6, Supplementary Fig. 2). The P. aeruginosa σ factor AlgU, which shows 79% amino acid similarity to E. coli σE, is involved in the regulation of resistance genes towards oxidative and heat-shock stress [Citation18,Citation60,Citation61]. AlgU was co-purified with RNAP from PAO1 cells infected by phage PEV2 [Citation62], supporting the upregulation of this sigma factor during infection. Furthermore, AlgU binds the promoter region of a key enzyme in the alginate biosynthesis pathway, algD [Citation63]. In the context of phage infection, this observation matches the upregulation of the cdrAB gene cluster, which is also involved in cell aggregation and the initiation of biofilm production [Citation64,Citation65]. These observations might indicate beneficial effects associated with infection progression because an increased physical cell to cell contacts would enable a fast infection of neighbouring cells by the newly released phage particles.
Repressed genes are associated with oxidative phosphorylation and virulence regulation
The majority of differentially expressed PAO1 transcripts were downregulated after ɸKZ infection. We considered genes with a log2 fold change below −2 as strongly downregulated and 57 transcripts fit this criterion (Supplementary Table 7). The strongest downregulated gene clusters were associated with lipopolysaccharide (LPS) biosynthesis (; cell wall). Of these, the genes wzx and wzy were the most strongly downregulated transcripts (log2 fold changes of −3.08 and −2.93) and wbpK, a NAD-dependent epimerase, has a TSS only detected during ɸKZ infection. Additional downregulated transcripts included type III secretion system (T3SS) genes, and among others the needle protein (pscF) and the ATPase (PA1697) as well as regulators of virulence genes, for example, VqsR [Citation66]. ExsA (PA1713), the transcriptional activator of the P. aeruginosa T3SS [Citation67], was also downregulated. This type of bacterial response, i.e., modulation of LPS biosynthesis and membrane-associated proteins, is reminiscent of responses to other lytic bacteriophages [Citation68,Citation69]. Several transcriptional regulators associated with cell motility and quorum-sensing systems were also downregulated in the ɸKZ infection condition.
Table 2. List of downregulated PAO1 transcripts during phage infection
Other gene clusters (PA5553-5558 and PA1317-PA1321) downregulated during infection encode the F-type ATPase of complex V (β, γ, δ and ε subunits) and the cytochrome bo3 ubiquinol oxidase (complex IV), a member of the heme-copper oxidase superfamily. Both complexes are part of the electron transport chain/aerobic respiratory chain of the host cell and responsible for the generation and the transport of energy. To our knowledge, the downregulation of these two complexes has not yet been previously described during phage infection. What causes this response is unclear, but we want to point out once more that just 83 out of the 369 ORFs of phage ɸKZ have a functional assignment, hence may contain functional homologues of the downregulated complexes IV and V that detour the native function.
Yet another interesting, downregulated gene cluster () includes PA2732, which may encode a type I restriction enzyme (by homology). This downregulation could be associated with the protection of the viral DNA during infection. By reducing transcription of a type I restriction enzyme in the cytoplasm, the degradation of the viral DNA could be limited, further transforming the host cell towards an optimized phage reproduction system. Given that strain PAO1 lacks a CRISPR/Cas system, this downregulation of restriction-modification systems seems plausible.
Phage infection results in upregulation of antitoxin, iron storage and biofilm-associated genes
In contrast to downregulation, we considered genes with a log2 fold change above +2 as significantly upregulated (Supplementary Table 7). Prominently upregulated transcripts in phage-infected cells included the arginine, leucine, glutathione and phenylalanine biosynthetic pathways, verifying the intervention of ɸKZ through rapid redirection of the host metabolism (). This mirrors a previous study, in which ɸKZ was shown to actively modulate the host metabolism by several virus-encoded AMGs to produce substrates for de novo pyrimidine synthesis and leading to an increase of amino sugars and nucleotides during infection [Citation41,Citation70].
Table 3. List of upregulated PAO1 transcripts during phage infection
The P. aeruginosa antitoxin ParD (PA0125) was enriched by a log2 fold change of 2.25. Together with toxin ParE (log2 fold change of 1.55), it forms a strong heterotetrameric complex (type II toxin-antitoxin system), inhibiting the interaction of the toxin with the DNA gyrase [Citation71,Citation72]. The enrichment of ParD transcripts during infection suggests a mechanism to protect the viral DNA from degradation by intracellular ParE toxins. This observation is also consistent with the downregulation of a hypothetical type I restriction enzyme PA2732. The protection of the viral genomic information at the start of the infection cycle appears plausible based on the accessibility and vulnerability of phage DNA to enzymes present in the cytoplasm at that stage of infection.
Furthermore, the heme-binding bacterioferritin bfrB (PA3531) transcript was more abundant during infection. This bacterioferritin is part of the iron supply machinery of the cell and contains two subunit types, which is unusual for a bacterial ferritin. Iron ions are an essential cofactor for many enzymes contributing to synthesis of DNA (ribonucleotide diphosphate reductase) and the generation of ATP (cytochromes). Both processes are of relevance for the phage reproduction because the production of a high number of new viral particles costs energy and each new progeny particle needs to be equipped with the complete viral genomic material [Citation73] . Furthermore, iron is one of the nutrients shown to be incorporated by phages. Model E. coli phage T4 harbours seven iron ions within the receptor-binding tip of each of its tail fibres [Citation74] and the apex domain of the central tail spike protein of E. coli phages P2 and ɸ92 contains one iron ion [Citation75]. This incorporation is suggested to stabilize the tip structures and to be widely utilized by diverse phages [Citation75,Citation76]. By upregulation of the iron-storage protein BfrB, the phage may ensure a permanent availability of this necessary cofactor for enzymes and an important structural component, transforming the host cell in an improved phage-producing environment.
CdrA, a putative adhesin and component of a cyclic diguanylate-regulated two partner secretion system, was the most upregulated transcript with a log2 fold change of 4.11 during ɸKZ infection (). Together with its transporter CdrB, this system is required for biofilm formation, as it induces auto-aggregation in liquid culture [Citation65]. Within context of phage infection, expression of an aggregation factor could enhance the infection rate of the phage progeny after cell lysis, based on physical proximity of potential host cells to the source of infection. Interestingly, the TSS of this operon could only be detected in infected PAO1 cells. This hints at the fact that this could be a specific, phage-driven induction of bacterial genes. These known gene functions notwithstanding, 48% of strongly enriched transcripts were uncharacterized proteins (Supplementary Table 7). Again, this shows that further investigations concerning the function of hypothetical proteins, even in model strains like P. aeruginosa PAO1, are urgently needed to elucidate their role and impact in other contexts than phage infection.
Conclusion and perspectives
Here, we applied dRNA-seq for the first time to a phage infection model, with the aim to annotate the ɸKZ TSS landscape and simultaneously analyse the host response early in infection. Our analysis identified 65 viral TSSs and 423 bacterial TSSs, as well as associated transcripts unique to infection. We demonstrated that dRNA-seq is sufficiently sensitive to observe the distinction between different infection stages, since two different promoter motifs were found that drive early and middle viral gene expression, respectively. Furthermore, we annotated several regulatory elements such as 5´UTRs, terminators and sORFs. These novel sORFs are relevant for further investigation and illustrate the wealth of data generated by this approach.
The impact of phage infection on transcription of the host provides a clear picture on the up- and downregulation of key pathways and genes. In a previous study [Citation52], no common host response could be distinguished for ɸKZ-infected PAO1 cells and as such, the differentially expressed genes detected in our study are distinct and therefore likely constitute a phage-mediated response. For example, upon infection upregulation of the host ParD antitoxin and downregulation of a type-I restriction enzyme (PA0125) suggests protection of the viral genome against degradation. Following a similar logic towards an efficient phage infection cycle, the upregulation of the aggregation factors CdrA and CdrB might facilitate further infections by the viral progeny. In this regard, the observed downregulation of transcripts involved in the oxidative phosphorylation were unexpected. While the significance of some of these observed transcriptional changes is unclear at the moment, further studies will be important to elucidate the interactions between the two organisms. Simultaneously, such analyses will lay the foundation for the functional annotation of bacterial ORFans (genes with no homology in databases) and expand our knowledge of protein functions in this model bacterium. Furthermore, it has become increasingly clear in recent studies that sRNA-mediated regulation occurs within both lysogenic (reviewed in Altuvia et al., 2018) and lytic phages [Citation45,Citation69,Citation77], although their function in the latter is less obvious. Here, ANNOgesic analysis predicted one 75 nt sRNA for ɸKZ, but a transcript about the exact length could not be detected by northern blot analysis. We conclude that if ɸKZ encodes its own suite of sRNAs, these would only be expressed in the later phase of infection as described for other phage-encoded sRNAs. The functional characterization of phage-encoded sRNAs and their interaction partners will need further investigations and may add a new layer of regulation to phage biology.
In the promoter analysis, the AlgU (σ22) motif was detected frequently in sequences associated with infection-specific TSSs. While this observation cannot independently indicate whether this is due to a stress reaction of the host cell or a direct target motif of the phage, it offers an interesting starting point for further investigations. Moreover, the here introduced global bacterial TSS data set may serve as a resource for the development of new models for bacterial promoter prediction. Such models could enable a bacteria-specific and fine-tuned search for promoters that are overlooked by traditional motif search tools.
We show in a one-step analysis of dRNA-seq data the feasibility of simultaneously (re-)annotation and analysis of viral and bacterial genomes and transcriptomes. Furthermore, it provides a pipeline for further studies on diverse uncharacterized bacteriophages and shed light on their complex interaction with the host. However, what will be even more important is the application of this approach to a variety of phages and their hosts, leading to global comparable data sets that ground further experiments to functionally explore interesting proteins and RNA transcripts. A good example is shown by the target prediction of sRNAs in bacteria: a more precise prediction is provided when homologous sRNA sequences from different distinct organisms can be analysed. To date, sRNAs are predicted for just a small number of virulent phages [Citation45,Citation69,Citation77], limiting the prediction of interaction partners via more precise tools. In addition, the dRNA-seq data will be an important requisite for RNA-protein interactome studies by techniques such as Grad-seq [Citation78], which require high-resolution transcriptomes.
Furthermore, conservation in target pathways triggered by different phages can be highlighted and provide insights in the arm-race between bacteria and phages. Therefore, TSS mapping by dRNA-seq is the first step that leads to complex data sets of diverse phages, revealing interesting homologous proteins and RNA transcripts, enabling their functional elucidation. Since many phage proteins are conserved even across ecosystems, this knowledge may be transferable to these related phages and their hosts in a pan-phage map of regulatory networks.
Data availability
The RNA data are available under the GEO accession GSE153067.
Disclosure of Interest
The authors report no conflict of interest.
Supplemental Material
Download Zip (519 KB)Disclosure statement
No potential conflict of interest was reported by the authors.
Supplemental material
Supplemental data for this article can be accessed here.
Additional information
Funding
References
- Ofir G, Sorek R. Contemporary phage biology: from classic models to new insights. Cell. 2018;172:1260–1270.
- Salmond GPC, Fineran PC. A century of the phage: past, present and future. Nat Rev. 2015;13:777–786.
- Truncaite L, Piešiniene L, Kolesinskiene G, et al. Twelve new MotA-dependent middle promoters of bacteriophage T4: consensus sequence revised. J Mol Biol. 2003;327:335–346.
- Nodwell JR, Greenblatt J. The nut site of bacteriophage λ is made of RNA and is bound by transcription antitermination factors on the surface of RNA polymerase. Genes Dev. 1991;5:2141–2151.
- Sen HY, Pfarr D, Strickler J, et al. Characterization of the transcription activator protein C1 of bacteriophage P22. J Biol Chem. 1992;267:14388–14397.
- Mesyanzhinov VV, Robben J, Grymonprez B, et al. The genome of bacteriophage φKZ of Pseudomonas aeruginosa. J Mol Biol. 2002;317:1–19.
- Ceyssens P-J, Minakhin L, Van den Bossche A, et al. Development of giant bacteriophage KZ is independent of the host transcription apparatus. J Virol. 2014;88:10501–10510.
- Chaikeeratisak V, Nguyen K, Egan ME, et al. The phage nucleus and tubulin spindle are conserved among large pseudomonas phages. Cell Rep. 2017;20:1563–1571.
- Yakunina M, Artamonova T, Borukhov S, et al. A non-canonical multisubunit RNA polymerase encoded by a giant bacteriophage. Nucleic Acids Res. 2015;43:10411–10420.
- Orekhova M, Koreshova A, Artamonova T, et al. The study of the phiKZ phage non-canonical non-virion RNA polymerase. Biochem Biophys Res Commun. 2019;511:759–764.
- Sharma CM, Hoffmann S, Darfeuille F, et al. The primary transcriptome of the major human pathogen helicobacter pylori. Nature. 2010;464:250–255.
- Sharma CM, Vogel J. Differential RNA-seq: the approach behind and the biological insight gained. Curr Opin Microbiol. 2014;19:97–105.
- Bischler T, Tan HS, Nieselt K, et al. Differential RNA-seq (dRNA-seq) for annotation of transcriptional start sites and small RNAs in helicobacter pylori. Methods. 2015;86:89–101.
- Yu S-H, Vogel J, Förstner KU. ANNOgesic: A Swiss army knife for the RNA-Seq based annotation of bacterial/archaeal genomes. Gigascience. 2018;7:1–11.
- Kropinski AM, Clokie MRJ, Lavigne R. Bacteriophages. 2018.
- Schulz S, Eckweiler D, Bielecka A, et al. Elucidation of sigma factor-associated networks in Pseudomonas aeruginosa reveals a modular architecture with limited and function-specific crosstalk. PLoS Pathog. 2015;11(3):1–21.
- Winsor GL, Griffiths EJ, Lo R, et al. Enhanced annotations and features for comparing thousands of Pseudomonasgenomes in the Pseudomonas genome database. Nucleic Acids Res. 2016;44:D646–53.
- Potvin E, Sanschagrin F, Levesque RC. Sigma factors in Pseudomonas aeruginosa. FEMS Microbiol Rev. 2008;32:38–55.
- Stover CK, Pham XQ, Erwin AL, et al. Complete genome sequence of Pseudomonas aeruginosa PAO1, an opportunistic pathogen. Nature. 2000;406:959–964.
- Galán-Vásquez E, Luna B, Martínez-Antonio A. The regulatory network of Pseudomonas aeruginosa. Microb Inform Exp. 2011;1:3.
- Tata M, Wolfinger MT, Amman F, et al. RNAseq based transcriptional profiling of Pseudomonas aeruginosa PA14 after short and long-term anoxic cultivation in synthetic cystic fibrosis sputum medium. PLoS One. 2016;11:1–18.
- Chan KG, Priya K, Chang CY, et al. Transcriptome analysis of Pseudomonas aeruginosa PAO1 grown at both body and elevated temperatures. PeerJ. 2016;2016:1–19.
- Rossi E, Falcone M, Molin S, et al. High-resolution in situ transcriptomics of Pseudomonas aeruginosa unveils genotype independent patho-phenotypes in cystic fibrosis lungs. Nat Commun. 2018;9:1–13.
- Kordes A, Preusse M, Willger SD, et al. Genetically diverse Pseudomonas aeruginosa populations display similar transcriptomic profiles in a cystic fibrosis explanted lung. Nat Commun. 2019;10:3397.
- Wurtzel O, Yoder-Himes DR, Han K, et al. The single-nucleotide resolution transcriptome of pseudomonas aeruginosa grown in body temperature. PLoS Pathog. 2012;8(9):e1002945.
- Gill EE, Chan LS, Winsor GL, et al. High-throughput detection of RNA processing in bacteria. BMC Genomics. 2018;19:223.
- You L, Suthers PF, Yin J. Effects of Escherichia coli physiology on growth of phage T7 in vivo and in silico. J Bacteriol. 2002;184:1888–1894.
- Irie Y, Starkey M, Edwards AN, et al. Pseudomonas aeruginosa biofilm matrix polysaccharide Psl is regulated transcriptionally by RpoS and post-transcriptionally by RsmA. Mol Microbiol. 2010;78:158–172.
- Atichartpongkul S, Fuangthong M, Vattanaviboon P, et al. Analyses of the regulatory mechanism and physiological roles of Pseudomonas aeruginosa OhrR, a transcription regulator and a sensor of organic hydroperoxides. J Bacteriol. 2010;192:2093–2101.
- Starr LM, Fruci M, Poole K. Pentachlorophenol induction of the pseudomonas aeruginosa mexAB-oprM efflux operon: involvement of repressors NalC and MexR and the antirepressor ArmR. PLoS One. 2012;7:1–9.
- Mendoza-Vargas A, Olvera L, Olvera M, et al. Genome-wide identification of transcription start sites, promoters and transcription factor binding sites in E coli. PLoS One. 2009;4:1–19.
- Shell SS, Wang J, Lapierre P, et al. Leaderless transcripts and small proteins are common features of the mycobacterial translational landscape. PLoS Genet. 2015;11:1–31.
- Zhang Y-F, Han K, Chandler CE, et al. Probing the sRNA regulatory landscape of P. aeruginosa: post-transcriptional control of determinants of pathogenicity and antibiotic susceptibility. Mol MicrobiolMicrobiol. 2017;30:499–501.
- Chihara K, Bischler T, Barquist L, et al. Conditional Hfq association with small noncoding RNAs in Pseudomonas aeruginosa revealed through comparative UV cross-linking immunoprecipitation followed by high-throughput sequencing. mSystems. 2019;4:1–15.
- Pita T, Feliciano JR, Leitão JH. Small noncoding regulatory RNAs from Pseudomonas aeruginosa and burkholderia cepacia complex. Int J Mol Sci. 2018;19:1–21.
- Thomason MK, Voichek M, Dar D, et al. A rhll 5´UTR-derived sRNA regulates RhlR-dependent quorum sensing in Pseudomonas aeruginosa. MBio. 2019;10:1–14.
- Sonnleitner E, Romeo A, Bläsi U. Small regulatory RNAs in Pseudomonas aeruginosa. RNA Biol. 2012;9:364–371.
- Falcone M, Ferrara S, Rossi E, et al. The small RNA ErsA of Pseudomonas aeruginosa contributes to biofilm development and motility through post-transcriptional modulation of AmrZ. Front Microbiol. 2018;9:1–12.
- Gómez-Lozano M, Marvig RL, Molin S, et al. Genome-wide identification of novel small RNAs in Pseudomonas aeruginosa. Env Microbiol. 2012;14:2006–2016.
- Livny J, Brencic A, Lory S, et al. Identification of 17 Pseudomonas aeruginosa sRNAs and prediction of sRNA-encoding genes in 10 diverse pathogens using the bioinformatic tool sRNAPredict2. Nucleic Acids Res. 2006;34:3484–3493.
- De Smet J, Zimmermann M, Kogadeeva M, et al. High coverage metabolomics analysis reveals phage-specific alterations to Pseudomonas aeruginosa physiology during infection. Isme J. 2016;10:1823–1835.
- Van den Bossche A, Hardwick SW, Ceyssens PJ, et al. Structural elucidation of a novel mechanism for the bacteriophage-based inhibition of the RNA degradosome. Elife. 2016;5:1–20.
- Wilusz JE. Controlling translation via modulation of tRNA levels. Wiley Interdiscip Rev RNA. 2015;6:453–470.
- Lavigne R, Sun WD, Volckaert G. PHIRE, a deterministic approach to reveal regulatory elements in bacteriophage genomes. Bioinformatics. 2004;20:629–635.
- Lood C, Danis‐Wlodarczyk K, Blasdel BG, et al. Integrative omics analysis of Pseudomonas aeruginosa virus PA5oct highlights the molecular complexity of jumbo phages. Environ Microbiol. 2020;22:1462–2920.14979.
- Duval M, Cossart P. Small bacterial and phagic proteins: an updated view on a rapidly moving field. Curr Opin Microbiol. 2017;39:81–88.
- Storz G, Wolf YI, Ramamurthi KS. Small proteins can no longer be ignored. Annu Rev Biochem. 2014;83:753–777.
- Ragunathan PT, Vanderpool CK. Cryptic-prophage-encoded small protein DicB protects escherichia coli from phage infection by inhibiting inner membrane receptor proteins. J Bacteriol. 2019;201:1–16.
- You J, Sun L, Yang X, et al. Regulatory protein SrpA controls phage infection and core cellular processes in Pseudomonas aeruginosa. Nat Commun. 2018;9:1–14.
- Ren ZJ, Black LW. Phage T4 SOC and HOC display of biologically active, full-length proteins on the viral capsid. Gene. 1998;215:439–444.
- Qin L, Fokine A, O’donnell E, et al. Structure of the small outer capsid protein, soc: a clamp for stabilizing capsids of T4-like phages. J Mol Biol. 2010;395:728.
- Blasdel BG, Ceyssens P-J, Chevallereau A, et al. Comparative transcriptomics reveals a conserved bacterial adaptive phage response (BAPR) to viral predation. bioRxiv. 2018;1–21.
- Hurwitz BL, Hallam SJ, Sullivan MB. Metabolic reprogramming by viruses in the sunlit and dark ocean. Genome Biol. [Internet] 2013; 14. Available from: http://genomebiology.com/2013/14/11/R123
- Breitbart M, Thompson LR, Suttle CA, et al. Exploring the vast diversity of marine viruses. Oceanography. 2007;20:135–139.
- Bailey TL, Boden M, Buske FA, et al. Tools for motif discovery and searching. Nucleic Acids Res. 2009;37:W202–W208.
- Totten PA, Cano Lara J, Lory S. The rpoN gene product of Pseudomonas aeruginosa is required for expression of diverse genes, including the flagellin gene. J Bacteriol. 1990;172:389–396.
- Dasgupta N, Wolfgang MC, Goodman AL, et al. A four-tiered transcriptional regulatory circuit controls flagellar biogenesis in Pseudomonas aeruginosa. Mol Microbiol. 2003;50:809–824.
- Starnbach MN, Lory S. The filA (rpoF) gene of Pseudomonas aeruginosa encodes an alternative sigma factor required for flagellin synthesis. Mol Microbiol. 1992;6:459–469.
- Lo YL, Chen CL, Shen L, et al. Characterization of the role of global regulator FliA in the pathophysiology of Pseudomonas aeruginosa infection. Res Microbiol. 2018;169:135–144.
- Martin DW, Schurr MJ, Yu H, et al. Analysis of promoters controlled by the putative sigma factor algu regulating conversion to mucoid in pseudomonas aeruginosa : relationship to 0 E and stress response. J Bacteriol. 1994;176:6688–6696.
- Schurr MJ, Deretic V. Microbial pathogenesis in cystic fibrosis: co-ordinate regulation of heat-shock response and conversion to mucoid in Pseudomonas aeruginosa. Mol Microbiol. 1997;24:411–420.
- Wagemans J, Blasdel BG, Van den Bossche A, et al. Functional elucidation of antibacterial phage ORFans targeting P seudomonas aeruginosa. Cell Microbiol. 2014;16:1822–1835.
- Martin DW, Holloway BW, Deretic V. Characterization of a locus determining the mucoid status of pseudomonas aeruginosa: algU shows sequence similarities with a bacillus sigma factor. J Bacteriol. 1993;175:1153–1164.
- Ha D-G, O´Toole G. c-di-GMP and its effects on biofilm formation and dispersion: a pseudomonas aeruginosa review. Microbiol Spectr. 2015;3:1–9.
- Borlee BR, Goldman AD, Murakami K, et al. Pseudomonas aeruginosa uses a cyclic-di-GMP-regulated adhesin to reinforce the biofilm extracellular matrix. Mol Microbiol. 2010;75:827–842.
- Liang H, Deng X, Ji Q, et al. The pseudomonas aeruginosa global regulator VqsR directly inhibits QscR to control quorum-sensing and virulence gene expression. J Bacteriol. 2012;194:3098–3108.
- Vakulskas CA, Brady KM, Yahr TL. Mechanism of transcriptional activation by Pseudomonas aeruginosa ExsA. J Bacteriol. 2009;191:6654–6664.
- Leskinen K, Blasdel BG, Lavigne R, et al. RNA-sequencing reveals the progression of phage-host interactions between φR1-37 and yersinia enterocolitica. Viruses. 2016;8(4):111.
- Chevallereau A, Blasdel BG, De Smet J, et al. Next-generation “-omics” approaches reveal a massive alteration of host RNA metabolism during bacteriophage infection of pseudomonas aeruginosa. PLoS Genet. 2016;12:1–20.
- Behzad H, Gojobori T, Mineta K. Challenges and opportunities of airborne metagenomics. Genome Biol Evol. 2015;7:1216–1226.
- Jiang Y, Pogliano J, Helinski DR, et al. ParE toxin encoded by the broad-host-range plasmid RK2 is an inhibitor of Escherichia coli gyrase. Mol Microbiol. 2002;44:971–979.
- Muthuramalingam M, White JC, Murphy T, et al. The toxin from a ParDE toxin-antitoxin system found in pseudomonas aeruginosa offers protection to cells challenged with anti-gyrase antibiotics. Mol Microbiol. 2019;111:441–454.
- Mahmoudabadi G, Milo R, Phillips R. Energetic cost of building a virus. Proc Natl Acad Sci U S A. 2017;114:E4324–33.
- Bartual SG, Otero JM, Garcia-Doval C, et al. Structure of the bacteriophage T4 long tail fiber receptor-binding tip. Proc Natl Acad Sci U S A. 2010;107:20287–20292.
- Browning C, Shneider MM, Bowman VD, et al. Phage pierces the host cell membrane with the iron-loaded spike. Structure. 2012;20:326–339.
- Bonnain C, Breitbart M, Buck KN. The Ferrojan horse hypothesis: iron-virus interactions in the ocean. Front Mar Sci. 2016;3:1–11.
- Altuvia S, Storz G, Papenfort K. Cross-regulation between bacteria and phages at a posttranscriptional level. Microbiol Spectr. 2018;6:1–14.
- Smirnov A, Förstner KU, Holmqvist E, et al. Grad-seq guides the discovery of ProQ as a major small RNA-binding protein. Proc Natl Acad Sci. 2016;113:11591–11596.