ABSTRACT
The COVID-19 emergency pandemic resulting from infection with SARS-CoV-2 represents a major threat to public health worldwide. There is an urgent clinical demand for easily accessible tools to address weaknesses and gaps in the management of COVID-19 patients. In this context, transcriptomic profiling of liquid biopsies, especially microRNAs (miRNAs), has recently emerged as a robust source of potential clinical indicators for medical decision-making. Nevertheless, the analysis of the circulating miRNA signature and its translation to clinical practice requires strict control of a wide array of methodological details. In this review, we indicate the main methodological aspects that should be addressed when evaluating the circulating miRNA profiles in COVID-19 patients, from preanalytical and analytical variables to the experimental design, impact of confounding, analysis of the data and interpretation of the findings, among others. Additionally, we provide practice points to ensure the rigour and reproducibility of miRNA-based biomarker investigations of this condition.
Abbreviations: ACE: angiotensin-converting enzyme; ARDS: acute respiratory distress syndrome; COVID-19: coronavirus disease 2019; ERDN: early Detection Research Network; LMWH: low molecular weight heparin; miRNA: microRNA; ncRNA: noncoding RNA; SARS-CoV-2: severe acute respiratory syndrome coronavirus-2; SOP: standard operating procedure.
Severe acute respiratory syndrome coronavirus-2 (SARS‐CoV‐2), a novel RNA virus from the Coronaviridae family, was identified at the end of 2019 in the city of Wuhan (China) from where it rapidly spread across the country and subsequently worldwide [Citation1]. Coronavirus disease 2019 (COVID-19) was declared a global pandemic by the World Health Organization on March 2020, accounting for more than 32.7 million confirmed cases worldwide and more than 991 thousand confirmed deaths in September 2020 [Citation2].
The clinical manifestations of infected patients range from asymptomatic or non-specific symptoms to severe systemic complications. Common symptoms include fever, dry cough, fatigue, dyspnoea, myalgia, and headache [Citation3,Citation4]. Although the pathogenesis of COVID-19 is still widely unknown, approximately 20% of COVID-19 patients develop a severe phenotype of respiratory illness, with an overall death rate of approximately 2–5% [Citation5,Citation6]. Complications of COVID-19 include acute respiratory distress syndrome (ARDS), respiratory failure, thrombosis, liver injury, acute myocardial injury, acute kidney injury, septic shock and even multiple organ failure [Citation7,Citation8].
COVID-19 shows high heterogeneity in the profile of risk factors, pathological causes, clinical manifestations and consequences [Citation9,Citation10]. In this scenario, there is a medical demand for reliable tools to monitor patient status [Citation11]. Novel biomarkers could enhance the proper management of those patients who are more likely to develop infection-derived complications while avoiding the unnecessary evaluation of those subjects at low risk. Accordingly, the development of prognostic models will be clinically useful for calculating individual probabilities of adverse clinical outcomes, which would ultimately impact medical decision-making by facilitating patient therapy and care. In terms of therapeutic guidance, a test to predict and monitor the response to the treatment available will be of paramount importance to target individuals most likely to respond to treatments. The potential benefits in other aspects, such as patient selection in COVID-19 clinical trials, should also be mentioned.
In the last decade, the transcriptome has emerged as a robust source of potential clinical indicators [Citation12,Citation13]. The use of blood transcriptomic profiling has been proposed for the medical management of different diseases, including cancer [Citation14], cardiovascular disease [Citation15,Citation16] and infectious diseases [Citation17]. Regarding the transcriptomic biomarkers, thousands of experimental studies have reported the noncoding transcriptome as a useful tool for a wide array of conditions. In particular, the composition and abundance of the circulating microRNA (miRNA) profile in minimally invasive liquid biopsies provide a new opportunity to measure phenotypic information. The use of circulating miRNAs in diagnosis, prognosis and therapeutic guidance has been extensively reviewed [Citation18–21].
In the short- and medium-term, a vast array of studies will address the potential role of peripheral blood miRNAs and other noncoding RNAs (ncRNAs) in the management of COVID-19. The role of these molecules in the pathological mechanisms linked to this condition will also be evaluated in the near future. However, the analysis of ncRNAs in liquid biopsies has been demonstrated to be challenging, especially in poor quality samples. Here, we raise the main technical challenges that should be addressed when evaluating the circulating miRNA profile in COVID-19 patients (). We aim to provide practical considerations to ensure rigour and reproducibility in miRNA-based studies on this condition.
Figure 1. Summary of the key methodological challenges that should be considered for peripheral blood microRNA profiling in COVID19 patients. General considerations for microRNA-based biomarker studies (blue) include experimental design, data interpretation and causality between microRNAs and outcomes; preanalytical factors (red) include sample quality and haemolysis, consistency across studies and sample collection, storage and processing; and analytical factors (green) include biosecurity in RNA isolation, data normalization and heparin and other pharmacological treatments. MiRNA: microRNA
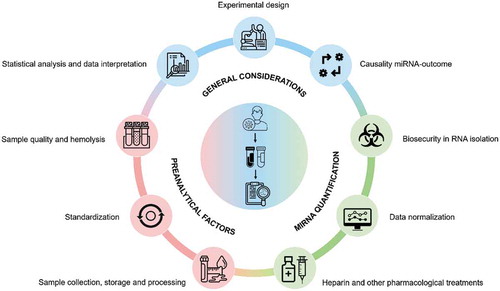
Methodological considerations, technical challenges and practice points
Critical factors, from blood collection, processing and storage to data analysis and interpretation, should be accounted for when embarking on investigations aiming to analyse the circulating miRNA profiles of COVID-19 patients:
General considerations for microRNA-based biomarker studies
There are some general considerations related to experimental design, data analysis and data interpretation that should be taken into account when evaluating the profiles of circulating miRNAs in COVID-19 patients.
A large percentage of previous miRNA studies have included a small number of subjects/patients, without specifying sample size calculations, limiting the robustness of the obtained conclusions [Citation22]. The replication of the results in independent and multicenter studies with larger and/or adequate population sizes is a key, and usually lacking, step in elucidating the real clinical applicability of circulating miRNAs. Furthermore, studies are generally based on artificial case-control approaches using selected patients and healthy controls with an inadequate consideration of comorbidities, medication use and confounding factors. This type of experimental design may be useful for exploring the molecular pathways associated with COVID-19. However, they overestimate the value of the biomarker under evaluation. The analysis of biomarker performance in a real clinical setting is a key step in obtaining convincing evidence.
The impact of potential confounders, including pharmacological treatments, as discussed below, and clinical variables, such as age, sex and comorbidities, on the miRNA profile should be considered in the experimental design (e.g. matched cases and controls in screenings) and the statistical analysis (e.g. multivariable models). Another aspect to be highlighted is the effect of disease stage and disease subtype. It has been described that SARS-CoV-2 infection is composed of different phases, i.e. early infection, pulmonary phase and hyperinflammation phase [Citation23], and is associated with a variety of deleterious consequences in different organ systems. Although this is a result itself, it may complicate the interpretation of the findings.
No single statistical method should be used alone to explore the performance of circulating miRNAs as biomarkers and to assess their incremental clinical use. In general, a considerable proportion of miRNA-based biomarker studies have analysed the univariate relation between the miRNAs and the outcome of interest. This type of approach misses valuable information. The analyses should explore the association between miRNAs and the outcome in conjunction with observable and measurable patient characteristics, including demographic, clinical and pharmacological variables. The addition of miRNAs to currently proposed algorithms or electronic health data should provide valuable information for the real clinical application of miRNAs as biomarkers. The information provided by miRNAs must not recapitulate the information available in the clinical history or that achieved by contemporaneous indicators.
The lack of specificity of individual miRNAs also justifies the use of these transcripts in conjunction with clinical variables and/or other miRNAs. For example, immunomodulatory miRNAs, such as miR-146a-5p, miR-150-5p or miR-155-5p, fluctuate in or mediate the response to diverse infections: human immunodeficiency virus, enterovirus, hepatitis C virus, and encephalitis virus, among others [Citation24–28]. An association between these miRNAs and non-infectious conditions, such as cardiovascular disease, metabolic disease and cancer, has also been described in the literature [Citation29–31]. These miRNAs may constitute biomarkers of the immune/inflammatory response and thus indicators of a specific mechanism of the disease that may complement other sources of biological and/or pathological information. Indeed, recent evidence suggests that miRNA signatures should be used as companion biomarkers to existing information [Citation32,Citation33].
It is also fundamental to compare miRNAs with already established biomarkers that are currently evaluated in clinical laboratories. For example, cardiac, inflammatory, coagulation or organ damage markers provide valuable information for the clinical management of COVID-19 patients [Citation34–37]. The analysis of other subclasses of ncRNAs or ncRNA modifications with potential in the field of biomarkers deserves consideration [Citation38,Citation39].
Disease phenotypes are a consequence of abnormalities in entire gene expression networks. This is particularly relevant in the case of miRNAs. In general, the biomarker potential of individual miRNAs is too low to justify their translation to clinical practice [Citation40]. This situation is in line with miRNA biology; the small fold change of a single miRNA in response to a physiological or pathological stressor has a modest impact on gene expression. Coordinated miRNA expression rather than the expression of individual miRNAs is critical for the regulation of complex biological mechanisms [Citation41]. The use of miRNA signatures is crucial [Citation42]. Advanced statistical tools and network analyses are fundamental to describe the interaction between miRNAs, other biomolecules and the phenotype [Citation43].
The development of clinical assays for circulating miRNAs is still in its infancy [Citation44]. The implementation of miRNA-based tests in the clinical routine has also been hampered by technical limitations and lack of rigour and reproducibility. Methodologies for miRNA detection and analysis are still evolving. Guidelines on best practices, standard operating procedures (SOPs) and/or harmonized miRNA quantification methods are crucial for increasing the consistency among results and facilitating comparability across studies. Furthermore, the addition of miRNA testing to clinical practice is currently time consuming. Miniaturization and development of automated and standardized assays should be intensified for their clinical application. Analyses on the implementation of miRNAs in terms of cost effectiveness should not be disregarded [Citation45]. In this context, initiatives such as the EU-CardioRNA COST Action CA17129 (www.cardiorna.eu), an international and interdisciplinary network aiming to accelerate the understanding of transcriptomics and further the translation of experimental data into usable applications to improve personalized medicine [Citation46,Citation47], constitute a key step.
Causal association between circulating microRNAs and the outcome of interest
Since miRNAs are master regulators of gene expression, these transcripts could provide relevant information about the pathological mechanisms that are mediated by the disease and its sequelae. The association of circulating miRNA profiles with the molecular pathways implicated in COVID-19 pathophysiology may constitute an interesting method for the development of novel therapeutic approaches. These results, although useful, should be interpreted with caution.
Whether circulating miRNAs are causative disease drivers is still unknown [Citation48,Citation49]. Circulating miRNAs are thought to participate in the exchange of epigenetic information between cells and tissues and therefore may constitute endocrine genetic signals [Citation50,Citation51]. Nevertheless, their metabolism in the circulation and their modulation of recipient cell behaviours in addition to the mechanisms that mediate miRNA production and secretion are not completely understood [Citation49].
The lack of cell- and tissue-specificity of miRNAs constitutes a limitation in identifying which organ is damaged by the disease. When miRNAs were described, it was proposed that the release of miRNAs from their cells of origin may provide tissue- and organ-specific information. Unfortunately, most miRNAs are ubiquitously abundant in solid tissues and whole blood. For example, Ludwig et al. reported that up to 82.9% are not specific to a single tissue [Citation52]. Fehlmann et al. [Citation53] proposed that only 7% of miRNAs are predominantly expressed in a single organ. The vast majority are ubiquitously expressed (26.4%) or expressed in more than 10 tissues (65.3%). Although adipose tissue and platelets seem to have a strong impact on the pool of circulating miRNAs [Citation54,Citation55], no specific organ determines the miRNA profile in the bloodstream [Citation53]. Furthermore, tissue-specific miRNAs are not necessarily found in the circulation, at least not under physiological conditions [Citation53]. Even after exposure to pathological conditions, the profile of extracellular miRNAs secreted by the cell does not necessarily correlate with the profile of intracellular miRNAs [Citation56].
Taking into account these limitations, bioinformatic analyses have emerged as a useful tool to identify the molecular pathways associated with a given set of miRNAs. There are a number of interesting databases and resources that have been used in recent years [Citation57]. Unfortunately, most bioinformatics methods depend on prior knowledge and are not based on mechanistic studies. The bias towards overrepresented mechanisms such as the cell cycle and cancer biology and the generation of large amounts of false-positive data limit the use of bioinformatic tools in identifying target mRNAs and molecular pathways [Citation57,Citation58]. The results provided by bioinformatic approaches should be experimentally validated to confirm the findings. Unfortunately, this validation step is complicated in plasma/serum samples.
Overall, the association of changes in the circulating miRNA profile with the pathological mechanism of the disease is challenging. Nevertheless, the use of miRNAs emerges as an interesting strategy to generate hypotheses on the molecular pathways linked to COVID-19.
Preanalytical conditions
A large number of biological samples, including plasma and serum, are generated during the diagnosis and monitoring of COVID-19 patients. Under adequate quality and biosecurity conditions, these samples can be used in research projects. Nevertheless, the priority for samples used in clinical laboratories obviously corresponds to clinical practice, especially during the peak of the pandemic, and thus, may not meet the conditions for miRNA quantification. Minimal preanalytical considerations are fundamental.
The preanalytical phase has been described as one of the main sources of technical variability in the evaluation of circulating miRNA profiles. According to Binderup et al. [Citation59], plasma preparation, together with miRNA purification, may account for 39–73% of the intra-assay variation. Accordingly, minimal control of the preanalytical variables and minimal quality standards should be taken into account for those samples collected in the clinical setting and recycled for research purposes, especially in readily accessible samples. Lack of consistency in these steps may introduce bias and contribute to technical variability. The use of standardized protocols for blood collection and processing, such as those proposed by the Early Detection Research Network (ERDN) [Citation60], should be considered. However, general guidelines for sample collection and handling have not been universally implemented.
Different factors from this early phase affect the quality of the generated data. The choice of the blood fraction (plasma or serum) and anticoagulant (EDTA, heparin or citrate) has a great impact on miRNA quantification. To avoid conflicting results, the same blood fraction and anticoagulant should be used consistently throughout a study. The direct comparison of results obtained from different blood fractions or anticoagulants is not recommended. The miRNA profiles of plasma and serum are essentially different, even from the same patient [Citation61]. The coagulation process alters the miRNA pattern and may also induce sample-to-sample variation [Citation61]. Samples should be collected in tubes containing an anticoagulant compatible with RT-qPCR. Heparin inhibits downstream enzymatic steps such as cDNA synthesis and PCR and therefore should be completely avoided (as discussed in detail below). Although less clear, it has been reported that citrate may interfere with RT-qPCR miRNA quantification, causing the dilution of plasma and triggering haemolysis [Citation62,Citation63]. EDTA and serum tubes are preferred and should be collected prior to heparin and citrate tubes to avoid contamination.
Notably, haemolysis deserves special attention. Haemolysis occurring during blood collection and processing affects the detection of individual miRNAs in plasma and serum samples [Citation64]. Hemolyzed samples should always be discarded from initial discovery phases but may be included in subsequent biological and technical validation phases, in case the candidates are not affected by this phenomenon. As such, specific studies aimed at evaluating the impact of haemolysis on candidates will provide relevant information about the confounding effect of haemolysis on miRNA detection [Citation64]. Haemolysis monitoring can be performed using different methods, including the estimation of ratios based on miRNAs that are affected (miR-451a) and unaffected (miR-23a-3p) by haemolysis or the measurement of plasma/serum absorbance at 414 nm [Citation65].
It is necessary to standardize, or at least to control, different preanalytical factors that in some cases are overlooked in miRNA-based biomarker studies. These factors include the storage of blood specimens until processing, sample transportation, centrifugation conditions (time, g-force, temperature, acceleration, type of rotor and number of steps) and the storage of plasma and serum samples, among others. Each of these factors influences the levels of miRNAs, and any deviation in those conditions should be taken into account in the interpretation of the data. It is important to remove cell debris and cells after blood collection within a few hours of sample collection. The time interval until sample processing can affect miRNA levels due to the presence of blood components. Therefore, fresh blood samples should be processed within 2–4 h after collection. It has been widely described that ncRNAs are stable in plasma and serum samples at room temperature for up to 24 h [Citation66,Citation67]. Nevertheless, this condition may not be accomplished for some archived samples recycled for research purposes. Storage at 4°C may induce activation of platelets and cell lysis, therefore affecting the miRNA profile [Citation68]. Alterations in the centrifugation conditions may affect the presence of the circulating miRNA profile, mainly due to platelet contamination [Citation69]. Centrifugation at a slow speed may not adequately remove cellular components. Alternatively, prolonged centrifugation protocols and/or centrifugation at a high speed may cause haemolysis.
Samples used for miRNA studies are generally collected following standard procedure, under fasting conditions and at the same time of day. This is not necessarily true for archived samples from COVID-19 patients. The effect of fasting and nonfasting conditions and the circadian variation in miRNA levels caused by the inconsistency in the blood collection time may also increase the variability in the results [Citation70]. For example, some miRNA transporters, such as lipoproteins [Citation71,Citation72], vary their concentration in circulation under fasting and nonfasting conditions, which ultimately impacts miRNA levels. Furthermore, it has been proposed that high levels of lipoproteins may negatively impact miRNA recovery during RNA isolation [Citation73].
Overall, it is crucial to consistently manage the samples to avoid or reduce technical variation. Consequently, the preanalytical phase should include a standard workflow and control. A detailed description of the methodology used for blood collection and processing should be reported in scientific publications to allow direct comparison of the results from independent investigations.
RNA isolation
We should stress that the appropriate level of risk must always be taken into account when handling samples from patients infected with SARS-CoV-2, since it is an agent with a high rate of transmissibility and a lethal capacity. Guanidine salt and phenol, components of most reagents used for RNA isolation, constitute an effective method to inactivate the virus without affecting subsequent molecular profiling. Nevertheless, alternative protocols have been proposed to inactivate the virus in biological samples, including heating up to 70°C or the use of surfactants such as Triton X-100. The impact of these treatments on RNA isolation and the detection efficiency should be rigorously evaluated in case this type of sample is used for miRNA quantification.
Similar to the preanalytical factors, miRNA purification constitutes one of the highest sources of intra-assay variance, up to 92% for some miRNAs according to McDonald et al. [Citation74]. RNA isolation is challenging due to its low abundance in the circulation, its short length, and the presence of contaminants such as immunoglobulins and other proteins in the samples. The evaluation of the concentration and quality of the isolated RNA using standard spectrophotometric methods (e.g. Nanodrop) or a Bioanalyzer is a challenge due to the low abundance of RNA in plasma and serum samples and the presence of contaminants that absorb at different wavelengths. Extraction methods need to be suitable for isolating small RNAs (smaller than 200 nucleotides). The addition of spike-in synthetic RNAs is recommended as a quality control of the isolation processes; however, the use of this tool does not inform about the technical variation introduced in the preanalytical phase.
The different isolation procedures show high variability in their efficiencies [Citation75], and the results cannot be directly transposed from one protocol to another. For miRNA isolation, both the combination of phenol/chloroform or phenol-free methods with silica columns for RNA absorption should be preferred. Previous data suggest that methods incuding RNA precipitation should be discarded. Indeed, Kim et al. [Citation76] reported the selective loss of small RNAs, including miRNAs, pre-miRNAs and other ncRNAs, with low GC content due to inefficient precipitation in samples with low RNA content in the starting material.
Heparin use
The interaction of commonly used therapeutic drugs in COVID-19 patients with miRNA quantification is one of the main analytical concerns in this type of study. This is especially relevant in the case of heparin. Heparin is an anticoagulant usually administered to COVID-19 patients during hospital stays. Prophylactic treatment with low molecular weight heparin (LMWH), in the absence of contraindications, is recommended in hospitalized patients [Citation77]. If LMWH is not available, unfractionated heparin or fondaparinux can be considered [Citation77].
Unfortunately, heparin, which cannot be removed during RNA extraction and copurifies with RNA during isolation from plasma or serum, binds to calcium and magnesium from the reaction buffers and ultimately inhibits the reverse transcriptase and polymerase enzymes used in RT-qPCR [Citation78,Citation79]. Different authors have reported that even a single heparin bolus significantly alters miRNA quantification using RT-qPCR [Citation80,Citation81]. Although the impact on endogenous miRNAs is less clear, heparin decreases the levels of the exogenous spike-in from Caenorhabditis elegans by approximately 3 cycles [Citation81], commonly used for data normalization. This effect disappears approximately 6 h after the administration of heparin and is closely associated with the half-life of heparin in the bloodstream.
Sample treatment with the heparinase enzyme prior to miRNA quantification reverses the effect of heparin, becoming a crucial step in overcoming its confounding power [Citation82]. Therefore, samples from hospitalized COVID-19 patients must be treated with heparinase. The use of heparinase protocols in all samples, including patients not treated with heparin, is recommended to improve the consistency across subjects from different experimental groups. Several protocols for this purpose have been described in the literature [Citation83,Citation84].
Notably, treating the samples with heparinase increases the number of steps for miRNA quantification and therefore increases sample-to-sample variation.
Other pharmacological treatments
It is essential to take into account the effect of other drugs administered to COVID-19 patients on the circulating miRNA profile. For example, anticoagulation therapy has been proposed as a first-line therapy in COVID-19 patients [Citation85]. However, it has been widely described that antiplatelet therapy, such as aspirin or clopidogrel, alters the levels of circulating miRNAs, particularly the levels of platelet-enriched miRNAs such as miR-126-3p, miR-191-5p or miR-223-3p, among others [Citation86,Citation87]. Therefore, special attention should be given with respect to the medications widely used in hospitalized COVID-19 patients, such as tocilizumab or remdesivir.
The common profile of hospitalized COVID-19 patients involves a clinical history of different diseases and a pharmacological history. The impact of other commonly used medications, such as statins, angiotensin-converting enzyme (ACE) inhibitors or beta blockers, on the circulating miRNA signature has also been described in independent studies [Citation88–90].
Normalization
Normalization constitutes one of the hot topics in the field of circulating miRNA quantification. The appropriate normalization strategy has been extensively reviewed in different publications [Citation22,Citation91–94]. There is no unique approach that is universally accepted by the different laboratories working in the field. Among the proposed approaches, the ones that stand out are the use of synthetic or biological spike-ins (e.g. cel-miR-39-3p), specific endogenous controls, the geometric mean of miRNA signatures and the combination of different strategies.
Some points deserve careful consideration in the context of the COVID-19 patient. As discussed above, the effect of heparin is especially relevant in the quantification of cel-miR-39-3p [Citation81]. In the case of low-quality samples, the use of blood-cell miRNAs as endogenous references (i.e. miR-16-5p or miR-451a) should be completely avoided. Furthermore, the heterogeneity of the patients and the disease may impact the selection of the appropriate control. One or several endogenous controls may function in a specific subphenotype of patients or a given disease. The COVID-19 patient shows a highly variable medical and pharmacological history, clinical presentation and end-organ damage. As such, the identification of one endogenous miRNA, or a small set or miRNAs, for normalization may be challenging. Appropriate preliminary investigations should be performed to validate the use of potential candidates. Several algorithms, such as NormFinder [Citation95] and geNorm [Citation96], have been used to explore the most suitable endogenous controls.
Conclusions
In the last decade, the literature has consistently proposed circulating miRNAs as useful biomarkers for the clinical management of different conditions. Despite such promising results, there are major concerns about the translation of these small transcripts to the clinic. MiRNA quantification is labour intensive and highly dependent on specimen collection, storage, handling and data analysis, which may lead to inconsistent or even contradictory results. The reproducibility among the results of independent research groups is generally modest [Citation97]. This situation, together with the low quality of the samples collected during the worst phases of the pandemic, makes prospective and retrospective miRNA studies in COVID-19 patients a challenge. Several points must be taken into account to report high-quality results. Here, we have discussed the general methodological and technical considerations and the most relevant preanalytical and analytical issues related to circulating miRNA quantification in samples from the COVID-19 patient.
In conclusion, circulating miRNAs are part of the tools at our disposal in the management of COVID-19 patients. Analyses of the circulating miRNA profile require the consideration of challenging methodological details to ensure a minimal quality of the samples when designing and performing studies focused on the quantification of these small ncRNAs. Protocols implemented for miRNA quantification should be consistent in both the preanalytical and analytical phases. The inclusion of detailed methodological information when describing protocols in publications will facilitate the comparison of the findings between independent studies.
Disclosure of interest
FB and DdGC have filed patents on microRNAs as biomarkers. Other authors report no conflict of interest.
Acknowledgments
LP acknowledges receiving financial support from the Ministry of Science, Innovation and Universities for the Training of University Lecturers (FPU19 / 03526). DdGC acknowledges receiving financial support from Instituto de Salud Carlos III (ISCIII), Miguel Servet 2020 (CP20/00041), cofunded by the European Social Fund (ESF), “Investing in your future.
Additional information
Funding
References
- Zhu N, Zhang D, Wang W, et al. A novel coronavirus from patients with pneumonia in China, 2019. N Engl J Med. 2020;382(8):727–733.
- World Health Organization. Coronavirus Disease (COVID-19). 2020; cited 2020 Sept 27. Available from: https://www.who.int/emergencies/diseases/novel-coronavirus-2019
- Shi Y, Wang G, Cai XP, et al. An overview of COVID-19. J Zhejiang Univ Sci B. 2020;21(5):343–360.
- Pascarella G, Strumia A, Piliego C, et al. COVID-19 diagnosis and management: a comprehensive review. J Intern Med. 2020;288(2):192–206.
- Khalili M, Karamouzian M, Nasiri N, et al. Epidemiological characteristics of COVID-19: a systematic review and meta-analysis. Epidemiol Infect. 2020;148:e130
- Li LQ, Huang T, Wang YQ, et al. COVID-19 patients’ clinical characteristics, discharge rate, and fatality rate of meta-analysis. J Med Virol. 2020;92(6):577–583.
- Huang C, Wang Y, Li X, et al. Clinical features of patients infected with 2019 novel coronavirus in Wuhan, China. Lancet. 2020;395(10223):497–506.
- Ackermann M, Verleden SE, Kuehnel M, et al. Pulmonary vascular endothelialitis, thrombosis, and angiogenesis in Covid-19. N Engl J Med. 2020;383(2):120–128.
- Docherty AB, Harrison EM, Green CA, et al. Features of 20 133 UK patients in hospital with covid-19 using the ISARIC WHO clinical characterisation protocol: prospective observational cohort study. BMJ. 2020;369:m1985
- Laing AG, Lorenc A, Del Molino Del Barrio I, et al. A dynamic COVID-19 immune signature includes associations with poor prognosis. Nat Med. 2020;26(10):1623–1635.
- Shen B, Yi X, Sun Y, et al. Proteomic and metabolomic characterization of COVID-19 patient sera. Cell. 2020;182(1):59–72.e15.
- Devaux Y. Transcriptome of blood cells as a reservoir of cardiovascular biomarkers. Biochim Biophys Acta Mol Cell Res. 2017;1864(1):209–216.
- Qian L, Li Q, Baryeh K, et al. Biosensors for early diagnosis of pancreatic cancer: a review. Transl Res. 2019;213:67–89.
- Best MG, Sol N, Kooi I, et al. RNA-seq of tumor-educated platelets enables blood-based pan-cancer, multiclass, and molecular pathway cancer diagnostics. Cancer Cell. 2015;28(5):666–676.
- Wingrove JA, Daniels SE, Sehnert AJ, et al. Correlation of peripheral-blood gene expression with the extent of coronary artery stenosis. Circ Cardiovasc Genet. 2008;1(1):31–38.
- Vargas J, Lima JAC, Kraus WE, et al. Use of the corus® CAD gene expression test for assessment of obstructive coronary artery disease likelihood in symptomatic non-diabetic patients. PLoS Curr. 2013;5
- Zak DE, Penn-Nicholson A, Scriba TJ, et al. A blood RNA signature for tuberculosis disease risk: a prospective cohort study. Lancet. 2016;387(10035):2312–2322
- De Gonzalo-Calvo D, Vea A, Bär C, et al. Circulating non-coding RNAs in biomarker-guided cardiovascular therapy: a novel tool for personalized medicine? Eur Heart J. 2019;40(20):1643–1650.
- van den Berg MMJ, Krauskopf J, Ramaekers JG, et al. Circulating microRNAs as potential biomarkers for psychiatric and neurodegenerative disorders. Prog Neurobiol. 2020;185:101732.
- Guay C, Regazzi R. Circulating microRNAs as novel biomarkers for diabetes mellitus. Nat Rev Endocrinol. 2013;9(9):513–521.
- Valihrach L, Androvic P, Kubista M. Circulating miRNA analysis for cancer diagnostics and therapy. Mol Aspects Med. 2020;72:100825.
- De Ronde MWJ, Ruijter JM, Moerland PD, et al. Study design and qPCR data analysis guidelines for reliable circulating miRNA biomarker experiments: a review. Clin Chem. 2018;64(9):1308–1318.
- Siddiqi HK, Mehra MR. COVID-19 illness in native and immunosuppressed states: a clinical–therapeutic staging proposal. J Heart Lung Transplant. 2020;39(5):405–407.
- Quaranta MT, Olivetta E, Sanchez M, et al. MiR-146a controls CXCR4 expression in a pathway that involves PLZF and can be used to inhibit HIV-1 infection of CD4+ T lymphocytes. Virology. 2015;478:27–38.
- Huang J, Wang F, Argyris E, et al. Cellular microRNAs contribute to HIV-1 latency in resting primary CD4 + T lymphocytes. Nat Med. 2007;13(10):1241–1247.
- Thounaojam MC, Kundu K, Kaushik DK, et al. MicroRNA 155 regulates japanese encephalitis virus-induced inflammatory response by targeting src homology 2-containing inositol phosphatase 1. J Virol. 2014;88(9):4798–4810.
- Ho BC, Yu IS, Lu LF, et al. Inhibition of miR-146a prevents enterovirus-induced death by restoring the production of type i interferon. Nat Commun. 2014;5(1):3344.
- Bandiera S, Pernot S, El Saghire H, et al. Hepatitis C virus-induced upregulation of microRNA miR-146a-5p in hepatocytes promotes viral infection and deregulates metabolic pathways associated with liver disease pathogenesis. J Virol. 2016;90(14):6387–6400.
- Nazari-Jahantigh M, Wei Y, Noels H, et al. MicroRNA-155 promotes atherosclerosis by repressing Bcl6 in macrophages. J Clin Invest. 2012;122(11):4190–4202.
- Bhatt K, Lanting LL, Jia Y, et al. Anti-inflammatory role of microrna-146a in the pathogenesis of diabetic nephropathy. J Am Soc Nephrol. 2016;27(8):2277–2288.
- Musilova K, Devan J, Cerna K, et al. miR-150 downregulation contributes to the high-grade transformation of follicular lymphoma by upregulating FOXP1 levels. Blood. 2018;132(22):2389–2400.
- Schulte C, Barwari T, Joshi A, et al. Comparative analysis of circulating noncoding rnas versus protein biomarkers in the detection of myocardial injury. Circ Res. 2019;125(3):328–340.
- Wong LL, Zou R, Zhou L, et al. Combining circulating microRNA and NT-proBNP to detect and categorize heart failure subtypes. J Am Coll Cardiol. 2019;73(11):1300–1313.
- Grasselli G, Tonetti T, Protti A, et al. Pathophysiology of COVID-19-associated acute respiratory distress syndrome: a multicentre prospective observational study. Lancet Respir Med. 2020;19(20):1–8.
- Cao J, Zheng Y, Luo Z, et al. Myocardial injury and COVID-19: serum hs-cTnI level in risk stratification and the prediction of 30-day fatality in COVID-19 patients with no prior cardiovascular disease. Theranostics. 2020;10(21):9663–9673.
- Elshazli RM, Toraih EA, Elgaml A, et al. Diagnostic and prognostic value of hematological and immunological markers in COVID-19 infection: a meta-analysis of 6320 patients. PLoS One. 2020;15(8):e0238160.
- Han Y, Zhang H, Mu S, et al. Lactate dehydrogenase, an independent risk factor of severe COVID-19 patients: a retrospective and observational study. Aging (Albany NY). 2020;12(12):11245–11258.
- Konno M, Koseki J, Asai A, et al. Distinct methylation levels of mature microRNAs in gastrointestinal cancers. Nat Commun. 2019;10(1):3888
- Tosar JP, Cayota A. Extracellular tRNAs and tRNA-derived fragments. RNA Biol. 2020;17(8):1149–1167.
- de Gonzalo-calvo D, Vilades D, Martínez-Camblor P, et al. Circulating microRNAs in suspected stable coronary artery disease: a coronary computed tomography angiography study. J Intern Med. 2019;286(3):341–355.
- Ebert MS, Sharp PA. Roles for microRNAs in conferring robustness to biological processes. Cell. 2012;149(3):515–524.
- Belmonte T, Mangas A, Calderon-Dominguez M, et al. Peripheral microRNA panels to guide the diagnosis of familial cardiomyopathy. Transl Res. 2020;218:1–15.
- de Gonzalo-calvo D, Martínez-Camblor P, Bär C, et al. Improved cardiovascular risk prediction in patients with end-stage renal disease on hemodialysis using machine learning modeling and circulating microribonucleic acids. Theranostics. 2020;10(19):8665–8676.
- Bonneau E, Neveu B, Kostantin E, et al. How close are miRNAs from clinical practice? A perspective on the diagnostic and therapeutic market. Electron J Int Fed Clin Chem Lab Med. 2019;30(2):114–127.
- Walter E, Dellago H, Grillari J, et al. Cost-utility analysis of fracture risk assessment using microRNAs compared with standard tools and no monitoring in the Austrian female population. Bone. 2018;108:44–54.
- Robinson EL, da Costa Gomes CP, Potočnjak I, et al. A year in the life of the EU-CardioRNA COST action: CA17129 catalysing transcriptomics research in cardiovascular disease. Noncoding RNA. 2020;6(2):17.
- da Costa Gomes CP, Ágg B, Andova A, et al. Catalyzing transcriptomics research in cardiovascular disease: the CardioRNA COST action CA17129. Noncoding RNA. 2019;5(2):31
- Williams Z, Ben-Dov IZ, Elias R, et al. Comprehensive profiling of circulating microRNA via small RNA sequencing of cDNA libraries reveals biomarker potential and limitations. Proc Natl Acad Sci U S A. 2013;110(11):4255–4260.
- Bär C, Thum T, De Gonzalo-Calvo D. Circulating miRNAs as mediators in cell-to-cell communication. Epigenomics. 2019;11(2):111–113.
- Zhang K, Dong C, Chen M, et al. Extracellular vesicle-mediated delivery of miR-101 inhibits lung metastasis in osteosarcoma. Theranostics. 2020;10(1):411–425.
- Cheng M, Yang J, Zhao X, et al. Circulating myocardial microRNAs from infarcted hearts are carried in exosomes and mobilise bone marrow progenitor cells. Nat Commun. 2019;10(1):959
- Ludwig N, Leidinger P, Becker K, et al. Distribution of miRNA expression across human tissues. Nucleic Acids Res. 2016;44(8):3865–3877.
- Fehlmann T, Ludwig N, Backes C, et al. Distribution of microRNA biomarker candidates in solid tissues and body fluids. RNA Biol. 2016;13(11):1084–1088.
- Kaudewitz D, Skroblin P, Bender LH, et al. Association of microRNAs and YRNAs with platelet function. Circ Res. 2016;118(3):420–432.
- Thomou T, Mori MA, Dreyfuss JM, et al. Adipose-derived circulating miRNAs regulate gene expression in other tissues. Nature. 2017;542(7642):450–455.
- de Gonzalo-calvo D, Cenarro A, Garlaschelli K, et al. Translating the microRNA signature of microvesicles derived from human coronary artery smooth muscle cells in patients with familial hypercholesterolemia and coronary artery disease. J Mol Cell Cardiol. 2017;106:55–67.
- Akhtar MM, Micolucci L, Islam MS, et al. Bioinformatic tools for microRNA dissection. Nucleic Acids Res. 2016;44(1):24–44.
- Godard P, Van Eyll J. Pathway analysis from lists of microRNAs: common pitfalls and alternative strategy. Nucleic Acids Res. 2015;43(7):3490–3497.
- Binderup HG, Madsen JS, Helweg Heegaard NH, et al. Quantification of microRNA levels in plasma – impact of preanalytical and analytical conditions. PLoS One. 2018;13(7):e0201069.
- Tuck MK, Chan DW, Chia D, et al. Standard operating procedures for serum and plasma collection: early detection research network consensus statement standard operating procedure integration working group. J Proteome Res. 2009;8(1):113–117.
- Wang K, Yuan Y, Cho JH, et al. Comparing the MicroRNA spectrum between serum and plasma. PLoS One. 2012;7(7):e41561. .
- Cui W, Ma J, Wang Y, et al. Plasma miRNA as biomarkers for assessment of total-body radiation exposure dosimetry. PLoS One. 2011;6(8):e22988.
- Moldovan L, Batte K, Wang Y, et al. Analyzing the circulating microRNAs in exosomes/extracellular vesicles from serum or plasma by qRT-PCR. Methods Mol Biol. 2013;1024:129–145.
- Pritchard CC, Kroh E, Wood B, et al. Blood cell origin of circulating microRNAs: a cautionary note for cancer biomarker studies. Cancer Prev Res. 2012;5(3):492–497.
- Blondal T, Jensby Nielsen S, Baker A, et al. Assessing sample and miRNA profile quality in serum and plasma or other biofluids. Methods. 2013;59(1):S1–S6.
- Mitchell PS, Parkin RK, Kroh EM, et al. Circulating microRNAs as stable blood-based markers for cancer detection. Proc Natl Acad Sci U S A. 2008;105(30):10513–10518.
- Vilades D, Martínez-Camblor P, Ferrero-Gregori A, et al. Plasma circular RNA hsa_circ_0001445 and coronary artery disease: performance as a biomarker. Faseb J. 2020;34(3):4403–4414.
- Mukai N, Nakayama Y, Ishi S, et al. Cold storage conditions modify microRNA expressions for platelet transfusion. PLoS One. 2019;14(7):e0218797.
- Cheng HH, Yi HS, Kim Y, et al. Plasma processing conditions substantially influence circulating microRNA biomarker levels. PLoS One. 2013;8(6):e64795
- Heegaard NHH, Carlsen AL, Lilje B, et al. Diurnal variations of human circulating cell-free micro-RNA. PLoS One. 2016;11(8):e0160577.
- Vickers KC, Palmisano BT, Shoucri BM, et al. MicroRNAs are transported in plasma and delivered to recipient cells by high-density lipoproteins. Nat Cell Biol. 2011;13(4):423–435.
- Niculescu LS, Simionescu N, Sanda GM, et al. MiR-486 and miR-92a identified in circulating HDL discriminate between stable and vulnerable coronary artery disease patients. PLoS One. 2015;10(10):e0140958.
- Kroh EM, Parkin RK, Mitchell PS, et al. Analysis of circulating microRNA biomarkers in plasma and serum using quantitative reverse transcription-PCR (qRT-PCR). Methods. 2010;50(4):298–301.
- McDonald JS, Milosevic D, Reddi HV, et al. Analysis of circulating microRNA: preanalytical and analytical challenges. Clin Chem. 2011;57(6):833–840.
- Kloten V, Neumann MHD, Di Pasquale F, et al. Multicenter evaluation of circulating plasma microRNA extraction technologies for the development of clinically feasible reverse transcription quantitative PCR and next-generation sequencing analytical work flows. Clin Chem. 2019;65(9):1132–1140.
- Kim YK, Yeo J, Kim B, et al. Short structured RNAs with low GC content are selectively lost during extraction from a small number of cells. Mol Cell. 2012;46(6):893–895.
- Levi M, Thachil J, Iba T, et al. Coagulation abnormalities and thrombosis in patients with COVID-19. Lancet Haematol. 2020;7(6):e438–e440.
- Willems M, Moshage H, Nevens F, et al. Plasma collected from heparinized blood is not suitable for HCV-RNA detection by conventional RT-PCR assay. J Virol Methods. 1993;42(1):127–130.
- Al-Soud WA, Rådström P. Purification and characterization of PCR-inhibitory components in blood cells. J Clin Microbiol. 2001;39(2):485–493.
- Boeckel JN, Thomé CE, Leistner D, et al. Heparin selectively affects the quantification of micrornas in human blood samples. Clin Chem. 2013;59(7):1125–1127.
- Kaudewitz D, Lee R, Willeit P, et al. Impact of intravenous heparin on quantification of circulating microRNAs in patients with coronary artery disease. Thromb Haemost. 2013;110(3):609–615.
- Li S, Zhang F, Cui Y, et al. Modified high-throughput quantification of plasma microRNAs in heparinized patients with coronary artery disease using heparinase. Biochem Biophys Res Commun. 2017;493(1):556–561.
- Plieskatt JL, Feng Y, Rinaldi G, et al. Circumventing qPCR inhibition to amplify miRNAs in plasma. Biomark Res. 2014;2(1):13.
- Johnson ML, Navanukraw C, Grazul-Bilska AT, et al. Heparinase treatment of RNA before quantitative real-time RT-PCR. Biotechniques. 2003;35(6):1140–1144.
- Nadkarni GN, Lala A, Bagiella E, et al. Anticoagulation, mortality, bleeding and pathology among patients hospitalized with COVID-19: a single health system study. J Am Coll Cardiol. 2020 August;76:1815–1826.
- Willeit P, Zampetaki A, Dudek K, et al. Circulating MicroRNAs as novel biomarkers for platelet activation. Circ Res. 2013;112(4):595–600.
- Shi R, Ge L, Zhou X, et al. Decreased platelet miR-223 expression is associated with high on-clopidogrel platelet reactivity. Thromb Res. 2013;131(6):508–513.
- Searles CD, Weber M, Baker MB, et al. MicroRNA expression profile in CAD patients and the impact of ACEI/ARB. Cardiol Res Pract. 2011;1(1):532915
- Sodi R, Eastwood J, Caslake M, et al. Relationship between circulating microRNA-30c with total- and LDL-cholesterol, their circulatory transportation and effect of statins. Clin Chim Acta. 2017;466:13–19.
- Solayman MH, Langaee TY, Gong Y, et al. Effect of plasma microRNA on antihypertensive response to beta blockers in the Pharmacogenomic Evaluation of Antihypertensive Responses (PEAR) studies. Eur J Pharm Sci. 2019;131:93–98.
- Felekkis K, Papaneophytou C. Challenges in using circulating micro-rnas as biomarkers for cardiovascular diseases. Int J Mol Sci. 2020;21(2):561.
- Zampetaki A, Mayr M. Analytical challenges and technical limitations in assessing circulating MiRNAs. Thromb Haemost. 2012;108(4):592–598.
- Moldovan L, Batte KE, Trgovcich J, et al. Methodological challenges in utilizing miRNAs as circulating biomarkers. J Cell Mol Med. 2014;18(3):371–390.
- Fernández-Sanjurjo M, De Gonzalo-Calvo D, Fernández-Garciá B, et al. Circulating microRNA as emerging biomarkers of exercise. Exerc Sport Sci Rev. 2018;46(3):160–171.
- Andersen CL, Jensen JL, Ørntoft TF. Normalization of real-time quantitative reverse transcription-PCR data: a model-based variance estimation approach to identify genes suited for normalization, applied to bladder and colon cancer data sets. Cancer Res. 2004;64(15):5245–5250.
- Vandesompele J, De Preter K, Pattyn F, et al. Accurate normalization of real-time quantitative RT-PCR data by geometric averaging of multiple internal control genes. Genome Biol. 2002;3(7):research0034.1.
- Kok MGM, de Ronde MWJ, Moerland PD, et al. Small sample sizes in high-throughput miRNA screens: a common pitfall for the identification of miRNA biomarkers. Biomol Detect Quantif. 2018;15:1–5.