ABSTRACT
The effect of information sentiment on popularity is meaningful to understand the information content on social media. The present research examined whether emotion values of information could predict the potential of popularity in two psychoinformatic experiments. A prime task was used with popular/unpopular information as prime and high/low sentiment stimuli as targets. In Experiment 1, we observed that unpopular information survived better than popular information. In Experiment 2, the electrophysiological priming effect was observed for unpopular and popular information. According to the findings, sentiment of information on social media plays a key role in information popularity.
Introduction
Social media facilitate the creation and sharing of information via virtual communities. Recently, sentiment of information on social media attracts the attention of researchers (Salas-Zárate et al., Citation2017; Wang, Huang, Wu, & Li, Citation2015). Sentiment is an emotional evaluation of a person or a topic or an interactive communication on social media (Bollen, Pepe, & Mao, Citation2011). According to Pleasure-Arousal-Dominance (PAD) model, arousal and valence are two dimensions of sentiment to affect information transmission on social media (Berger, Citation2011; Berger & Milkman, Citation2012; Bradley & Lang, Citation2000; Choi & Toma, Citation2014; Wang, Zhou, Jin, Fang, & Lee, Citation2017). For example, Choi and Toma (Citation2014) suggested that information with positive valence drove transmission better than those with negative valence (Choi & Toma, Citation2014; Wang et al., Citation2017). Besides, other studies found that high-arousal information boosted more information transmission compared to low-arousal information (Berger, Citation2011; Berger & Milkman, Citation2012). However, the impulse to spread information on social media cannot be simply attributed to one dimension of sentiment but is driven by complex sentiment states with multiple dimensions. Since information transmission experiences temporal evolution from ‘burst’, ‘peak’ to ‘fade’ (Berger, Citation2014), information popularity involves the saliency of topics before users decide to share information in social network (Gabor & Huberman Bernardo, Citation2010; Hu, Hu, Fu, Fang, & Xu, Citation2017). Existing researches have shown that information popularity is influenced by celebrity involvement, users’ interest and so on (Lasswell, Citation1948; Reece et al., Citation2017). However, it is elusive whether and how sentiment of information plays a role in popularity.
Psychoinformatics is proposed by researchers, who use experimental approaches to investigate cognitive processes and behaviors on social media (Montag, Duke, & Markowetz, Citation2016; Yarkoni, Citation2012). This methodology gives way to the combination of psychology and computer science. Prime paradigm (Hoedemaker & Gordon, Citation2017; Meyer & Schvaneveldt, Citation1971) investigates the facilitation effect of words’ processing so the presentation of the prime activates a head start and causes faster processing of related targets compared to unrelated targets. Although patterns of priming tasks vary, the general mechanism of head start is established (McNamara, Citation2005; Neely & Keefe, Citation1989). Additionally, event-related potential (ERP) is increasingly applied to reveal the neural responses. The stimuli with high sentiment were suggested to elicit high amplitudes of positive ERP components. For example, larger P300, a stimulus-evoked positive component with a peak around 300 ms, represented higher valence of rewarding stimuli (Ma et al., Citation2011; Yeung, Holroyd, & Cohen, Citation2005) and reflected stronger affective arousal due to attention allocation. Besides, stimulus-evoked late positive potential (LPP) starting from 300 ms was related to sustained evaluative processing of emotions (Hajcak & Nieuwenhuis, Citation2006). LPP amplitudes were higher for pleasant picture than neutral pictures (Hua et al., Citation2014) and for high-arousal words (Delaney-Busch, Wilkie, & Kuperberg, Citation2016).
In this study, we examined whether emotion values (i.e., valence and arousal) of information on social media influenced popularity on social media in two psychoinformatic experiments. In Experiment 1, we conducted a prime task with popular/unpopular information as prime and high/low sentiment pictures as targets. Then, participants reported valence and arousal levels of popular/unpopular information on a nine-likert scale. We predicted that popular information had higher valence and arousal than unpopular information. We further predicted that popular information as prime would lead to faster processing of targets with high sentiment but slower processing of targets with low sentiment, and vice versa. In Experiment 2, a new group of participants completed the prime task and simultaneously recorded prime-locked ERP as well as target-locked ERP. We predicted that the prime would evoke higher attention allocation and sustain evaluative processing of related targets, resulting in higher positive amplitudes.
Experiment 1
Methods and materials
Participants
Forty undergraduates were recruited from Zhejiang Sci-tech University but one was removed due to low accuracy rate (accuracy rate = 73.14%). Thirty-nine subjects for further analysis (mean age = 21.21, age ranging from 19 to 23, 35 right-handed, 33 females) were randomly selected. Handedness was assessed (Annett, Citation1970) and informed consents were obtained from all participants with normal or corrected-to-normal vision. None had a history of neurological problems. All participants were native Chinese speakers and were paid for their participation. This study was approved by local ethical committee and conducted according to the principles in the Declaration of Helsinki.
Materials
Online information was mined from Zhejiang Sci-tech University’s public account on wechat, a Facebook-like social media in China. This public account updates a piece of information every day. Users can click on titles (limited to 60 Chinese characters) to read the information in depth. The number of viewers is automatically added when users click on the titles. We selected 365 pieces of online short titles between 20 April 2016 and 20 April 2017 and prepared a ranking list in descending order according to the number of users who clicked the short titles. Thirty-six pieces of online titles at the top or bottom of the ranking list were used as popular or unpopular information, respectively. In order to imitate the context of social media, popular/unpopular information was set on the wechat background as prime. Control prime was a piece of information only with the wechat background.
Target images in the prime task were retrieved from IAPS (International Affective Picture System). Thirty-six images were used as targets with high sentiment (HIAPS, mean arousal = 4.62, mean valence = 5.26). Another 36 images were selected as targets with low sentiment (LIAPS, mean arousal = 2.46, mean valence = 4.89). Neutral targets were white pictures (NIAPS, R = 255, G = 255, B = 255). The visual angles of prime and targets were 21.53° × 28.70° × and 21.53° × 28.70° respectively.
All stimuli had the same luminance (14 cd/m2) and root-mean-square (RMS) contrast. The experimental task was presented on a 15-in. CRT computer monitor (1366 × 768 pixels, 60 HZ) connected to a 2 GHz Pentium computer. The background of the monitor was gray (R = 128, G = 128, B = 128) and the foreground was black (R = 0, G = 0, B = 0).
Procedure
Participants quietly sat in front of the computer monitor and received instructions before the experiment. Each trial began with a fixation cross for 800 ms, followed by a prime stimulus with a duration of 3000 ms. Then a gray screen was followed for 50 ms and then we presented a target stimulus which was required to be judged as quickly and correctly as possible. The target stimulus disappeared until participants had response (see ). Two keys on the computer keyboard, ‘F’ and ‘J’, were used for responses. Handedness and responses were counterbalanced across participants. The task was implemented by using E-prime 2.0 software (Psychology Software Tool, Inc., USA). Moreover, 108 trials in total were randomly presented. Reaction time (RT) was recorded for each participant. Participants practiced before the actual experiment in order to familiarize themselves with the procedure. Lastly, participants rated the valence and arousal levels in a 9-point likert scale for each piece of popular/unpopular information. Valence was recorded by asking the participants for their happiness and arousal was calmness when they saw the information.
Data analysis
The average RTs were fed into 3 × 3 repeated measures of analysis of variance (ANOVA). We used post-hoc tests to compare the differences in RTs per the three prime levels. We also performed other post-hoc analyses to examine the mean differences in RTs per the three target levels. With regard to the likert scale results, paired-sample T-tests were used to compare the valence of popular and unpopular information or the arousal of popular and unpopular information. Mean and standard errors (S.E.) were reported in the results. The mean differences were significant at the 0.05 level (two-tailed). SPSS software was used for statistical analyses. The degree of freedom of the F-ratio was corrected according to the Huynh–Feldt method when there was a lack of sphericity (Mauchly’s test of sphericity p < 0.05). Cohen’s d was used for effect size of post-hoc analyses while ηp2 (partial eta squared) assessed the effect size of F value.
Results
We observed that valence and arousal ratings of popular information (valence: mean = 5.56, S.E. = 0.17; arousal: mean = 4.62, S.E. = 0.27) were significantly higher than unpopular information (valence: mean = 4.60, S.E. = 0.20; arousal: mean = 3.50, S.E. = 0.26) (valence: t38 = 6.62, p < 0.001, d = 0.88; arousal: t38 = 7.94, p < 0.001, d = 0.85) [)].
Figure 2. Behavioral results in Experiment 1. (a) Illustrated the mean ratings of valence and arousal for popular information/unpopular information. The ratings were assessed in a nine-likert scale. Error bars were 95% confidence interval. ‘***’ indicated p < 0.001. (b) Indicated the mean reaction time for three types of IAPS targets per prime. Abbreviation: HIAPS, IAPS targets with high sentiment; LIAPS, IAPS targets with low sentiment; NIAPS, neutral white stimuli. Error bars were 95% confidence interval. ‘*’ indicated p < 0.05. The unit for RT was milliseconds.
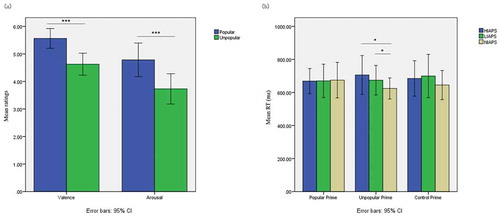
The mean accuracy rate was 98.47% (S.E. = 0.41). As seen in ), the RTs analyses for correct trials showed that there was no significant main effect of prime [F (2,76) = 0.12, p = 0.88, ηp2 = 0.003, sphericity p > 0.05] nor significant main effect of target [F (2,76) = 1.30, corrected p = 0.26, ηp2 = 0.03, sphericity p < 0.001], nor significant interaction between prime and target [F (4,152) = 2.07, corrected p = 0.11, ηp2 = 0.05, sphericity p < 0.001]. Post-hoc analyses indicated that when the prime was unpopular information, the reaction time for NIAPS (mean = 624.17, S.E. = 31.53) was significantly lower compared to those of HIAPS (mean = 706.03, S.E. = 58.22, p = 0.02) and LIAPS (mean = 674.32, S.E. = 44.23, p = 0.02). However, when the prime was popular information, there were no significant differences among HIAPS (mean = 668.72, S.E. = 37.62), LIAPS (mean = 670.11, S.E. = 49.83) and NIAPS (mean = 674.50, S.E. = 52.99, all p > 0.05).
Experiment 2
Methods and materials
Participants
A new group of participants (n = 22, mean age = 21.73, age ranging from 18 to 25, 10 females, 19 right-handed) was randomly recruited from Ningbo University. We followed the same recruitment rules as Experiment 1.
Materials
The materials were the same as Experiment 1. Since these materials were from Zhejiang Sci-tech University, participants all reported to be unfamiliar with the stimulus.
Procedure
In the experiment, the scalp of EEG with 64 Ag/AgCl electrodes was recording when participants were performing the prime task. The data recording system was the standard international 10–20 system with Neuroscan Synamp2 Amplifier (Scan 4.3.1, Neurosoft Labs, Inc., Virginia, USA). The online sampling rate was 1000 Hz with band-pass 0.05–70 Hz with left mastoid as a reference electrode during recording. Horizontal Electrooculogram (HEOG) electrodes and vertical Electrooculogram (VEOG) electrodes were placed at 10 mm from the lateral canthi of both eyes and at 10 mm above and below the left eye, respectively. The impedances of electrodes were controlled below 5 kΩ. After the ERP recording, participants assessed valence and arousal ratings for popular/unpopular information in a nine-likert scale.
EEG data analyses
The raw EEG data were preprocessed by using the SPM12 (http://www.fil.ion.ucl.ac.uk/spm/). It was downsampled into 250 Hz with removal of 50 Hz noise. ERP was digitally filtered with a high-pass 1 Hz and then with a low-pass 100 Hz (zero phase shift). Independent component analysis was used to remove the artifacts from HEOG and VEOG. Data were re-referenced into the average of all electrodes and then segmented into epochs with a time window from −100 ms (100 ms pre-stimulus onset) to 1000 ms (1000 ms post-stimulus onset). The epochs were time-locked to the onset of prime (prime-locked ERP) and onset of targets (target-locked ERP). The 100 ms pre-stimulus was used as baseline and all epochs were baseline-corrected. Then, we calculated the mean amplitudes per three types of prime-locked ERP and per 3 × 3 types of target-locked ERP. The 64 electrodes were classified into three brain areas according to the scalp distribution (see ): frontal area, central area and parietal area. The mean amplitudes per prime and per target were analyzed at each brain area across a time window from 0 ms (onset of stimuli) to 1000 ms.
Figure 3. Electrode distributions. The scalp electrodes were illustrated. Red, yellow and green indicated frontal, central and parietal areas, respectively. Left and right mean left/right brain hemispheres.
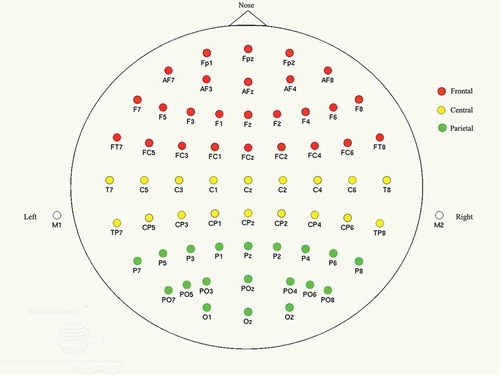
All statistical analyses were completed by using SPSS. Repeated measures of ANOVA with three primes (popular, unpopular, control) × 3 targets (HIAPS, LIAPS, NIAPS) were performed by using the target-locked ERP amplitudes per brain area. Post-hoc analyses compared target-locked ERP differences of three primes per target for each brain area. Mean and S.E. were reported. Bonferroni corrections were used to compensate for the significance level at 0.05 (two-tailed). The Huynh-Feldt correction was used when there was a lack of sphericity (Mauchly’s test of sphericity p < 0.05). ηp2 were reported to assess the effect size of statistical analysis F values. Pearson linear correlations were performed to explore the relationships between valence and prime-locked ERP amplitudes and the relationships between the arousal and prime-locked ERP amplitudes per electrode.
Results
Behavioral results
We conducted paired-sample t-tests to compare the rating scores of popular/unpopular information. The results showed that valence and arousal of popular information (valence: mean = 5.12, S.E. = 0.12; arousal: mean = 5.28, S.E. = 0.32) was significantly higher than unpopular information (valence: mean = 4.70, S.E. = 0.13; arousal: mean = 4.27, S.E. = 0.30) (valence: t21 = 2.53, p = 0.01, d = 0.53; arousal: t21 = 4.96, p < 0.001, d = 0.82).
Uncorrected trials were excluded in RT analysis and mean accuracy rate was 99.49% (S.E. = 0.16). The RT for prime had no main effect [F (2, 42) = 1.32, p = 0.27, sphericity p > 0.05, ηp2 = 0.05] nor interaction effect with target [F (4, 84) = 1.55, corrected p = 0.21, sphericity p < 0.05, ηp2 = 0.06]. The significant main effect of target was observed [F (2, 42) = 9.35, p < 0.001, sphericity p > 0.05, ηp2 = 0.30]. Post-doc analyses found that when the targets were LIAPS, unpopular prime (mean = 615.74, S.E. = 30.88) lead to shorter RTs compared to control prime (mean = 679.71, S.E. = 40.99, p = 0.01), but was not significantly different from popular prime (mean = 639.55, S.E. = 39.40, p = 0.17).
ERP results
Uncorrected trials were excluded from analyses for target-locked ERP amplitudes. As for frontal area, there was no significant main effect of prime [F (2, 42) = 1.59, corrected p = 0.21, sphericity p < 0.05, ηp2 = 0.07], nor significant interaction effect between prime and target [F (4, 84) = 1.48, p = 0.21, sphericity p > 0.05, ηp2 = 0.06]. However, we found a significant main effect of target [F (2, 42) = 5.72, p = 0.006, sphericity p > 0.05, ηp2 = 0.21]. Post-hoc analyses indicated that there was priming effect at frontal sites when the LIAPS was target: the mean target-locked ERP amplitudes after unpopular primes (mean = 0.08, S.E. = 0.30) were higher compared to that of popular primes (mean = −1.04, S.E. = 0.37, p = 0.005) and that of control primes (mean = −1.04, S.E. = 0.46, p = 0.03). As regard to central area, there was marginally significant main effect of prime [F (2, 42) = 3.00, p = 0.06, sphericity p > 0.05, ηp2 = 0.12], but no significant main effect of target [F (2, 42) = 1.09, p = 0.34, sphericity p > 0.05, ηp2 = 0.05], nor interaction effect between prime and target [F (4, 84) = 1.21, corrected p = 0.31, sphericity p < 0.05, ηp2 = 0.05]. Post-hoc analyses indicated that when LIAPS was target, unpopular prime (mean = 1.02, S.E. = 0.36) still had stronger effect on amplitudes of target-locked ERP compared to that of control prime (mean = 0.17, S.E. = 0.24, p = 0.05), but not significantly stronger compared to popular prime (mean = 0.33, S.E. = 0.22, p = 0.11). For parietal area, the results showed that there was significant main effect of target [F(2, 42) = 13.87, corrected p < 0.001, sphericity p < 0.05, ηp2 = 0.39], but no significant main effect of prime [F (2, 42) = 1.02, p = 0.36, sphericity p > 0.05, ηp2 = 0.04] nor interaction between prime and target [F (4, 84) = 0.53, corrected p = 0.68, sphericity p < 0.05, ηp2 = 0.02]. Post-hoc analyses showed that the priming effect was observed for HIAPS targets: popular prime (mean = 1.57, S.E. = 0.50) evoked higher amplitudes of target-locked ERP than that of unpopular prime (mean = 0.64, S.E. = 0.41, p = 0.03). (See –).
Figure 4. The priming effect of the target-locked ERP amplitudes at frontal, central and parietal areas within the time window 0–1000 ms. Abbreviations: HIAPS, IAPS targets with high sentiment; LIAPS, IAPS targets with low sentiment; NIAPS, neutral white stimuli. X and Y axes indicated the types of targets and 95% confidence interval of mean amplitudes, respectively. The unit of Y-axis was µV. Blue, green and yellow circles indicated the control, popular and unpopular primes, respectively. The symbol ‘*’ and ‘**’ means p < 0.05 and p < 0.01, respectively.
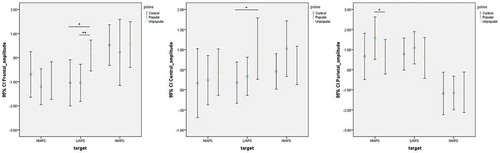
Figure 5. Grand mean target-locked ERP amplitudes across subjects. The graph was an illustration of grand mean target-locked ERP amplitudes within the time window −100–1000 ms across subjects. X-axis was the time window with the unit of milliseconds; Y axis was the grand mean amplitudes with the unit of µV. (a) Indicated the grand mean amplitudes at channel POZ when the target was IAPS with high arousal and high valence. (b) Indicated the grand mean amplitudes at channel FZ when the target was IAPS with low arousal and low valence. The blue, red and yellow lines indicated the control, unpopular and popular primes, respectively.
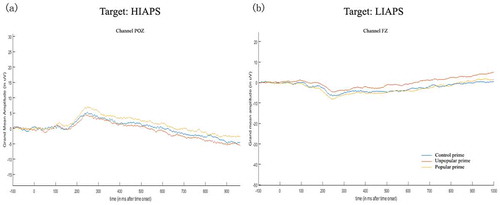
Figure 6. Topography of grand mean target-locked ERP amplitudes across subjects. It illustrated the scalp topography for target-locked ERP at 456 ms after the onset of target. The color bar was ranging from −20 to 20 µV. (a) Means when the target was IAPS with high sentiment, popular prime and unpopular prime evoked the target-locked ERP, mainly obviously different at parietal electrodes. (b) Means when the target was IAPS with low sentiment, popular/unpopular prime elicited the target-locked ERP, mainly different at frontal-central electrodes.
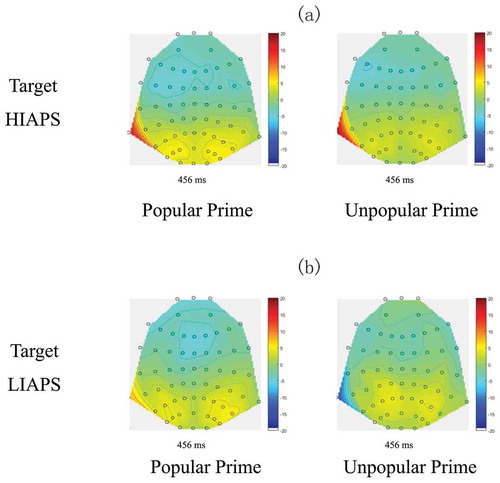
Correlations between valence ratings and prime-locked ERP amplitudes were significantly positive at some frontal electrodes. By contrast, arousal ratings and prime-locked ERP amplitudes were significantly positively correlated at right fronto-central-parietal electrodes and negatively correlated at left fronto-central-parietal electrodes (see and ).
Table 1. Correlations between self-reported ratings and prime-locked ERP amplitudes.
Figure 7. Scatter graphs for Pearson correlations between self-report ratings and prime-locked ERP amplitudes. (a) indicated the positive correlations between prime-locked amplitudes with valence ratings of popular/unpopular information at channels FP1, FPZ, FP2. (b) Indicated the positive correlations between prime-locked amplitudes and arousal ratings of popular/unpopular information at channels F6, C6, P6. (c) Indicated the negative correlations between prime-locked ERP amplitudes and arousal ratings of popular/unpopular information at channels F5, CP5, PO5. Linear regression models and tendency lines were shown for each correlation graph. The linear R2 was shown on the top right of each correlation graph. The X-axis was the rating scores; Y-axis was mean prime-locked ERP amplitudes for the time window 0–1000 ms. The unit for amplitudes was µV.
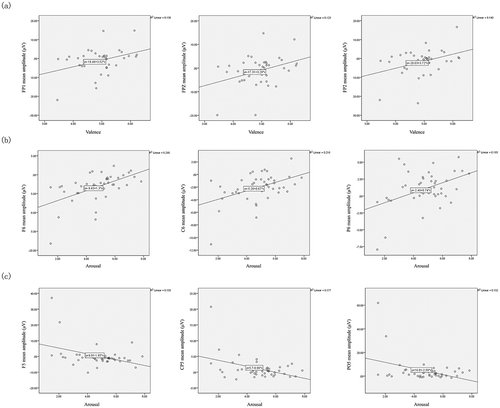
Discussion
We found that unpopular information elicited lower sentiment in terms of valence and arousal and survived better than popular information in Experiment 1. In Experiment 2, popular information showed ERP priming effect with the topography distributions at parietal area while prime effect was present at frontal area for unpopular information. Furthermore, arousal effect was distinct from valence effect on popularity in terms of ERP topography distributions.
Studies about sentiment analysis of social media are mostly based on thematic analysis methods (Dai, Han, Dai, & Xu, Citation2015). However, the challenge is quantitative measurements of emotions on social media. Currently, psychoinformatics and PAD model provide space to describe emotion status for information processing via experiments on social media (CitationLuo, Pan, Wang, & Huang; Mehrabian, Citation1996). In the study, observations in self-reported ratings are meaningful to understand the cognitive multi-dimension construct of information content on social media. In Experiment 1, lagged RT of emotional targets and preferential survival for unpopular information may be associated with the notion of ‘negative bias’ (Bebbington, MacLeod, Ellison, & Fay, Citation2017; Wang, Huang, Ma, & Li., Citation2012) and processing selectivity (Kalish, Griffiths, & Lewandowsky, Citation2007), which enables us to perceive and avoid potential danger.
Priming effect is usually explained by the ‘response level account’ theory (McNamara, Citation2005; Neely & Keefe, Citation1989), which assumes that priming stimuli automatically trigger response tendencies to facilitate the processing of subsequent stimuli. Consistently, the enhancement of positive amplitudes of targets after related priming information may be attributed to attention allocation or evaluation values of targets (Aquado, Diequez-Risco, Mendez-Bertolo, Pozo, & Hinojosa, Citation2013; Perez-Osorio, Muller, & Wykowska, Citation2017). In our study, participants paid more attention and evoked stronger evaluation of targets with high sentiment when the prime was popular information, and vice versa. We noticed that topography distributions of priming effect (Experiment 2) were different for popular/unpopular information. As researchers have agreed that dorsal attention system includes superior parietal cortex and frontal eye lobes, while the ventral attention system consists of the inferior temporal-parietal cortex and inferior frontal gyrus (Case, Citation1992; Corbetta & Shulman, Citation2002). The complex ventral and dorsal attention system may contribute to the explanation of different distributions of priming effect.
The positive correlations between valence ratings and prime-locked ERP amplitudes at bilateral frontal electrodes support previous literatures which demonstrated that brain activation in frontal areas was commonly related to alpha band to speed up the information processing of emotion stimuli (Kemp et al., Citation2002). But positive correlations between arousal and prime-locked ERP indicated the right-hemisphere preponderance, which may be related to late gamma band (Keil et al., Citation2001).
In conclusion, from the psychinformatic view, this study helps us to better understand the preliminary urge of popularity on social media. Unpopular information may capture more attention and survive better than popular information. The ERP results also confirmed the priming effect and indicated the distinct topography distributions of the multidimensional sentiment effect on popularity.
Author Contributions
YH developed the study concept. All authors contributed to the study design. Testing and data collection were performed by YH and YS. YH performed the data analysis under the supervision of XP, YM and QM. YH drafted the manuscript, and XP, YS, YM, QM provided critical revisions. LS provided help for the ERP study. All authors approved the final version of the manuscript for submission.
Data Availability
All data in the manuscript are available when requested.
Acknowledgments
We are appreciated that suggestion provided by Yufeng Dong about drafted manuscript. We also thank Zhongju Liao for the discussion on experiment design. We thank Xiaoyu Xu, Jing Yang, Hai Liu for collecting data.
Disclosure statement
No potential conflict of interest was reported by the authors.
Additional information
Funding
References
- Annett, M. (1970). A classification of hand preference by association analysis. British Journal of Psychology, 61(3), 303–321.
- Aquado, L., Diequez-Risco, T., Mendez-Bertolo, C., Pozo, M. A., & Hinojosa, J. A. (2013). Priming effects on the N400 in the affective priming paradigm with facial expressions of emotion. Cognitive, Affective & Behavioral Neuroscience, 13(2), 284–296.
- Bebbington, K., MacLeod, C., Ellison, T. M., & Fay, N. (2017). The sky is falling: Evidence of a negativity bias in the social transmission of information. Evolution and Human Behavior, 38, 92–101.
- Berger, J. (2011). Arousal increases social transmission of information. Psychological Science, 22(7), 891–893.
- Berger, J. (2014). Word of mouth and interpersonal communication: A review and directions for future research. Journal of Consumer Psychology, 24, 586–607.
- Berger, J., & Milkman, K. L. (2012). What makes online content viral. Journal of Marketing Research, 49(2), 192–205.
- Bollen, J., Pepe, A., & Mao, H. (2011). Modeling public mood and emotion: Twitter sentiment and socio-economic phenomena. In L. Adamic, R. Baeza-Yates, & S. Counts (Eds.), Proceedings of the fifth international AAAI conference on weblogs and social media. Palo Alto, CA
- Bradley, M. M., & Lang, P. J. (2000). Affective reactions to acoustic stimuli. Psychophysiology, 37(2), 204-215.
- Case, R. (1992). The role of the frontal lobes in the regulation of cognitive development. Brain and Cognition, 20(1), 51–73.
- Choi, M., & Toma, C. L. (2014). Social sharing through interpersonal media: Patterns and effects on emotional well-being. Computers in Human Behavior, 36, 530–541.
- Corbetta, M., & Shulman, G. L. (2002). Control of goal-directed and stimulus-driven attention in the brain. Nature Reviews Neuroscience, 3, 201–215.
- Dai, W., Han, D., Dai, Y., & Xu, D. (2015). Emotion recognition and affective computing on vocal social media. Information & Management, 52, 777–788.
- Delaney-Busch, N., Wilkie, G., & Kuperberg, G. (2016). Vivid: How valence and arousal influence word processing under different task demands. Cognitive, Affective, & Behavioral Neuroscience, 16, 415–432.
- Gabor, S., & Huberman Bernardo, A. (2010). Predicting the popularity of online content. Communications of the ACM, 53(8), 80–88.
- Hajcak, G., & Nieuwenhuis, S. (2006). Reappraisal modulates the electrocortical response to unpleasant pictures. Cognitive, Affective, & Behavioral Neuroscience, 6, 291–297.
- Hoedemaker, R. S., & Gordon, P. C. (2017). The onset and time course of semantic priming during rapid recognition of visual words. Journal of Experimental Psychology: Human Perception and Performance, 43(5), 881–902.
- Hu, Y., Hu, C., Fu, S., Fang, M., & Xu, W. (2017). Predicting key events in the popularity evolution of online information. PloS One, 12(1), e0168749.
- Hua, M., Han, Z., Chen, S., Yang, M., Zhou, R., & Hu, S. (2014). Late positive potential (LPP) modulation during affective picture processing in preschoolers. Biological Psychology, 101, 77–81.
- Kalish, M. I., Griffiths, T. L., & Lewandowsky, S. (2007). Iterated learning: Intergenerational knowledge transmission reveals inductive biases. Psychonomic Bulletin & Review, 14(2), 288.
- Keil, A., Muller, M. M., Gruber, T., Wienbruch, C., Stolarova, M., & Elbert, T. (2001). Effects of emotional arousal in the cerebral hemispheres: A study of oscillatory brain activity and event-related potentials. Clinical Neurophysiology, 112, 2057–2068.
- Kemp, A. H., Gray, M. A., Eide, P., Silberstein, R. B., & Nathan, P. J. (2002). Steady-state visually evoked potential topography during processing of emotional valence in healthy subjects. Neuroimage, 17(4), 1684–1692.
- Lasswell, H. D. (1948). The structure and function of communication in society. Communication Ideas, 24, 215–228.
- Luo, J., Pan, X., Wang, S., & Huang, Y. (2019). Identifying target audience on enterprise social network. Industrial Management & Data Systems, 46(4), 406–420.
- Ma, Q., Shen, Q., Xu, Q., Li, D., Shu, L., & Weber, B. (2011). Empathic responses to others’ gains and losses: An electrophysiological investigation. NeuroImage, 54(3), 2472–2480.
- McNamara, T. P. (2005). Semantic priming: Perspectives from memory and word recognition. New York, NY: Psychology Press.
- Mehrabian, A. (1996). Pleasure-arousal-dominance: A general framework for describing and measuring individual differences in temperament. Current Psychology, 14, 261–292.
- Meyer, D. E., & Schvaneveldt, R. W. (1971). Facilitation in recognizing pairs of words: Evidence of a dependence between retrieval operations. Journal of Experimental Psychology, 90(2), 227–234.
- Montag, C., Duke, E., & Markowetz, A. (2016). Toward Psychoinformatics: Computer science meets psychology. Computational and Mathematical Methods in Medicine, 2016, 2983685.
- Neely, J. H., & Keefe, D. E. (1989). Semantic context effects on visual word processing: A hybrid prospective/retrospective processing theory. The Psychology of Learning and Motivation, 24, 207–248.
- Perez-Osorio, J., Muller, H. J., & Wykowska, A. (2017). Expectations regarding action sequences modulate electrophysiological correlates of the gaze-cueing effect. Psychophysiology, 54(7), 942–954.
- Reece, A. G., Reagan, A. J., Lix, K. L. M., Dodds, P. S., Danforth, C. M., & Langer, E. J. (2017). Forecasting the onset and course of mental illness with Twitter data. Scientific Reports, 7, 13006.
- Salas-Zárate, M. D. P., Medina-Moreira, J., Lagos-Ortiz, K., Luna-Aveiga, H., Rodríguez-García, M. Á., & Valencia-García, R. (2017). Sentiment analysis on tweets about diabetes: An aspect-level approach. Computational and Mathematical Methods in Medicine, 2017, 5140631.
- Wang, B., Huang, Y., Wu, X., & Li, X. (2015). A fuzzy computing model for identifying polarity of Chines sentiment words. Computational Intelligence and Neuroscience, 2015, 525437.
- Wang, C., Zhou, Z., Jin, X., Fang, Y., & Lee, M. K. O. (2017). The influence of affective cues on positive emotion in predicting instant information sharing on microblogs: Gender as a moderator. Information Processing & Management, 53, 721–734.
- Wang, X., Huang, Y., Ma, Q., & Li., N. (2012). Event-related potential P2 correlates of implicit aesthetic experience. Neuroreport, 23(14), 862–866.
- Yarkoni, T. (2012). Psychoinformatics: New horizons at the interface of the psychological and computing sciences. Current Directions in Psychological Science, 21(6), 391–397.
- Yeung, N., Holroyd, C. B., & Cohen, J. D. (2005). ERP correlates of feedback and reward processing in the presence and absence of response choice. Cerebral Cortex, 15(5), 535–544.