Abstract
Aberrant DNA methylation is known to occur in cancer, including hematological malignancies such as acute myeloid leukemia (AML). However, less is known about whether specific methylation profiles characterize specific subcategories of AML. We examined this issue by using comprehensive high-throughput array-based relative methylation analysis (CHARM) to compare methylation profiles among patients in different AML cytogenetic risk groups. We found distinct profiles in each group, with the high-risk group showing overall increased methylation compared with low- and mid-risk groups. The differentially methylated regions (DMRs) distinguishing cytogenetic risk groups of AML were enriched in the CpG island shores. Specific risk-group associated DMRs were located near genes previously known to play a role in AML or other malignancies, such as MN1, UHRF1, HOXB3, and HOXB4, as well as TRIM71, the function of which in cancer is not well characterized. These findings were verified by quantitative bisulfite pyrosequencing and by comparison with results available at the TCGA cancer genome browser. To explore the potential biological significance of the observed methylation changes, we correlated our findings with gene expression data available through the TCGA database. The results showed that decreased methylation at HOXB3 and HOXB4 was associated with increased gene expression of both HOXB genes specific to the mid-risk AML, while increased DNA methylation at DCC distinctive to the high-risk AML was associated with increased gene expression. Our results suggest that the differential impact of cytogenetic changes on AML prognosis may, in part, be mediated by changes in methylation.
Keywords:
Abbreviations
AML | = | acute myeloid leukemia |
CHARM | = | comprehensive high-throughput array-based relative methylation analysis |
DMR | = | differentially methylated region |
TCGA | = | The Cancer Genome Atlas Research Network |
Introduction
Abnormal DNA methylation has been observed in acute myeloid leukemia (AML) and, therefore, proposed as a contributing factor to the pathogenesis of the disease.Citation1 AML patients have benefited from treatment with hypomethylating agents such as azacitidine and decitabine, although not all respond equally.Citation2 Previous studies showed associations of certain methylation patterns with specific chromosome abnormalities and gene mutations and suggested a prognostic significance to these patterns.Citation3–12 However, it remains unclear if DNA methylation profiles differ according to AML cytogenetic risk group, the principal predictor of outcome in AML.
This study used comprehensive high-throughput array-based relative methylation analysis (CHARM) to identify differentially methylated regions (DMRs) in AML patients with different cytogenetic risk profiles. Previous studies have demonstrated that CHARM, unbiasedly evaluating methylation changes in 4.6 million CpG sites across the genome, provides almost 100% sensitivity and 90% specificity.Citation13,14 We have found that unique sets of DMR can distinguish cytogenetic risk groups in AML. Those results were further validated using quantitative pyrosequencing and by analysis of additional independent patient cohorts.
Results
CHARM presented methylation profiles that are distinct to AML compared with normal samples and to each cytogenetic risk group of AML
Demographic information for the samples used in this study is presented in . By evaluating CpG sites throughout the entire genome, CHARM methylation profiling effectively distinguished 4 groups: (1) normal controls; (2) patients with low-risk cytogenetics; (3) patients with intermediate-risk cytogenetics; and (4) patients with high-risk cytogenetics (). Specifically, pairwise comparison indicated that the AML genome was characterized by increased methylation compared with age-matched controls ( top). CHARM analysis identified 668 DMRs associated with AML compared with normal controls, most of which showed hypermethylation in AML [641 (96%); bottom]. Among the AML patients, DMRs differentiating between cytogenetic risk groups were identified. The majority of the DMRs distinguishing high-risk from low- or mid-risk AML showed increased methylation in the high-risk group: 251 out of the 262 (96%; bottom) and 323 out of the 325 (99%; bottom). DMRs showed increased methylation in high-risk compared with low-risk and mid-risk, respectively. Among the 95 DMRs that differentiate between low- and mid-risk groups, 50 DMRs showed higher methylation in mid-risk, while 45 had higher methylation in the low-risk group ( bottom).
Figure 1. Heat map showing the methylation values of CHARM probes that differentiate among control and 3 cytogenetic risk groups of AML patients based on unsupervised agglomerative hierarchical clustering. Each column represents a patient. Each row denotes a probe. The top 20 probes are displayed as an example to show how the samples can be classified into 4 distinct groups, normal control, low-risk, mid-risk, and high-risk AML, based on the methylation values (Agglomerative Coefficient = 0.77).
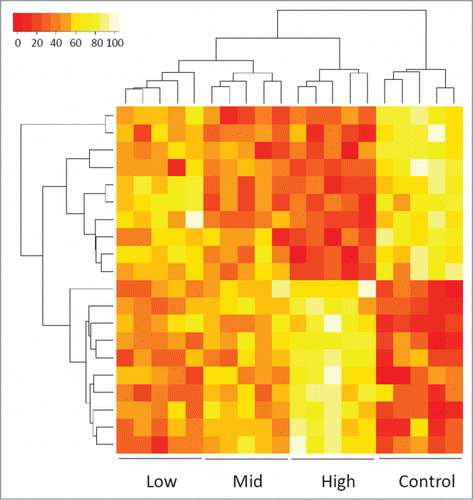
Figure 2. Overview of the CHARM analyses performed in the current study. Each panel presents results from a pairwise comparison: (A) Normal control vs. all AML; (B) low-risk vs. mid-risk AML; (C) mid-risk vs. high-risk AML; (D) low-risk vs. high-risk AML; (E) younger vs. older AML; and (F) younger vs. older control. Top: heatmaps of all the differentially methylated regions (DMRs) that distinguish the 2 groups, with each row representing a DMR. Bottom: Dot plots presenting the average methylation difference of each DMR generated using this pairwise comparison. Dots above the 0 line (blue) denote DMRs with higher methylation in the group on the right side of the corresponding heatmap.
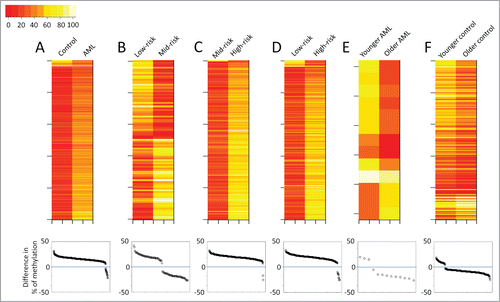
Table 1 Demographic information for all samples used in this study
Age effect on DNA methylation
Besides cytogenetic risk, we investigated the effect of age on DNA methylation by comparing 6 younger AML patients (age 18 to 26) with 6 older ones (age 53 to 60). Both groups included 2 each of the low-, mid-, and high-risk patients. Although older patients showed slightly reduced global methylation, DNA methylation profiles were largely similar between the 2 groups. Only 13 DMRs were statistically significant (P < 0.01; ), 10 of which showed lower methylation in the older patients ( bottom). To investigate if similar findings would be obtained in healthy individuals, we compared CD34+ enriched cells obtained from granulocyte colony-stimulating factor (G-CSF) mobilized peripheral blood apheresis collection from 5 younger (age 23 to 29) and 5 older (age 53 to 64) healthy individuals. Again, more DMRs showed decreased methylation with age (). Although 263 DMRs (P < 0.01) were identified between the 2 age groups ( top), the majority of the DMRs only showed a methylation difference less than 20% ( bottom).
DMRs distinguishing different risk groups of AML were enriched at CpG island shores
We next investigated the physical location of the DMRs in relation to CpG islands (CGIs; ). The DMRs distinguishing different cytogenetic risk groups were enriched at CpG island shores, defined as genomic regions within 2 kb of CGICitation14. Although 33% of the CHARM array probes target the CGIs because of their high CpG content, the DMRs located within CGIs were only 17–19% (). In contrast, while only 5% of the CHARM probes would theoretically hybridize to CGI shores because of the lower CpG content in these regions, approximately 15% of the DMRs distinguishing different cytogenetic risk groups were located at the CGI shores (P < 0.05). Conversely, the DMRs that differentiate AML as a whole from normal did not show significant enrichment at the CGI shores ().
Figure 3. Distribution of DMRs identified in pair-wise CHARM analyses in relation to CpG islands (CGI). (A) AML vs. Normal; (B) low- vs. mid-risk AML; (C) low- vs. high-risk AML; and (D) mid- vs. high-risk AML. As denoted on the X axis, DMR positions are defined as “islands” (cover or overlap more than 50% of a CGI), “overlap” (0.1–50% of a CGI), or located 0–500 bp, 500–1000 bp, 1000–2000 bp, 2000–3000 bp, or greater than 3000 bp from the nearest CGI. The percentage of each group is presented for the DMRs of interest (filled circles). The empty squares present the null distribution (Mean±STD), calculated by repeated (100 times) simulation using genomic fragments randomly selected among CpG regions targeted by the array.
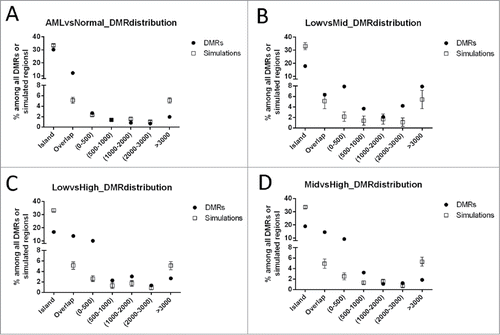
DMRs associated with individual cytogenetic risk groups were identified
We next investigated which specific DMRs were associated with each cytogenetic risk group (). In order to identify DMRs associated with low-cytogenetic risk AML, we examined the overlap of DMRs from the low- vs. mid-risk and low- vs. high-risk comparisons and identified 25 regions. Five of these DMRs (20%) showed increased methylation compared with the other 2 AML risk groups whereas 20 (80%) showed decreased methylation. Of these 25 low-risk associated DMRs, 4 were also identified in the normal vs AML comparison. They showed lower methylation in AML than in normal samples; likewise, they displayed lower methylation in the low-risk AML than in other AML. Similarly, we found 23 mid-risk associated DMRs, with decreased methylation in the mid-risk group compared with other AML groups. Among these, 12 were also found to differentiate AML and normal samples, with higher methylation in AML. Interestingly, high-risk AML defined by cytogenetics showed increased global methylation compared with low- and mid-risk AML, with a total of 133 DMRs identified, among which 43 showed AML associated hypermethylation relative to normal. Examples of DMRs associated with each cytogenetic risk group are listed in with their location relative to the closest CGI and gene(s) specified.
Table 2 The numbers of Differentially Methylated Regions (DMRs) associated with AML and each cytogenetic risk group
Table 3: Representative DMRs associated with individual cytogenetic risk groups of AML
Bisulfite pyrosequencing verification of DMRs associated with specific cytogenetic risk groups
To verify the methylation changes identified by CHARM, we selected DMRs associated with different cytogenetic risk groups and performed 2 types of validations: (1) technical validation by measuring the methylation level in the DMR sub-regions using quantitative bisulfite pyrosequencing ( and ); and (2) external validation using results available at the TCGA cancer genome browserCitation15 (https://genome-cancer.ucsc.edu/) (Additional File 1) . We selected 8 DMRs based on the DMR ranking by CHARM and the availability of pre-designed assays. Among those DMRs, MN1, TRIM71, and UHRF1 were found to be associated with low-risk AML and demonstrated decreased methylation (P < 0.005; and ). These differences remain significant after Bonferroni correction for 8 tests (Alpha value lowered from 0.05 to 0.00625). The MN1 pyrosequencing analysis differentiated the low-risk group from the mid- and high-risk groups by 20% and 23%, respectively. The TRIM71 pyrosequencing assay showed 59% and 42% lower methylation in the low-risk group compared with the mid- and high-risk groups, respectively. One DMR associated with mid-risk AML, near genes HOXB3 and HOXB4, displayed decreased methylation in mid-risk compared to other AML patients (P = 0.014; and ). This difference would not be statistically significant with a Bonferroni correction. Four DMRs associated with high-risk AML (NEFL, DCC, PITX1 and one near DUOX2 and DUOXA2), displayed a higher average methylation in the high-risk cytogenetic group relative to all other AML patients (). However, due to the high variability in this group (standard deviation over mean of the methylation value was 77% for NEFL, 62% for DCC, 71% for PITX1, and 92% for DUOX2), the differences seen in these high-risk associated DMRs were not statistically significant by pyrosequencing (). We were able to obtain methylation data of AML patients from the TCGA cancer genome browser for all the above DMRs except the one in intron 1 of MN1. Consistent with our findings, the data from “TCGA acute myeloid leukemia (LAML) DNA methylation (HumanMethylation450)” showed a low-risk specific decrease of methylation in TRIM71 and UHRF1, as well as mid-risk specific decrease of methylation in HOXB3/4 (Additional File 1A, B, and C). The methylation in DCC, NEFL, DUOX2/DUOXA2, and PITX1 was similar among all 3 risk groups of AML (Additional File 1D, E, and F).
Figure 4. Methylation levels in DMRs and expression of nearby genes. (A) MN1; (B) TRIM71; (C) UHRF1; (D) HOXB3 and HOXB4; (E) DCC; (F) NEFL; (G) DUOX2 and DUOXA2; (H) PITX1. Within each panel: the plot on the left presents DNA methylation levels of DMR and adjacent genomic regions measured by CHARM from low-, mid, and high-risk AML (n = 5 in each), with the relative locations of the gene, CGI(s), DMR, and the pyrosequenced region shown above; the plot in the middle presents DNA methylation levels of each CpG site within a sub-region of the DMR measured by quantitative bisulfite pyrosequencing (8 low-, 10 mid-, and 10 high-risk patients; n = 28 total); the right panel shows the average mRNA expression Z scores (±SE) downloaded from cBioPortal for Cancer Genomics (32 low-, 101 mid-, and 37 high-risk patients). Low-, mid-, and high-risk AML were denoted using green, orange, and red, respectively. Lines demonstrate the Loess fitted mean per group. Asterisks in the expression plots denote expression in a given risk-group that is significantly different from the 2 other groups based on ANOVA followed by TukeyHSD tests.
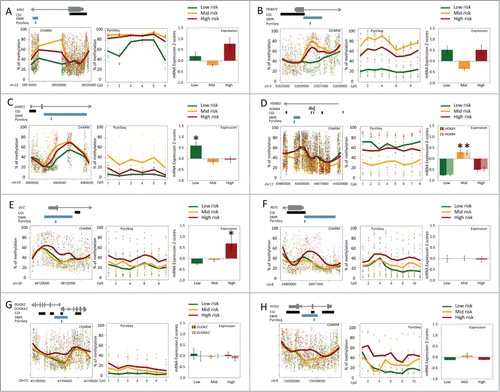
Table 4 DMRs verified using quantitative bisulfite pyrosequencing
Expression changes in DMR associated genes
Methylation changes often lead to expression alterations in adjacent genes, which can lead to functional changes in the cell. We, therefore, investigated if there were specific expression changes associated with methylation of those DMRs identified in our study. We extracted data from the publically available TCGA database and compared the mRNA expression z-scores in AML patients stratified into low- (n = 32), mid- (n = 101), and high-risk (n = 37) groups based on cytogenetic findings (). The mRNA expression z-scores of each gene were obtained through comparing the RNA seq V2 RSEM (RNA-Seq by Expectation Maximization) results with the expression distribution in tumors that are diploid for the gene (cBioPortal for Cancer Genomics at http://www.cbioportal.org/public-portal/).Citation16,17 Comparison between methylation and expression showed that, for some genes, alterations in methylation were associated with changes in expression (). Among the DMRs associated with low-risk AML, UHRF1 showed expression in low-risk that was significantly different from both mid- and high-risk groups (, P < 0.01). The decreased methylation in intron 2 and promoter CpG island shore of UHRF1 was associated with increased gene expression. A mid-risk associated DMR, located in intron 1 of HOXB3 and near the 5′ of HOXB4, showed decreased methylation () compared with low- and high-risk groups. The expression of HOXB3 and HOXB4 in mid-risk AML was significantly higher than in low- and high-risk AML (, P < 0.0001). Among the high-risk associated DMRs, deleted in colorectal carcinoma gene (DCC) showed an mRNA expression level that was distinctive in high-risk AML. The increased methylation at the 5′ untranslated region (5′UTR) was associated with higher expression of DCC in high-risk AML patients (, P < 0.02)
Discussion
By applying CHARM, a whole-genome methylation analysis, we have demonstrated that different cytogenetic risk groups of AML are epigenetically distinct. Pairwise comparison between AML and normal controls showed that AML samples were relatively hypermethylated. This observation is consistent with a recent study by TCGA,Citation1 which showed increased methylation, particularly in CpG dense regions, of AML patients compared with healthy controls. Similarly, Figueroa et al. showed most of the methylation signatures distinguishing between normal and AML as a whole showed hypermethylation, although certain subtypes of AML, such as the NPM1-mutation subgroup showed even distribution of hyper- and hypomethylation compared with normal.Citation12 Pairwise comparisons between the AML cytogenetic risk groups indicated that global DNA methylation profiles differed significantly and that a set of specific DMRs can be used to differentiate cytogenetic risk groups.
DNA methylation changes associated with specific subgroups of AML were reported in previous studies. Wilop et al. reported that DNA methylation patterns tend to be similar between AML samples with the same chromosomal abnormalitiesCitation18; similarly, Denerberg et al. has shown a non-significant trend for global methylation differences among cytogenetic or molecular (FLT3, NPM1, CEBPA, and RAS) subgroups of AML.Citation19 Figueroa et al. identified well-defined methylation patterns associated with specific chromosome abnormalities in AML, such as inv(16)/t(16;16), t(8;21), t(15;17), deletions of 5q and 7q, as well as gene mutations of NPM1 and CEBPA.Citation12 They also identified 5 methylation signatures with no other common morphologic or molecular features, but with distinct clinical outcome. Finally, the TCGA study showed distinct DNA methylation patterns in specific gene-fusion and molecular subtypes of AML patients, particularly in CpG-sparse regions of the genome.Citation1 Our study is unique in its identification of specific DMRs associated with each cytogenetic risk group. We were able to validate our findings from CHARM in half of the DMRs chosen for further evaluation using quantitative pyrosequencing. Using the independent TCGA data set, we also found that differences in methylation levels were related with differences in mRNA expression levels in selected genes.
Various methods exist for array-based genome-wide methylation profiling. The CHARM method was based on the McrBC method, which had been shown by a rigorous comparison study to have the best overall performance considering sensitivity and segment sizes in comparison with the HELPCitation12 and the MeDIP methods.Citation13 However, no direct comparison is available for the CHARM method and the Infinium Methylation Assay commonly used currently (Human Methylation 450 BeadChip Kit by Illumina). CHARM assessed the largest number (4.6 million) of CpG loci and genes among all known methylation profiling platforms.
In our study, there were more DMRs unique to high-risk AML (133) than to the low-(25) and mid-risk (23) groups; this indicates a more aberrant state of the epigenome in patients with aggressive and advanced disease. Interestingly, while the majority of the low- and mid-risk associated DMRs were characterized by a decrease in methylation, all of the high-risk associated DMRs demonstrated increased methylation compared with other groups.
Some of these cytogenetic risk associated DMRs mapped to genes previously shown to play a role in AML or other malignancies. For example, the MN1 oncogene is dysregulated in AML, and multiple studies have shown a link between high MN1 expression and poor clinical outcome in cytogenetically normal AML (CN-AML),Citation20,21 while low levels of MN1 expression were associated with favorable outcomes.Citation22 Our study demonstrated lower methylation in low-risk AML of a DMR located in intron 1 of MN1, within the promoter CpG island shore. Moreover, analyses of expression data showed a trend of increased expression in the high-risk group compared with low- and mid-risk [ANOVA P < 0.0001, Tukey HSD comparison P (low vs. high) = 0.054 and P (mid vs. high) < 0.0001]. (). In the case of HOXB3, we showed decreased methylation in mid-risk compared with other AML samples. Consistent with the findings of Roche et al who compared the expression of multiple HOX genes in different cytogenetic risk groups and found the expression restricted to mid-risk AML by cytogenetics,Citation23 our analysis of the TCGA data (2013) showed higher HOXB3 and HOXB4 expression in mid-risk AML patients than in patients of other risk groups (). Although there are different theories regarding the effect of aberrant DNA methylation on gene expression, our observations are largely consistent with recent consensus: hypermethylation in a gene body would increase gene expression, whereas methylation in the gene promoter would decrease gene expression. Perhaps somewhat unexpected was a high-risk AML associated DMR mapped to the deleted in colorectal carcinoma gene (DCC). DCC has been reported to function as a tumor suppressor gene in colorectal carcinoma and in other human malignancies including AML.Citation24 Inokuch et al reported that decreased DCC expression is associated with a poor prognosis in AML.Citation24 However, we identified a DMR at the 5′UTR of the gene near the DCC promoter CpG island with increased methylation in the high-risk AML samples. Its expression based on TCGA data was higher in the high-risk AML compared with other AML patients (), contradicting previous reports. Additional validation of DCC methylation and expression levels associated with AML outcome is warranted.
Pyrosequencing analyses targeting subregions of high-risk associated DMRs showed that although the methylation values on average were increased in high-risk AML, the differences were not statistically significant due to high variability. This may be explained by the stochastic state of the methylome in advanced cancerCitation25 and the technical distinctions between pyrosequencing and CHARM. CHARM identifies methylation differences across contiguous genomic regions using comprehensive algorithms such as genome-weighted smoothing. Therefore, it is possible that although CHARM identified significant methylation difference in a 2 Mb genomic region, not all subregions targetable by pyrosequencing (less than 200 bp) would show statistically significant differences.
We also explored the effect of age on DNA methylation in AML, because age is a well-studied risk factor in AML and changes in methylation are also associated with aging. Recent studies targeting the whole genome demonstrated overall hypomethylation in association with aging as well as increased methylation in specific promoter CGIs.Citation26–28 In this study, we also observed decreased methylation in older patients. However, the methylation difference between “younger” and “older” AML patients was less prominent than the difference between cytogenetic risk groups. The comparison of “younger” and “older” CD34+ cells from age-matched healthy controls showed very similar methylation profiles to those of the AML patients, such that the older individuals showed decreased overall methylation, but the methylation difference in age-related DMRs was relatively small compared with that of the AML related and cytogenetic risk associated DMRs. This finding suggests that the age span in this study is not big enough between the “younger” (<30 y) and the “older” (50–60 y) groups to show a prominent age effect. Most AML studies use age 60 y to group “young” and “old” patients.
CHARM measures methylation changes of CpG sites across the genome, which provides the opportunity to assess the location of DMRs in relation to CpG islands as well as different components of genes. A previous study showed that aberrant methylation in colon cancer is enriched in CpG island shores,Citation14 similar to what we observed in prostate cancer.Citation29 In the current study, DMRs that differentiate between normal and AML did not show significant enrichment at the island shore comparing with simulated genomic regions. Unlike colon and prostate cancers, AML is characterized by aberrant hypermethylation that occurs mostly at the CpG island. This indicates that aberrant DNA methylation in different types of cancer may have distinct physical distributions in the genome. Nevertheless, DMRs that differentiate AML cytogenetic subgroups demonstrated enrichment in the shore regions.
In summary, this current study showed that AML is characterized by aberrant hypermethylation; however, different cytogenetic risk groups of AML display distinct patterns of DNA methylation. This suggests DNA methylation levels may be useful for prognosis. Future studies are needed to investigate whether the aberrant methylation profiles facilitate or merely coexist with various chromosomal or molecular abnormalities. Moreover, now that DMRs have been demonstrated to help distinguish cytogenetic risk groups, they may also be useful for prognosis in AML patients without cytogenetic abnormalities, which is the largest subgroup of AML.
Methods
Patients and materials
Bone marrow samples were obtained from the Fred Hutchinson Cancer Research Center (FHCRC) leukemia repository, Seattle. Fifteen age-matched patients were divided into 3 equal groups based on cytogenetics: (1) high-risk AML, defined as patients with a complex or monosomal karyotype, inv(3)/t(3;3), or t(6;9); (2) intermediate-risk AML, defined as patients with normal karyotype, trisomy 8, t(9;11), or others; and (3) low-risk AML, defined as patients with t(8;21) or inv(16). Patients with t(15;17) were excluded. Five age-matched normal individuals (within 5-years of age difference) served as control. Thirteen additional AML samples were used for DMR validation. To study age effects, CD34+ enriched granulocyte colony-stimulating factor (G-CSF) mobilized peripheral blood samples were obtained from the Core Center of Excellence (CCEH) Haematopoietic Cell Repository at FHCRC. All samples were collected under protocols approved by the FHCRC Institutional Review Board and consent was provided in keeping with international standards set forth by the Helsinki Agreement.
Comprehensive High-throughput Array-based Relative Methylation analysis (CHARM)
Genomic DNA was extracted using the Gentra Puregene Blood Kit (Qiagen, Cat no. 158422). Sample preparation was performed using the method described previously.Citation30 Genomic DNA was sheared, digested with McrBC, gel-purified, labeled and hybridized to a customized NimbleGen HD2 array (Roche, Cat no. 05224390001). The array uses tiled 50mer-probes targeting 4.6 million CpG sites across the genome. Data were analyzed and the significance of differentially methylated regions was calculated with R and the Bioconductor CHARM package using previously described algorithms.Citation14 Briefly, for every pairwise comparison, the average methylation values (M) of individual probes across the 5 samples per cytogenetic risk group were calculated. The difference of averaged M values (ΔM) and standard errors (s.e.m.) of the samples were used to calculate z scores (ΔM /s.e.m.(: ΔM)). Probes carrying z-scores with a False Discovery Rate (FDR) of 5% or lower were identified as statistically significant. Regions with contiguous statistically significant values were identified as differential methylation regions (DMRs). The statistical significance of each DMR was calculated using a permutation test. Those DMRs with P values smaller than 0.01 were considered statistically significant.
Identification of cytogenetic risk associated DMRs
CHARM analysis by pairwise comparisons identified DMRs between any 2 cytogenetic risk groups. A similar comparison was carried out between normal and all AML. DMRs associated with low-cytogenetic risk AML were identified as genomic regions overlapping the DMRs from the low- vs. mid-risk and low- vs. high-risk comparisons. Similar steps were taken to generate mid- and high-risk AML associated DMRs.
Bisulfite pyrosequencing
Bisulfite pyrosequencing was performed by EpigenDx on all the samples from the CHARM cohort plus 13 additional AML samples (). Sequences analyzed include MN1, TRIM71, UHRF1, HOXB3, HOXB4, DCC, NEFL, DUOX2, DUOXA2, and PITX1. The genomic coordinates of the specific target sequences are shown in the Results.
Gene expression analysis
mRNA Expression z-Scores (RNA Seq V2 RSEM) deposited by the Cancer Genome Atlas Research Network (TCGA) study were downloaded from cBioPortal for Cancer Genomics. mRNA data were compared among low- (n = 32), mid- (n = 101), and high-risk (n = 37) AML patients based on cytogenetic classification provided by the study. Targeted statistical analyses were then performed between different risk groups via the unpaired t test.
Statistical analysis
All statistical analyses were performed using R unless explained otherwise. To determine the statistical significance of the P values with 8 tests, the α value was adjusted using Bonferroni method (http://www.graphpad.com/guides/prism/6/statistics/index.htm?stat_the_bonferroni_method.htm), which divides the significance threshold (0.05) by the number of tests (n = 8).
Disclosure of Potential Conflicts of Interest
No potential conflicts of interest were disclosed.
Authorship Contributions
XQ and LD performed the experiments. XQ, JD, LD, BS, and MF analyzed and interpreted the data. DLS and SH provided repository materials and technical expertise. EE and FRA provided clinical expertise and advised on study design. MF and XQ designed the study and obtained funding. All authors contributed to the writing process and approved the final manuscript.
Supplemental_Material.pdf
Download PDF (160.6 KB)Funding
This study was supported in part by P30 CA015704. Technical development and generation of normal control data were supported by a Pilot & Feasibility grant awarded to X Qu and M Fang from DK056465 Core Center of Excellence in Hematology. Some of the AML samples were obtained from the FHCRC Leukemia Repository, which is supported by CA018029.
Supplemental Material
Supplemental data for this article can be accessed on the publisher's website.
References
- Cancer Genome Atlas Research N. Genomic and epigenomic landscapes of adult de novo acute myeloid leukemia. N Engl J Med 2013; 368:2059–74; PMID:23634996; http://dx.doi.org/10.1056/NEJMoa1301689
- Estey EH. Epigenetics in clinical practice: the examples of azacitidine and decitabine in myelodysplasia and acute myeloid leukemia. Leukemia 2013; 27:1803–12; PMID:23757301; http://dx.doi.org/10.1038/leu.2013.173
- Ohgami RS, Ma L, Ren L, Weinberg OK, Seetharam M, Gotlib JR, Arber DA. DNA methylation analysis of ALOX12 and GSTM1 in acute myeloid leukaemia identifies prognostically significant groups. Brit J Haematol 2012; 159:182–90; PMID:22924777; http://dx.doi.org/10.1111/bjh.12029
- Oing C, Jost E, Dahl E, Wilop S, Brummendorf TH, Galm O. Aberrant DNA hypermethylation of the ITIH5 tumor suppressor gene in acute myeloid leukemia. Clin Epigenetics 2011; 2:419–23; PMID:22704354; http://dx.doi.org/10.1007/s13148-011-0043-5
- Yan P, Frankhouser D, Murphy M, Tam HH, Rodriguez B, Curfman J, Trimarchi M, Geyer S, Wu YZ, Whitman SP, et al. Genome-wide methylation profiling in decitabine-treated patients with acute myeloid leukemia. Blood 2012; 120:2466–74; PMID:22786882; http://dx.doi.org/10.1182/blood-2012-05-429175
- Deneberg S, Guardiola P, Lennartsson A, Qu Y, Gaidzik V, Blanchet O, Karimi M, Bengtzen S, Nahi H, Uggla B, et al. Prognostic DNA methylation patterns in cytogenetically normal acute myeloid leukemia are predefined by stem cell chromatin marks. Blood 2011; 118:5573–82; PMID:21960591; http://dx.doi.org/10.1182/blood-2011-01-332353
- Negrotto S, Ng KP, Jankowska AM, Bodo J, Gopalan B, Guinta K, Mulloy JC, Hsi E, Maciejewski J, Saunthararajah Y. CpG methylation patterns and decitabine treatment response in acute myeloid leukemia cells and normal hematopoietic precursors. Leukemia 2012; 26:244–54; PMID:21836612; http://dx.doi.org/10.1038/leu.2011.207
- Lin TC, Hou HA, Chou WC, Ou DL, Yu SL, Tien HF, Lin LI. CEBPA methylation as a prognostic biomarker in patients with de novo acute myeloid leukemia. Leukemia 2011; 25:32–40; PMID:20927134; http://dx.doi.org/10.1038/leu.2010.222
- Lugthart S, Figueroa ME, Bindels E, Skrabanek L, Valk PJ, Li Y, Meyer S, Erpelinck-Verschueren C, Greally J, Lowenberg B, et al. Aberrant DNA hypermethylation signature in acute myeloid leukemia directed by EVI1. Blood 2011; 117:234–41; PMID:20855866; http://dx.doi.org/10.1182/blood-2010-04-281337
- Alvarez S, Suela J, Valencia A, Fernandez A, Wunderlich M, Agirre X, Prosper F, Martin-Subero JI, Maiques A, Acquadro F, et al. DNA methylation profiles and their relationship with cytogenetic status in adult acute myeloid leukemia. PloS One 2010; 5:e12197; PMID:20808941; http://dx.doi.org/10.1371/journal.pone.0012197
- Griffiths EA, Gore SD, Hooker CM, Mohammad HP, McDevitt MA, Smith BD, Karp JE, Herman JG, Carraway HE. Epigenetic differences in cytogenetically normal versus abnormal acute myeloid leukemia. Epigenetics 2010; 5:590–600; PMID:20671427; http://dx.doi.org/10.4161/epi.5.7.12558
- Figueroa ME, Lugthart S, Li Y, Erpelinck-Verschueren C, Deng X, Christos PJ, Schifano E, Booth J, van Putten W, Skrabanek L, et al. DNA methylation signatures identify biologically distinct subtypes in acute myeloid leukemia. Cancer Cell 2010; 17:13–27; PMID:20060365; http://dx.doi.org/10.1016/j.ccr.2009.11.020
- Irizarry RA, Ladd-Acosta C, Carvalho B, Wu H, Brandenburg SA, Jeddeloh JA, Wen B, Feinberg AP. Comprehensive high-throughput arrays for relative methylation (CHARM). Genome Res 2008; 18:780–90; PMID:18316654; http://dx.doi.org/10.1101/gr.7301508
- Irizarry RA, Ladd-Acosta C, Wen B, Wu Z, Montano C, Onyango P, Cui H, Gabo K, Rongione M, Webster M, et al. The human colon cancer methylome shows similar hypo- and hypermethylation at conserved tissue-specific CpG island shores. Na Genet 2009; 41:178–86; PMID:19151715; http://dx.doi.org/10.1038/ng.298
- Cline MS, Craft B, Swatloski T, Goldman M, Ma S, Haussler D, Zhu J. Exploring TCGA Pan-Cancer data at the UCSC Cancer Genomics Browser. Sci Rep 2013; 3:2652; PMID:24084870; http://dx.doi.org/10.1038/srep02652
- Cerami E, Gao J, Dogrusoz U, Gross BE, Sumer SO, Aksoy BA, Jacobsen A, Byrne CJ, Heuer ML, Larsson E, et al. The cBio cancer genomics portal: an open platform for exploring multidimensional cancer genomics data. Cancer Dis 2012; 2:401–4; PMID:22588877; http://dx.doi.org/10.1158/2159-8290.CD-12-0095
- Gao J, Aksoy BA, Dogrusoz U, Dresdner G, Gross B, Sumer SO, Sun Y, Jacobsen A, Sinha R, Larsson E, et al. Integrative analysis of complex cancer genomics and clinical profiles using the cBioPortal. Sci Signal 2013; 6:pl1; PMID:23550210; http://dx.doi.org/10.1126/scisignal.2004088
- Wilop S, Fernandez AF, Jost E, Herman JG, Brummendorf TH, Esteller M, Galm O. Array-based DNA methylation profiling in acute myeloid leukaemia. Br J Haematol 2011; 155:65–72; PMID:21790528; http://dx.doi.org/10.1111/j.1365-2141.2011.08801.x
- Deneberg S, Grovdal M, Karimi M, Jansson M, Nahi H, Corbacioglu A, Gaidzik V, Dohner K, Paul C, Ekstrom TJ, et al. Gene-specific and global methylation patterns predict outcome in patients with acute myeloid leukemia. Leukemia 2010; 24:932–41; PMID:20237504; http://dx.doi.org/10.1038/leu.2010.41
- Aref S, Ibrahim L, Morkes H, Azmy E, Ebrahim M. Meningioma 1 (MN1) expression: refined risk stratification in acute myeloid leukemia with normal cytogenetics (CN-AML). Hematology 2013; 18:277–83; PMID:23394438; http://dx.doi.org/10.1179/1607845412Y.0000000065
- Langer C, Marcucci G, Holland KB, Radmacher MD, Maharry K, Paschka P, Whitman SP, Mrozek K, Baldus CD, Vij R, et al. Prognostic importance of MN1 transcript levels, and biologic insights from MN1-associated gene and microRNA expression signatures in cytogenetically normal acute myeloid leukemia: a cancer and leukemia group B study. J Clin Oncol 2009; 27:3198–204; PMID:19451432; http://dx.doi.org/10.1200/JCO.2008.20.6110
- Schwind S, Marcucci G, Kohlschmidt J, Radmacher MD, Mrozek K, Maharry K, Becker H, Metzeler KH, Whitman SP, Wu YZ, et al. Low expression of MN1 associates with better treatment response in older patients with de novo cytogenetically normal acute myeloid leukemia. Blood 2011; 118:4188–98; PMID:21828125; http://dx.doi.org/10.1182/blood-2011-06-357764
- Roche J, Zeng C, Baron A, Gadgil S, Gemmill RM, Tigaud I, Thomas X, Drabkin HA. Hox expression in AML identifies a distinct subset of patients with intermediate cytogenetics. Leukemia 2004; 18:1059–63; PMID:15085154; http://dx.doi.org/10.1038/sj.leu.2403366
- Inokuchi K, Yamaguchi H, Hanawa H, Tanosaki S, Nakamura K, Tarusawa M, Miyake K, Shimada T, Dan K. Loss of DCC gene expression is of prognostic importance in acute myelogenous leukemia. Clin Cancer Res 2002; 8:1882–8; PMID:12060632
- Hansen KD, Timp W, Bravo HC, Sabunciyan S, Langmead B, McDonald OG, Wen B, Wu H, Liu Y, Diep D, et al. Increased methylation variation in epigenetic domains across cancer types. Nat Genet 2011; 43:768–75; PMID:21706001; http://dx.doi.org/10.1038/ng.865
- McClay JL, Aberg KA, Clark SL, Nerella S, Kumar G, Xie LY, Hudson AD, Harada A, Hultman CM, Magnusson PK, et al. A methylome-wide study of aging using massively parallel sequencing of the methyl-CpG-enriched genomic fraction from blood in over 700 subjects. Hum Mol Genet 2014; 23:1175–85; PMID:24135035; http://dx.doi.org/10.1093/hmg/ddt511
- Heyn H, Li N, Ferreira HJ, Moran S, Pisano DG, Gomez A, Diez J, Sanchez-Mut JV, Setien F, Carmona FJ, et al. Distinct DNA methylomes of newborns and centenarians. Proc Nat Acad Sci U S A 2012; 109:10522–7; PMID:22689993; http://dx.doi.org/10.1073/pnas.1120658109
- Issa JP. Aging and epigenetic drift: a vicious cycle. J Clin Invest 2014; 124:24–9; PMID:24382386; http://dx.doi.org/10.1172/JCI69735
- Qu X, Bluemn E, Nelson P, Vessella R, Fang M. Genome-wide methylation analyses in advanced-stage prostate cancer models. Cancer Res 2012; 72:A25.
- Ladd-Acosta C, Aryee MJ, Ordway JM, Feinberg AP. Comprehensive high-throughput arrays for relative methylation (CHARM). Curr Protoc Hum Genet 2010; Chapter 20:Unit 20 1 1-19; PMID:20373514