Abstract
Germline pathogenic mutations in BRCA1 increase risk of developing breast cancer. Screening for mutations in BRCA1 frequently identifies sequence variants of unknown pathogenicity and recent work has aimed to develop methods for determining pathogenicity. We previously observed that tumor DNA methylation can differentiate BRCA1-mutated from BRCA1-wild type tumors. We hypothesized that we could predict pathogenicity of variants based on DNA methylation profiles of tumors that had arisen in carriers of unclassified variants. We selected 150 FFPE breast tumor DNA samples [47 BRCA1 pathogenic mutation carriers, 65 BRCAx (BRCA1-wild type), 38 BRCA1 test variants] and analyzed a subset (n=54) using the Illumina 450K methylation platform, using the remaining samples for bisulphite pyrosequencing validation. Three validated markers (BACH2, C8orf31, and LOC654342) were combined with sequence bioinformatics in a model to predict pathogenicity of 27 variants (independent test set). Predictions were compared with standard multifactorial likelihood analysis. Prediction was consistent for c.5194-12G>A (IVS 19-12 G>A) (P>0.99); 13 variants were considered not pathogenic or likely not pathogenic using both approaches. We conclude that tumor DNA methylation data alone has potential to be used in prediction of BRCA1 variant pathogenicity but is not independent of estrogen receptor status and grade, which are used in current multifactorial models to predict pathogenicity.
Introduction
Breast cancer remains the most diagnosed cancer in women, with an overall incidence in the UK of 1 in 8 (http://www.cancerresearchuk.org/cancer-info/cancerstats/). Fortunately, advances in treatment and screening have resulted in reduced mortality rate.Citation1 We now understand that breast cancer is a heterogeneous disease, with different individuals benefiting from different treatments depending on hormone receptor expression and genetic mutations. Distinct novel molecular subgroups have been identified using a wide array of technologies, suggesting the need for novel therapies targeting specific molecular alterations.Citation2,3 A minority of breast cancers (5-10%) is considered hereditary, and approximately a quarter of these are due to germline mutations in known cancer susceptibilities genes, including BRCA1 (OMIM# 113705) and BRCA2 (OMIM# 600185).Citation4 Families presenting with multiple breast cancer cases and families with early onset ovarian cancer are currently tested by sequencing these two genes, and this informs clinicians in the management of individual treatment, and decisions concerning screening, chemoprevention or prophylactic surgery for unaffected mutation carriers. Both triple negative tumors and BRCA1 germline mutated tumors are significantly enriched in the basal subtype, which is associated with poor prognosis. Current estimates suggest that up to 15% of triple negative tumors are BRCA1 germline mutation carriers.Citation5,6
BRCA1 variants that are considered pathogenic mutations include those leading to protein truncation or mRNA degradation, and risk-associated missense alterations affecting protein function accompanied by clinical evidence of pathogenicity. However, there are also a considerable number of BRCA1 variants of uncertain clinical significance (>500 different variantsCitation7) namely missense variants with undetermined effect on function or risk, small insertions or deletions, or alterations in noncoding sequence. Attempts to classify these unclassified variants have utilized a range of analyses. Segregation data can be used to identify if a variant tracks with disease within a family; however, this is difficult to apply to some variants since they are individually rare.Citation8 In silico approaches have been used to examine sequence evolutionary conservation predicting the effect of specific amino acid substitutionsCitation9 and potential splicing alterations.Citation10 A multifactorial model has been developed to integrate these different data together, starting with an empirical prior probability based on bioinformatics predictions and incorporating likelihood ratios derived from independent data sources to generate a posterior probability of pathogenicity.Citation8 The advantages of using this approach are that it incorporates all available information from multiple data types in a single analysis with a numeric output and allows for the addition of extra data.Citation11 The posterior probability generated can then be used to classify the variant into 1 of 5 classes: Class 1 (probability <0.001) – non pathogenic, or of no clinical significance; Class 2 (probability 0.001-0.049) – likely non pathogenic or of little clinical significance; Class 3 (probability 0.05-0.949) – uncertain; Class 4 (probability 0.95-0.99) – likely pathogenic; Class 5 (probability >0.99) – definitely pathogenic.Citation12
We have previously shown that breast tumors from patients with pathogenic germline BRCA1 mutations have distinct DNA methylation profiles compared to familial breast cancer cases with no BRCA1 or BRCA2 mutations (BRCAx).Citation13 In this previous study, 81.3% of tumors with germline mutated BRCA1 were correctly predicted using a support vector learning machine approach based on DNA methylation data. The methylation profiles of BRCAx tumors are very similar to those of sporadic breast cancers.Citation14 The present study aimed to assess the DNA methylation of both published candidate genes and novel regions that differ between tumors from germline BRCA1-mutation carriers (referred to as BRCA1 tumors) and tumors from BRCAx families (germline BRCA1-wild type), with the goal of providing an additional tool useful for the classification of unclassified BRCA1 variants.
Results
DNA methylation of prior candidate genes is dependent on estrogen receptor (ER) status
Candidate regions were identified from previous dataCitation13,14 in which DNA methylation levels were significantly different between tumors of BRCA1-mutation carriers and those of BRCAx individuals (Table S1). Twelve candidate regions were analyzed in bisulphite converted DNA from 150 tumors (BRCA1, BRCAx and BRCA1 test variant) by pyrosequencing. We validated the expected difference between BRCA1 and BRCAx tumors for 6/12 candidate regions, observing lower median methylation in BRCA1 tumors for five candidate regions [CD9 (OMIM# 143030), SGK1 (OMIM# 602958), ERCC3 (OMIM# 133510), and 2 regions in FGF2 (OMIM# 134920), wilcoxon rank sum test, P<0.05] and significantly higher methylation in BRCA1 tumors for one gene [CD40 (OMIM# 109535), P=0.02] (Table S1). Logistic regression analysis showed that methylation status was significantly associated with BRCA1 mutation status (Table S1). Since ER status is a known predictor of BRCA1 mutation status [local recurrence (LR) ranging from 0.08-0.90 for ER-negative status, dependent on grade],Citation15 we assessed whether differences observed in methylation between BRCA1 and BRCAx tumors in the remaining five candidate regions of interest were independent of these known histopathological predictors. Using a generalized linear model we showed that methylation differences observed in these five regions were associated with ER status (P-value <0.05) (Table S1). ER status and mutation status are highly associated in this dataset [generalized linear model (glm) P-value = 4.31 × 10−7], and none of the five candidates were significantly independent from ER status, indicating that they should not be used in conjunction with existing pathology LRsCitation15 in multifactorial modeling for prediction of variant pathogenicity. In light of these findings, further analyses considering grade, another predictor of mutation status, were not pursued.
Genome-wide analysis identifies novel differentially methylated loci that define BRCA1 mutation status
The overall study design is shown in . The Illumina HumanMetylation 450 (450K) BeadChip array has successfully been used to assess DNA methylation in formalin-fixed, paraffin-embedded (FFPE)-derived DNA samples using appropriate quality control and restoration methods.Citation16 We selected 60 samples [BRCA1 pathogenic variants (n=20), BRCAx (n=20), and BRCA1 test variant (n=20)] for array analysis, of which 54 (90%) samples passed quality control [BRCA1 pathogenic (18/20), BRCAx (19/20), BRCA1 test variant (17/20)]; 482351/485577 (99.3%) probes passed quality control (QC). The Wilcoxon rank sum test was used in three analyses to identify (i) probes with significant differences between tumors from patients with germline BRCA1 mutations and tumors from BRCAx cases [false discovery rate (FDR) q<0.05, n=250 probes]; (ii) probes with significant differences between ER positive tumors and ER negative tumors (FDR q<0.05, n=55148 probes); and (iii) probes with significant differences between low grade (Grades 1 and 2) and high grade (Grade 3) tumors (FDR q<0.05, n=29 probes). Using a Venn diagram we show that 23 probes were apparently unique to the mutation class analysis (). Probes with an absolute methylation difference between groups of less than 5% were excluded, leaving 18 probes with a range of absolute difference in methylation between 5% and 30%. We used consensus clustering of the array data using the 18 selected probes that differentiated BRCA1 pathogenic tumors from the BRCAx tumors to determine with which cluster each of the BRCA1 test variant tumors clustered. This method confirmed only two tumor clusters based on these data () and found nine BRCA1 test set variant tumors clustered with pathogenic BRCA1 mutated tumors, and eight BRCA1 test set variant tumors clustered with BRCAx tumors.()
Figure 1. Study Design. (A) Representation of the exonic position of the known mutations in the BRCA1 gene used in this study, including pathogenic (missense and truncating) and test variants. Some variants are represented by multiple tumors. (B) Flow diagram illustrating study design. Rectangular boxes indicate samples, hexagonal boxes represent experimental processes, and diamond-shaped boxes denote bioinformatics processes or analysis.
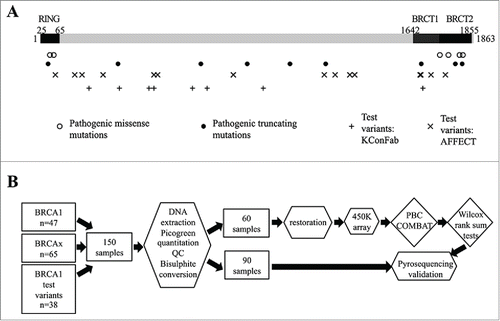
Figure 2. Methylation array (450K) analysis defines pathogenic and neutral variants. (A) Venn diagram representing the comparison of significant probe lists (FDR <0.05), showing 23 probes only significant in the mutation status analysis. (B) Consensus clustering using 18 probes with a difference in methylation between BRCA1 mutated and BRCAx greater than 5% identified two main clusters, as shown by kmeans plot. (C) The resulting correlation matrix from these two clusters shows that these clusters correlate with mutation status. Unclassified variants in green clustered with either the BRCA1 tumors or the BRCAx tumors.
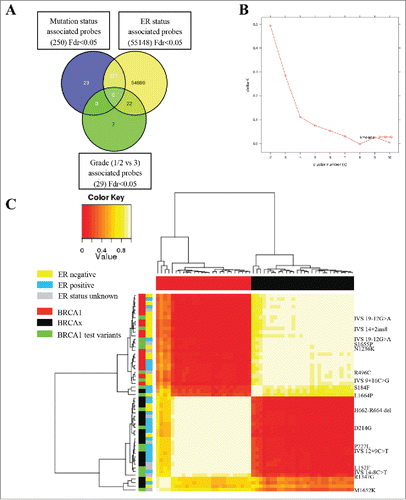
Pyrosequencing assays were designed for these 18 loci, and optimized for 16. Methylation analysis of these regions was conducted for 150 FFPE samples (54 matched to the array, the 90 independent samples, and the 6 that did not pass array QC criteria). Intraclass correlation coefficients (ICC) of assays were calculated to compare the β values obtained from the array to pyrosequencing values for matched samples, and the Wilcoxon rank sum test used to validate the difference between the BRCA1 mutated and BRCAx group in the independent samples (). A significant difference (P<0.05) between BRCA1 samples and BRCAx samples was validated for 5 loci [450K probe IDs cg24667115 (BACH2 OMIM# 605394), cg03029255 (C8orf31), cg02502358 (LYRM9), cg21645762 (LOC654342) and cg12472473 (chr13q34)] in the independent group of samples.
Table 1. Candidate gene validation by pyrosequencing.
However, the cg12472473 (13q34) locus harbored a CG>TG SNP with a minor allele frequency (MAF) of 0.2 within the CpG dinucleotide of interest, and pyrosequencing genotyping showed that the T genotype was overrepresented in the BRCAx group (chi squared test, P<0.05). This region was therefore excluded from further analysis. The technical and biological validation of the four loci is shown in . Further, despite selecting array probes that apparently predicted mutation status independent of ER and grade status (see ), logistic regression analysis of the pyrosequencing data showed that probes cg24667115 (BACH2) and cg02502358 (LYRM9) were not independently associated with mutation status when ER status was also included in the model (). Additionally, there was evidence that methylation status of cg02502358 (LYRM9), cg24667115 (BACH2) and cg21645762 (LOC654342) were correlated with grade, although this analysis was based on approximately half of the individuals, for which we had information on all variables, which reduces the statistical power of the analysis.
Figure 3. Validation of LYRM9, BACH2, LOC654342, and C8orf31 loci. (A) Strip charts show array β values for the four validated loci plotted by mutation status. Blue dotted line is plotted at the median of BRCA1 and BRCAx samples. ER negative samples are colored red, ER positive samples are colored blue, and those samples for which ER status is unknown are colored green [BRCA1 (n=18), BRCAx (n=19), BRCA1 test variant (n=17), except BACH2, where BRCA1 n=17]. (B) Strip charts showing the pyrosequencing methylation value of the independent group of samples validating the difference observed on the array [LYRM9:BRCA1 (n=19), BRCAx (n=29), test variant (UV) (n=11); BACH2:BRCA1 (n=19), BRCAx (n=27), test variant (UV) (n=18); C8orf31: BRCA1 (n=16), BRCAx (n=11), test variant (UV)(n=20). LOC654342:BRCA1 (n=9), BRCAx (n=18), test variant (UV) (n=7)].
![Figure 3. Validation of LYRM9, BACH2, LOC654342, and C8orf31 loci. (A) Strip charts show array β values for the four validated loci plotted by mutation status. Blue dotted line is plotted at the median of BRCA1 and BRCAx samples. ER negative samples are colored red, ER positive samples are colored blue, and those samples for which ER status is unknown are colored green [BRCA1 (n=18), BRCAx (n=19), BRCA1 test variant (n=17), except BACH2, where BRCA1 n=17]. (B) Strip charts showing the pyrosequencing methylation value of the independent group of samples validating the difference observed on the array [LYRM9:BRCA1 (n=19), BRCAx (n=29), test variant (UV) (n=11); BACH2:BRCA1 (n=19), BRCAx (n=27), test variant (UV) (n=18); C8orf31: BRCA1 (n=16), BRCAx (n=11), test variant (UV)(n=20). LOC654342:BRCA1 (n=9), BRCAx (n=18), test variant (UV) (n=7)].](/cms/asset/faf43370-5d63-41b9-a2dd-adf438ca7206/kepi_a_1111504_f0003_oc.gif)
Table 2. Logistic regression analysis of candidate genes.
As for candidate region analysis described above, these findings suggest that the majority of methylation probes that are associated with mutation status are, in fact, not independent of ER or grade. Thus, with the exception of cg03029255 (C8orf31), the markers identified in this study should not be used in conjunction with existing ER and grade LRs in multifactorial likelihood prediction of variant pathogenicity.
Predictive capacity of different methylation markers of mutation status
Pearson's correlation coefficient was calculated for the pyrosequencing data of each locus against the others, and the R2 value for all comparisons was less than 0.2, indicating a low extent of correlation [R2 (BACH2 vs. C8orf31) = 0.0436, R2 (BACH2 vs. LOC654342) = 0.1719, R2 (BACH2 vs. LYRM9) = 0.0207, R2 (C8orf31 vs. LOC654342) = 0.1935, R2 (C8orf31 vs. LYRM9) = 0.0953, R2 (LOC654342 vs. LYRM9) = 0.0356].
The β values at these four loci were converted to z-scores and combined in a logistic regression model. Due to missing data for some of these markers, there were not enough data points to fit a model to more than three markers. LYRM9 was therefore removed from the model. Using the Leave One Out Cross Validation (LOOCV) method based on methylation data of the three remaining markers alone, 32 out of 37 samples are correctly predicted (86%). The pyrosequencing methylation data was also converted to z-scores, and the combined logistic regression model correctly predicted 78 out of 97 [80% (positive predictive value =76%), (negative predictive value =79%)] of samples with known mutation status using only methylation data.
The logistic regression model including methylation of the three validated loci was used to predict all tumor samples from test set variant carriers based on methylation alone, and results were compared to current classification based on other evidence including ER and grade among other variables (). To represent these predictions for all samples based on their methylation, included those of known pathogenicity, likelihood ratios (mLRs) were calculated using the probability values generated by the prediction model (probability of pathogenicity divided by 1 minus the probability of pathogenicity, shown in Tables S2 and S3), and the log of these values was plotted (). This indicates which samples of the same variant were similar in pathogenicity prediction (for example, BRCA1 IVS 19-12 G>A) and those that are more discordant (Arg1347Gly), and also illustrates the spread of methylation prediction for the known variants (BRCA1 and BRCAx extremes of plot).
Figure 4. Combined methylation likelihood ratios. LRs were calculated for each sample using the model including only methylation. The log of the combined LR is plotted here corresponding to each variant assayed.
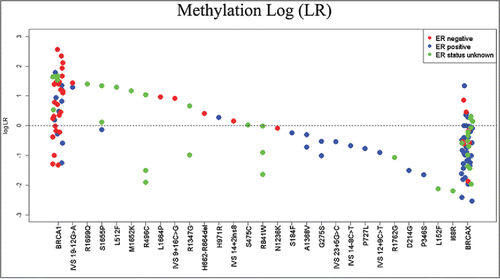
Table 3. Combined summary predictions of all unknown variants analyzed.
Of the 27 unique variants in the test set, one is known to be an intermediate risk allele from extensive segregation studies (Arg1699Gln),Citation17 and 15 have sufficient information available to place them in Class 1, 2, or 4, 5, based on multifactorial likelihood analysis that uses currently accepted predictors of mutation status. Of the latter, one was considered Class 5 (pathogenic), one Class 4 (likely pathogenic), seven were Class 1 (non pathogenic), 6 were Class 2 (likely non pathogenic), and the remainder were Class 3 (uncertain) after classification. Comparing these segregation and multifactorial results to those from methylation analysis alone, the intermediate risk variant Arg1699Gln had an LR of 25.218, the two Class 5 variant IV19-12 G>A samples had LRs of 19.6 and 27.24 in favor of pathogenicity, and the Class 4 variant BRCA1 IVS14+2 ins8 had an LR of 1.43. The Class 1 variants had LRs ranging from 0.008 to 19.75 (with more than 60% of these <1), while the Class 2 variants had LRs ranging from 0.02 to 1.05.
There were 27 unique BRCA1 variants within these 37 individuals, five of which had at least two independent tumor samples from different analyzed individuals, and the logistic regression model resulted in probabilities of pathogenicity for each sample. These probabilities were converted to LRs and combined by multiplication. Prior probabilities for these variants based on bioinformatics analysis (see Materials and Methods) were combined with the likelihood ratio calculated for each variant, and these posterior probabilities are summarized in . Using the established posterior probability cut-offs for the IARC 5 tier classification system,Citation12 BRCA1 IVS 19-12 G>A would be classified as pathogenic (Class 5). Arg496Cys and Arg841Trp would be classified as neutral (Class 1). This would suggest that, at least based on current information, the likelihood ratios derived from the methylation results based on three probes are concordant for the majority of variants with the largest discordance observed being with Class 3 (uncertain) variants. When these likelihood ratios are combined with the bioinformatics prior probabilities, the predictions for Class 1 and 2 variants become far more consistent, but the prediction for BRCA1 IVS14+2 ins8 becomes more uncertain.
Discussion
Multiple studies have identified distinct epigenetic profiles that correlate with different breast cancer subtypes and/or ER status. The most recent example is data from The Cancer Genome Atlas (TCGA) project, which used shared probes between 27K and 450K analysis on 802 tumors to distinguish five groups. Two groups were highlighted: Group 3 showed a hypermethylated phenotype and was enriched for luminal subtype ER positive tumors, while Group 5 showed the lowest levels of methylation in the probes selected and this group overlapped with basal-like subtype ER negative tumors.Citation2 The association between methylation and subtype/ER status is recapitulated by several other studies.Citation14,18-24 which show a lower level of methylation in ER negative/basal-like tumors compared to ER positive/luminal tumors. We previously showed a difference in methylation between familial breast tumors with different BRCA1 germline mutations compared to BRCAx, and found that the methylation clusters formed were independent of the intrinsic subtype, and therefore had the potential to be independent of ER status.Citation13 We hypothesized that this difference in DNA methylation profile could contribute evidence to classify BRCA1 sequence variants of uncertain clinical significance.
We undertook a two-phase study to assess the value of DNA methylation for predicting pathogenicity of BRCA1 variants, the strength of which was the large number of BRCA1 mutant carrier and BRCAx (BRCA1 wild type) tumors used as a reference to generate the predictive algorithm. There is no evidence to suggest that BRCAx tumors have significantly different methylation profiles to sporadic breast cancers.Citation14 The first phase analyzed 12 previously reported candidate regions and validated 5 regions to be strongly associated with mutation status, but also showed that these associations were not independent of ER status or grade. In the second phase, using the Illumina 450K BeadChip array to conduct a genome-wide analysis, we showed that DNA methylation profiles are largely driven by ER status, with 55148 probes significantly associated with ER status. The mechanism by which this occurs is still not understood, and it is unknown whether these changes in methylation occur at ER target genes or as a consequence of ER transcriptional regulation. Very few probes were significantly associated with grade.
Using the Illumina 450K BeadChip array, we also identified 250 loci associated with mutation status, 23 of which initially appeared to be independent of tumor ER status and grade. Of these, 18 had an absolute methylation difference between groups of greater than 5%, and using these 18 novel loci, we were able to cluster the samples into two groups of BRCA1 carrier and BRCAx tumors. The test set samples from variant carriers clustered with the BRCA1 pathogenic or BRCAx subgroups. Of these 18 loci, four sites were validated by pyrosequencing in an independent group of samples, but logistic regression suggested that for all but one probe, the association was not truly independent from ER status and grade in the independent samples. Using the data to predict the mutation status from these methylation values alone, a logistic regression model was created and used to predict the pathogenicity of the 27 different test variants, and these predictions based on methylation data were compared to current evidence regarding pathogenicity using other information sources (summarized in , detailed in Table S4). Combining the predictions from the methylation models with known prior probabilities for each variant, BRCA1 IVS 19-12 G>A would be classified as pathogenic (Class 5), and Arg496Cys and Arg841Trp would be classified as neutral (Class 1). These classifications matched those based on multifactorial likelihood analysis or segregation analysis, so the utilization of this methylation data supports the predictions of these variants.
Further comparison of the current classifications and the methylation derived classifications revealed very few major inconsistencies; a further 8 variants (Ala1368Val, Gly275Ser, Arg1347Gly, His971Arg, BRCA1 IVS 12+9 C>T, Pro346Ser, Pro727Leu, Ser475Cys) were classified by both methods as Class 1 (non pathogenic) or Class 2 (likely non pathogenic). A further three variants (Ser1655Pro, BRCA1 IVS 23+5 G>C, BRCA1 IVS 9+16 C>G) were classified by both methods in the broad uncertain Class 3, indicating for these few variants there is not enough evidence to categorize them confidently by either method. The methylation derived classification correctly predicts the Class 4 variant within this test set, BRCA1 IVS 14+2 ins 8, as Class 4. Due to missing pathology, lack of previous investigation or a combined LR between 0.5 and 2.0 (which therefore does not pass thresholds recommended by the ENIGMA BRCA classification guidelines, see http://www.enigmaconsortium.org/), six variants do not have a current classification based on multifactorial likelihood analysis or segregation analysis but are all classified by the methylation model as Class 1 or Class 2 (Ile68Arg, Leu152Phe, BRCA1 IVS 9+16C>G, Asn1236Lys, Arg1762Gly, Ser475Cys). It is therefore difficult to assess the validity of the methylation predictions for these variants. Finally, two variants with current uncertain classification (Asp214Gly and His662-Arg664del) share methylation characteristics with the less pathogenic variants of Class 1 and 2.
The relationship between loss of BRCA1 function and a direct or indirect mechanism that influences DNA methylation in normal or cancerous breast tissue remains unclear and an area for future research. We observed two samples that exhibited BRCA1 promoter methylation (>10% by pyrosequencing); one BRCAx sample and one test variant sample (BRCA1 IVS 9+16C>G). The BRCAx sample was predicted to be more similar to a pathogenic BRCA1 mutation (probability from methylation model = 0.8778, LR=7.18) (), while the test variant also had a probability of 0.89 and LR of 8.1575 (Table S2), indicating the potential similarity with pathogenic variants; however, the posterior probability for this sample was 0.14 and remained Class 3 (uncertain). Therefore, it remains unclear whether BRCA1 promoter methylation, which may be more heterogeneous in the different tumor cells, influences the DNA methylation profile in the same manner as germline BRCA1 pathogenic mutations.
The BRCA1 variant Arg1699Gln has been shown to be defective in the formation of foci in response to DNA damage, and also has some effect on transcriptional activity; however, this variant was not categorized as high risk, due to the mixture of intermediate and defective phenotypes in the functional assaysCitation25 Another study, using clinical parameters to assess pathogenicity, classified this variant as deleterious,Citation26 and a structural approach also indicated a pathogenic phenotype.Citation27 A recent functional complementation assay.Citation28 provided additional evidence of pathogenicity, based on proliferation and cisplatin response assays, as well as sensitivity to PARP inhibitors. The most extensive genetic study of 68 families showed that this variant was associated with an intermediate risk.Citation17 Our logistic regression model based on DNA methylation indicated that this variant was probably pathogenic, returning a probability of pathogenicity of 0.97998. The methylation data supports increased risk known to be associated with this variant.
One of the limitations of our study is the small number of ER positive tumors with BRCA1 pathogenic mutations, and ER negative tumors without any known mutations (BRCAx). However, this is indicative of an existing bias, which makes it difficult to discover mutation specific changes, when the ER status is so closely related to the methylation levels, and this bias is inherent in most available study data. An interesting question to consider is whether the ER positive BRCA1 tumors are in fact sporadic cases; recent studies, including whole genome massively parallel sequencing analysis of both ER positive and negative BRCA1 mutated tumors, provide evidence that this is not the case.Citation29,30 A further limitation of this study is the assumption, based on previous data,Citation13 that all BRCA1 mutant tumors have similar profiles for different missense or truncating mutations and the underlying hypothesis that aberrant BRCA1 function is the driver of the aberrant methylation profile. Much larger numbers of independent tumors with the same BRCA1 variants would be required to address this limitation. A limitation of using pyrosequencing is that the fragmented DNA from FFPE samples resulted in higher numbers of samples failing QC in each of the assays. Array based methods have restoration procedures that are unsuitable for pyrosequencing, and thus we found between 30-75% of samples variably failed QC for each assay (). Due to the relative rarity of these variants, FFPE blocks are the most available source of material; however, they are subject to degradation in the quality of DNA, as well as lower yields depending on size of tumor and amount of remaining archival tissue. Furthermore, we did not have access to adjacent normal tissue of the same patients, which may have provided further information on whether the genetic variants influence the methylation of normal tissue in addition to the tumor tissue. Lastly, common to many tumor methylation profiling studies, we have not accounted for the numerous environmental exposures and factors that might influence or confound the methylation profile of the tumor, such as age, BMI, alcohol, smoking, and the tumor microenvironment. Future studies should record such data on subjects to allow adjusting for these factors in defining differential methylation.
In conclusion, we have developed a methylation-based prediction tool that adds useful information that may be included in the multifactorial model to classify BRCA1 variants of uncertain clinical significance, but note that most methylation markers identified are not truly independent of ER and grade status. Thus, methylation data should be considered as complementary to other pathology data for multifactorial likelihood prediction modeling and may be useful for classifying variants with tumors where these clinical variables may be absent. Measurement of methylation allows a quantitative approach and, with the appropriate controls, methylation characterization provides promise to reduce inter-laboratory variability in pathological marker calls(such as immunohistochemistry methodsCitation31-33) and improves application of this alternative tumor pathology characteristic for mutation prediction studies in the future. The methylation data measured using pyrosequencing can be incorporated into prediction models easily, and may capture risk information associated with multiple other pathological features. High-throughput analysis of multiple samples is also feasible, and we have shown here that FFPE-derived DNA is amenable to this analysis, allowing the use of archival tissue, essential for the investigation of rare variants. In summary, this work suggests that the methylation markers will have value for future variant classification for BRCA1 and potentially for other genes with known tumor methylation phenotypes, such as MLH1 (OMIM# 120436) in colon cancer (Cancer Genome Atlas Network, 2012b).
Materials and Methods
Samples
A total of 150 breast tumor DNA samples were available for analysis, comprising of three groups: germline BRCA1 mutated tumors [henceforth referred to as BRCA1 (n=47)], germline BRCA1 wild type tumors from women from high risk families [BRCAx (n=65)], and the designated test variant tumors (n=38). Thirty-seven of these samples (14 BRCA1, 23 BRCAx) were previously extracted and described.Citation13 Ninety samples of known BRCA1/2 germline status [BRCAx (n=43), BRCA1 (n=32), BRCA1 test variants (n=15)] were collected by the Kathleen Cunningham Foundation for Research into Breast Cancer (kConFab) consortium. Ethical approval for recruitment was obtained from the institutional review boards or ethic committees of all sites, and written informed consent was given by each participant.Citation34 The tumors designated as BRCAx came from women from high-risk families ascertained by kConFab and, in each case, the tumor donor had undergone germline BRCA1/2 mutation testing by full sequencing of the coding region and splice junctions and multiplex ligation dependent probe amplification (http://www.kconfab.org/). Additional tumors from BRCA1 test variant carriers were collected by the AFFECT study (n=23), for which ethics approval for recruitment was obtained from Brighton East Ethics Committee (REC: 06/Q1907/135) and each participant gave written informed consent. Although test variants were considered unclassified at study initiation, additional information has since allowed class to be assigned to at least some of these (see below). All variants are described using the cDNA nucleotide numbering which uses +1 as the A of the ATG translation initiation codon in the reference sequence, with the initiation codon as codon 1. A representative section of each FFPE tumor sample was stained by hematoxylin and eosin staining and evaluated by a pathologist to verify tumor content (>70% tumor) and histology; between 3 and 9 unstained slides from each tumor were needle-macro dissected, before standard phenol/chloroform DNA extraction and ethanol precipitation. The locations of the exonic sequence variants (pathogenic and test variants) assessed within the BRCA1 gene are represented in (6 additional intronic variations investigated are not shown). Several variants had tumor samples from more than one carrier: seven of the designated test set () and nine of the pathogenic variant set (Table S3). This study was approved by South West London REC4 (REC: 11/LO/0145).
Laboratory analyses
Analysis of candidate regions
Candidate regions were identified previouslyCitation13 and, in addition, using likelihood ratio analysis on publicly available Illumina Goldengate array data for BRCA1 and BRCAx breast cancer tumors.Citation14 All pyrosequencing assays were designed using the PyroMark Assay Design software. A common tag was placed on either the forward or reverse primer (depending on the strand to be sequenced) and a common universal biotinylated primer was used for all reactions in a semi-nested two round PCR assay.Citation35 PCR primers, cycling conditions, sequencing primers, and sequence to analyze are detailed in Table S5. Assays were optimized with fully methylated gDNA (100%) (Zymo Research) compared to unmethylated DNA (0%, whole genome amplified DNA (Genomiphi V2, GE Healthcare). All PCR products were confirmed to be single bands by agarose gel electrophoresis. Assay quality was further assessed by comparing matched FFPE and fresh frozen samples with correlation coefficients ranging from R2=0.65 to 0.83 and agreement between array and pyrosequencing measured using intraclass correlation (Table1). Methylation values were calculated as an average of all CpG sites within each assay, as determined by the Pyro-QCpG software (Qiagen).
Illumina 450K BeadChip genome-wide analysis
Array sample selection was based upon DNA concentration quantified by Picogreen fluorescent nucleic acid stain (Invitrogen), qPCR quality control performance (Illumina FFPE QC kit) and multiplex GAPDH PCR.Citation36 Bisulphite conversion of 500 ng of each sample was performed using the EZ-96 DNA Methylation-Gold™ Kit according to the manufacturer's protocol (Zymo Research, Orange, CA). Samples underwent restoration using the Illumina Infinium HD Restoration protocol, and 4 μl of bisulphite-converted restored DNA was used for hybridization on the Infinium HumanMethylation450 BeadChip, following the Illumina Infinium HD Methylation protocol. Hybridization, scanning, and raw data processing was performed by UCL Genomics (www.genomics.ucl.ac.uk/). The intensities of the images were extracted using the GenomeStudio (v.2011.1) Methylation module (1.9.0) software, which normalizes within-sample data using different internal controls that are present on the HumanMethylation450 BeadChip and internal background probes. The methylation score for each CpG was represented as a β value according to the fluorescent intensity ratio representing any value between 0 (unmethylated) and 1 (completely methylated). Raw microarray data and processed normalized data will be available from Gene Expression Omnibus (GEO) (GSE72277). Post-array sample QC was implemented to check technical aspects of the array (staining, hybridization, target removal, extension, bisulphite conversion, specificity, non-polymorphism, and negative controls) using a threshold of >3SD outside the normal distribution of each probe set across all samples. In total, 54 samples [90%: BRCA1 (n=18), BRCAx (n=19), BRCA1 test variant (n=17)] passed these assessments. Detection P-values were also used to evaluate probe performance, and probes that failed in more than 20% of samples were discarded, leaving 482351/485577 probes (99.3%) available for analysis. Peak-based correction was used to normalize the data between the two probe types,Citation23 and COMBATCitation37 was used to correct for batch effect between chips.
Statistical analysis
Wilcoxon rank sum test was used to determine statistical significance between methylation data (pyrosequencing percentage or 450K array β value) of the BRCA1 and BRCAx group, with FDR adjusted P<0.05 considered significant. A generalized linear model (glm), implemented in R, was used to interrogate the independence of mutation status, ER status, and grade, and to generate a prediction model, which was utilized by the predict command in R for predicting variant pathogenicity. The clustcomp command from the clusterCons R package was used to perform consensus clustering on β value of the 18 probes of all samples on the Illumina 450K BeadChip array. Pearson's correlation coefficient between probes was calculated using the cor function in R, and reported as an R2 value.
A generalized linear model was constructed and used to predict pathogenicity using the “predict” function in R version 2.15.1. The resulting probabilities were converted to likelihood ratios by calculating probability/1-probability. The posterior probability was calculated as follows: the posterior odds was calculated using prior probabilities obtained from sequence bioinformatics (as described below) multiplied by the likelihood ratio of each variant and 1/1-prior probability. This was then converted to a poster probability by dividing this value by itself plus 1.
Assessment of test set variant classifications based on current evidence
After completion of analysis and during manuscript preparation, an extensive literature review and database search was undertaken to identify the most up-to-date information pertinent to variant classification for the entire test set variants. The most up-to-date posterior probability based on multifactorial likelihood analysis was accessed from a public website displaying information collated from the literature (http://brca.iarc.fr/LOVD/home.php). For variants that did not reach Class 1 (non pathogenic or of little clinical significance) or Class 5 (pathogenic), the posterior probability was recalculated using additional information relevant to multifactorial analysis. Methods used were as described previously,Citation38 with two exceptions. First, prior probability of pathogenicity was updated to incorporate possible effects of sequence variation of splicing, based on in silico splicing prediction algorithms adapted for this purpose (Tavtigian, personal communication). Specifically, all exonic sequence variants plus intronic variants detected in the vicinity of the splice junction sequences with allele frequencies <0.5% were scored for their potential impact on splicing using MaxEntScan (MES), which computes the maximum entropy score of a given sequence using splice site models trained on human data.Citation39 MES was calibrated by calculating the average and standard deviation of MES scores for the wild type splice junctions in BRCA1, BRCA2, and ATM, allowing raw MES scores to be converted into z-scores. Based on BRCA1 and BRCA2 mutation screening data used previously to calibrate Align-GVGD,Citation7,9 rare variants that fall within the acceptor or donor region and reduce the MES score for the splice signal in which they fall showed ~97% probability to damage splice junction function when they result in a calibrated MES z-score <-2 (for donors) or <-1.5 (for acceptors), or ~34% probability, when they result in a calibrated MES z-score between -2 and 0 (for donors) or between -1.5 and 0.5 (for acceptors). Additionally, exonic rare variants that increased the MES donor score of their sequence context and resulted in a calibrated MES donor z-score >0 had ~64% probability to create a de novo donor, while if they resulted in a calibrated MES z-score between -2 and 0, the probability to create a de novo donor was ~30% (Spurdle, Goldgar, Parsons, unpublished data). These MES-based rules were used to identify rare sequence variants that are likely to alter mRNA splicing. For exonic variants that resulted in a missense substitution, the higher of the two priors (missense vs. splicing) took precedence for multifactorial likelihood analysis. Secondly, revised pathology LRs were drawn from a recent large-scale age-stratified analysis of 4477 BRCA1 mutation carriers, 2565 BRCA2 mutation carriers, and 47565 breast cancer cases with no known mutation (Spurdle et al., 2015). Additional information included breast tumor ER and grade status extracted from the literature, family history, segregation and co-occurrence likelihood ratios (LRs), assessed from a previously described data set,Citation7 and further segregation and pathology data, compiled for the variants identified through the kConFab and AFFECT studies. Published mRNA assay data were identified from the literature, where this was available. Current class, and rationale for classification, is summarized in Table S4 for all test variants.
Authors' Contributions
Experiments and analysis were performed by KJF. KJF and NSS participated in sample preparation. M-EB performed pathological review of all tumors. ABS, MP, JM, and DEG provided samples and/or participated in data analysis and interpretation. JMF, RB, ABS, and JM conceived the study design and coordination. KJF and JMF drafted the manuscript.
Disclosure of Potential Conflicts of Interest
No potential conflicts of interest were disclosed.
Declarations
Ethical approval references are listed in the methods. All authors read and approved the final manuscript.
Supplementary Material
Supplemental data for this article can be accessed on the publisher website.
Supplemental_.zip
Download Zip (1.1 MB)Acknowledgments
This study was funded by Breast Cancer Now Project 2010NovPR06. JMF and KJF were funded by Breast Cancer Now. ABS is supported by an NHMRC Senior Research Fellowship. MP was supported by funding from NHMRC project grant ID 1010719. The authors acknowledge funding from Cancer Research UK (A13086) and the National Institute for Health Research (NIHR) Biomedical Research Center based at Imperial College Healthcare NHS Trust and Imperial College London. We wish to thank Eveline Niedermayr, all the KConFab research nurses and staff, the heads and staff of the Family Cancer Clinics, and the Clinical Follow up Study (funded by NHMRC grants 145684, 288704 and 454508) for their contributions to this resource, and the many families who contribute to KConFab. KConFab is supported by grants from the National Breast Cancer Foundation, the National Health and Medical Research Council (NHMRC) and by the Queensland Cancer Fund, the Cancer Councils of New South Wales, Victoria, Tasmania and South Australia, and the Cancer Foundation of Western Australia. The AFFECT study is funded by Breast Cancer Now and Guy's and St. Thomas' Charity. AFFECT samples contributed by Louise Izatt (Guy's Hospital, London), Shirley Hodgson (St Georges Hospital, London), Sue Kenwrick (Addenbrooks Hospital, Cambridge) and Fiona Douglas (Freeman Hospital, Newcastle).
References
- Ferlay J, Shin HR, Bray F, Forman D, Mathers C, Parkin DM. Estimates of worldwide burden of cancer in 2008: GLOBOCAN 2008. Int J Cancer 2010; 127:2893–917; PMID:21351269; http://dx.doi.org/10.1002/ijc.25516
- TCGA. Comprehensive molecular portraits of human breast tumours. Nature 2012; 490:61–70; PMID:23000897; http://dx.doi.org/10.1038/nature11412
- Curtis C, Shah SP, Chin SF, Turashvili G, Rueda OM, Dunning MJ, Speed D, Lynch AG, Samarajiwa S, Yuan Y, et al. The genomic and transcriptomic architecture of 2,000 breast tumours reveals novel subgroups. Nature 2012; 486:346–52; PMID:22522925; http://dx.doi:10.1038/nature10983
- Smith P, McGuffog L, Easton DF, Mann GJ, Pupo GM, Newman B, Chenevix-Trench G, Szabo C, Southey M, Renard H, et al. A genome wide linkage search for breast cancer susceptibility genes. Genes Chromosomes Cancer 2006; 45:646–55; PMID:16575876; http://dx.doi.org/10.1002/gcc.20354
- Eggington JM, Bowles KR, Moyes K, Manley S, Esterling L, Sizemore S, Rosenthal E, Theisen A, Saam J, Arnell C, et al. A comprehensive laboratory-based program for classification of variants of uncertain significance in hereditary cancer genes. Clin Genet 2014; 86:229–37; PMID:24304220; http://dx.doi.org/10.1111/cge.12315
- Lindor NM, Goldgar DE, Tavtigian SV, Plon SE, Couch FJ. BRCA1/2 sequence variants of uncertain significance: a primer for providers to assist in discussions and in medical management. Oncologist 2013; 18:518–24; PMID:23615697; http://dx.doi.org/10.1634/theoncologist.2012-0452
- Easton DF, Deffenbaugh AM, Pruss D, Frye C, Wenstrup RJ, Allen-Brady K, Tavtigian SV, Monteiro AN, Iversen ES, Couch FJ, et al. A systematic genetic assessment of 1,433 sequence variants of unknown clinical significance in the BRCA1 and BRCA2 breast cancer-predisposition genes. Am J Hum Genet 2007; 81:873–83; PMID:17924331; http://dx.doi.org/10.1086/521032
- Goldgar DE, Easton DF, Byrnes GB, Spurdle AB, Iversen ES, Greenblatt MS. Genetic evidence and integration of various data sources for classifying uncertain variants into a single model. Hum Mutat 2008; 29:1265–72; PMID:18951437; http://dx.doi.org/10.1002/humu.20897
- Tavtigian SV, Byrnes GB, Goldgar DE, Thomas A. Classification of rare missense substitutions, using risk surfaces, with genetic- and molecular-epidemiology applications. Hum Mutat 2008; 29:1342–54; PMID:18951461; http://dx.doi.org/10.1002/humu.20896
- Spurdle AB, Couch FJ, Hogervorst FB, Radice P, Sinilnikova OM. Prediction and assessment of splicing alterations: implications for clinical testing. Hum Mutat 2008; 29:1304–13; PMID:18951448; http://dx.doi.org/10.1002/humu.20901
- Tavtigian SV, Greenblatt MS, Goldgar DE, Boffetta P. Assessing pathogenicity: overview of results from the IARC Unclassified Genetic Variants Working Group. Hum Mutat 2008; 29:1261–4; PMID:18951436; http://dx.doi.org/10.1002/humu.20903
- Plon SE, Eccles DM, Easton D, Foulkes WD, Genuardi M, Greenblatt MS, Hogervorst FB, Hoogerbrugge N, Spurdle AB, Tavtigian SV. Sequence variant classification and reporting: recommendations for improving the interpretation of cancer susceptibility genetic test results. Hum Mutat 2008; 29:1282–91; PMID:18951446; http://dx.doi.org/10.1002/humu.20880
- Flanagan JM, Cocciardi S, Waddell N, Johnstone CN, Marsh A, Henderson S, Simpson P, da Silva L, Khanna K, Lakhani S, et al. DNA methylome of familial breast cancer identifies distinct profiles defined by mutation status. Am J Hum Genet 2010; 86:420–33; PMID:20206335; http://dx.doi.org/10.1016/j.ajhg.2010.02.008
- Holm K, Hegardt C, Staaf J, Vallon-Christersson J, Jonsson G, Olsson H, Borg A, Ringner M. Molecular subtypes of breast cancer are associated with characteristic DNA methylation patterns. Breast Cancer Res 2010; 12:R36; PMID:20565864; http://dx.doi.org/10.1186/bcr2590
- Spurdle AB, Couch FJ, Parsons MT, McGuffog L, Barrowdale D, Bolla MK, Wang Q, Healey S, Schmutzler RK, Wappenschmidt B, et al. Refined histopathological predictors of BRCA1 and BRCA2 mutation status: a large-scale analysis of breast cancer characteristics from the BCAC, CIMBA, and ENIGMA consortia. Breast Cancer Res 2014; 16:3419: In Press; http://dx.doi.org/10.1186/s13058-014-0474-y
- Thirlwell C, Eymard M, Feber A, Teschendorff A, Pearce K, Lechner M, Widschwendter M, Beck S. Genome-wide DNA methylation analysis of archival formalin-fixed paraffin-embedded tissue using the Illumina Infinium HumanMethylation27 BeadChip. Methods 2010; 52:248–54; PMID:20434562; http://dx.doi.org/10.1016/j.ymeth.2010.04.012
- Spurdle AB, Whiley PJ, Thompson B, Feng B, Healey S, Brown MA, Pettigrew C, Van Asperen CJ, Ausems MG, Kattentidt-Mouravieva AA, et al. BRCA1 R1699Q variant displaying ambiguous functional abrogation confers intermediate breast and ovarian cancer risk. J Med Genet 2012; 49:525–32; PMID:22889855; http://dx.doi.org/10.1136/jmedgenet-2012-101037
- Bediaga NG, Acha-Sagredo A, Guerra I, Viguri A, Albaina C, Ruiz Diaz I, Rezola R, Alberdi MJ, Dopazo J, Montaner D, et al. DNA methylation epigenotypes in breast cancer molecular subtypes. Breast Cancer Res 2010; 12:R77; PMID:20920229; http://dx.doi.org/10.1186/bcr2721
- Van der Auwera I, Yu W, Suo L, Van Neste L, van Dam P, Van Marck EA, Pauwels P, Vermeulen PB, Dirix LY, Van Laere SJ. Array-based DNA methylation profiling for breast cancer subtype discrimination. PLoS One 2010; 5:e12616; PMID:20830311; http://dx.doi.org/10.1371/journal.pone.0012616
- Ronneberg JA, Fleischer T, Solvang HK, Nordgard SH, Edvardsen H, Potapenko I, Nebdal D, Daviaud C, Gut I, Bukholm I, et al. Methylation profiling with a panel of cancer related genes: association with estrogen receptor, TP53 mutation status and expression subtypes in sporadic breast cancer. Mol Oncol 2011; 5:61–76; PMID:21212030; http://dx.doi.org/10.1016/j.molonc.2010.11.004
- Kamalakaran S, Varadan V, Giercksky Russnes HE, Levy D, Kendall J, Janevski A, Riggs M, Banerjee N, Synnestvedt M, Schlichting E, et al. DNA methylation patterns in luminal breast cancers differ from non-luminal subtypes and can identify relapse risk independent of other clinical variables. Mol Oncol 2011; 5:77–92; PMID:21169070; http://dx.doi.org/10.1016/j.molonc.2010.11.002
- Killian JK, Bilke S, Davis S, Walker RL, Jaeger E, Killian MS, Waterfall JJ, Bibikova M, Fan JB, Smith WI, Jr, et al. A methyl-deviator epigenotype of estrogen receptor-positive breast carcinoma is associated with malignant biology. Am J Pathol 2011; 179:55–65; PMID:21641572; http://dx.doi.org/10.1016/j.ajpath.2011.03.022
- Dedeurwaerder S, Desmedt C, Calonne E, Singhal SK, Haibe-Kains B, Defrance M, Michiels S, Volkmar M, Deplus R, Luciani J, et al. DNA methylation profiling reveals a predominant immune component in breast cancers. EMBO Mol Med 2011; 3:726–41; PMID:21910250; http://dx.doi.org/10.1002/emmm.201100801
- Fackler MJ, Umbricht CB, Williams D, Argani P, Cruz LA, Merino VF, Teo WW, Zhang Z, Huang P, Visvananthan K, et al. Genome-wide methylation analysis identifies genes specific to breast cancer hormone receptor status and risk of recurrence. Cancer Res 2011; 71:6195–207; PMID:21825015; http://dx.doi.org/10.1158/0008-5472.CAN-11-1630
- Lovelock PK, Spurdle AB, Mok MT, Farrugia DJ, Lakhani SR, Healey S, Arnold S, Buchanan D, Couch FJ, Henderson BR, et al. Identification of BRCA1 missense substitutions that confer partial functional activity: potential moderate risk variants? Breast Cancer Res 2007; 9:R82; PMID:18036263; http://dx.doi.org/10.1186/bcr1826
- Gomez Garcia EB, Oosterwijk JC, Timmermans M, van Asperen CJ, Hogervorst FB, Hoogerbrugge N, Oldenburg R, Verhoef S, Dommering CJ, Ausems MG, et al. A method to assess the clinical significance of unclassified variants in the BRCA1 and BRCA2 genes based on cancer family history. Breast Cancer Res 2009; 11:R8; PMID:19200354; http://dx.doi.org/10.1186/bcr2223
- Clapperton JA, Manke IA, Lowery DM, Ho T, Haire LF, Yaffe MB, Smerdon SJ. Structure and mechanism of BRCA1 BRCT domain recognition of phosphorylated BACH1 with implications for cancer. Nat Struct Mol Biol 2004; 11:512–8; PMID:15133502; http://dx.doi.org/10.1038/nsmb775
- Bouwman P, van der Gulden H, van der Heijden I, Drost R, Klijn CN, Prasetyanti P, Pieterse M, Wientjens E, Seibler J, Hogervorst FB, et al. A high-throughput functional complementation assay for classification of BRCA1 missense variants. Cancer Discov 2013; 3:1142–55; PMID:23867111; http://dx.doi.org/10.1158/2159-8290.CD-13-0094
- Tung N, Wang Y, Collins LC, Kaplan J, Li H, Gelman R, Comander AH, Gallagher B, Fetten K, Krag K, et al. Estrogen receptor positive breast cancers in BRCA1 mutation carriers: clinical risk factors and pathologic features. Breast Cancer Res 2010; 12:R12; PMID:20149218; http://dx.doi.org/10.1186/bcr2478
- Natrajan R, Mackay A, Lambros MB, Weigelt B, Wilkerson PM, Manie E, Grigoriadis A, A'Hern R, van der Groep P, Kozarewa I, et al. A whole-genome massively parallel sequencing analysis of BRCA1 mutant oestrogen receptor-negative and -positive breast cancers. J Pathol 2012; 227:29–41; PMID:22362584; http://dx.doi.org/10.1002/path.4003
- Oyama T, Ishikawa Y, Hayashi M, Arihiro K, Horiguchi J. The effects of fixation, processing and evaluation criteria on immunohistochemical detection of hormone receptors in breast cancer. Breast Cancer 2007; 14:182–8; PMID:17485904; http://dx.doi.org/10.2325/jbcs.976
- Marinsek ZP, Nolde N, Kardum-Skelin I, Nizzoli R, Onal B, Rezanko T, Tani E, Ostovic KT, Vielh P, Schmitt F, et al. Multinational study of oestrogen and progesterone receptor immunocytochemistry on breast carcinoma fine needle aspirates. Cytopathology 2013; 24:7–20; PMID:23082931; http://dx.doi.org/10.1111/cyt.12024
- Wells CA, Sloane JP, Coleman D, Munt C, Amendoeira I, Apostolikas N, Bellocq JP, Bianchi S, Boecker W, Bussolati G, et al. Consistency of staining and reporting of oestrogen receptor immunocytochemistry within the European Union–an inter-laboratory study. Virchows Arch 2004; 445:119–28; PMID:15221370; http://dx.doi.org/10.1007/s00428-004-1063-8
- Mann GJ, Thorne H, Balleine RL, Butow PN, Clarke CL, Edkins E, Evans GM, Fereday S, Haan E, Gattas M, et al. Analysis of cancer risk and BRCA1 and BRCA2 mutation prevalence in the kConFab familial breast cancer resource. Breast Cancer Res 2006; 8:R12; PMID:16507150; http://dx.doi.org/10.1186/bcr1377
- Royo JL, Hidalgo M, Ruiz A. Pyrosequencing protocol using a universal biotinylated primer for mutation detection and SNP genotyping. Nat Protoc 2007; 2:1734–9; PMID:17641638; http://dx.doi.org/10.1038/nprot.2007.244
- van Beers EH, Joosse SA, Ligtenberg MJ, Fles R, Hogervorst FB, Verhoef S, Nederlof PM. A multiplex PCR predictor for aCGH success of FFPE samples. Br J Cancer 2006; 94:333–7; PMID:16333309; http://dx.doi.org/10.1038/sj.bjc.6602889
- Johnson WE, Li C, Rabinovic A. Adjusting batch effects in microarray expression data using empirical Bayes methods. Biostatistics 2007; 8:118–27; PMID:16632515; http://dx.doi.org/10.1093/biostatistics/kxj037
- Whiley PJ, Parsons MT, Leary J, Tucker K, Warwick L, Dopita B, Thorne H, Lakhani SR, Goldgar DE, Brown MA, et al. Multifactorial likelihood assessment of BRCA1 and BRCA2 missense variants confirms that BRCA1:c.122A>G(p.His41Arg) is a pathogenic mutation. PLoS One 2014; 9:e86836; PMID:24489791; http://dx.doi.org/10.1371/journal.pone.0086836
- Yeo G, Burge CB. Maximum entropy modeling of short sequence motifs with applications to RNA splicing signals. J Comput Biol 2004; 11:377–94; PMID:15285897; http://dx.doi.org/10.1089/1066527041410418