ABSTRACT
Abnormal fatty acid metabolism and availability are landmarks of metabolic diseases, which in turn are associated with aberrant DNA methylation profiles. To understand the role of fatty acids in disease epigenetics, we sought DNA methylation profiles specifically induced by arachidonic (AA) or oleic acid (OA) in cultured cells and compared those with published profiles of normal and diseased tissues. THP-1 monocytes were stimulated with AA or OA and analyzed using Infinium HumanMethylation450 BeadChip (Illumina) and Human Exon 1.0 ST array (Affymetrix). Data were corroborated in mouse embryonic fibroblasts. Comparisons with publicly available data were conducted by standard bioinformatics. AA and OA elicited a complex response marked by a general DNA hypermethylation and hypomethylation in the 1–200 μM range, respectively, with a maximal differential response at the 100 μM dose. The divergent response to AA and OA was prominent within the gene body of target genes, where it correlated positively with transcription. AA-induced DNA methylation profiles were similar to the corresponding profiles described for palmitic acid, atherosclerosis, diabetes, obesity, and autism, but relatively dissimilar from OA-induced profiles. Furthermore, human atherosclerosis grade-associated DNA methylation profiles were significantly enriched in AA-induced profiles. Biochemical evidence pointed to β-oxidation, PPAR-α, and sirtuin 1 as important mediators of AA-induced DNA methylation changes. In conclusion, AA and OA exert distinct effects on the DNA methylome. The observation that AA may contribute to shape the epigenome of important metabolic diseases, supports and expands current diet-based therapeutic and preventive efforts.
Abbreviations
450K array | = | Infinium HumanMethylation450 BeadChip |
5mdC | = | 5-methyl-2′-deoxycytidine |
AA | = | arachidonic acid |
AACpG | = | AA-induced differentially methylated CpG |
CGI | = | CpG island |
CpG | = | 5′-CG-3′ dinucleotide |
DB-CpG | = | CpG scored by Δβ |
EPA | = | eicosapentaenoic acid |
FACpG | = | differentially methylated CpG common to the AACpG, OACpG and PACpG sets |
FDR | = | false discovery rate |
MEF | = | mouse embryonic fibroblasts |
N-CpG | = | number of CpGs normalized by dividing the number of differentially methylated CpGs by the total number of CpGs represented in the 450K array for a given gene |
OA | = | oleic acid |
ACpG | = | OA-induced differentially methylated CpG |
PA | = | palmitic acid |
PACpG | = | PA-induced differentially methylated CpG |
PPAR | = | peroxisome proliferator-activated receptor |
SIRT | = | sirtuin. Official acronyms were used for all genes |
Introduction
An organism's fatty acid (FA) pool reflects both composition of the diet and endogenous synthesis. Furthermore, abnormal FA levels have long been recognized to participate in metabolic diseases such as diabetes.Citation1 Since metabolic diseases are frequently associated with aberrant DNA methylation profiles,Citation2 it is conceivable that FAs are among factors that mediate remodeling of the epigenome in response to dietary composition, the cell's metabolic status and pathogenic signals. In humans, only a handful of studies have investigated a link between DNA methylation and fat intake, focusing on specific genes associated with FA metabolism, inflammation and regulation of circadian rhythms.Citation3-8 Furthermore, a recent genome-wide study comparing peripheral whole blood methylation profiles to both quantity and quality of dietary fat intake in adolescents, found a larger number of methylation changes associated with the latter.Citation9 In that study, DNA methylation profiles associated with polyunsaturated:saturated FA ratio were related to pathways regulated by the peroxisome proliferator-activated receptor α (PPAR-α) and adipogenesis. Conversely, no pathways were identified of DNA methylation profiles associated with the monounsaturated:saturated FA ratio. In addition, numerous mouse model studies have addressed short and long term epigenetic effects of diets containing variable combinations of specific FAs.Citation8,10
Although diet-based studies are important as they recognize the complexity of the organism's response to nutrients, mechanistic insights can be gained by a simplified complementary approach based on the manipulation of individual FA levels. Accordingly, the effects of short FAs, such as butyrate, on DNA methylation have been long recognized.Citation11 In addition, very low-density lipoprotein (VLDL) elicited a global DNA hypermethylation response that is markedly stronger than the one induced by low- or high-density lipoprotein in cultured human THP-1 macrophages.Citation12,13 The fact that VLDL is characteristically rich in triglycerides suggests that FAs might be mediators of the epigenetic responses to VLDL. Accordingly, arachidonic acid (AA) induces DNA hypomethylation in human umbilical vein endothelial cells at 3 μM concentration, globally and of angiogenesis regulator kinase insert domain receptor gene promoter.Citation14,15 Another study demonstrated that 100 μM eicosapentaenoic acid (EPA) directly modifies the methylation status of CCAAT / enhancer-binding protein delta gene.Citation16 As for cellular disease models, palmitic acid (PA) was shown to induce global DNA hypermethylation in primary human myocytes and ex vivo human pancreatic islet cells at a 500 μM and 1 mM dose, respectively, affecting targets such as the PPAR-γ co-activator 1A gene.Citation17,18 Furthermore, a recent study in a cellular model of hepatic cancer has shown that a mixture of oleic acid (OA) and PA elicits hypermethylation of selected imprinted gene promoters.Citation19 Possible mechanisms of epigenetic regulation by FAs include binding to PPARs, a family of transcription factors that regulate numerous metabolic processes via ligand-dependent transcriptional activation and repression.Citation20,21
Currently, it is unknown whether the above-described epigenetic effects are FA-specific, as is the contribution of FAs to disease-related methylation profiles. To understand those issues, we focused on the 2 long-chain unsaturated FAs, OA and AA, which are known to exert generally opposite cellular inflammatory responses.Citation22-24 We studied the effects of these particular FAs on the epigenome and transcriptome of THP-1 cells, a widely accepted human monocyte model,Citation25 and compared our results to available DNA methylation data of several human diseases and normal tissues. The implications of our results are discussed in the context of current knowledge of epigenetic regulation by lipid components and dietary factors, and their contribution to disease risk.
Results
Effects of the pure AA and OA on global DNA methylation in cultured cells
We first examined the effects of the pure FAs, AA, and OA, on global DNA methylation, i.e., total normalized 5mdC content in cultured human THP-1 monocytes. Stimulation experiments were carried out for 24 h using FAs in the 0–200 μM concentration range. These concentrations are below or within the reported circulating FA range—see, for example, Higashiyama et al.Citation26 The rationale for using a 24-hour stimulation is that epigenetic responses to lipoproteins were observed in THP-1 macrophages, a differentiated version of THP-1 monocytes.Citation12,13 In accordance with a similar study of AA-stimulated THP-1 cells, where cell proliferation was scored based on 3H-thymidine incorporation,Citation27 FAs did not affect cell proliferation as assessed by cell counting. Overall, AA and OA elicited distinct responses. AA induced a dose-dependent DNA hypermethylation peaking at the 100 μM dose and amounting to a ∼10.5% increase in 5mdC content at 100 μM relative to the 1 μM dose (). In turn, OA induced a weaker response, with an overall DNA hypomethylation at 100 μM relative to the 1 μM dose. Noticeably, the effect of OA was significantly different from the one of the vehicle BSA only at doses >100 μM. Neither OA nor BSA elicited statistically significant responses relative to unstimulated cells or cells stimulated with the 1 μM of any FA, up to the 50 μM dose. To validate the divergent AA and OA dose responses, a 24-hour co-stimulation experiment, in which one FA was held constant at 100 μM concentration while the other varied between 1–100 μM, was performed. The results confirmed the distinct DNA methylation responses to AA and OA in THP-1 cells [; note that the respective responses at 100 μM were not different (P = 0.08)]. Importantly, the observed AA- and OA-induced DNA methylation changes were not specific for THP-1 monocytes, as human embryonic kidney 293 cells also displayed distinct responses to these FAs following a 24-hour stimulation (Supplementary Fig. 1). These experiments were exhaustively repeated and their results were consistent across time (2004–2013), cell culture laboratories, and THP-1 cell stocks (Sweden, Mexico, and Spain for either), HPLC platforms (Mexico and Spain), and total 5mdC assays (HPLC-based or the ELISA-based MethylFlash system).
Figure 1. Effects of pure FAs on global DNA methylation in THP-1 monocytes. A, FA dose-response following a 24-hour stimulation. B, co-stimulation with AA and OA, in which each FA was held constant at the 100 μM dose (symbols in graph A) and the other varied between 1–100 μM (indicated as “variable FA” in the horizontal axis legend). Data in A were obtained by the HPLC-based 5mdC quantitation, data in B by the MethylFlash assay (see text for details). Data points represent averages and SD values. The horizontal dashed line indicates the value corresponding to unchallenged (control) cells. The latter value differs in panels A and B because of the different assays used. C, ALU methylation levels. Symbols are as in A. In all cases, asterisks indicate the significance of the difference in comparison with the respective 1 μM dose. Horizontal brackets in A indicate the AA or OA doses at which the response is significantly different from the response to the vehicle BSA. *, P<0 .05; **, P<0 0.01 (Scheffé post hoc test).
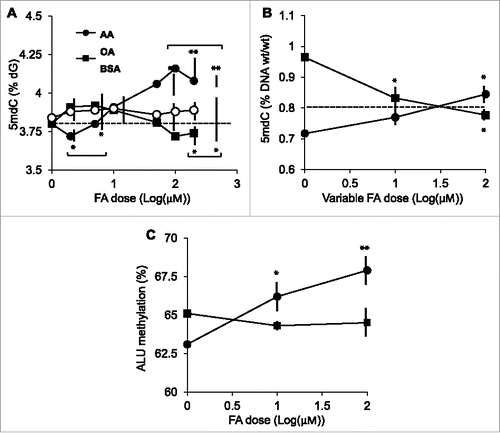
As an independent validation of the total 5mdC data, we performed an ALU-specific methylation assay. ALUs are an abundant transposon family and have often been used as a surrogate of total 5mdC determination.Citation28 In accordance with total 5mdC data, AA induced a significant dose-dependent increase in ALU methylation while OA showed a tendency to hypomethylation, although not statistically significant in the 1–100 μM range ().
Effects of AA and OA on specific CpG site methylation
To detail genomic regions undergoing FA dose-dependent differential DNA methylation at the CpG level resolution, we analyzed THP-1 monocytes stimulated with 1, 10, or 100 μM AA or OA, using the Infinium HumanMethylation450 BeadChip arrays (450K array). The platform represents >485,000 intergenic and intragenic, non-repeated element CpGs.Citation29,30 The rationale for using 100 μM as maximal dose was that it yielded the most divergent effects between the 2 FAs in the total 5mdC assay.
Unsupervised clustering analysis of 450K array data showed that the FA-induced methylation profiles were more divergent at 100 μM FA dose compared to the relatively similar 1 and 10 μM-dose profiles (), mirroring global 5mdC data (compare with ). Next, we sought evidence for an AA dose-dependent DNA hypermethylation response and an opposite one for OA in 450K array data. We restricted our analysis to cells challenged with increasing—1, 10 and 100 μM—FA doses, as the unstimulated cell data reflect a non-physiological FA-free situation. A further reason for not including BSA-stimulated cells in the 450K array analysis, was that global 5mdC data indicated that the global trend of OA-induced DNA hypomethylation was statistically different from the corresponding BSA data set. Before conducting a detailed analysis of the 450K array data, we validated the methylation profiles of 11 CpGs corresponding to 6 genes by pyrosequencing. Genes were randomly selected among CpGs with absolute Δβ>0.1 between 1–100 μM AA. In all cases, pyrosequencing reproduced the DNA methylation profile of the 7 CpGs represented on the 450K array and additional flanking 4 CpGs (Supplementary Fig. 2). Subsequently, we sought autosomal CpGs that showed dose-dependent DNA methylation profiles, i.e., CpGs with statistically significant FA dose-dependent DNA methylation changes. We initially set a Δβ>0.2 between the extreme 1 and 100 μM FA doses as a stringent criterion to identify FA dose-dependent profiles. Such a filtering yielded 283 and 135 CpGs for AA and OA, respectively, a figure that is inconsistent with the global 5mdC data shown above. We therefore reasoned that FAs imposed small and widespread CpG methylation changes. Consequently, CpGs displaying an absolute Δβ>0.005 between consecutive FA doses (i.e., with absolute Δβ>0.01 between the extreme 1 and 100 μM FA doses) were considered for further analysis. We first separated the CpG sets for each FA into 2 subsets that displayed a positive or negative Δβ between extreme FA doses, and performed a Spearman's correlation test separately on the CpGs subsets. This allowed us to perform a one-tailed test using an absolute r>0.985 threshold value corresponding to P<0.05, for each subset. Those CpGs will be referred to as AACpGs or OACpGs. The analysis identified 57,187 AACpGs and 48,917 OACpGs, corresponding to 14,483 and 13,914 genes, respectively (a complete list of AACpGs and OACpGs can be provided upon request). The absolute Δβs between 100 and 1 μM FA doses ranged between 0.29–0.01 for the 2 sets compounded. None of the AACpGs or OACpGs reached the genome-wide significance threshold of P<10−7. A minority of AACpGs (6,432 or 11.2%) overlapped with OACpGs, corresponding to 3,722 genes.
Biological significance of AACpGs and OACpGs
Although the AA- and OA-induced differential methylation did not reach genome-wide significance, a substantial number of converging findings support a pathobiological significance for AACpGs and OACpGs.
First, in agreement with global DNA methylation data, a general tendency for AA-induced hypermethylation and OA-induced hypomethylation was observed, i.e., the majority of AACpGs (76.4%) and OACpGs (74.7%) showed dose-dependent hyper- and hypo-methylation, respectively (P = 6.7×10−32, Chi-square test; ). Likewise, the compounded weight of hypermethylated AACpGs was higher than the hypomethylated counterparts: the sum of Δβs between 100 and 1 μM AA was 1,661.3 and −408.7 for the 2 sets, respectively. Conversely, the net effect of OACpGs was hypomethylation, since the sum of Δβs between 100 and 1 μM OA was 381.6 and −1,308.7 for the hyper- and hypo-methylated OACpG set, respectively. This overall divergent DNA methylation profile included the 6,432 common CpGs between the AACpG and OACpG sets, as the M values between 100 and 1 μM FA doses showed a negative correlation between the 2 sets (r = −0.14, P<10−6).
Figure 3. - Distribution of hypermethylated and hypomethylated AACpGs and OACpGs. The AACpG and OACpG sets are ordered for increased Δβ between the 2 extreme FA doses. The horizontal dashed lines indicate the overall average Δβ for each CpG set.
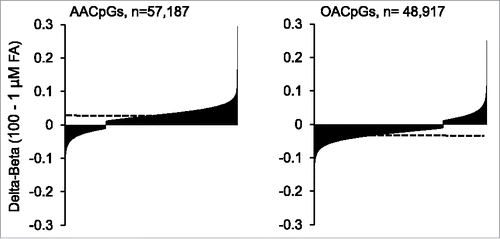
Second, at the gene level, most AACpGs and OACpGs co-localized to gene bodies and promoters and, at the same time, were remarkably divergent for the direction of DNA methylation change. For all overlapping genes, AACpG and OACpGs showed hyper- and hypo-methylation, respectively, between extreme FA doses (P<10−5 in all cases). This tendency was particularly evident of genes that harbored >50 AACpG or AOCpG/gene, which included protein tyrosine phosphatase, receptor type, N polypeptide 2 (PTPRN2), mitotic arrest deficient-like 1 (MAD1L1), PR domain containing 16 (PRDM16), tenascin-XB (TNXB), regulatory-associated protein of mTOR (RPTOR), inositol-1,4,5-trisphosphate 5-phosphatase (INPP5A), adenosine deaminase, RNA-specific, B2 (ADARB2), sidekick cell adhesion molecule 1 (SDK1), ATPase, class VI, type 11A (ATP11A), tubulin folding cofactor D (TBCD), and disco-interacting protein homolog 2 (DIP2C). Furthermore, such divergence in FA-specific methylation profiles was also observed of at least 2 large intergenic regions spanning 605.7 kb and 758.0 kb in size on chromosome 4 and 8, respectively. shows illustrative examples of reciprocal AA- or OA-induced methylation profiles of three genes that showed more >50 AACpG or AOCpG/gene and of the intergenic region on chromosome 8. All, or only overlapping AACpGs and OACpGs, are shown (left and right panels, respectively).
Figure 4. Examples of locus-specific methylation profiles of AACpGs and OACpGs. Graphs on the left show the changes in DNA methylation of AACpGs (solid circles) and OACpGs (open squares). P indicates the position and number of CpGs mapping to promoters. Graphs on the right show the Δβ values of common CpGs to the AACpG and OACpG sets in each gene or intergenic region. P-values refer to unpaired (left) and paired (right) non-parametric tests (Mann-Whitney U test and Wilcoxon test, respectively).
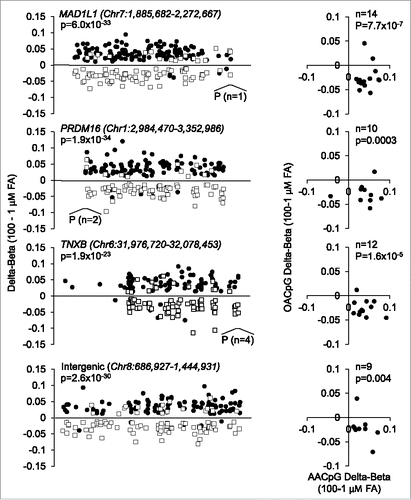
Third, functional annotation analysis using the top 150 genes ranked by number of differentially methylated AACpG and OACpGs, normalized by the number of probes present in the 450K array for a given gene (N-CpGs), revealed significant enrichments for the G protein-coupled receptor (GPCR) and olfactory signaling pathways (REACT_14797 and hsa04740, respectively; Supplementary Table 1). Conversely, no signaling pathways were identified for the top 150 genes ranked by Δβ (DB-CpGs) (Supplementary Table 1). Remarkably, functional gene analysis of differentially expressed genes between 100 and 1 μM AA and OA doses revealed a significant enrichment of the same GPCR signaling pathway (FDR < 10−4 in both cases; see paragraph below “FA-induced CpG methylation and gene expression”). Notably, the average absolute Δβ value of the top 150 N-AACpG and N-OACpGs were ∼4-fold smaller relative to DB-AACpG and DB-OACpGs (0.042 and 0.044 vs. 0.174 and 0.161 on average, respectively; Supplementary Table 2), again pointing to small but biologically relevant effects.
Fourth, we compared AACpG or OACpG to a study addressing the effects of PA on the DNA methylome of primary human pancreatic islets, a model of pro-inflammatory free FA-induced diabetes.Citation18 Notably, reminiscent of the effects of AA in THP-1 monocytes, PA induces a net DNA hypermethylation relative to pancreatic islets stimulated with FA-free BSA alone. In a first instance, we asked whether any differentially methylated CpGs were shared by the 2 studies, i.e., the dose-dependent AACpGs and OACpGs, and PA-induced differentially methylated CpGs (PACpGs). Remarkably, despite significant experimental design differences between the PA-stimulated pancreatic islet model and AA- or OA-stimulated THP-1 cells, including FA concentration (1 mM and 1–100 μM range), incubation time (48 and 24 hours) and cell type (pancreatic islets and THP-1 monocytes), a 10–12% overlap between CpGs was observed. A total of 445 CpGs corresponding to 430 genes, were common to the AACpG, OACpG and PACpG datasets (referred to as FACpGs; Supplementary Table 3). A functional annotation analysis revealed a significant enrichment for regulation by a number of transcription factors, including the biologically plausible CCAAT/enhancer binding protein (C/EBP), α (CEBPA), a critical activator of adipocyte-specific genes (FDR = 8.2×10−7) and PPAR-α (FDR = 0.03), but not the related PPAR-γ (FDR = 0.33).Citation31,32 Furthermore, we found that the M-values between AACpGs and PACpGs were positively correlated (r = 0.21, P = 1.20×10−5) and the majority (72.8%) were hypermethylated in both sets. Conversely, no correlation between the OACpGs and PACpGs was observed (r = 0.08, P = 0.10) and the majority (70.3%) of FACpGs were hypo- and hyper-methylated in the 2 sets, respectively. Furthermore, akin to AA and OACpGs, functional analysis of the top 150 normalized (number of differentially methylated CpG divided by the number of probes in the 450K array) PACpGs revealed enrichment in G-protein and olfactory signal transduction pathways (Supplementary Table 1). These data suggested a functional divergence between AA and PA on the one hand, and OA on the other hand with respect to DNA methylation.
The analogies between FA-stimulated THP-1 cells and a model of pancreatic inflammation raised the question whether similar coincidences exist within a range of human diseases. To address that issue, we compared AACpGs and OACpGs to the 450K array-based human DNA methylation profiling data sets that were available to date. These datasets covered a wide range of disease typology and conditions, i.e., metabolic diseases (atherosclerosis, obesity, type 2 diabetes),Citation33-35 psychiatric disorders (autism—Brodmann areas 10 and 24 profiling—and schizophrenia),Citation36,37 cancer (leukemia, colorectal carcinoma, hepatocellular carcinoma, blood from breast cancer patients),Citation38-41 and agingCitation42 (Supplementary Table 4). The inclusion of the mentioned studies is justified by the general relevance of FAs as suppliers of energy and biologically active metabolites, and their recognized involvement in psychiatric disorders (reviewed inCitation43). In addition, the comparative analysis included 450K array-based DNA methylation profiles of 8 non-diseased tissues.Citation44 The portion of differentially methylated CpGs identified in each study, that overlapped AACpG or OACpGs ranged between 12–17%. Furthermore, despite differences in Δβ cut-off between arrays (Supplementary Table 4) we observed no significant correlation between the extent of overlap and Δβ cut-off values. Excluding the breast cancer blood data set, which did not represent the disease target tissue, we observed a notably lower overlap in cancer compared to metabolic, psychiatric and normal tissue sets (P<0 0.01 in all comparisons, Scheffé post-hoc test; aging was not included in the analysis given that it represented a single study). Since we found no correlation between extent of overlap and number of differentially methylated CpGs yielded by each study (r = −0.12, n = 20, P = 0.62), our data suggested a non-random overlap despite the relatively high number of AACpGs and OACpGs. Gene function analysis for the top 150 N-CpG-harboring genes revealed a common significant enrichment in the GPCR signaling pathway among the cancer and autism (BA24) profiles (FDR<10−2 in all cases), but not in normal tissue samples, suggesting an underlying biological specificity (in italics in Supplementary Table 1).
Next, we compared the methylation state of AACpGs and OACpGs to that of differentially methylated CpGs identified in diseased or normal tissue. Clustering analysis of CpG methylation levels (Δβ) averaged by gene, clearly showed that the AA- and PA-induced profiles were more similar to each other than to OA-induced profiles. Furthermore, the former clustered with metabolic disease, aging and autism profiles, whereas cancer, schizophrenia and normal tissue-specific profiles grouped away from FAs (). These patterns were readily observed in the clustering analysis of the top 50 genes, ranked by the number of overlapping CpGs (). For example, the majority of genes were consistently hypermethylated following stimulation with AA or PA but hypomethylated following stimulation with OA.
Figure 5. Clustering analysis of CpGs that are common to AACpG or OACpG or both, and to the indicated disease and normal tissue DNA methylation profiles. A, Overall data clustering. B, Top 50 genes ranked by the number of harbored CpGs included in the clustering analysis. Asterisks indicate the top 11 genes ranked by the number of AACpG/gene. Red and blue, hypermethylation and hypomethylation, respectively. The tissue origin or relation is indicated for non-normal tissue samples as follows: Ad, adipose tissue; Ao, aorta; Bl, blood; Br, brain; In, intestine; Li, liver; Pa, pancreas.
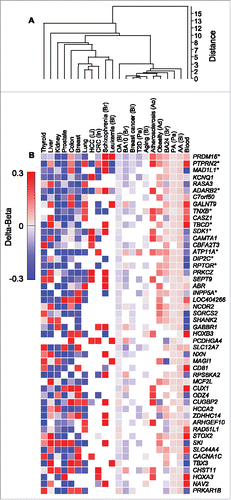
Fifth, we asked whether AACpGs significantly overlap with a set of 450K array methylation profiles obtained in human aortic atherosclerotic lesions of varying histological severity.Citation45 That study identified 1,985 autosomal CpGs, which significantly change their methylation status with lesion progression (grade-CpGs), mostly by drifting toward hypermethylation as lesion grade increases. Grade-CpGs were significantly enriched in AACpGs, as 510 CpGs overlapped between the 2 sets and 324 showed the same methylation trend, mostly (311 out of 324) toward hypermethylation both in response to increasing AA doses and with lesion progression (P = 0.011 for enrichment, hypergeometric test). A list of the 324 overlapping and concordant CpGs between the 2 sets is shown in Supplementary Table 5.
Sixth, AA- and OA-induced DNA methylation profiles showed a degree of specificity for sequences with specific epigenetic and functional signatures. As for preference for hypo- or hyper-methylated sequences, we analyzed the distribution of the AACpG and OACpG across β-values. We observed a clear enrichment of the initially hypermethylated group, i.e., at 1 μM FA dose for both AACpGs and OACpGs, that was independent of the direction of methylation change (Supplementary Figure 3). As for gene compartments and GC content, hypermethylated AACpGs preferentially mapped to sites within open sea (i.e., >4 kb from the nearest CpG island), whereas hypomethylated AACpGs mapped preferentially to CpG islands (P = 0.031 and P = 0.009, respectively, compared to the 450K array probe distribution; Supplementary Figure 4). No other CpG set showed any significant mapping preference.
Overall, these findings support a broad biological significance and instructive mechanism(s), rather than random seeding, for the overall comparatively small AA- and OA-induced DNA methylation profiles.
FA-induced CpG methylation and gene expression
To assess the impact of dose-dependent changes in DNA methylation on gene expression, RNA was extracted from the same cells used to produce 450K array data and analyzed using the Affymetrix Human Exon 1.0 ST platform. To probe for an association between expression and DNA methylation, we focused on AACpGs and OACPGs that colocalized to either exonic or promoter regions. For promoter and exonic CpGs, we correlated dose-dependent changes in DNA methylation with average expression values of all exons or the corresponding exon probe, respectively. While DNA methylation changes in any genomic context showed both positive and negative associations with transcription, context-dependent correlations were uncovered; in particular, a negative correlation in the promoter, 5′UTR and first exon and a positive correlation within gene body and 3′UTR regions. Importantly, average exonic Δβ values between the 100 and 1 μM FA dose were higher of AACpGs compared to OACpGs (0.023 and −0.021, respectively). Since this mirrored the overall tendency of all AACpG and OACpGs, we concluded that gene body methylation was more frequently associated with an increase, rather than a decrease, in transcription. RT-PCR analysis of genes that showed a high number of opposite dose-dependent changes in DNA methylation following stimulation with AA and OA, also supported a tendency for a positive association between gene body methylation and expression for some of the genes analyzed (,C). Furthermore, functional gene analysis revealed a significant enrichment in GPCR signaling (FDR<10−4 in both cases), mirroring the functional enrichment observed in FA-induced methylation profiles (see above).
Figure 6. Effects of dose-dependent changes in DNA methylation on gene expression. A, Correlation between 100–1 μM AA or OA Δβ and expression array-based corresponding expression levels. B, RT-PCR analysis of selected genes following AA and AO stimulation of THP-1 cells for 24 h at 1 and 100 μM concentration. C, GAPDH RNA-normalized expression. The bars for each gene indicate, left to right: 1 μM AA, 100 μM AA, 1 μM OA, 100 μM OA, respectively.
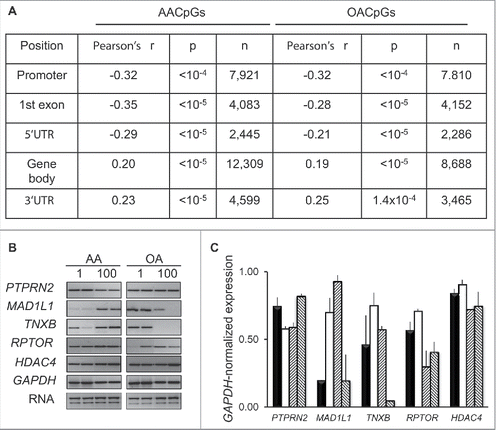
Factors mediating FA-induced changes in DNA methylation
To explore potential mechanisms underlying AA- and OA-induced changes in DNA methylation, we focused on PPAR-α and PPAR-γ, given that both are expressed in THP-1 cells and that FAs, or derivatives thereof, are known ligands of these nuclear receptors.Citation46,31 First, because the above presented functional annotation data suggested a potential role for PPAR-α, but not PPAR-γ in regulating FACpG methylation and, second, due to the link between G-protein cannabinoid receptor signaling pathway and PPAR-α transcription pathway,Citation47,48 we examined the effects of the PPAR-α and PPAR-γ antagonists GW6471 and GW9662, respectively, on the FA-induced methylation responses. GW6471, significantly and substantially, inhibited global DNA hypermethylation induced by 100 μM ΑΑ ιν a doσϵ-dependent fashion, while no effect was observed in cells stimulated with OA (). As for GW9662, neither AA- nor OA-induced methylation responses were significantly affected. Carnitine palmitoyltransferase-1 (CPT1), an enzyme that regulates the import of long-chain FA transport into the mitochondria, is a known target of PPAR-α. Akin to the PPAR-α antagonist, etomoxir, an inhibitor of CPT1, significantly inhibited global DNA hypermethylation induced by 100 μM ΑΑ ιν a doσϵ-dependent fashion (), while no significant effect of cells stimulated with OA was observed ().
Figure 7. Participation of PPARs and β-oxidation in AA- or OA-induced global DNA methylation changes. THP-1 monocytes were stimulated with 100 μM FA for 24 h following a 1 h pre-stimulation with inhibitors of β-oxidation (etomoxir), PPAR-α (GW6471) or PPAR-γ (GW9662). Data are represented as difference in DNA methylation between AA and AO-stimulated and unstimulated cells. The corresponding inhibitor concentration is indicated (μM). Statistical significance is in comparisons with cells grown in the absence of any inhibitor (C). The horizontal dashed line indicates the baseline (unstimulated cells) level. For statistics symbols and test, see legend of .
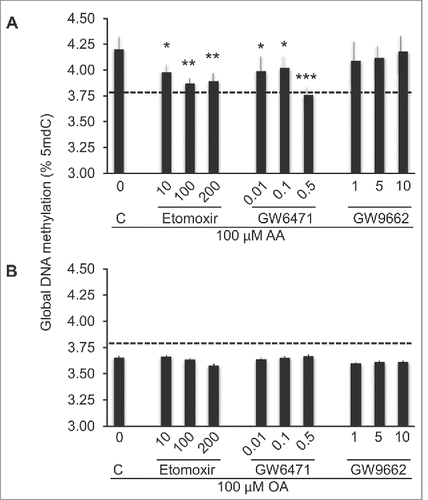
Numerous data lend support to complex interactions between PPARs and sirtuins, the latter of which also regulate transcriptional networks of critical metabolic processes via their NAD+ dependent histone deacetylase activity.Citation49 To understand whether sirtuins played a role in the FA methylation responses, mouse embryonic fibroblasts (MEFs) obtained from SIRT1-, SIRT2-, or SIRT6-null mice and their respective matched WT controls, were stimulated with 100 μM AA or OA for 24 hours. These particular sirtuins were chosen due to their facultative or preferential nuclear location and prior evidence for their regulatory roles in FA oxidation (reviewed inCitation49). Importantly, both AA and OA elicited methylation responses in WT MEFs, which were comparable to those observed in human THP-1 cells. However, among the analyzed mutant MEFs, AA-, and OA-specific DNA methylation responses were only significantly inhibited in SIRT1-null cells (). As further validation of SIRT1 participation in AA-induced hypermethylation, we examined the effects of SIRT1 inhibitors, sirtinol and splitomycin, in THP-1 monocytes. Both inhibitors led to reduced levels of DNA methylation in cells stimulated with 100 μM AA, while TSA, a general histone deacetylase inhibitor with a preference for non-NAD+ dependent histone deacetylases, failed to elicit any effects ().
Figure 8. Participation of histone deacetylases in AA- or OA-induced global DNA methylation changes. A, DNA methylation response in MEFs genetically null for SIRT1, −2, or −6 (indicated as “null”) and matched WT controls, stimulated with 100 μM AA or OA for 24 h, or unstimulated (U). Statistical significance is in comparison with corresponding WT cells. B, Effects of inhibitors of SIRT1 (sirtinol, splitomycin) and broad range histone deacetylases (TSA) on the 100 μM AA-induced response. For statistics symbols and test, see legend of .
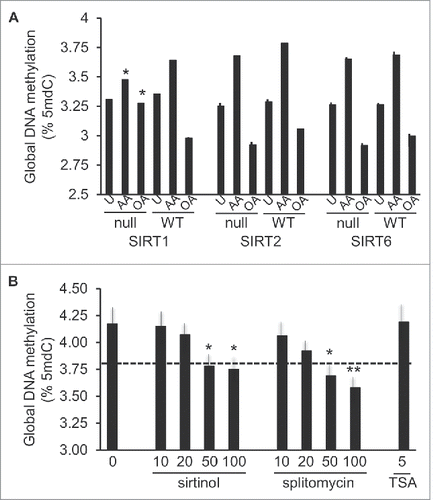
Discussion
We have uncovered distinct DNA methylation profiles induced by AA and OA, 2 unsaturated FAs generally known for their pro-inflammatory and anti-inflammatory properties, respectively. Our global, ALU-specific and individual CpG methylation data obtained in various laboratories across a long period of time (see Results), consistently show divergent effects of the 2 FAs in the analyzed dose range, i.e., AA and OA induce DNA hypermethylation and hypomethylation, respectively, both in human and mouse cultured cells. A comparative analysis of publicly available human DNA methylome data, further confirm the biological relevance of these findings, despite the different criteria used to identify relevant CpG loci. The pro-inflammatory FAs AA and PA induce markedly similar DNA methylation profiles, compared to the corresponding OA-induced profiles. Yet, FA-induced methylation and expression profiles are both enriched in the GPCR signaling function, suggesting antagonistic effects on common cellular pathways. Those similarities are further supported when comparing FA-induced profiles with disease- and normal tissue-specific corresponding data. AA and PA cluster together with metabolic diseases (atherosclerosis, obesity, type 2 diabetes), aging, and autism. OA, on the other hand, clusters more weakly with the latter conditions. This clustering therefore closely reflects the known distinct pathobiological properties of AA and PA on the one side, and OA in the other. Furthermore, several of the identified target genes are relevant from a nutritional and evolutionary perspective. For example, a 12-week supplementation with AA and DHA in baboons is associated with downregulation of PTPRN2 in the cerebral cortex.Citation50 Likewise, an association between DNA methylation, a sequence variant of PTPRN2 and islet insulin secretion has also recently been identified.Citation51 In turn, TNXB is one of the two genes showing population-specific polymorphisms associated with dietary adaption to fat, milk, and meat.Citation52 These associations, although independent and converging, are not definite proof of causality. Also, the effects of FAs presented here are quantitatively modest, reflecting the 10% or less change in DNA methylation profiles that was detected in response to FA, high-fat diet and exercise.Citation18,53,54 Despite these limitations, the data provide testable hypotheses to better understand the regulation of the DNA methylome by FAs and possibly to improve diet-based strategies to improve human health.
These comparisons offer a number of insights into the pathophysiological relevance of the epigenetic responses to FAs. In the case of human atherosclerosis, we show concordance with 2 previously published studies. First, the histological grade-associated CpGsCitation45 are significantly enriched in AACpGs. Second, 16 of the most represented genes across the analyzed DNA methylation profiling studies, are densely hypermethylated in the early stages of the aortic atherosclerosis and display a direction of DNA methylation change that is concordant with the one of AA and PA.Citation33 In both atherosclerosis and AA-stimulated cells, hypermethylation is prominent within the body of these genes. Taken together, these comparative data suggest that a portion of the DNA hypermethylation that characterizes atherosclerosis in its initial phases and during the progression of the stable vascular lesion,Citation33,45 may be seeded by circulating pro-inflammatory FAs or their metabolites. Coupled with evidence that hypomethylating agents can slow the progression of the vascular lesion,Citation55 our observations may lead to testable nutritional strategies to reprogram or safeguard the vascular epigenome. As for autism, the involvement of AA in that condition has been established by early prospective studies documenting the effects of AA-depleted infant formulas on autism risk and by a recent comparative study of red blood cell AA content between autistic patients and controls.Citation56,57 Another finding of our study is the divergence of type 2 diabetes DNA methylation profiles from the ones of AA and, notably, PA. The hypermethylated loci identified in both AA- and PA-stimulated cells show a distant, if any, resemblance to the corresponding profiles in diabetes, thus revealing a set of CpGs that do not model the involvement of free pro-inflammatory FAs in diabetes. Additionally, we show that FA-induced profiles are relatively distant from normal tissue-specific ones. This result is expected, as due to the ubiquitous access of circulating FAs to organs and tissues, it is likely that FAs seed DNA methylation profiles that represent common features of the majority of the organism´s epigenomes. Incidentally, our findings confirm and expand the previous observation that normal blood displays a strikingly unique DNA methylation profile, compared to the other tissues and diseases tested.Citation44 In addition to all these considerations, it is noteworthy that the specificity of the overlap with a number of published studies constitutes a powerful independent validation of our 450K array-based results.
Pathways linked to β-oxidation play a pivotal role in determining FA-related methylation profiles. We show that AA-induced DNA hypermethylation is sensitive to inhibition of FA import to mitochondria and is downstream to PPAR-α and SIRT1, 2 central regulators of FA metabolism and β-oxidation.Citation48 THP-1 monocytes, in contrast to cancer cells in general, rely more on β-oxidation than glycolysis for energy production and AA has been shown to be a substrate for β-oxidation in THP-1 cells.Citation58,59 Both these observations support the effects of β-oxidation inhibition in our model system. Indeed, the comparatively small overlap between cancer and FA-induced DNA methylation profiles may be explained by the Warburg effect which states that cancer cells mainly produce ATP from glycolysis even in conditions of hypoxia.Citation60 Following this logic, the DNA hypomethylation observed in diabetes is a predictable consequence of the defective mitochondrial activity associated with that disease.Citation61 In general, blockage of the mitochondrial machinery rapidly shifts ATP production to glycolysis to assure cell survival. We assume that such an adaptation takes place in THP-1 cells following exposure to β-oxidation inhibitor etomoxir, suggesting that inhibition of AA-induced DNA hypermethylation by etomoxir does not result from reduced ATP supply to chromatin modifiers. On the other hand, the OA-induced hypomethylation response is not affected by these inhibitors. This suggests that the distinct AA and OA-induced methylation profiles might relate to FA-specific differences in β-oxidation. Indeed, both in vitro and a recent in-vivo dietary study in humans show that OA relative to other FAs, such as PA and EPA, is a poor substrate of β-oxidation,Citation62-64 although the opposite has also been reported.Citation65 Alternatively, the increased levels of specific intermediate β-oxidation products observed in PA- relative to OA-stimulated human skeletal muscle cells could also explain part, or all of, the PA-induced methylation.Citation62 Although our data contrast with the predominant view that SIRT1 is activated solely in condition of starvation, NAD+ independent SIRT1-mediated upregulation of oxidative metabolism has been reported in several other studies.Citation66-68 Finally, the observation that SIRT1 participates in the AA-induced DNA hypermethylation response in MEFs, indicates that the corresponding response observed in THP-1 cells is not a cancerous cell-restricted, p53- and MIR34a-dependent event.Citation69
The PPAR-α specific regulation of AA-induced DNA methylation, presumably reflects the observation that polyunsaturated FAs are more potent activators of PPAR-α than PPAR-γ.Citation70 The involvement of PPAR-α, but not PPAR-γ, is further supported by the results of the functional annotation analysis of FACpG-harboring genes. SIRT6 can also be activated by long-chain FAs, in particular OA, and contributes to regulation of β-oxidation.Citation71,72 However, knockdown of SIRT6 in MEFs had no effect on FA-induced changes in DNA methylation.
Our observation that AA and OA participate in shaping metabolic disease-specific DNA methylomes through β-oxidation, PPAR-α, and sirtuin 1 signaling, has potential implications for diet-oriented therapy and prevention. Indeed, it has been argued that the high-fat, low-carbohydrate ketogenic diet, which is successful in clinical management of patients with inborn errors of metabolism and pharmacoresistant epilepsy, may be beneficial in a much broader range of diseases than previously recognized.Citation73,74 Conversely, a low-fat, low-carbohydrate diet can lead to normalization of β cell function and insulin resistance in individuals with type 2 diabetes.Citation75 However, whether or not a shift in substrate preference toward fat oxidation lowers disease risk is still heavily debated. For example, prolonged dietary administration of the PPAR-α agonist WY-14643, is associated with a gradual decreased in global and LINE DNA methylation and hepatocarcinogenesis in mice.Citation76,77 Furthermore, the differential tumorigenic responses of xenotransplanted leukemia cells THP-1 or NB4 exposed to either a high-fat or high-carbohydrate diet,Citation78 underlines the importance of understanding energy metabolism of disease subtypes prior to dietary interventions.
Furthermore, we uncover a positive correlation between gene body methylation and expression in THP-1 cells. That correlation represents the behavior of a majority, but not all genes, underlining a complex association between transcription and gene body methylation. Similar conclusions have been drawn in several genome-wide studies of DNA methylation with recent reports suggesting that gene body methylation increases gene expression levels.Citation79,80
Methods
Cell culture
THP-1 monocytes were cultured in RPMI-1640 medium (Gibco) supplemented with 2mM L-Glutamine (Sigma), 10% fetal calf serum (Gibco) and 1% Penicillin/Streptomycin (Gibco). Cells were never allowed to grow above a 1–1.5×106 cells/ml concentration. MEFs obtained from SIRT1- or SIRT6-null mice were a kind gift from Dr. Raúl Mostoslavsky (Harvard University, Boston, USA) and Dr. Eva Bober (MPI, Bad Nauheim, Germany), respectively. SIRT2-null MEFs were generated by standard procedures from SIRT2-null mice. WT MEFs were derived from littermates of each mutant mice strain. MEFs and HEK293T cells were grown in the same conditions as THP-1 monocytes but with DMEM as medium. Pure FAs (Sigma) were conjugated with cell culture-grade FA-free BSA (fraction V, FA-free, Sigma no. 820022) to achieve a FA:BSA 6:1 ratio, essentially as described.Citation81 Typically, 5–6×106 cells in 10 ml medium were stimulated with 100x BSA-FA mix in 2% FCS. Exclusion of trypan blue was used as a criterion for viability. The inhibitors etomoxir, GW9662, GW6471, sirtinol, splitomycin or trichostatin A (TSA) were used at the concentrations and experimental conditions reported in previous studies.Citation82,83 At least 3 technical and biological replicates were performed for each experiment, excluding microarray experiments.
Global DNA methylation
DNA was extracted from cultured cells by standard methods (DNeasy system, Qiagen). For the measurement of global DNA methylation levels, 5-methyl-2′-deoxycytidine (5mdC) and 2′-deoxyguanosine (dG) were determined by an HPLC-based method.Citation84 Normalized 5mdC levels were calculated as percentage 5mdC/dG. When indicated, global DNA methylation was measured by the MethylFlash system (Epigentek). The assay yielded 5mdC values as percentage of PicoGreen-quantified input DNA (wt/wt). Each value was multiplied by 4 to obtain a final value comparable to that obtained by the HPLC-based method. The MethylFlash assay yielded responses to AA and OA in THP-1 monocytes, that were consistent with the ones obtained by the HPLC-based 5mdC quantitation, except for baseline (unstimulated cells) values that were 5% lower (P>0 0.05, data corresponding to a total of 15 and 21 experiments, respectively).
ALU repeat methylation
ALU element methylation was determined according to Sirivanichsuntorn and collaborators.Citation28 Briefly, bisulfite-treated DNA was amplified by PCR with the ALU-specific primers AluF: 5′-GGY GYG GTG GTT TAY GTT TGT AA-3′ and AluR: 5′-TTA ATA AAA ACR AAA TTT CAC CAT ATT AAC CAA AC-3′ as follows: 95°C for 30s, 53°C for 20s (40 cycles), and 72°C for 20s. Subsequently, the 117 bp ALU amplicon was digested overnight at 65°C with TaqI (Invitrogen). The resulting products, ranging between 42 and 117 bp depending on the methylation states of ALU elements, were separated by gel electrophoresis, stained with GelRed and quantified using ImageLab (BioRad).
DNA methylation arrays
To identify targets of FA-induced DNA methylation we used the Infinium HumanMethylation450 BeadChip platform (Illumina). Three biological replicates were pooled in equal proportion for each FA concentration. Pooling effectively averages the data and reduces noise.Citation85 DNA quality checks, bisulfite modification, hybridization, data normalization with the GenomeStudio software (Illumina) and Beta value calculation were carried out as described elsewhere.Citation29,86 SNP-proximal probes and probes with a detection P-value >0.05, i.e., not statistically different from the background, were discarded as reported.Citation45 The methylation level for each cytosine, expressed as a β-value, was calculated as the fluorescence intensity ratio of methylated to unmethylated versions of each probe. Beta values ranged between 0 (unmethylated) and 1 (methylated). Logit-transformed β-values (M-values) were used in statistical tests unless indicated.Citation87 β or Δβ values were used for data description, due to their more intuitive nature. The annotation relating to CpG islands (CGIs) uses the following nomenclature: “shore,” each of the 2 kb-sequences flanking a CGI; “shelf,” each of the 2 kb-sequences next to a shore; “open sea,” DNA not included in shores, shelves or CGIs.Citation29 TSS200 or TSS1500 indicate the region between position −200 bp or −1,500 bp and the Transcription Start Site (TSS), respectively. When a cytosine mapped to different genic elements due to the presence of multiple alternative transcripts, all mapping variants were counted. Functional gene annotation was analyzed with the DAVID tool (http://david.abcc.ncifcrf.gov/). 450K array data are accessible in NCBI's Gene Expression OmnibusCitation88 with the accession number GSE67331. DNA methylation array data of selected CpGs were validated by pyrosequencing (PyroMark Q96 ID, Qiagen). Sequences of pyrosequencing primers are shown in Supplementary Table 6.
Expression arrays
Genome expression was analyzed by Human Exon 1.0 ST arrays (Affymetrix) and we used the Affymetrix Expression Console™ software (version 1.3) and Robust Multichip Average algorithm (RMA) to normalize and analyze array data. The library used was the HuEx-1_0-st-v2.r2.pgf with the probe set HuEx-1_0-st-v2.r2.dt1.hg18.extended.mps (gene level) and HuEx-1_0-st-v2.r2.dt1.hg18.full.mps (exon level). Expression array data are accessible in NCBI's Gene Expression OmnibusCitation88 with the accession number GSE57076. Validation of selected targets was performed by semi-quantitative PCR normalized to GAPDH expression levels.
Statistics
The Kruskal-Wallis test was performed to test for differences in DNA methylation levels between treatments or doses for continuous variables. If the overall comparison was significant, samples were re-analyzed by ANOVA followed by Scheffé's post-hoc test to identify specific different groups. When comparing 2 groups, the Mann-Whitney U test (unpaired samples) or the Wilcoxon test (paired samples). The Chi-square test was applied to compare percentages. Correlations were tested by calculating the Pearson's correlation coefficient and the associated p value. Tests were performed with the Statistica software (StatSoft) or Excel statistics tools (Microsoft Office 2011 for Macintosh). The significance of CpG enrichment was estimated with the hypergeometric test using an online tool (www.geneprof.org/GeneProf/tools/hypergeometric.jsp).
Disclosure of potential conflicts of interest
No potential conflicts of interest were disclosed.
KEPI_A_1161873_supplementary_material.zip
Download Zip (363.3 KB)Acknowledgments
We thank Drs. Holger Heyn and Anna Marazuela-Duque for advice and assistance; Dr. Luis Delaye and Alejandro Gonzalez for granting us access to their computer cluster facility (funded by the State of Guanajuato's Council for Science and Technology (CONCyTEG), project “Laboratorio de aprendizaje e investigación en cómputo biológico LAICBIO” no. GTO-2012-C02-187442). This work was generously funded by: CONCyTEG grant no. 08-03-K662-020-A01, Mexican Council for Science and Technology (CONACyT) Basic Science (Ciencia Básica) grant no. 83401 to G.L; CONACyT Sabbatical Fellowships no. 166209 to G.L. and no. 166058 to S.Z; CONACyT Ph.D. Fellowship no. 334370 to G.A.S.-M; the Spanish Ministry of Economy and Competitiveness (MINECO) grant SAF2011-25619 to A.V.
References
- Randle PJ, Garland PB, Hales CN, Newsholme EA. The glucose fatty-acid cycle. Its role in insulin sensitivity and the metabolic disturbances of diabetes mellitus. Lancet 1963; 1:785-9; PMID:13990765; http://dx.doi.org/10.1016/S0140-6736(63)91500-9
- Keating ST, El-Osta A. Epigenetics and Metabolism. Circ Res 2015; 116:715-36; PMID:25677519; http://dx.doi.org/10.1161/CIRCRESAHA.116.303936
- Brøns C, Jacobsen S, Nilsson E, Rönn T, Jensen CB, Storgaard H, Poulsen P, Groop L, Ling C, Astrup A, et al. Deoxyribonucleic Acid Methylation and Gene Expression of PPARGC1A in Human Muscle Is Influenced by High-Fat Overfeeding in a Birth-Weight-Dependent Manner. J Clin Endocrinol Metab 2010; 95:3048-56; PMID:20410232; http://dx.doi.org/10.1210/jc.2009-2413
- Milagro FI, Gómez-Abellán P, Campión J, Martínez JA, Ordovás JM, Garaulet M. CLOCK, PER2 and BMAL1 DNA Methylation: Association with Obesity and Metabolic Syndrome Characteristics and Monounsaturated Fat Intake. Chronobiol Int 2012; 29:1180-94; PMID:23003921; http://dx.doi.org/10.3109/07420528.2012.719967
- Hermsdorff HH, Mansego ML, Campión J, Milagro FI, Zulet MA, Martínez JA. TNF-alpha promoter methylation in peripheral white blood cells: relationship with circulating TNFα, truncal fat and n-6 PUFA intake in young women. Cytokine 2013; 64:265-71; PMID:23796695; http://dx.doi.org/10.1016/j.cyto.2013.05.028
- Lee H-S, Barraza-Villarreal A, Hernandez-Vargas H, Sly PD, Biessy C, Ramakrishnan U, Romieu I, Herceg Z. Modulation of DNA methylation states and infant immune system by dietary supplementation with ω-3 PUFA during pregnancy in an intervention study. Am J Clin Nutr 2013; 98:480-7; PMID:23761484; http://dx.doi.org/10.3945/ajcn.112.052241
- Aslibekyan S, Wiener HW, Havel PJ, Stanhope KL, O'Brien DM, Hopkins SE, Absher DM, Tiwari HK, Boyer BB. DNA methylation patterns are associated with n-3 fatty acid intake in Yup'ik people. J Nutr 2014; 144:425-30; PMID:24477300; http://dx.doi.org/10.3945/jn.113.187203
- Hoile SP, Clarke-Harris R, Huang R-C, Calder PC, Mori TA, Beilin LJ, Lillycrop KA, Burdge GC. Supplementation with N-3 long-chain polyunsaturated fatty acids or olive oil in men and women with renal disease induces differential changes in the DNA methylation of FADS2 and ELOVL5 in peripheral blood mononuclear cells. PLoS One 2014; 9:e109896; PMID:25329159; http://dx.doi.org/10.1371/journal.pone.0109896
- Voisin S, Almén MS, Moschonis G, Chrousos GP, Manios Y, Schiöth HB. Dietary fat quality impacts genome-wide DNA methylation patterns in a cross-sectional study of Greek preadolescents. Eur J Hum Genet 2015; 23:654-62; PMID:25074463; http://dx.doi.org/10.1038/ejhg.2014.139
- Burdge GC, Lillycrop KA. Fatty acids and epigenetics. Curr Opin Clin Nutr Metab Care 2014; 17:156-61; PMID:24322369; http://dx.doi.org/10.1097/MCO.0000000000000023
- Christman JK, Price P, Pedrinan L, Acs G. Correlation between hypomethylation of DNA and expression of globin genes in Friend erythroleukemia cells. Eur J Biochem 1977; 81:53-61; PMID:271073; http://dx.doi.org/10.1111/j.1432-1033.1977.tb11926.x
- Lund G, Andersson L, Lauria M, Lindholm M, Fraga MF, Villar-Garea A, Ballestar E, Esteller M, Zaina S. DNA methylation polymorphisms precede any histological sign of atherosclerosis in mice lacking apolipoprotein E. J Biol Chem 2004; 279:29147-54; PMID:15131116; http://dx.doi.org/10.1074/jbc.M403618200
- Rangel-Salazar R, Wickström-Lindholm M, Aguilar-Salinas CA, Alvarado-Caudillo Y, Døssing KB V, Esteller M, Labourier E, Lund G, Nielsen FC, Rodríguez-Ríos D, et al. Human native lipoprotein-induced de novo DNA methylation is associated with repression of inflammatory genes in THP-1 macrophages. BMC Genomics 2011; 12:582; PMID:22118513; http://dx.doi.org/10.1186/1471-2164-12-582
- Kiec-Wilk B, Polus A, Mikolajczyk M, Mathers JC. Beta-carotene and arachidonic acid induced DNA methylation and the regulation of pro-chemotactic activity of endothelial cells and its progenitors. J Physiol Pharmacol 2007; 58:757-66; PMID:18195486
- Kiec-Wilk B, Razny U, Mathers JC, Dembinska-Kiec A. DNA methylation, induced by beta-carotene and arachidonic acid, plays a regulatory role in the pro-angiogenic VEGF-receptor (KDR) gene expression in endothelial cells. J Physiol Pharmacol 2009; 60:49-53; PMID:20065496
- Ceccarelli V, Racanicchi S, Martelli MP, Nocentini G, Fettucciari K, Riccardi C, Marconi P, Di Nardo P, Grignani F, Binaglia L, et al. Eicosapentaenoic acid demethylates a single CpG that mediates expression of tumor suppressor CCAAT/enhancer-binding protein delta in U937 leukemia cells. J Biol Chem 2011; 286:27092-102; PMID:21659508; http://dx.doi.org/10.1074/jbc.M111.253609
- Barrès R, Osler ME, Yan J, Rune A, Fritz T, Caidahl K, Krook A, Zierath JR. Non-CpG methylation of the PGC-1alpha promoter through DNMT3B controls mitochondrial density. Cell Metab 2009; 10:189-98; http://dx.doi.org/10.1016/j.cmet.2009.07.011
- Hall E, Volkov P, Dayeh T, Bacos K, Rönn T, Nitert MD, Ling C. Effects of palmitate on genome-wide mRNA expression and DNA methylation patterns in human pancreatic islets. BMC Med 2014; 12:103; PMID:24953961; http://dx.doi.org/10.1186/1741-7015-12-103
- Lambert M-P, Ancey P-B, Esposti DD, Cros M-P, Sklias A, Scoazec J-Y, Durantel D, Hernandez-Vargas H, Herceg Z. Aberrant DNA methylation of imprinted loci in hepatocellular carcinoma and after in vitro exposure to common risk factors. Clin Epigenetics 2015; 7:15; PMID:25755686; http://dx.doi.org/10.1186/s13148-015-0053-9
- Sugii S, Evans RM. Epigenetic codes of PPARγ in metabolic disease. FEBS Lett 2011; 585:2121-8; PMID:21605560; http://dx.doi.org/10.1016/j.febslet.2011.05.007
- Blattler A, Farnham PJ. Cross-talk between site-specific transcription factors and DNA methylation states. J Biol Chem 2013; 288:34287-94; PMID:24151070; http://dx.doi.org/10.1074/jbc.R113.512517
- Calder PC. Polyunsaturated fatty acids and inflammatory processes: New twists in an old tale. Biochimie 2009; 91:791-5; PMID:19455748; http://dx.doi.org/10.1016/j.biochi.2009.01.008
- Jové M, Laguna JC, Vázquez-Carrera M. Agonist-induced activation releases peroxisome proliferator-activated receptor beta/delta from its inhibition by palmitate-induced nuclear factor-kappaB in skeletal muscle cells. Biochim Biophys Acta 2005; 1734:52-61; http://dx.doi.org/10.1016/j.bbalip.2005.02.002
- Gao D, Griffiths HR, Bailey CJ. Oleate protects against palmitate-induced insulin resistance in L6 myotubes. Br J Nutr 2009; 102:1557-63; PMID:19622194; http://dx.doi.org/10.1017/S0007114509990948
- Qin Z. The use of THP-1 cells as a model for mimicking the function and regulation of monocytes and macrophages in the vasculature. Atherosclerosis 2012; 221:2-11; PMID:21978918; http://dx.doi.org/10.1016/j.atherosclerosis.2011.09.003
- Higashiyama A, Kubota Y, Marumo M, Konishi M, Yamashita Y, Nishimura K, Fukuda Y, Okamura T, Wakabayashi I. Association between serum long-chain n-3 and n-6 polyunsaturated fatty acid profiles and glomerular filtration rate assessed by serum creatinine and cystatin C levels in Japanese community-dwellers. J Epidemiol 2015; 25:303-11; PMID:25728619; http://dx.doi.org/10.2188/jea.JE20140093
- Finstad HS, Kolset SO, Holme JA, Wiger R, Farrants AK, Blomhoff R, Drevon CA. Effect of n-3 and n-6 fatty acids on proliferation and differentiation of promyelocytic leukemic HL-60 cells. Blood 1994; 84:3799-809; PMID:7949136
- Sirivanichsuntorn P, Keelawat S, Danuthai K, Mutirangura A, Subbalekha K, Kitkumthorn N. LINE-1 and Alu hypomethylation in mucoepidermoid carcinoma. BMC Clin Pathol 2013; 13:10; PMID:23510117; http://dx.doi.org/10.1186/1472-6890-13-10
- Sandoval J, Heyn H, Moran S, Serra-Musach J, Pujana MA, Bibikova M. Validation of a DNA methylation microarray for 450,000 CpG sites in the human genome. Epigenetics 2011; 6:692-702; PMID:21593595; http://dx.doi.org/10.4161/epi.6.6.16196
- Bibikova M, Barnes B, Tsan C, Ho V, Klotzle B, Le JM, Delano D, Zhang L, Schroth GP, Gunderson KL, et al. High density DNA methylation array with single CpG site resolution. Genomics 2011; 98:288-95; PMID:21839163; http://dx.doi.org/10.1016/j.ygeno.2011.07.007
- Schupp M, Lazar MA. Endogenous ligands for nuclear receptors: digging deeper. J Biol Chem 2010; 285:40409-15; PMID:20956526; http://dx.doi.org/10.1074/jbc.R110.182451
- Christy RJ, Yang VW, Ntambi JM, Geiman DE, Landschulz WH, Friedman AD, Nakabeppu Y, Kelly TJ, Lane MD. Differentiation-induced gene expression in 3T3-L1 preadipocytes: CCAAT/enhancer binding protein interacts with and activates the promoters of two adipocyte-specific genes. Genes Dev 1989; 3:1323-35; PMID:2606350; http://dx.doi.org/10.1101/gad.3.9.1323
- Zaina S, Heyn H, Carmona FJ, Varol N, Sayols S, Condom E, Ramírez-Ruz J, Gomez A, Gonçalves I, Moran S, et al. DNA methylation map of human atherosclerosis. Circ Cardiovasc Genet 2014; 7:692-700; PMID:25091541; http://dx.doi.org/10.1161/CIRCGENETICS.113.000441
- Benton MC, Johnstone A, Eccles D, Harmon B, Hayes MT, Lea RA, Griffiths L, Hoffman EP, Stubbs RS, Macartney-Coxson D. An analysis of DNA methylation in human adipose tissue reveals differential modification of obesity genes before and after gastric bypass and weight loss. Genome Biol 2015; 16:8; PMID:25651499; http://dx.doi.org/10.1186/s13059-014-0569-x
- Dayeh T, Volkov P, Salö S, Hall E, Nilsson E, Olsson AH, Kirkpatrick CL, Wollheim CB, Eliasson L, Rönn T, et al. Genome-Wide DNA Methylation Analysis of Human Pancreatic Islets from Type 2 Diabetic and Non-Diabetic Donors Identifies Candidate Genes That Influence Insulin Secretion. PLoS Genet 2014; 10:e1004160; PMID:24603685; http://dx.doi.org/10.1371/journal.pgen.1004160
- Nardone S, Sams D, Reuveni E, Getselter D, Oron O, Karpuj M, Elliott E. DNA methylation analysis of the autistic brain reveals multiple dysregulated biological pathways. Transl Psychiatry 2014; 4:e433; PMID:25180572; http://dx.doi.org/10.1038/tp.2014.70
- Wockner LF, Noble EP, Lawford BR, Young RM, Morris CP, Whitehall VL, Voisey J. Genome-wide DNA methylation analysis of human brain tissue from schizophrenia patients. Transl Psychiatry 2014; 4:e339; PMID:24399042; http://dx.doi.org/10.1038/tp.2013.111
- Nordlund J, Bäcklin CL, Wahlberg P, Busche S, Berglund EC, Eloranta M-L, Flaegstad T, Forestier E, Frost B-M, Harila-Saari A, et al. Genome-wide signatures of differential DNA methylation in pediatric acute lymphoblastic leukemia. Genome Biol 2013; 14:r105; PMID:24063430; http://dx.doi.org/10.1186/gb-2013-14-9-r105
- Shen J, Wang S, Zhang Y-J, Wu H-C, Kibriya MG, Jasmine F, Ahsan H, Wu DP, Siegel AB, Remotti H, et al. Exploring genome-wide DNA methylation profiles altered in hepatocellular carcinoma using Infinium HumanMethylation 450 BeadChips. Epigenetics 2013; 8:34-43; PMID:23208076; http://dx.doi.org/10.4161/epi.23062
- Heyn H, Carmona FJ, Gomez A, Ferreira HJ, Bell JT, Sayols S, Ward K, Stefansson OA, Moran S, Sandoval J, et al. DNA methylation profiling in breast cancer discordant identical twins identifies DOK7 as novel epigenetic biomarker. Carcinogenesis 2013; 34:102-8; PMID:23054610; http://dx.doi.org/10.1093/carcin/bgs321
- Naumov VA, Generozov E V, Zaharjevskaya NB, Matushkina DS, Larin AK, Chernyshov S V, Alekseev M V, Shelygin YA, Govorun VM. Genome-scale analysis of DNA methylation in colorectal cancer using Infinium HumanMethylation450 BeadChips. Epigenetics 2013; 8:921-34; PMID:23867710; http://dx.doi.org/10.4161/epi.25577
- Steegenga WT, Boekschoten M V, Lute C, Hooiveld GJ, de Groot PJ, Morris TJ, Teschendorff AE, Butcher LM, Beck S, Müller M. Genome-wide age-related changes in DNA methylation and gene expression in human PBMCs. Age (Dordr) 2014; 36:9648; PMID:24789080; http://dx.doi.org/10.1007/s11357-014-9648-x
- Liu JJ, Green P, John Mann J, Rapoport SI, Sublette ME. Pathways of polyunsaturated fatty acid utilization: Implications for brain function in neuropsychiatric health and disease. Brain Res 2014; 1597:220-46; PMID:25498862; http://dx.doi.org/10.1016/j.brainres.2014.11.059
- Lowe R, Slodkowicz G, Goldman N, Rakyan VK. The human blood DNA methylome displays a highly distinctive profile compared with other somatic tissues. Epigenetics 2015; 10:274-81; PMID:25634226; http://dx.doi.org/10.1080/15592294.2014.1003744
- Valencia-Morales MDP, Zaina S, Heyn H, Carmona FJ, Varol N, Sayols S, Condom E, Ramírez-Ruz J, Gomez A, Moran S, et al. The DNA methylation drift of the atherosclerotic aorta increases with lesion progression. BMC Med Genomics 2015; 8:7; PMID:25881171; http://dx.doi.org/10.1186/s12920-015-0085-1
- Shu H, Wong B, Zhou G, Li Y, Berger J, Woods JW, Wright SD, Cai TQ. Activation of PPARalpha or gamma reduces secretion of matrix metalloproteinase 9 but not interleukin 8 from human monocytic THP-1 cells. Biochem Biophys Res Commun 2000; 267:345-9; PMID:10623622; http://dx.doi.org/10.1006/bbrc.1999.1968
- Zheng X, Sun T, Wang X. Activation of type 2 cannabinoid receptors (CB2R) promotes fatty acid oxidation through the SIRT1/PGC-1α pathway. Biochem Biophys Res Commun 2013; 436:377-81; PMID:23747418; http://dx.doi.org/10.1016/j.bbrc.2013.05.108
- Purushotham A, Schug TT, Xu Q, Surapureddi S, Guo X, Li X. Hepatocyte-specific deletion of SIRT1 alters fatty acid metabolism and results in hepatic steatosis and inflammation. Cell Metab 2009; 9:327-38; PMID:19356714; http://dx.doi.org/10.1016/j.cmet.2009.02.006
- Houtkooper RH, Pirinen E, Auwerx J. Sirtuins as regulators of metabolism and healthspan. Nat Rev Mol Cell Biol 2012; 13:225-38; PMID:22395773; http://dx.doi.org/10.1038/nrn3209
- Kothapalli KSD, Anthony JC, Pan BS, Hsieh AT, Nathanielsz PW, Brenna JT. Differential cerebral cortex transcriptomes of baboon neonates consuming moderate and high docosahexaenoic acid formulas. PLoS One 2007; 2:e370; PMID:17426818; http://dx.doi.org/10.1371/journal.pone.0000370
- Olsson AH, Volkov P, Bacos K, Dayeh T, Hall E, Nilsson EA, Ladenvall C, Rönn T, Ling C. Genome-Wide Associations between Genetic and Epigenetic Variation Influence mRNA Expression and Insulin Secretion in Human Pancreatic Islets. PLoS Genet 2014; 10:e1004735; PMID:25375650; http://dx.doi.org/10.1371/journal.pgen.1004735
- Hancock AM, Witonsky DB, Ehler E, Alkorta-Aranburu G, Beall C, Gebremedhin A, Sukernik R, Utermann G, Pritchard J, Coop G, et al. Colloquium paper: human adaptations to diet, subsistence, and ecoregion are due to subtle shifts in allele frequency. Proc Natl Acad Sci U S A 2010; 107Suppl:8924-30; PMID:20445095; http://dx.doi.org/10.1073/pnas.0914625107
- Jacobsen SC, Brøns C, Bork-Jensen J, Ribel-Madsen R, Yang B, Lara E, Hall E, Calvanese V, Nilsson E, Jørgensen SW, et al. Effects of short-term high-fat overfeeding on genome-wide DNA methylation in the skeletal muscle of healthy young men. Diabetologia 2012; 55:3341-9; PMID:22961225; http://dx.doi.org/10.1007/s00125-012-2717-8
- Lindholm ME, Marabita F, Gomez-Cabrero D, Rundqvist H, Ekström TJ, Tegnér J, Sundberg CJ. An integrative analysis reveals coordinated reprogramming of the epigenome and the transcriptome in human skeletal muscle after training. Epigenetics 2014; 9:1557-69; PMID:25484259; http://dx.doi.org/10.4161/15592294.2014.982445
- Cao Q, Wang X, Jia L, Mondal AK, Diallo A, Hawkins GA, Das SK, Parks JS, Yu L, Shi H, et al. Inhibiting DNA Methylation by 5-Aza-2′-deoxycytidine ameliorates atherosclerosis through suppressing macrophage inflammation. Endocrinology 2014; 155:4925-38; PMID:25251587; http://dx.doi.org/10.1210/en.2014-1595
- Schultz ST, Klonoff-Cohen HS, Wingard DL, Akshoomoff NA, Macera CA, Ji M, Bacher C. Breastfeeding, infant formula supplementation, and Autistic Disorder: the results of a parent survey. Int Breastfeed J 2006; 1:16; PMID:16978397; http://dx.doi.org/10.1186/1746-4358-1-16
- Brigandi SA, Shao H, Qian SY, Shen Y, Wu B-L, Kang JX. Autistic children exhibit decreased levels of essential Fatty acids in red blood cells. Int J Mol Sci 2015; 16:10061-76; PMID:25946342; http://dx.doi.org/10.3390/ijms160510061
- Barbieri B, Alvelius G, Papadogiannakis N. Lower arachidonic acid content and preferential beta-oxidation of arachidonic acid over palmitic acid in tumour cell lines as compared to normal lymphoid cells. Biochem Mol Biol Int 1998; 45:1105-12; PMID:9762408
- Suganuma K, Miwa H, Imai N, Shikami M, Gotou M, Goto M, Mizuno S, Takahashi M, Yamamoto H, Hiramatsu A, et al. Energy metabolism of leukemia cells: glycolysis versus oxidative phosphorylation. Leuk Lymphoma 2010; 51:2112-9; PMID:20860495; http://dx.doi.org/10.3109/10428194.2010.512966
- Warburg O. On the origin of cancer cells. Science 1956; 123:309-14; PMID:13298683; http://dx.doi.org/10.1126/science.123.3191.309
- Koves TR, Ussher JR, Noland RC, Slentz D, Mosedale M, Ilkayeva O, Bain J, Stevens R, Dyck JR, Newgard CB, et al. Mitochondrial overload and incomplete fatty acid oxidation contribute to skeletal muscle insulin resistance. Cell Metab 2008; 7:45-56; PMID:18177724; http://dx.doi.org/10.1016/j.cmet.2007.10.013
- Bakke SS, Moro C, Nikolić N, Hessvik NP, Badin P-M, Lauvhaug L, Fredriksson K, Hesselink MK, Boekschoten M V, Kersten S, et al. Palmitic acid follows a different metabolic pathway than oleic acid in human skeletal muscle cells; lower lipolysis rate despite an increased level of adipose triglyceride lipase. Biochim Biophys Acta 2012; 1821:1323-33; PMID:22796147; http://dx.doi.org/10.1016/j.bbalip.2012.07.001
- Guo W, Xie W, Lei T, Hamilton JA. Eicosapentaenoic acid, but not oleic acid, stimulates beta-oxidation in adipocytes. Lipids 2005; 40:815-21; PMID:16296400; http://dx.doi.org/10.1007/s11745-005-1443-8
- Kien CL, Bunn JY, Stevens R, Bain J, Ikayeva O, Crain K, Koves TR, Muoio DM. Dietary intake of palmitate and oleate has broad impact on systemic and tissue lipid profiles in humans. Am J Clin Nutr 2014; 99:436-45; PMID:24429541; http://dx.doi.org/10.3945/ajcn.113.070557
- Lim J-H, Gerhart-Hines Z, Dominy JE, Lee Y, Kim S, Tabata M, Xiang YK, Puigserver P. Oleic acid stimulates complete oxidation of fatty acids through protein kinase A-dependent activation of SIRT1-PGC1α complex. J Biol Chem 2013; 288:7117-26; PMID:23329830; http://dx.doi.org/10.1074/jbc.M112.415729
- Khan SA, Sathyanarayan A, Mashek MT, Ong KT, Wollaston-Hayden EE, Mashek DG. ATGL-Catalyzed Lipolysis Regulates SIRT1 to Control PGC-1α/PPAR-α Signaling. Diabetes 2015; 64:418-26; PMID:25614670; http://dx.doi.org/10.2337/db14-0325
- Gerhart-Hines Z, Dominy JE, Blättler SM, Jedrychowski MP, Banks AS, Lim J-H, Chim H, Gygi SP, Puigserver P. The cAMP/PKA pathway rapidly activates SIRT1 to promote fatty acid oxidation independently of changes in NAD(+). Mol Cell 2011; 44:851-63; PMID:22195961; http://dx.doi.org/10.1016/j.molcel.2011.12.005
- Nin V, Escande C, Chini CC, Giri S, Camacho-Pereira J, Matalonga J, Lou Z, Chini EN. Role of deleted in breast cancer 1 (DBC1) protein in SIRT1 deacetylase activation induced by protein kinase A and AMP-activated protein kinase. J Biol Chem 2012; 287:23489-501; PMID:22553202; http://dx.doi.org/10.1074/jbc.M112.365874
- Yamakuchi M, Ferlito M, Lowenstein CJ. miR-34a repression of SIRT1 regulates apoptosis. Proc Natl Acad Sci U S A 2008; 105:13421-6; PMID:18755897; http://dx.doi.org/10.1073/pnas.0801613105
- Forman BM, Chen J, Evans RM. Hypolipidemic drugs, polyunsaturated fatty acids, and eicosanoids are ligands for peroxisome proliferator-activated receptors alpha and delta. Proc Natl Acad Sci U S A 1997; 94:4312-7; PMID:9113986; http://dx.doi.org/10.1073/pnas.94.9.4312
- Feldman JL, Baeza J, Denu JM. Activation of the protein deacetylase SIRT6 by long-chain fatty acids and widespread deacylation by mammalian sirtuins. J Biol Chem 2013; 288:31350-6; PMID:24052263; http://dx.doi.org/10.1074/jbc.C113.511261
- Masri S, Rigor P, Cervantes M, Ceglia N, Sebastian C, Xiao C, Roqueta-Rivera M, Deng C, Osborne TF, Mostoslavsky R, et al. Partitioning circadian transcription by SIRT6 leads to segregated control of cellular metabolism. Cell 2014; 158:659-72; PMID:25083875; http://dx.doi.org/10.1016/j.cell.2014.06.050
- Seyfried TN, Flores R, Poff AM, D'Agostino DP, Mukherjee P. Metabolic therapy: A new paradigm for managing malignant brain cancer. Cancer Lett 2015; 356:289-300; PMID:25069036; http://dx.doi.org/10.1016/j.canlet.2014.07.015
- Vidali S, Aminzadeh S, Lambert B, Rutherford T, Sperl W, Kofler B, Feichtinger RG. MITOCHONDRIA: The ketogenic diet-a metabolism-based therapy. Int J Biochem Cell Biol 2015; 63:55-9; PMID:25666556; http://dx.doi.org/10.1016/j.biocel.2015.01.022
- Lim EL, Hollingsworth KG, Aribisala BS, Chen MJ, Mathers JC, Taylor R. Reversal of type 2 diabetes: normalisation of beta cell function in association with decreased pancreas and liver triacylglycerol. Diabetologia 2011; 54:2506-14; PMID:21656330; http://dx.doi.org/10.1007/s00125-011-2204-7
- Peters JM, Cheung C, Gonzalez FJ. Peroxisome proliferator-activated receptor-alpha and liver cancer: where do we stand? J Mol Med (Berl) 2005; 83:774-85; PMID:15976920; http://dx.doi.org/10.1007/s00109-005-0678-9
- Pogribny IP, Tryndyak VP, Woods CG, Witt SE, Rusyn I. Epigenetic effects of the continuous exposure to peroxisome proliferator WY-14,643 in mouse liver are dependent upon peroxisome proliferator activated receptor alpha. Mutat Res 2007; 625:62-71; PMID:17586532; http://dx.doi.org/10.1016/j.mrfmmm.2007.05.004
- Tsunekawa-Imai N, Miwa H, Shikami M, Suganuma K, Goto M, Mizuno S, Takahashi M, Mizutani M, Horio T, Komatsubara H, et al. Growth of xenotransplanted leukemia cells is influenced by diet nutrients and is attenuated with 2-deoxyglucose. Leuk Res 2013; 37:1132-6; PMID:23806233; http://dx.doi.org/10.1016/j.leukres.2013.05.017
- Yang X, Han H, De Carvalho DD, Lay FD, Jones PA, Liang G. Gene Body Methylation Can Alter Gene Expression and Is a Therapeutic Target in Cancer. Cancer Cell 2014; 26:577-90; PMID:25263941; http://dx.doi.org/10.1016/j.ccr.2014.07.028
- Schroeder DI, Jayashankar K, Douglas KC, Thirkill TL, York D, Dickinson PJ, Williams LE, Samollow PB, Ross PJ, Bannasch DL, et al. Early Developmental and Evolutionary Origins of Gene Body DNA Methylation Patterns in Mammalian Placentas. PLoS Genet 2015; 11:e1005442; PMID:26241857; http://dx.doi.org/10.1371/journal.pgen.1005442
- Marshall C, Hitman GA, Cassell PG, Turner MD. Effect of glucolipotoxicity and rosiglitazone upon insulin secretion. Biochem Biophys Res Commun 2007; 356:756-62; PMID:17379187; http://dx.doi.org/10.1016/j.bbrc.2007.03.047
- Selva DM, Hammond GL. Thyroid hormones act indirectly to increase sex hormone-binding globulin production by liver via hepatocyte nuclear factor-4alpha. J Mol Endocrinol 2009; 43:19-27; PMID:19336534; http://dx.doi.org/10.1677/JME-09-0025
- Ali FY, Armstrong PCJ, Dhanji A-RA, Tucker AT, Paul-Clark MJ, Mitchell JA, Warner TD. Antiplatelet actions of statins and fibrates are mediated by PPARs. Arterioscler Thromb Vasc Biol 2009; 29:706-11; PMID:19150877; http://dx.doi.org/10.1161/ATVBAHA.108.183160
- Magaña AA, Wrobel K, Caudillo YA, Zaina S, Lund G, Wrobel K. High-performance liquid chromatography determination of 5-methyl-2′-deoxycytidine, 2′-deoxycytidine, and other deoxynucleosides and nucleosides in DNA digests. Anal Biochem 2008; 374:378-85; http://dx.doi.org/10.1016/j.ab.2007.11.026
- Peng X, Wood CL, Blalock EM, Chen KC, Landfield PW, Stromberg AJ. Statistical implications of pooling RNA samples for microarray experiments. BMC Bioinformatics 2003; 4:26; PMID:12823867; http://dx.doi.org/10.1186/1471-2105-4-26
- Heyn H, Li N, Ferreira HJ, Moran S, Pisano DG, Gomez A, Diez J, Sanchez-Mut J V., Setien F, Carmona FJ, et al. Distinct DNA methylomes of newborns and centenarians. Proc Natl Acad Sci U S A 2012; 109:10522-7; PMID:22689993; http://dx.doi.org/10.1073/pnas.1120658109
- Du P, Zhang X, Huang C-C, Jafari N, Kibbe WA, Hou L, Lin SM. Comparison of Beta-value and M-value methods for quantifying methylation levels by microarray analysis. BMC Bioinformatics 2010; 11:587; PMID:21118553; http://dx.doi.org/10.1186/1471-2105-11-587
- Edgar R, Domrachev M, Lash AE. Gene Expression Omnibus: NCBI gene expression and hybridization array data repository. Nucleic Acids Res 2002; 30:207-10; PMID:11752295; http://dx.doi.org/10.1093/nar/30.1.207