ABSTRACT
The placenta regulates the in utero environment and functionally impacts fetal development. Candidate gene studies identified variation in placental DNA methylation is associated with newborn neurologic and behavioral outcomes including movement quality, lethargic behavior, attention, and arousal. We sought to identify novel regions of variable DNA methylation associated with newborn attention, lethargy, quality of movement, and arousal by performing an epigenome-wide association study in 335 infants from a US birth cohort. Methylation status was quantified using the Illumina HumanMethylation450 BeadChip array and associations to newborn outcomes assessed by the NICU Network Neurobehavioral Scales (NNNS) were identified while incorporating established bioinformatics algorithms to control for confounding by cell type composition. Methylation of CpGs within FHIT (cg15970800) and ANKRD11 (cg16710656) demonstrated genome-wide significance (P < 1.8 × 10−7) in specific associations with infant attention. CpGs whose differential methylation was associated with all 4 neurobehavioral outcomes were common to 50 genes involved in biological processes relating to cellular adhesion and nervous system development. Comprehensive methylation profiling identified relationships between methylation of FHIT and ANKRD11, which have been previously linked to neurodevelopment and behavioral outcomes in genetic association studies. Subtle changes in DNA methylation of these genes within the placenta may impact normal variation of a newborn's ability to alter and track visual and auditory stimuli. Gene ontology analysis suggested that those genes with variable methylation related to these outcomes are over-represented in biological pathways involved in brain development and placental physiology, supportive of our hypothesis for a key role of the placenta in neurobehavioral outcomes.
Introduction
As the incidence rates of pervasive developmental disorders and mental illnesses rise, there is a great need to understand the underlying biology, as well as to identify early biomarkers of these disorders. Human studies and animal models have revealed that the prenatal environment has long-term impacts on health outcomes.Citation1 The placenta is the master regulator of the environment of the developing fetus and is a key tissue to understand the mechanistic basis of the fetal origins of adult disease paradigm.Citation2,3 The placenta expresses a number of neuropeptide and endocrine hormones,Citation4 and alterations to placental physiology have been associated with a number of acute and long-term outcomes in the developing infant.Citation5,6
Epigenetic regulation of the placental genome can influence placental function and signaling, with long-term impacts on fetal health. This study focuses on DNA methylation, a chemical modification that typically occurs in cytosines that are followed by guanine (known as CpGs).Citation7 DNA methylation in key regulatory regions, including the promoter, 5′UTR, and gene body has been shown to interfere with the transcription of DNA into mRNA, as reviewed in Kulis and Esteller.Citation7 DNA methylation can be environmentally modified during the in utero period and epigenetic changes are thought to be an important mechanism by which fetal programming can occur.Citation8 A growing body of research is focused on examining the placental epigenome as a mediator of the in utero environment on children's health outcomes.Citation9
Prior work by our group and others has examined variation in DNA methylation of candidate genes involved in key metabolic and endocrine processes and its relationship to newborn neurobehavioral outcomes assessed using the NICU Network Neurobehavioral scales (NNNS).Citation9-15 The NNNS is designed to evaluate neurobehavioral outcomes across multiple domains, including cognitive and neurological outcomes, habituation, and stress response, and can be utilized to evaluate central nervous system integrity, behavior, and interactive responses in a broadly applicable fashion.Citation16 The NNNS has established validity in evaluating and predicting cognitive and behavioral outcomes in both healthy infants,Citation17 preterm infants,Citation18-20 and infants that have been exposed to some degree of in utero adversity.Citation21-28 Specific newborn characteristics that are quantified within the NNNS assessment, including newborn attention, quality of movement, lethargy, and arousal, have been repeatedly linked to methylation of genes involved in the serotonin response,Citation13 the development and regulation of the HPA axis,Citation10-12,14,15 and leptin regulation.Citation29
It is likely that additional genes and pathways, beyond those candidate genes, can be implicated in the development of complex neurobehavioral phenotypes. In this study, we sought to examine the relationship between DNA methylation across the placental epigenome using the Illumina Infinium HumanMethylation450 BeadChip (450K) array and infant neurobehavior, as quantified in the NNNS assessment, in 335 newborns from the Rhode Island Child Health Study (RICHS). With this approach, we aim to identify novel regions of variable DNA methylation associated with characteristics of infant behavior and neurologic function and explore underlying molecular pathways associated with differential methylation in regards to these neurobehavioral traits within a healthy population of infants.
Results
Descriptive statistics of newborns
Demographic characteristics of the 335 infants in this study are shown in . The distribution of birth weight groups reflects the study sampling strategy, which oversampled for large for gestational age (LGA, >90th birth weight percentile, 28.1%) and small for gestational age infants (SGA, <10th birth weight percentile, 20%). Females and males are nearly evenly distributed in this study (51.3% vs. 48.9%). The prevalence of anxiety and depression in our population were 12.2 and 13.4%, respectively, which is comparable to the reported prevalence in other cohort studies.Citation30 The majority of women were white (75.8%) and had achieved a high school degree or higher (72.67%), and 48.4% had a BMI of 25 kg/m2 or higher and, thus, were categorized as overweight/obese. The infants in this study exhibit NNNS outcomes that are normally distributed across the possible range of the scores (). The range of these 4 NNNS scores is comparable to norms exhibited within similar, low risk populations.Citation31
Table 1. Descriptive Statistics of Study Participants.
Table 2. Description of NNNS outcomes and summary of Study Participants.
Results of epigenome-wide association study (EWAS)
The distributions of coefficients and P values from reference-free corrected based models examining the association between DNA methylation and newborn arousal, attention, lethargy, and quality of movement are depicted as volcano plots in , with the results of the models unadjusted for differences in cellular proportions shown in Supplemental Fig. 1. With a significance cutoff of P < 0.005, differential methylation of 2,229 CpGs was associated with infant arousal, 1,930 CpGs associated with attention, 2,169 CpGs associated with lethargy, and 1,989 CpGs associated with quality of movement after adjusting for cellular composition.
Figure 1. Volcano plot displaying results of adjusted reference free models for arousal (A), Attention (B), Lethargy (C) and Quality of Movement (D), where each dot represents 1 CpG site. The red line represents a significance level of P < 0.05, and a blue line represents a significance level of P < 0.005. CpGs that are highly significant after correction for multiple comparisons are shown by red asterisks.
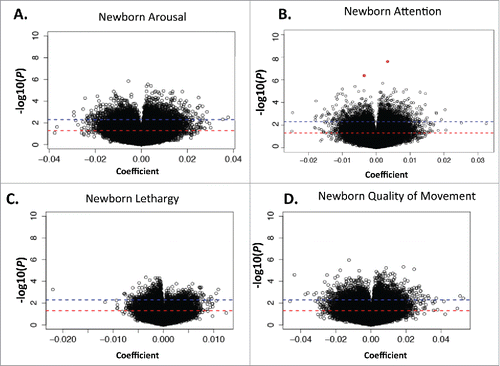
To examine which CpGs demonstrated the greatest change in their coefficient by the reference-free correction, and are those most likely influenced by cellular composition, we ranked the CpG sites by their delta value, representing the difference in coefficients between the adjusted and unadjusted models. As expected, the majority of the CpGs highly associated with NNNS outcomes were not those CpG sites most significantly affected by cellular composition correction (). Of the 2,229 CpGs whose methylation was associated with arousal (unadjusted P < 0.005), only 17 CpGs had significant changes (unadjusted P < 0.005) in coefficient estimates. None of the CpGs associated with other NNNS scores had significant changes in coefficient estimates, using an unadjusted P value of 0.005.
Figure 2. Volcano plot displaying the changes in β between adjusted and unadjusted model and associated P values for arousal (A), Attention (B), Lethargy (C) and Quality of Movement (D), where dot represents 1 CpG site. The red line represents a significance level of unadjusted P < 0.05 in association with delta value. CpG sites with an unadjusted P value of <0 .005 in association with NNNS outcome, which are above the blue line in , are shaded gray. No CpGs were significant after correction for multiple comparisons.
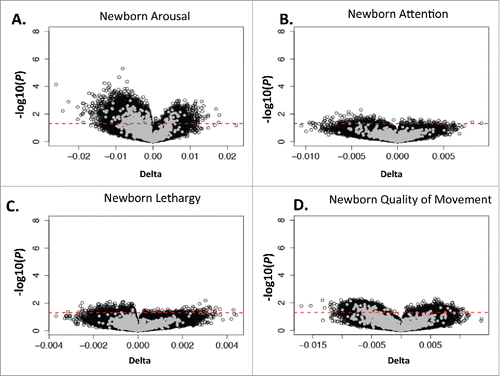
Two CpGs reached genome-wide significance (P < 1.8 × 10−7) in their association with attention (depicted as red dots in ). These CpGs include cg15970800 (P = 2.42 × 10−8), located in the FHIT 5′ UTR and cg16710656 (P = 4.25 x10−7), located in the ANKRD11 gene body. depicts the relationship between methylation of these CpGs and normalized attention scores. There was one potential outlier of cg16710656, but removing this outlier made no difference in the effect estimate of the model. shows results of individual linear regression models of these CpG sites and attention controlled for confounders. These models revealed that a 1% increase in methylation of cg15970800 is associated with a 0.15 standard deviation increase in infant attention (Estimate 0.15, P = 7.04 × 10−4). A 1% increase in methylation of cg16710656 was associated with a 0.17 standard deviation decrease in NNNS attention score (Estimate −0.17, P = 2.32 × 10−5).
Figure 3. Scatter plots of unadjusted model between (A) methylation of cg15970800 (FHIT 5′ UTR) and normalized infant attention scores, and (B) methylation of cg16710656 (ANKRD11 gene body) and normalized infant attention scores.
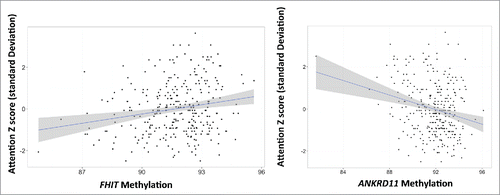
Table 3. Linear regression model of ANKRD11 and FHIT methylation with infant attention.
Relationship between methylation of genes and molecular pathways
To examine broader patterns of epigenetic regulation of genes and neurobehavioral outcomes, we examined over-representation of gene ontology terms of the genes represented by the CpGs identified with differential methylation associated with each of the NNNS scores at P < 0.005. The top 10 gene ontology (GO) terms overrepresented by these genes for each NNNS outcome are shown in , and are listed in Supplemental Table 1. REVIGO was utilized to visualize and interpret GO terms based on semantic similarity. None of the top 10 GO terms overlapped between these NNNS scores, but these terms exhibited several commonalities based on REVIGO semantic networks. Specifically, differential methylation of genes involved in sterol and cholesterol transport was associated with infant arousal. Based on semantic similarity, these GO terms were related to neurotransmitter uptake and synaptic transmission, which were associated with attention (). Additionally, infant attention was associated with differential methylation of genes in 3 pathways involving receptor internalization (). These GO terms can be traced back to the same common ancestor, the biological process of transport, which is broadly defined as the movement of substances within and between cells. We observed associations between methylation of genes involving cell projection organization and dendrite development and infant attention, and methylation of genes involving dendritic spine morphogenesis, cell organization projection, and cellular projection organization in relation to quality of movement. () These GO terms can be traced back to the common gene ontology ancestors of synaptic transmission and ultimately neurotransmitter transport. These biological processes are related to the development of the dendritic spine, which influences neuron development, and influences the development and function of the brain and nervous system.
Figure 4. Summary of the top 10 GO terms enriched among genes from the CpGs with highly significant correlations and NNNS scores, as visualized within cytoscape using REVIGO. GO terms are scaled to the log2 enrichment of that term. Terms in pink text represent those associated with attention, purple terms are associated with arousal, blue terms are associated with lethargy, and green terms are associated with quality of movement.
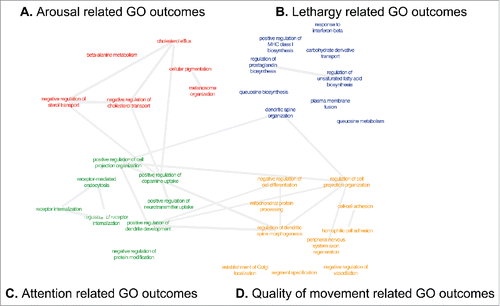
The overlap of CpGs whose differential methylation was associated with individual NNNS scores is displayed in . Overall, the vast majority of the CpGs whose methylation was associated with NNNS outcomes were unique to each outcome, with the exception of attention and lethargy, which had 284 overlapping CpGs. There were no CpGs in common across all 4 NNNS scores. On the other hand, at the gene level, there was greater overlap across the NNNS outcomes (). There were 51 genes whose methylation was associated with all 4 NNNS outcomes, and the full list of these genes can be found in Supplemental Table 2. Gene ontology analysis of these 51 genes identified over-representation of genes in pathways involving cellular adhesion and nervous system development ( and Supplemental Table 3). Several of these biological pathways were also specifically over-represented among genes whose methylation was associated with infant quality of movement.
Figure 5. (A) Venn diagrams of similarities between CpGs with highly significant correlations (P < 0.005) between multiple NNNS scores. (B) Similarities from genes from the CpGs with highly significant correlations and NNNS scores. (C) Word cloud of top 15 GO terms enriched among genes from the CpGs with highly significant correlations and NNNS scores across all 4 GO terms (51 genes). GO terms are scaled to the log2 enrichment of that term.
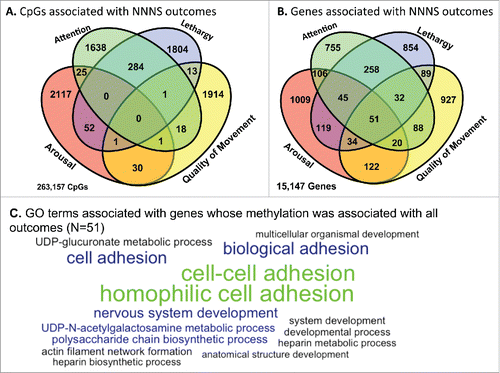
Discussion
We identified a statistically significant relationship between methylation of a CpG located within FHIT and newborn attention, and a negative relationship between methylation of ANKRD11 and infant attention scores. Genetic polymorphisms of these genes have been associated with attention related phenotypes,Citation32-34 and this study suggests that epigenetic variability of these genes may also contribute to an infant's ability to track visual and auditory stimuli. We observed increased DNA methylation in genes involved in biological pathways related to neurobehavior and cellular transport, which may be reflective of the influence of placental function on neurological outcomes.
Most tissues sampled for biomarker development, including the placenta, exhibit cellular heterogeneity, which can confound the association between the epigenetic biomarker and outcome. This study is among the first to implement statistical tools that adjust for cellular heterogeneity when performing comprehensive profiling assessments of methylation.Citation35 We observed that there were negligible changes in coefficient estimates in the model unadjusted for cellular heterogeneity and the final reference free model among CpGs who exhibited methylation associated with individual NNNS scores, suggesting that the underlying cellular heterogeneity did not significantly affect the relationship between methylation of the top CpGs and NNNS outcomes. We still cannot conclusively state that we are observing specific variation of DNA methylation within any one-cell type, but we can likely exclude cellular composition as a confounder at least in respect to our findings of CpGs associated with attention, lethargy, and quality of movement. Intriguingly, of the 4 NNNS scores we examined, only those CpGs that were significantly associated with infant arousal had significant changes in coefficient estimates after adjusting for cellular composition. This observation may suggest that differences in the underlying cellular composition present in the placenta may be related to infant arousal. It is unclear what types of changes would be represented by these specific genes, but future work should address if there are specific cells present in the placenta that exhibit differences in the methylation status of these genes.
Two CpGs within ANKRD11 and FHIT were significantly associated with attention. Low infant attention is characterized by little spontaneous interest in following a visual or auditory stimuli during the examination, and premature infants with low attention are more likely to be in a group of infants characterized by delays in motor concepts and language skills, lower IQ, and behavioral problems at age 3 in long-term study of high risk pregnancies.Citation28 In the 5′UTR of FHIT (fragile histidine triad region), cg15970800 exhibited a significant positive relationship between methylation and infant attention. This gene is a tumor suppressor that is located in a fragile section of the genome that is a frequent target of deletions and alterations.Citation36 Aberrant methylation of this gene has been linked to environmental exposures such as cigarette smoke.Citation37 Although the function of FHIT in fetal development remains unclear, it is expressed in the embryo over the course of development.Citation38 Copy number variation in this gene has been linked to autism spectrum disorder,Citation32 suggesting that alterations to this gene may play a role in neurological function.
On the other hand, we observed a significant negative relationship between methylation of a cg16710656 within the ANKRD11 gene body and infant attention. This gene encodes the ankryn repeat domain-containing protein, which acts as a nuclear co-regulator in the developing brain.Citation39 Regulation of this gene is implicated in neurodevelopment, as deletions of this gene have been associated with the developmental disorder KBG syndrome,Citation33 which is a rare developmental disease that results in physical abnormalities, developmental delays, and lifelong intellectual deficits.Citation40 Additionally, haplotype insufficiency of this gene has been associated with autistic features and cognitive impairment.Citation34 Further analysis is needed to understand the influence of genetic variation, copy number variation, and other mitigating factors along with DNA methylation in ANKRD11 and FHIT expression within the placenta. Mechanistic work beyond the scope of our analysis is necessary to understand the function of these genes within the placenta and how their variation underlies certain aspects of neurodevelopment.
In line with the distinctiveness of the 4 NNNS scores examined here and their unique underlying physiologies, we identified limited overlap of specific CpGs and genes and no overlap between the top 5 gene ontology terms across the 4 NNNS outcomes. This likely suggests that prenatal programming is occurring through unique genes and molecular pathways to regulate different aspects of neurobehavioral development. This is concurrent with other findings using the NNNS assessment, where different NNNS summary scores have been associated with unique and specific epigenetic patterningCitation10,11,13,41,42 or factors from the prenatal environment.Citation20-27
Quality of movement and infant attention were associated with methylation of genes involving neurological development and function, including neurotransmitter uptake, dopamine uptake involved in synaptic transmission, and dendritic spine organization. The placenta is an endocrine organ that expresses similar neuropeptides to the human brain,Citation43 and previous studies have identified associations between methylation of genes that are expressed within the human brain, such as the serotonin receptor HTR2A.Citation13 Animal models have revealed the importance of placental expression of genes regulating serotonin response on fetal brain development, as the placenta acts as a transient source of serotonin during development.Citation44 Several other genes for which placental methylation has been associated with infant health are also expressed in the brain, such as HTR2A,Citation45 NR3C1,Citation46 FKBP5,Citation47 and LEP.Citation48 Epigenetic regulation by the placenta may influence these important neuropeptides that direct the development and function of the fetal brain. Additionally, it is possible that the DNA methylation patterns in the placenta are indicative of those within the fetal brain, thus making the placenta a surrogate tissue. More work is needed to understand the parallels between epigenetic patterning, gene expression, and function of these genes, and the molecular pathways between the placenta and fetal brain.
The placenta is responsible for communicating between the mother and fetus through transport of nutrients, neuropeptides, and endocrine hormones. These processes occur through diffusion, transporter-mediated mechanisms, and endo/exocytosis.Citation49 NNNS outcomes were associated with methylation of genes involving different components of cellular transport. It remains unclear how alterations in placental transport influence the production, excretion, or transport of important neuropeptides and hormones from mother or placenta to fetus and the influence in their ultimate role in the developing fetal brain.
CpGs exhibiting differential methylation associated with lethargy and attention showed some overlap, which may be reflective of the shared influence of cognitive development involved in situational awareness and reactivity to the examination. Although there was no overlap between methylation of individual CpGs across all 4 NNNS outcomes, there was significant overlap at the gene level, suggesting that epigenetic patterning of these critical genes in different regions may influence multiple NNNS outcomes in a coordinated fashion. These overlapping genes included AUTS2, which is involved in neuronal development and linked to neurodevelopmental disorders,Citation50 and PCDHA, which traditionally localizes to synaptic junctions and is involved in neurogenesis, and is also implicated in neurodevelopmental disorders such as autism.Citation51 These genes were involved with molecular pathways involving cellular adhesion and nervous system development. The gene ontology (GO) keywords most associated with these genes were different from the gene ontology keywords that were most associated with individual NNNS outcomes with the exception of infant quality of movement, but the overall broad context involving cellular physiology and neurological processes remained consistent, reflecting the importance of these functions within the placenta and their relationship with postnatal neurological functions.
This study represents the first large-scale, comprehensive assessment of placental epigenetics and infant neurobehavior to date. These results should be interpreted with acknowledgment to the limitations of the current Illumina 450K methylation array. The probes on this array are not evenly spaced throughout the genome, and are enriched in specific regions. These regions do not align with DNA methylation sites commonly studied, such as NR3C1, as discussed in Weder et al.Citation52, or any sites previously examined in these infants. As a result of this, we were unable to validate previous associations identified between specific hypothesis-driven regions quantified through pyrosequencing and infant neurobehavior.Citation10-13,29,42 These regions are not represented completely by the Illumina array, as the 2 different technologies operate within their own niche.
We did not quantify the relationship between expression and methylation in these term placenta samples, as expression may represent only a cross-section of the gestational period, while we assume that methylation represents a more stable, and potentially long-term marker. For our pathway analyses, we elected to include variable regions of methylation based on a FDR correction in order to reduce our chances of making a type 1 error. There is still a chance we are finding associations solely due to chance because of the large number of comparisons being made. Further experimental analysis within placental tissue is required to understand how perturbation of these biological pathways alters placental physiology and function.
The NNNS assessment is a validated measure of many aspects of infant neurobehavior that can provide valuable information about early neurobehavioral development. However, there is limited understanding of the underlying changes in fetal brain physiology that produce differential NNNS scores, and more work is necessary to link NNNS outcomes to complex later life phenotypes such as anxiety and depression. Although the NNNS assessment is performed in the hospital to remove confounding postnatal environmental influences, there is also a possibility that the NNNS summary scores could be biased based on medical or other factors in the fetal environment immediately after birth. Our group has recently examined how various medical and demographic factors could influence performance on the NNNS within this healthy newborn population.Citation53
Although this study represents the largest, to date, to link newborn neurobehavior to placental methylation in a comprehensive fashion, the sample size is still limited, and does not afford opportunities to examine stratified effects, especially by sex, where they may be differential relationships of interest. We also recognize that generalizability of these findings may be effected by various underlying biases within our study population, including the oversampling for small and large for gestational infants, the somewhat limited representation of minority populations, and the focus on a healthy newborn population from mothers with limited prenatal exposures to tobacco, alcohol, or other drugs of abuse. We suggest that our findings should be examined among such populations in order to better understand if these results are consistent, or if there may be inflection points where these environmental or demographic factors might change the relationships we have observed.
The unique design of our study, the focus on the placenta, and our statistical analysis techniques strengthens the findings of our analysis in relation to similar epidemiological studies. As the placenta is the master regulator of the fetal environment, placental methylation may be a more relevant biomarker of the fetal environment than other tissues. Our results are also strengthened by our use of the NNNS assessment, which integrates multiple domains of neurobehavior, was designed to be comparable across multiple populations, and applicable to a broader research setting.Citation16 This assessment has established validity in identifying high-risk infants and predicting later life health outcomes.Citation17-28 The cohort examined in this study represents a relatively healthy population, and the maternal environment experienced by these infants is likely similar to the population at large. These results suggest that there is an underlying level of variation in behavioral phenotypes as well as a level of epigenetic variation in the placenta of otherwise healthy infants, and that in some cases the variation in the placenta is related to the variation observed in neurobehavioral outcomes. Importantly, our analyses suggest that transport and functions similar to those that occur within the developing central nervous system, which provides support for our hypothesis of a central role of the placenta in neurobehavioral development, and suggests that further studies should characterize these potential similarities. Our finding is also significant because many cases of developmental deficiency and mental illness cannot be traced to high-risk pregnancies, there is no clear-cut environmental factor driving this risk, and genetic variation alone has not explained the vast majority of pervasive developmental disorders or more common mental illnesses. Epigenetic variation as a mediator of these effects, or acting on its own, may be an additional mechanism to understand the biology underlying these conditions.
Materials and methods
Study population
The infants involved in this analysis (N = 335) represent a randomly selected subset of infants enrolled in the Rhode Island Child Health Study (RICHS) from September 2010 until February 2013 (N = 537) that completed the neurobehavioral assessments (NNNS) at birth. RICHS recruited mother-infant pairs from Women and Infants Hospital of Rhode Island. Newborns considered LGA (large for gestational age) and SGA (small for gestational age) were matched to AGA infants (adequate for gestational age) on sex, gestational age (±3 days), and maternal age (±2 years). Further Information about the cohort has been previously described.Citation12 All patients provided written informed consent for participation under protocols approved by the institutional review boards at Women and Infants Hospital and Dartmouth College. The subset examined for DNA methylation did not differ from the parent cohort in any key demographic factors including birth weight group, infant sex, or maternal age. The NNNS assessment was conducted after the first 24 hours of life but before hospital discharge. Placentas were collected within 2 hours of birth, with 3 samples taken from each of 4 quadrants, and placed immediately in RNALater. After at least 72 hours, samples were removed from RNALater, snap-frozen in liquid nitrogen, and homogenized to ensure heterogeneous sampling from all areas of the placenta.
DNA methylation assessments and preprocessing
DNA was isolated from placental tissue using DNeasy® blood and tissue kits (Qiagen, Valencia, CA) and bisulfite converted using the EZ DNA Methylation kit (Zymo, Irvine, CA). Epigenome wide DNA methylation at single nucleotide resolution using the Infinium HumanMethylation450 BeadChip (Illumina, San Diego, CA), which was processed at the Biomedical Genomics Center at the University of Minnesota. DNA methylation was extracted from the raw methylation files using the minfi package in R.Citation54 Poor quality probes that fell below the limit detection (P < 0.001) were removed from analysis (26,517 probes) and the data was adjusted for type 1 and type 2 probe variation using functional normalization.Citation55 Next, we adjusted for batch effects using the R package ComBAT.Citation56 This array produces β values, which represents a ratio of fluorescent signals of methylated versus unmethylated DNA at each CpG site. At this stage, probes that linked to the X or Y chromosome were removed (11,648 probes); polymorphic CpGs (95,012 probes), and cross hybridizing probesCitation57 (18,564 probes) were also removed from the data set. To improve the interpretability and increase analytic power, we removed all probes with a range of methylation across samples lower than 5% (65,503 probes), which was used as a cutoff in similar analyses.Citation58 The final data set consisted of 270,981 probes.
Statistical analysis
In order to model the relationship between methylation at each CpG and infant arousal, attention, lethargy, and arousal, linear regression was performed for each of the CpGs. Each model was adjusted for gender (male vs. female) birth weight group (average for gestational age vs. SGA and LGA) and cell type using the Ref Free EWAS package (Version 1.3).Citation59 The estimated dimension parameter for these analyses was 47. To determine the influence of the cell type correction on highly significant CpGs, we determined the difference between coefficient estimates in the unadjusted and adjusted model (Δ). The R package LIMMA was used to determine intersecting CpGs whose methylation was associated with individual NNNS outcomes at a threshold of P<0.005 and their associated genes.
To identify biological pathways between neurobehavioral outcomes and CpGs, we performed gene ontology analysis on genes represented by CpGs associated with NNNS outcomes at a threshold of P < 0.005. Gene ontology analysis was performed using the bioconductor package “GOseq”.Citation60 This package corrects for selection bias present in array data by calculating a probability weighing function, which weighs the chance of selecting a gene when forming a null distribution, which is generated through random sampling using the Wallenius distribution.Citation60 The GO category is tested for over or under representation among a series of differentially methylated genes and the null. The background used for creating this null distribution included the 19,361 genes represented in the initial array data used for the linear regression models (270,981 CpGs). We elected to use the term ontology biological pathways, which describes a series of events accomplished by one or more organized assemblies of molecular functions.Citation61 The results of GOSeq analysis were visualized and summarized using REVIGO,Citation62 which performs a clustering procedure to identify semantic associations between terms using a force directed layout algorithm, implemented within the cytoscape software environment.Citation63
We performed additional linear regression to examine the relationship between CpGs whose methylation most reliably was associated with neurobehavioral outcomes as defined as a false discovery rate lower than 0.05 at the Bonferroni threshold, which was more stringent than statistical cutoffs used in similar analyses.Citation58 NNNS assessments were normalized through z scoring, and β values multiplied by 100 to represent the percent of DNA methylated. We initially included maternal ethnicity (white, other), maternal age group (18–30 vs. 30-40), maternal anxiety and depression as reported through medical chart data (no vs. yes), and maternal obesity (BMI < 25 vs. BMI > 25) as covariates in the model, and used backward selection to remove these covariates because they were not significantly associated with NNNS scores and did not alter the estimates of effect of methylation on NNNS scores by greater than 10%. Fetal sex and birth weight groups were retained in the adjusted model. All data was analyzed in R version 3.1.1.
Disclosure of potential conflicts of interest
No potential conflicts of interest were disclosed.
KEPI_A_1195534_s02.zip
Download Zip (717.7 KB)Acknowledgments
The authors would like to thank the staff at Women and Infants Hospital, particularly Joyce Lee and Erica Oliveira for their help with the data collection, as well as Ashlee Roberts, Roshen John and Lauren Kwan for their assistance with biological processing and data entry. Additionally, we would like to thank Gregory Way and Kevin Johnson with their assistance with the data analysis and coding. This work is supported by NIH-NIMH R01MH094609, NIH-NIEHS R01ES022223 and NIH-NIEHS P01 ES022832/EPA RD83544201. Its contents are solely the responsibility of the grantee and do not necessarily represent the official views of the US EPA. Further, the US EPA does not endorse the purchase of any commercial products or services mentioned in the publication. The sponsors of this research had no role in study design, analysis and interpretation of data, in writing of the report or decision to submit the report for publication.
Funding
This work is supported by NIH-NIMH R01MH094609, NIH-NIEHS R01ES022223 and NIH-NIEHS P01 ES022832/EPA RD83544201
References
- Gluckman PD, Hanson MA, Beedle AS. Early life events and their consequences for later disease: a life history and evolutionary perspective. Am J Hum Biol 2007; 19:1-19; PMID:17160980; http://dx.doi.org/10.1002/ajhb.20590
- Jansson T, Powell TL. Role of placental nutrient sensing in developmental programming. Clin Obstet Gynecol 2013; 56:591-601; PMID:23703224; http://dx.doi.org/10.1097/GRF.0b013e3182993a2e
- Koukoura O, Sifakis S, Spandidos DA. DNA methylation in the human placenta and fetal growth (Review). Mol Med Rep 2012; 5:883-9; PMID:22294146; http://dx.doi.org/10.3892/mmr.2012.763
- Mesiano S. CHAPTER 11 - The Endocrinology of Human Pregnancy and Fetoplacental Neuroendocrine Development. In: Barbieri JFSL, ed. Yen & Jaffe's Reproductive Endocrinology (Sixth Edition). Philadelphia: W.B. Saunders, 2009:249-81; http://dx.doi.org/10.1016/B978-1-4557-2758-2.00012-3
- Misra DP, Salafia CM, Charles AK, Miller RK. Placental measurements associated with intelligence quotient at age 7 years. J Dev Orig Health Dis 2012; 3:190-7; PMID:25102009; http://dx.doi.org/10.1017/S2040174412000141
- Salafia CM, Yampolsky M, Misra DP, Shlakhter O, Haas D, Eucker B, Thorp J. Placental surface shape, function, and effects of maternal and fetal vascular pathology. Placenta 2010; 31:958-62; PMID:20933281; http://dx.doi.org/10.1016/j.placenta.2010.09.005
- Kulis M, Esteller M. DNA methylation and cancer. Adv Genet 2010; 70:27-56; PMID:20920744; http://dx.doi.org/10.1016/B978-0-12-380866-0.60002-2
- Kubota T, Miyake K, Hariya N, Mochizuki K. Understanding the epigenetics of neurodevelopmental disorders and DOHaD. J Dev Orig Health Dis 2015; 6:96-104; PMID:25708304; http://dx.doi.org/10.1017/S2040174415000057
- Lesseur C, Paquette AG, Marsit CJ. Epigenetic regulation of infant neurobehavioral outcomes. Med Epigenet 2014; 2:71-9; PMID:25089125; http://dx.doi.org/10.1159/000361026
- Appleton AA, Lester BM, Armstrong DA, Lesseur C, Marsit CJ. Examining the joint contribution of placental NR3C1 and HSD11B2 methylation for infant neurobehavior. Psychoneuroendocrinology 2014; 52c:32-42; PMID:25459891; http://dx.doi.org/10.1016/j.psyneuen.2014.11.004
- Bromer C, Marsit CJ, Armstrong DA, Padbury JF, Lester B. Genetic and epigenetic variation of the glucocorticoid receptor (NR3C1) in placenta and infant neurobehavior. Dev Psychobiol 2013; 55:673-83; PMID:22714792; http://dx.doi.org/10.1002/dev.21061
- Marsit CJ, Maccani MA, Padbury JF, Lester BM. Placental 11-β hydroxysteroid dehydrogenase methylation is associated with newborn growth and a measure of neurobehavioral outcome. PloS one 2012; 7:e33794; PMID:22432047; http://dx.doi.org/10.1371/journal.pone.0033794
- Paquette AG, Lesseur C, Armstrong DA, Koestler DC, Appleton AA, Lester BM, Marsit CJ. Placental HTR2A methylation is associated with infant neurobehavioral outcomes. Epigenetics 2013; 8:796-801; PMID:23880519; http://dx.doi.org/10.4161/epi.25358
- Paquette AG, Lester BM, Koestler DC, Lesseur C, Armstrong DA, Marsit CJ. Placental FKBP5 genetic and epigenetic variation is associated with infant neurobehavioral outcomes in the RICHS cohort. PloS One 2014; 9:e104913; PMID:25115650; http://dx.doi.org/10.1371/journal.pone.0104913
- Paquette AG, Lester BM, Lesseur C, Armstrong DA, Guerin DJ, Appleton AA, Marsit CJ. Placental epigenetic patterning of glucocorticoid response genes is associated with infant neurodevelopment. Epigenomics 2015; 7(5):767-79; PMID:26343289; http:dx.doi.org/10.2217/epi.15.28
- Tronick E, Lester BM. Grandchild of the NBAS: The NICU network neurobehavioral scale (NNNS) a review of the research using the NNNS. J Child Adolesc Psychiatr Nurs 2013; 26:193-203; PMID:23909942; http://dx.doi.org/10.1111/jcap.12042
- Sucharew H, Khoury JC, Xu Y, Succop P, Yolton K. NICU Network Neurobehavioral Scale profiles predict developmental outcomes in a low-risk sample. Paediatr Perinat Epidemiol 2012; 26:344-52; PMID:22686386; http://dx.doi.org/10.1111/j.1365-3016.2012.01288.x
- El-Dib M, Massaro AN, Glass P, Aly H. Neurobehavioral assessment as a predictor of neurodevelopmental outcome in preterm infants. J Perinatol 2012; 32:299-303; PMID:21760584; http://dx.doi.org/10.1038/jp.2011.100
- Barros MC, Mitsuhiro S, Chalem E, Laranjeira RR, Guinsburg R. Neurobehavior of late preterm infants of adolescent mothers. Neonatology 2011; 99:133-9; PMID:20733334; http://dx.doi.org/10.1159/000313590
- Stephens BE, Liu J, Lester B, Lagasse L, Shankaran S, Bada H, Bauer C, Das A, Higgins R. Neurobehavioral Assessment Predicts Motor Outcome in Preterm Infants. J Pediatr 2010; 156:366-71; PMID:19880137; http://dx.doi.org/10.1016/j.jpeds.2009.09.042
- Smith JR, McGrath J, Brotto M, Inder T. A randomized-controlled trial pilot study examining the neurodevelopmental effects of a 5-week M Technique intervention on very preterm infants. Adv Neonatal Care 2014; 14:187-200; PMID:24858669; http://dx.doi.org/10.1097/ANC.0000000000000093
- Montirosso R, Del Prete A, Bellu R, Tronick E, Borgatti R. Level of NICU quality of developmental care and neurobehavioral performance in very preterm infants. Pediatrics 2012; 129:e1129-37; PMID:22492762; http://dx.doi.org/10.1542/peds.2011-0813
- Conradt E, Sheinkopf SJ, Lester BM, Tronick E, LaGasse LL, Shankaran S, Bada H, Bauer CR, Whitaker TM, Hammond JA. Prenatal substance exposure: neurobiologic organization at 1 month. J Pediatr 2013; 163:989-94.e1; PMID:23743094; http://dx.doi.org/10.1016/j.jpeds.2013.04.033
- Smith LM, Paz MS, LaGasse LL, Derauf C, Newman E, Shah R, Arria A, Huestis MA, Haning W, Strauss A, et al. Maternal depression and prenatal exposure to methamphetamine: neurodevelopmental findings from the infant development, environment, and lifestyle (ideal) study. Depress Anxiety 2012; 29:515-22; PMID:22555777; http://dx.doi.org/10.1002/da.21956
- Jones HE, O'Grady KE, Johnson RE, Velez M, Jansson LM. Infant neurobehavior following prenatal exposure to methadone or buprenorphine: results from the neonatal intensive care unit network neurobehavioral scale. Subst Use Misuse 2010; 45:2244-57; PMID:20482340; http://dx.doi.org/10.3109/10826084.2010.484474
- Coleman MB, Glass P, Brown J, Kadom N, Tsuchida T, Scafidi J, Chang T, Vezina G, Massaro AN. Neonatal neurobehavioral abnormalities and MRI brain injury in encephalopathic newborns treated with hypothermia. Early Hum Dev 2013; 89:733-7; PMID:23787090; http://dx.doi.org/10.1016/j.earlhumdev.2013.05.006
- Marcus S, Lopez JF, McDonough S, Mackenzie MJ, Flynn H, Neal CR, Jr., Gahagan S, Volling B, Kaciroti N, Vazquez DM. Depressive symptoms during pregnancy: impact on neuroendocrine and neonatal outcomes. Infant Behav Dev 2011; 34:26-34; PMID:21035873; http://dx.doi.org/10.1016/j.infbeh.2010.07.002
- Liu J, Bann C, Lester B, Tronick E, Das A, Lagasse L, Bauer C, Shankaran S, Bada H. Neonatal neurobehavior predicts medical and behavioral outcome. Pediatrics 2010; 125:e90-8; PMID:19969621; http://dx.doi.org/10.1542/peds.2009-0204
- Lesseur C, Armstrong DA, Murphy MA, Appleton AA, Koestler DC, Paquette AG, Lester BM, Marsit CJ. Sex-specific associations between placental leptin promoter DNA methylation and infant neurobehavior. Psychoneuroendocrinology 2014; 40:1-9; PMID:24485470; http://dx.doi.org/10.1016/j.psyneuen.2013.10.012
- Gavin NI, Gaynes BN, Lohr KN, Meltzer-Brody S, Gartlehner G, Swinson T. Perinatal depression: a systematic review of prevalence and incidence. Obstet Gynecol 2005; 106:1071-83; PMID:16260528; http://dx.doi.org/10.1097/01.AOG.0000183597.31630.db
- Fink NS, Tronick E, Olson K, Lester B. Healthy newborns' neurobehavior: norms and relations to medical and demographic factors. J Pediatr 161:1073-9.e3; PMID:22727876; http://dx.doi.org/10.1016/j.jpeds.2012.05.036
- Girirajan S, Dennis MY, Baker C, Malig M, Coe BP, Campbell CD, Mark K, Vu TH, Alkan C, Cheng Z, et al. Refinement and discovery of new hotspots of copy-number variation associated with autism spectrum disorder. Am J Hum Genet 2013; 92:221-37; PMID:23375656; http://dx.doi.org/10.1016/j.ajhg.2012.12.016
- Sirmaci A, Spiliopoulos M, Brancati F, Powell E, Duman D, Abrams A, Bademci G, Agolini E, Guo S, Konuk B, et al. Mutations in ANKRD11 Cause KBG Syndrome, Characterized by Intellectual Disability, Skeletal Malformations, and Macrodontia. Am J Hum Genet 2011; 89:289-94; PMID:21782149; http://dx.doi.org/10.1016/j.ajhg.2011.06.007
- Isrie M, Hendriks Y, Gielissen N, Sistermans EA, Willemsen MH, Peeters H, Vermeesch JR, Kleefstra T, Van Esch H. Haploinsufficiency of ANKRD11 causes mild cognitive impairment, short stature and minor dysmorphisms. Eur J Hum Genet 2012; 20:131-3; PMID:21654729; http://dx.doi.org/10.1038/ejhg.2011.105
- Houseman EA, Molitor J, Marsit CJ. Reference-free cell mixture adjustments in analysis of DNA methylation data. Bioinformatics 2014; 30:1431-9; PMID:24451622; http://dx.doi.org/10.1093/bioinformatics/btu029
- Sard L, Accornero P, Tornielli S, Delia D, Bunone G, Campiglio M, Colombo MP, Gramegna M, Croce CM, Pierotti MA, et al. The tumor-suppressor gene FHIT is involved in the regulation of apoptosis and in cell cycle control. Proc Natl Acad Sci U S A 1999; 96:8489-92; PMID:19514467; http://dx.doi.org/10.1073/pnas.96.15.8489
- Kim JS, Kim H, Shim YM, Han J, Park J, Kim DH. Aberrant methylation of the FHIT gene in chronic smokers with early stage squamous cell carcinoma of the lung. Carcinogenesis 2004; 25:2165-71; PMID:15231689; http://dx.doi.org/10.1093/carcin/bgh217
- Falvella FS, Menegola E, Giavini E, Ottey M, Fidanza V, Croce CM, Huebner K, Dragani TA. Expression of Fhit protein during mouse development. Anat Rec 2000; 260:208-11; PMID:10993957; http://dx.doi.org/10.1002/1097-0185(20001001)260:2%3c208::AID-AR100%3e3.0.CO;2-0
- Gallagher D, Voronova A, Zander Mark A, Cancino Gonzalo I, Bramall A, Krause Matthew P, Abad C, Tekin M, Neilsen Paul M, Callen David F, et al. Ankrd11 Is a Chromatin Regulator Involved in Autism that Is Essential for Neural Development. Dev Cell 2015; 32:31-42; PMID:25556659; http://dx.doi.org/10.1016/j.devcel.2014.11.031
- Herrmann J, Pallister PD, Tiddy W, Opitz JM. The KBG syndrome-a syndrome of short stature, characteristic facies, mental retardation, macrodontia and skeletal anomalies. Birth Defects Orig Artic Ser 1975; 11:7-18; PMID:1218237
- Appleton AA, Armstrong DA, Lesseur C, Lee J, Padbury JF, Lester BM, Marsit CJ. Patterning in Placental 11-B Hydroxysteroid Dehydrogenase Methylation According to Prenatal Socioeconomic Adversity. PloS One 2013; 8:e74691; PMID:24040322; http://dx.doi.org/10.1371/journal.pone.0074691
- Conradt E, Lester BM, Appleton AA, Armstrong DA, Marsit CJ. The roles of DNA methylation of NR3C1 and 11beta-HSD2 and exposure to maternal mood disorder in utero on newborn neurobehavior. Epigenetics 2013; 8:1321-9; PMID:24135662; http://dx.doi.org/10.4161/epi.26634
- Hsiao EY, Patterson PH. Placental regulation of maternal-fetal interactions and brain development. Dev Neurobiol 2012; 72:1317-26; PMID:22753006; http://dx.doi.org/10.1002/dneu.22045
- Bonnin A, Goeden N, Chen K, Wilson ML, King J, Shih JC, Blakely RD, Deneris ES, Levitt P. A transient placental source of serotonin for the fetal forebrain. Nature 2011; 472:347-50; PMID:21512572; http://dx.doi.org/10.1038/nature09972
- Pazos A, Cortes R, Palacios JM. Quantitative autoradiographic mapping of serotonin receptors in the rat brain. II. Serotonin-2 receptors. Brain Res 1985; 346:231-49; PMID:4052777; http://dx.doi.org/10.1016/0006-8993(85)90857-1
- Cao-Lei L, Suwansirikul S, Jutavijittum P, Meriaux SB, Turner JD, Muller CP. Glucocorticoid receptor gene expression and promoter CpG modifications throughout the human brain. J Psychiatr Res 2013; 47:1597-607; PMID:23948638; http://dx.doi.org/10.1016/j.jpsychires.2013.07.022
- Scharf SH, Liebl C, Binder EB, Schmidt MV, Muller MB. Expression and regulation of the Fkbp5 gene in the adult mouse brain. PloS One 2011; 6:e16883; PMID:21347384; http://dx.doi.org/10.1371/journal.pone.0016883
- Morash B, Li A, Murphy PR, Wilkinson M, Ur E. Leptin gene expression in the brain and pituitary gland. Endocrinology 1999; 140:5995-8; PMID:10579368http://dx.doi.org/10.1210/endo.140.12.7288
- Burton GJ, Fowden AL. The placenta: a multifaceted, transient organ. Philos Trans R Soc Lond B Biol Sci 2015; 370:20140066; PMID:25602070; http://dx.doi.org/10.1098/rstb.2014.0066,53:10.1111/ppe.12294
- Oksenberg N, Ahituv N. The role of AUTS2 in neurodevelopment and human evolution. Trends Genet 2013; 29:600-8; PMID:24008202; http://dx.doi.org/10.1016/j.tig.2013.08.001
- Anitha A, Thanseem I, Nakamura K, Yamada K, Iwayama Y, Toyota T, Iwata Y, Suzuki K, Sugiyama T, Tsujii M, et al. Protocadherin α (PCDHA) as a novel susceptibility gene for autism. J Psychiatry Neurosci 2013; 38:192-8; PMID:23031252; http://dx.doi.org/10.1503/jpn.120058
- Weder N, Zhang H, Jensen K, Yang BZ, Simen A, Jackowski A, Lipschitz D, Douglas-Palumberi H, Ge M, Perepletchikova F, et al. Child abuse, depression, and methylation in genes involved with stress, neural plasticity, and brain circuitry. J Am Acad Child Adolesc Psychiatry 2014; 53:417-24.e5; PMID:24655651; http://dx.doi.org/10.1016/j.jaac.2013.12.025
- Appleton AA, Murphy MA, Koestler DC, Lesseur C, Paquette AG, Padbury JF, Lester BM, Marsit CJ. Prenatal Programming of Infant Neurobehaviour in a Healthy Population. Paediatr perinat Epidemiol 2016; 30(4):367-75; PMID:27004434
- Aryee MJ, Jaffe AE, Corrada-Bravo H, Ladd-Acosta C, Feinberg AP, Hansen KD, Irizarry RA. Minfi: a flexible and comprehensive Bioconductor package for the analysis of Infinium DNA methylation microarrays. Bioinformatics 2014; 30:1363-9; PMID:24478339; http://dx.doi.org/10.1093/bioinformatics/btu049
- Fortin J-P, Labbe A, Lemire M, Zanke BW, Hudson TJ, Fertig EJ, Greenwood CM, Hansen KD. Functional normalization of 450k methylation array data improves replication in large cancer studies. Genome Biol 2014; 15:503; PMID:25599564; http://dx.doi.org/10.1186/s13059-014-0503-2
- Johnson WE, Li C, Rabinovic A. Adjusting batch effects in microarray expression data using empirical Bayes methods. Biostatistics 2007; 8:118-27; PMID:16632515; http://dx.doi.org/10.1093/biostatistics/kxj037
- Chen YA, Lemire M, Choufani S, Butcher DT, Grafodatskaya D, Zanke BW, Gallinger S, Hudson TJ, Weksberg R. Discovery of cross-reactive probes and polymorphic CpGs in the Illumina Infinium HumanMethylation450 microarray. Epigenetics 2013; 8:203-9; PMID:23314698; http://dx.doi.org/10.4161/epi.23470
- Essex MJ, Boyce WT, Hertzman C, Lam LL, Armstrong JM, Neumann SMA, Kobor MS. Epigenetic Vestiges of Early Developmental Adversity: Childhood Stress Exposure and DNA Methylation in Adolescence. Child Dev 2013; 84:58-75; PMID:21883162; http://dx.doi.org/10.1111/j.1467-8624.2011.01641.x
- Houseman EA, Molitor J, Marsit CJ. Reference-free cell mixture adjustments in analysis of DNA methylation data. Bioinformatics 2014; 30:1431-9; PMID:24451622; http://dx.doi.org/10.1093/bioinformatics/btu029
- Young MD, Wakefield MJ, Smyth GK, Oshlack A. Method Gene ontology analysis for RNA-seq: accounting for selection bias. Genome Biol 2010; 11:R14; PMID:20132535; http://dx.doi.org/10.1186/gb-2010-11-2-r14
- Consortium TGO. Gene Ontology Consortium: going forward. Nucleic Acids Res 2015; 43:D1049-D56; PMID:25428369; http://dx.doi.org/10.1093/nar/gku1179
- Supek F, Bošnjak M, Škunca N, Šmuc T. REVIGO Summarizes and Visualizes Long Lists of Gene Ontology Terms. PloS One 2011; 6:e21800; PMID:21789182; http://dx.doi.org/10.1371/journal.pone.0021800
- Shannon P, Markiel A, Ozier O, Baliga NS, Wang JT, Ramage D, Amin N, Schwikowski B, Ideker T. Cytoscape: a software environment for integrated models of biomolecular interaction networks. Genome Res 2003; 13:2498-504; PMID:14597658; http://dx.doi.org/10.1101/gr.1239303