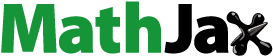
ABSTRACT
Although there is growing evidence that exposure to ambient particulate matter is associated with global DNA methylation and gene-specific methylation, little is known regarding epigenome-wide changes in DNA methylation in relation to particles and, especially, particle components. Using the Illumina Infinium HumanMethylation450 BeadChip, we examined the relationship between one-year moving averages of PM2.5 species (Al, Ca, Cu, Fe, K, Na, Ni, S, Si, V, and Zn) and DNA methylation at 484,613 CpG probes in a longitudinal cohort that included 646 subjects. Bonferroni correction was applied to adjust for multiple comparisons. Bioinformatics analysis of the Kyoto Encyclopedia of Genes and Genomes (KEGG) pathway enrichment was also performed. We observed 20 Bonferroni significant (P-value < 9.4× 10−9) CpGs for Fe, 8 for Ni, and 1 for V. Particularly, methylation at Schlafen Family Member 11 (SLFN11) cg10911913 was positively associated with measured levels of all 3 species. The SLFN11 gene codes for an interferon-induced protein that inhibits retroviruses and sensitizes cancer cells to DNA-damaging agents. Bioinformatics analysis suggests that gene targets may be relevant to pathways including cancers, signal transduction, and cell growth and death. Ours is the first study to examine the epigenome-wide association between ambient particles species and DNA methylation. We found that long-term exposures to specific components of ambient particle pollution, especially particles emitted during oil combustion, were associated with methylation changes in genes relevant to immune responses. Our findings provide insight into potential biologic mechanisms on an epigenetic level.
Introduction
The associations between fine particulate matter (particles with an aerodynamic size of ≤ 2.5 µm, PM2.5) and health outcomes are well established. It is estimated that PM2.5 leads to 3.15 million premature deaths per year on a global scale.Citation1 Nationwide and multiple-community studies report that exposure to PM2.5 is linked with an increased risk of hospital admissions and mortality in the US, and that certain PM2.5 species may be more toxic than others.Citation2–5
DNA methylation is an epigenetic process in which a methyl group is added to deoxycytosine bases to form deoxymethylcytosine. The literature to date indicates that DNA methylation may be an important pathway linking particulate matter (PM) to health outcomes.Citation6–10 For example, changes in patterns of DNA methylation may be associated with processes leading to cardiovascular diseases..Citation11,12 There is growing evidence that exposure to PM is associated with global DNA methylation and gene-specific methylation,Citation6,7,13,14 especially for exposure to metal-rich PM in occupational settings.Citation15,16 Yet, little is known regarding how PM exposure affects DNA methylation on a genome-wide level.
Measurements of DNA methylation at cytosine-guanine dinucleotide (CpG) loci have recently become available; for instance, the Illumina Infinium HumanMethylation450 BeadChip (450K) measures methylation at more than 450,000 CpG sites. This makes an epigenome-wide association study of the relationship between particles and DNA methylation possible. Several studies have investigated the epigenome-wide association between smoking, an important PM source, and DNA methylation. Using the 450K platform and Bonferroni correction, Joubert et al.Citation17 identified 26 CpGs with methylation changes in newborns related to maternal smoking during pregnancy in a large Norwegian birth cohort. Specifically, the authors documented changes at CpGs in cytochrome p450 1A1 (CYP1A1) and aryl-hydrocarbon receptor repressor (AHRR), genes known to play a key role in the aryl hydrocarbon receptor (AhR) signaling pathway that mediates the detoxification of components of tobacco smoke. In a more recent study, the authors identified numerous loci associated with maternal smoking during pregnancy in a genome-wide consortium meta-analysis and found substantial persistence of such effects in later childhood.Citation18 Zeilinger et al.Citation19 conducted an epigenome-wide study comparing methylation levels among current, former, and never smokers in a population-based panel, and found widespread differences in the degree of site-specific methylation as a function of tobacco smoking. Moreover, they observed that the most significant associations with smoking were for DNA methylation sites in AHRR.
To date, very few epigenome-wide association studies have focused on exposures to ambient particles, with the exception of one recent study that identified several CpG sites associated with cumulative exposure to ambient particles among populations from Germany and the US.Citation20 To our knowledge, no epigenome-wide association studies have investigated the toxicity of specific PM species. Such research would be of great importance because epigenome-wide association research based on DNA methylation microarrays could provide insight into epigenetic mechanisms underlying associations between air particles and health outcomes and thereby improve our understanding of human disease. To our knowledge, our study is the first to examine epigenome-wide associations between PM2.5 species and DNA methylation using data from a large longitudinal cohort.
Methods
Study population
Study subjects were participants of the Normative Aging Study (NAS), a closed cohort that was established in 1963 by the Department of Veterans Affairs. This ongoing longitudinal study of aging enrolled 2,280 community-dwelling, healthy men living in the Greater Boston area.Citation21 Participants were free of known chronic medical conditions at enrollment and have undergone examinations every 3 to 5 y on a continuous rolling basis. All participants provided written informed consent. The study was reviewed and approved by the Institutional Review Boards of all participating institutions.
We restricted our analysis to study visits with DNA samples, which were collected from subjects starting in 1999. A comparison of visits with DNA samples and visits without showed no substantial differences with regard to body mass index, education, alcohol consumption, and smoking, although older participants were less likely to provide DNA samples (mean age 74.5 vs. 76.3 years; P-value of t-test in mean age < 0.05). In addition, since Caucasians comprise most of our study population (approximately 97%), we excluded non-Caucasians to reduce study heterogeneity that may be introduced by diverse genetic ancestry. We also excluded observations that did not have complete information on the exposures or covariates of interest. In the final analyses, samples from 646 subjects with 1,031 total visits were included. Of the 646 subjects, 314 presented for one visit, 279 presented for 2 visits, and 53 presented for 3 visits. The flowchart of our study participants is shown in .
DNA methylation
DNA samples were collected between 1999 and 2013. We used the QIAamp DNA Blood Kit (Qiagen, CA, USA) to extract DNA from buffy coat, and performed bisulfite conversion with the EZ-96 DNA Methylation Kit (Zymo Research, CA, USA). To minimize batch effects, we randomized chips across plates and randomized samples based on a 2-stage age stratified algorithm so that age distributed similarly across chips and plates.
We measured DNA methylation of CpG probes using Illumina Infinium HumanMethylation450 BeadChip.Citation22 Quality control analysis was performed, where samples with >1% of probes with a detection P-value > 0.05 were removed, and probes with P-value > 5% samples with a bead count < 3 or with >1% samples with a detection P-value > 0.05 were removed. The remaining samples were preprocessed using the Illumina-type background correctionCitation23 and normalized with dye-biasCitation24 and BMIQ3 adjustments,Citation25 which were used to generate methylation values. Extreme outliers, defined by Tukey's method (i.e., <25th percentile – 3 × IQR or > 75th percentile + 3 × IQR), were trimmed. As a result, 484,613 CpG probes were included in the working set.
We used the β value to indicate methylation level at each CpG site. The β value represents the ratio of the fluorescence of the methylated signal to the combined methylated and unmethylated signals; that is, β = intensity of the methylated signal (M) / [intensity of the unmethylated signal (U) + intensity of the methylated signal (M) + 100].Citation22
The methylation data has been uploaded to the database of Genotypes and Phenotypes (dbGaP; available at https://www.ncbi.nlm.nih.gov/gap) established by the National Institutes of Health, along with some other basic information (dbGaP accession phs000853.v1.p1).
Air pollution
We measured daily concentrations of PM2.5 mass and species at the stationary monitoring supersite at the Harvard University Countway Library, Boston, MA, USA. PM2.5 was assessed by the Tapered Element Oscillating Microbalance (TEOM 1400a, Rupprecht & Patashnick Co.), and PM species were assessed using the Energy Dispersive X-ray Fluorescence Spectrometer (Epsilon 5, PANalytical).
To estimate long-term effects of air pollution on DNA methylation, we calculated one-year moving average concentrations for PM2.5 and the following 11 species: Al, Ca, Cu, Fe, K, Na, Ni, S, Si, V, and Zn. These species are representative of different sources of particles: Al, Ca, Fe, and Si are major components of road dust; Cu and Zn originate from traffic emissions; Ni and V are tracers of oil combustion; S is a regional pollutant that is associated with coal combustion and to a lesser extent with metallurgic activities; Na is a major component of sea salt particles; K originates from wood burning and soil.
Statistical analysis
For each specific CpG site, we applied a linear mixed-effects model as described below,(1)
(1) where Yij is the methylation level of subject i at visit j, Specij and PM2.5ij are one-year moving average species and PM2.5 concentrations before visit j, Ageij is subject i's age at visit j (this notation is relevant to all other covariates, since we used values assessed at each visit in our analyses), and µi is the random intercept that accounts for correlation within subject. The full formula can be found in the Supplemental Document.
In the analysis, we controlled for the following covariates a priori based on the relevant literature:Citation6,19,26,27 total PM2.5 mass, cell proportions (CD4+ T lymphocytes, CD8+ T lymphocytes, natural killer cells, B cells, monocytes) estimated by the Houseman et al. method,Citation28–30 age, body mass index [BMI, computed as weight (in kilograms) divided by height (in square meters)], cigarette pack years, smoking status (never, ever), alcohol consumption (≥ 2 drinks/day, < 2 drinks/day), years of education, year and season (spring: March–May, summer: June–August, fall: September–November, winter: December–February). Potential batch effects were also considered, including plate, the position of the chip on plate, and the row and column position on the chip.
Since overall type I error inflates as the number of statistical tests increases, we used Bonferroni correction to adjust for multiple comparisons. This method, which accounts for the number of CpG sites and exposures tested, has been widely used in similar studies.Citation31–33 As a result, the adjusted level of significance in the current study was P-value = 9.4× 10−9, i.e., 0.05 / (484,613× 11). After identifying significant CpGs, we further checked whether they were non-unimodal in the dip test,Citation34 were cross-reactive,Citation35 or were located within 10 bases of SNPs relevant to European populations.Citation36
Our study population was a heavily smoking population. Since we controlled for smoking status and pack years in the analysis, we believe there was sufficient adjustment, but we understand that the presence of a large number of smokers may obscure or distort associations. To address this, we have performed subset analyses among nonsmokers and smokers.
All statistical analyses were performed using R 3.1.2.Citation37
Bioinformatics analysis
We performed Kyoto Encyclopedia of Genes and Genomes (KEGG) pathway analysisCitation38 using the genes targets of CpGs, identified by the Illumina annotation assignment, with Benjamini-Hochberg false discovery rates (FDR)Citation39 <1% and <5%, respectively. Bioinformatics analysis was performed using Database for Annotation, Visualization and Integrated Discovery (DAVID) Bioinformatics Resources 6.7.Citation31,32 Briefly, DAVID consists of an integrated biologic knowledgebase with wide annotation content coverage. It allowed us to identify the most relevant pathways associated with the gene lists we provided.
Results
Characteristics of study subjects are presented in . At baseline, the mean age was 74 y [standard deviation (SD) = 7]. The study population was well educated, with a mean of 15 y of education (SD = 3). Furthermore, 186 of the 646 (28.8%) individuals never smoked, and 131 (20.3%) had 2 or more drinks per day.
Table 1. Characteristics of the study population.
shows the descriptive summary of concentrations of PM2.5 mass and species. During the study period 1,999 – 2,013, the annual average PM2.5 concentration was 10.5 (SD = 1.1) µg/m3. Among the examined species, S accounted for the largest mass proportion (10%). The oil combustion tracers, Ni and V, accounted for 0.03% and 0.04% of PM2.5 mass with mean concentrations of 0.0037 (SD = 0.0013) µg/m3 and 0.0041 (SD = 0.0012) µg/m3, respectively. We report a strong correlation between Ni and V (r = 0.96).
Table 2. Summary of one-year moving averages of PM2.5 mass and species across all study visits (year 1999–2013).
presents CpGs that reached Bonferroni-corrected significance (P-value < 9.4 × 10−9) in relation to PM2.5 species. After excluding CpGs that were non-unimodal in the dip test, were cross-reactive, or contained SNPs within 10 bases, we observed 20 significant CpGs for Fe, 8 for Ni, and 1 for V. Particularly, Schlafen Family Member 11 (SLFN11) cg10911913 was significant for all 3 metals. The ratio of methylation at this CpG site is expected to increase by 0.073, 0.031, and 0.044 per IQR increase in the one-year moving averages of Fe, Ni, and V, respectively. We found strong seasonal patterns at this site for the 3 metals (Figure S1 in the Supplemental Material), with the highest methylation levels in the spring. We also found that methylation levels at CASZ1 cg16238819, CUEDC2 cg06024834, ONECUT1 cg15446043, and FOXO4 cg12453500 were related to both Fe and Ni. To illustrate the epigenome-wide association between PM2.5 species and methylation, we plotted the -log10(P-value) from the mixed-effects models for all the 484,613 CpG sites in . The horizontal line represents the Bonferroni-corrected significance threshold. Subset analyses among nonsmokers and smokers showed consistent associations between PM species and methylation within smoking subgroups. For example, the coefficients for Fe at our most significant CpG (cg10911913) were 0.122 overall (Table S1 in the Supplemental Document), 0.131 among smokers and 0.086 among nonsmokers, and were statistically significant at a genome-wide level for both smoking and non-smoking subgroups. We observed the same trend for Ni and V.
Table 3. Genome-wide associations of one-year moving averages of PM2.5 species with DNA methylation: CpGs with Bonferroni-corrected statistical significance (P-value < 9.4× 10−9).
Figure 2. Genome-wide associations of one-year moving averages of PM2.5 species with DNA methylation, displayed by Manhattan plots. The horizontal line represents the significance threshold with Bonferroni correction (P-value < 9.4 × 10−9). We originally found 22 significant CpGs for Fe, 9 for Ni, and 1 for V, as shown in . After excluding CpGs that were non-unimodal in the dip test, were cross-reactive, or contained SNPs in the last 10 bases, 20 CpGs remained significantly associated with Fe, 8 with Ni, and 1 with V.
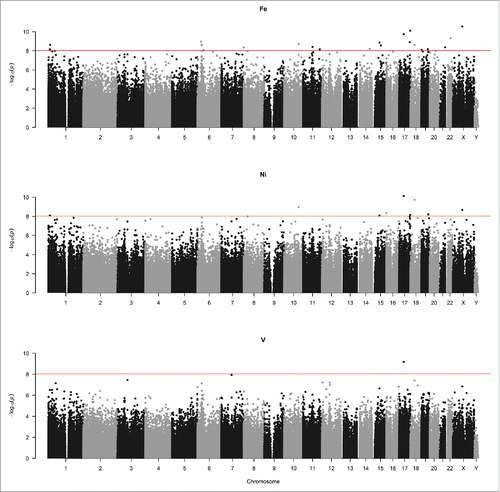
Results of the bioinformatics analysis are shown in . We conducted KEGG pathway enrichment analysis for gene targets of CpGs with FDR <1% and <5%, respectively. Pathways whose enrichment FDRs were less than 5% were considered significant. In the analysis for gene targets of CpGs with FDR <1%, we identified 9 significant KEGG pathways. Three of them (i.e., “pathways in cancer,” “non-small cell lung cancer,” and “glioma”) belong to the “Cancers” network. Results for gene targets of CpGs with FDR <5% suggest that those genes may also be linked to other cancers (e.g., myeloid leukemia) and pathways in the immune system. Other identified pathways include the diabetes and mitogen-activated protein kinases (MAPK) signaling pathways; the latter is involved in cellular functions including cell proliferation, differentiation, and migration.
Discussion
In this study, we investigated the relationship between PM2.5 species and epigenome-wide DNA methylation in a large longitudinal cohort of elderly men. To the best of our knowledge, this is the first study that examined epigenome-wide associations between ambient PM2.5 components and DNA methylation. We adjusted for multiple tests for the 11 PM components and 484,613 CpGs analyzed. We observed that 20 CpGs met the conservative Bonferroni-corrected level of significance (P-value < 9.4 × 10−9) in the analysis for Fe, 8 for Ni, and 1 for V. Fe mainly originates from crustal sources and the iron and steel industry; Ni and V are by-products of oil combustion, whereas Ni is also associated with the iron and steel industry.Citation40 The strong correlations between these species are shown in ; the correlation coefficients were 0.93 for Fe-Ni, 0.86 for Fe-V, and 0.96 for Ni-V. In addition, we found that all 3 metals were associated with methylation levels at cg10911913 within the SLFN11 gene. Our bioinformatics analysis suggests that gene targets of CpGs with FDR < 1% may be relevant to pathways including cancers, signal transduction, and cell growth and death.
To date, only a limited number of research studies have examined the association of ambient particles with DNA methylation. Most of them used surrogates for global DNA methylation, such as methylation at repetitive elements, or focused on methylation in candidate genes. The main shortcomings of these studies are: 1) methylation levels measured by surrogates do not consider the location in the genome and the patterns of methylation at surrogates may not well represent the patterns of gene-specific methylation, and 2) the use of candidate genes can only reveal methylation changes in particular genes selected a priori and may miss the genes that exhibit the most profound effects.
One previous study regarding PM exposure and DNA methylation reported that exposures to 90-day moving averages of black carbon and sulfate particles concentrations were associated with hypomethylation of the long interspersed nucleotide element-1 (LINE-1) and the short interspersed nucleotide element Alu.Citation6 Baccarelli et al.Citation41 found decreased LINE-1 methylation in association with 7-day moving average black carbon and PM2.5 levels, whereas no association was observed for Alu methylation. A controlled human exposure experiment observed hypomethylation of Alu and the toll-like receptor 4 (TLR4) gene among 15 healthy adults exposed to fine concentrated ambient particles (CAPs) for 130 min.Citation13 Recently, a longitudinal study reported a relationship between decreased methylation at 3 CpG loci located in the nitric oxide synthase 2, inducible (NOS2A) gene and short-term exposures to organic carbon, elemental carbon, NO3−, and NH4+ among 28 patients with chronic obstructive pulmonary disease.Citation7
A more recent epigenome-wide study by Panni et al. on short- and mid-term PM2.5 exposure among 3 independent cohorts—the US NAS cohort and the German KORA F3 and F4 cohorts—identified 12 CpG sites at a Bonferroni adjusted significance level.Citation20 Methylation at 9 out of these 12 CpGs showed increases related to PM exposure. This trend was consistent with our study: we found that 27 out of the 29 associations across all species displayed increased methylation levels. However, our study did not identify the same CpGs. A possible explanation for this difference is that the previous study evaluated short- or medium-term effects of PM, whereas we focused on long-term exposures. In addition, the results of the previous study were from a meta-analysis of 3 cohorts, including our study population. The 2 German cohorts had a much larger sample size than the NAS cohort (2299 vs. 657 participants), and the Panni et al. study reported heterogeneity at some CpG sites across the cohorts. Hence, their findings were more likely to reveal methylation changes within the German population and cannot be generalized to the US NAS population.
The most consistent finding of our study was the significant increase in methylation at SLFN11 cg10911913 that was associated with one-year moving average concentrations of the PM2.5 species Fe, Ni, and V. Schlafen Family Member (SLFN) genes encode a family of proteins that have been implicated in the regulation of cell growth and T cell development.Citation42,43 SLFN11, a member of the SLFN family, potently and specifically abrogates the production of retroviruses, e.g., human immunodeficiency virus (HIV-1).Citation33 Using cells with endogenously high and low SLFN11 expression and siRNA-mediated silencing, Zoppoli et al.Citation44 found that SLFN11 causally determines cell death and cell cycle arrest in response to DNA-damaging agents in cancer cells from different tissues of origin. SLFN11 cg10911913 is located in a region whose function is promoter associated. Our results showed that methylation at this CpG site was positively associated with one-year moving averages of the PM2.5 species Fe, Ni, and V. Our analysis suggests that exposure to ambient particles, especially those from oil combustion, was associated with hypermethylation of CpGs within SLFN11, which might lead to its downregulation. This hypothesis is consistent with recent literature on air-pollution-induced activation of viral sequences integrated in the human genome. For example, a study of truck drivers and office workers in Beijing, China showed a significant association of exposure to elemental carbon—taken as a marker of traffic particle exposure—with altered expression of viral microRNAs, possibly reflecting reactivation of latent retroviral sequences integrated in the host DNA.Citation45
We also observed that methylation at one cut homeobox 1 (ONECUT1) cg15446043 was positively associated with Fe and Ni exposures. ONECUT1 can inhibit hepatitis B virus gene expression and DNA replication via both transcriptional and post-transcriptional mechanisms.Citation46
In addition, we found that long-term exposure to Fe was linked to methylation in other genes expressed in immune cells, including cytohesin 1 (CYTH1) and ribosomal protein S6 kinase (RPS6KB2). CYTH1 regulates adhesion and transendothelial migration of monocytes, T lymphocytes, and dentritic cells; it is also a key regulator of neutrophil adhesion to endothelial cells and to components of the extracellular matrix.Citation47,48 RPS6KB2 plays an important role in neutrophilic differentiation and neutrophilic proliferation in human promyelocytic leukemia (HL-60) cells. Hence, methylation changes at pertinent CpG sites within these 2 genes may affect immune function.
Interestingly, we found that Fe was associated with methylation levels of CpGs in genes that regulate the cell cycle, such as CDC5L cg22877480 and RPS6KB2 cg26457823.Citation49,50 Cell division cycle 5-like (CDC5L) is a crucial regulator of cell cycle G2/M progression and a component of pre-mRNA processing; hence, previous research has suggested it as a target for cancer therapyCitation50,51 RPS6KB2 is also highly active in the G2 and M phases.Citation49
The DAVID bioinformatics analysis also indicated that Fe and Ni were associated with type II diabetes mellitus, insulin signaling pathways, and the MAPK pathway. This is consistent with previous work. For instance, long-term PM2.5 exposure resulted in systemic inflammation, increased visceral adiposity, and insulin resistance among mice on a high fat diet.Citation52 In mice consuming normal diets, PM exposure was a risk factor for the development of type II diabetes, as it induced insulin resistance and impaired glucose tolerance.Citation53 Similarly, in humans, long-term exposure to particulate matter was linked to increased risk of type II diabetes in a large cohort study.Citation54 We have also reported that particulate air pollution was associated with changes in methylation in the MAPK pathway within the NAS cohort, but were unable to analyze metal components as in this study.Citation55
Earlier, we found that particles from oil combustion were associated with markers of inflammation and endothelial dysfunction, and with increased blood pressure in the NAS population.Citation56,57
The current study may indicate potential mechanisms of PM-related health effects on an epigenetic level: specifically, a proinflammatory stimulus such as air pollution may modify methylation in genes relevant to immune responses, inducing inflammation and endothelial dysfunction, thereby influencing cardiovascular health. Our study has several strengths. First, this is the first study that examined the effects of ambient particulate matter species on epigenome-wide DNA methylation. Previous studies often estimated DNA methylation associated with ambient PM using surrogates for global methylation or gene-specific methylation at candidate genes. An epigenome-wide study like ours offers a novel perspective on such an association, and implicates potential biologic mechanisms on an epigenetic level. Second, we used a stringent Bonferroni correction threshold to adjust the level of significance, which accounted both for the 11 PM2.5 species and for the number of CpG sites analyzed. Although we observed a small number of significant CpGs due to the strict correction, the probability of false positives is expected to remain appropriate. Third, the study population was geographically stable and well followed since enrollment in 1,963.
On the other hand, this study has several notable limitations. One major limitation is that we were unable to perform a replication analysis due to a lack of appropriate comparison cohorts. The German KORA cohorts had PM2.5 mass data but did not have measurements on PM species. Since the chemical composition of air pollutants in Germany may differ from that in the US, we were not able to impute species data from PM2.5 measurements. We do not have information on gene expression, and thus we cannot confirm the expected functional implications of the identified methylation changes. Further research on gene expression in relation to PM exposures is needed. Additionally, we are unable to rule out residual confounding. The bioinformatics analysis of pathways used annotations of methylation sites within genes and thus genes that include more sites on the 450K array, such as those involved in cancer, are more likely to arise in pathway results.Citation58 Last but not least, there exists a generalizability issue because our study population consisted almost entirely of elderly Caucasian men. One should consider factors like age, sex, and race when generalizing our findings to other populations.
In summary, we examined the association of long-term exposure to PM2.5 species with differential DNA methylation in a 450K epigenome-wide association study using a large longitudinal cohort. Our study added evidence on the association of ambient particulate exposure with epigenome-wide DNA methylation.Citation20 It also suggests differing relative toxicities of PM species, which is especially important given the US. National Research Council's recommendation to consider determining the differential risk of PM2.5 species a research priority.Citation59 Furthermore, loci identified in the study provide a possible link between PM2.5 and its identified health effects. Specifically, the current study suggests that particles from oil combustion are associated with methylation changes in genes relevant to immune responses. Further studies are needed to explore the mechanisms behind the relationship between ambient particles and DNA methylation.
Disclosure of potential conflicts of interest
No potential conflicts of interest were disclosed.
Disclaimers
The contents are solely the responsibility of the grantee and do not necessarily represent the official views of the funders. Further, the funders do not endorse the purchase of any commercial products or services mentioned in the publication.
Supplementary_materials.docx
Download MS Word (162.8 KB)Funding
This work was supported by the National Institute of Environmental Health Sciences ES000002, ES021733 and ES015172–01 and the US. Environmental Protection Agency RD-83587201. A.J. was supported by the National Institute of Environmental Health Sciences K99 ES023450. D.S. was supported by a VA Research Career Scientist Award. The VA Normative Aging Study is supported by the Cooperative Studies Program/Epidemiology Research and Information Centers of the US. Department of Veterans Affairs and is a component of the Massachusetts Veterans Epidemiology Research and Information Center, Boston, Massachusetts.
References
- Lelieveld J, Evans JS, Fnais M, Giannadaki D, Pozzer A. The contribution of outdoor air pollution sources to premature mortality on a global scale. Nature 2015; 525:367-71; PMID:26381985; http://dx.doi.org/10.1038/nature15371
- Zanobetti A, Franklin M, Koutrakis P, Schwartz J. Fine particulate air pollution and its components in association with cause-specific emergency admissions. Environ Health 2009; 8:58; PMID:20025755; http://dx.doi.org/10.1186/1476-069X-8-58
- Dai L, Zanobetti A, Koutrakis P, Schwartz JD. Associations of fine particulate matter species with mortality in the United States: a multicity time-series analysis. Environ Health Perspect 2014; 122:837-42; PMID:24800826; http://dx.doi.org/10.1289/ehp.1307568
- Krall JR, Anderson GB, Dominici F, Bell ML, Peng RD. Short-term exposure to particulate matter constituents and mortality in a national study of U.S. urban communities. Environ Health Perspect 2013; 121:1148-53; PMID:23912641; http://dx.doi.org/10.1289/ehp.1206185
- Peng R, Bell M, Geyh A, McDermott A, Zeger S, Samet J, Dominici F. Emergency admissions for cardiovascular and respiratory diseases and the chemical composition of fine particle air pollution. Environ Health Perspect 2009; 117:957-63; PMID:19590690; http://dx.doi.org/10.1289/ehp.0800185
- Madrigano J, Baccarelli A, Mittleman MA, Wright RO, Sparrow D, Vokonas PS, Tarantini L, Schwartz J. Prolonged exposure to particulate pollution, genes associated with glutathione pathways, and DNA methylation in a cohort of older men. Environ Health Perspect 2011; 119:977-82; PMID:21385671; http://dx.doi.org/10.1289/ehp.1002773
- Chen R, Qiao L, Li H, Zhao Y, Zhang Y, Xu W, Wang C, Wang H, Zhao Z, Xu X, et al. Fine Particulate Matter Constituents, Nitric Oxide Synthase DNA Methylation and Exhaled Nitric Oxide. Environ Sci Technol 2015; 49:11859-65; PMID:26372312; http://dx.doi.org/10.1021/acs.est.5b02527
- Baccarelli A, Bollati V. Epigenetics and environmental chemicals. Curr Opin Pediatr 2009; 21:243-51; PMID:19663042; http://dx.doi.org/10.1097/MOP.0b013e32832925cc
- Belinsky SA, Palmisano WA, Gilliland FD, Crooks LA, Divine KK, Winters SA, Grimes MJ, Harms HJ, Tellez CS, Smith TM, et al. Aberrant promoter methylation in bronchial epithelium and sputum from current and former smokers. Cancer Res 2002; 62:2370-7; PMID:11956099
- Chen H, Li S, Liu J, Diwan BA, Barrett JC, Waalkes MP. Chronic inorganic arsenic exposure induces hepatic global and individual gene hypomethylation: implications for arsenic hepatocarcinogenesis. Carcinogenesis 2004; 25:1779-86; PMID:15073043; http://dx.doi.org/10.1093/carcin/bgh161
- Baccarelli A, Tarantini L, Wright RO, Bollati V, Litonjua AA, Zanobetti A, Sparrow D, Vokonas PS, Schwartz J. Repetitive element DNA methylation and circulating endothelial and inflammation markers in the VA normative aging study. Epigenetics 2010; 5:222-8; PMID:20305373; http://dx.doi.org/10.4161/epi.5.3.11377
- Castro R, Rivera I, Struys EA, Jansen EE, Ravasco P, Camilo ME, Blom HJ, Jakobs C, Tavares de Almeida I. Increased homocysteine and S-adenosylhomocysteine concentrations and DNA hypomethylation in vascular disease. Clin Chem 2003; 49:1292-6; PMID:12881445; http://dx.doi.org/10.1373/49.8.1292
- Bellavia A, Urch B, Speck M, Brook RD, Scott JA, Albetti B, Behbod B, North M, Valeri L, Bertazzi PA, et al. DNA hypomethylation, ambient particulate matter, and increased blood pressure: findings from controlled human exposure experiments. J Am Heart Assoc 2013; 2:e000212; PMID:23782920; http://dx.doi.org/10.1161/JAHA.113.000212
- Madrigano J, Baccarelli A, Mittleman MA, Sparrow D, Spiro A, 3rd, Vokonas PS, Cantone L, Kubzansky L, Schwartz J. Air pollution and DNA methylation: interaction by psychological factors in the VA Normative Aging Study. Am J Epidemiol 2012; 176:224-32; PMID:22798479; http://dx.doi.org/10.1093/aje/kwr523
- Fan T, Fang SC, Cavallari JM, Barnett IJ, Wang Z, Su L, Byun HM, Lin X, Baccarelli AA, Christiani DC. Heart rate variability and DNA methylation levels are altered after short-term metal fume exposure among occupational welders: a repeated-measures panel study. BMC Public Health 2014; 14:1279; PMID:25512264; http://dx.doi.org/10.1186/1471-2458-14-1279
- Kile ML, Fang S, Baccarelli AA, Tarantini L, Cavallari J, Christiani DC. A panel study of occupational exposure to fine particulate matter and changes in DNA methylation over a single workday and years worked in boilermaker welders. Environ Health 2013; 12:47; PMID:23758843; http://dx.doi.org/10.1186/1476-069X-12-47
- Joubert BR, Haberg SE, Nilsen RM, Wang X, Vollset SE, Murphy SK, Huang Z, Hoyo C, Midttun O, Cupul-Uicab LA, et al. 450K epigenome-wide scan identifies differential DNA methylation in newborns related to maternal smoking during pregnancy. Environ Health Perspect 2012; 120:1425-31; PMID:22851337; http://dx.doi.org/10.1289/ehp.1205412
- Joubert BR, Felix JF, Yousefi P, Bakulski KM, Just AC, Breton C, Reese SE, Markunas CA, Richmond RC, Xu CJ, et al. DNA Methylation in Newborns and Maternal Smoking in Pregnancy: Genome-wide Consortium Meta-analysis. Am J Hum Genet 2016; 98:680-96; PMID:27040690; http://dx.doi.org/10.1016/j.ajhg.2016.02.019
- Zeilinger S, Kuhnel B, Klopp N, Baurecht H, Kleinschmidt A, Gieger C, Weidinger S, Lattka E, Adamski J, Peters A, et al. Tobacco smoking leads to extensive genome-wide changes in DNA methylation. PLoS One 2013; 8:e63812; PMID:23691101; http://dx.doi.org/10.1371/journal.pone.0063812
- Panni T, Mehta AJ, Schwartz JD, Baccarelli AA, Just AC, Wolf K, Wahl S, Cyrys J, Kunze S, Strauch K, et al. A Genome-Wide Analysis of DNA Methylation and Fine Particulate Matter Air Pollution in Three Study Populations: KORA F3, KORA F4, and the Normative Aging Study. Environ Health Perspect 2016; 124(7):983-90; PMID:26731791; http://dx.doi.org/10.1289/ehp.1509966
- Bell B, Rose C, Damon A. The Normative Aging Study: an interdisciplinary and longitudinal study of health and aging. Aging Hum Dev 1972; 3:4-17; http://dx.doi.org/10.2190/GGVP-XLB5-PC3N-EF0G
- Bibikova M, Barnes B, Tsan C, Ho V, Klotzle B, Le JM, Delano D, Zhang L, Schroth GP, Gunderson KL, et al. High density DNA methylation array with single CpG site resolution. Genomics 2011; 98:288-95; PMID:21839163; http://dx.doi.org/10.1016/j.ygeno.2011.07.007
- Triche TJ, Jr., Weisenberger DJ, Van Den Berg D, Laird PW, Siegmund KD. Low-level processing of Illumina Infinium DNA Methylation BeadArrays. Nucleic Acids Res 2013; 41:e90; PMID:23476028; http://dx.doi.org/10.1093/nar/gkt090
- Davis S, Du P, Bilke S, Triche T, Jr., Bootwalla M. methylumi: Handle Illumina methylation data. R package version 2.17.0., 2015
- Teschendorff AE, Jones A, Fiegl H, Sargent A, Zhuang JJ, Kitchener HC, Widschwendter M. Epigenetic variability in cells of normal cytology is associated with the risk of future morphological transformation. Genome Med 2012; 4:24; PMID:22453031; http://dx.doi.org/10.1186/gm323
- Zhu ZZ, Hou L, Bollati V, Tarantini L, Marinelli B, Cantone L, Yang AS, Vokonas P, Lissowska J, Fustinoni S, et al. Predictors of global methylation levels in blood DNA of healthy subjects: a combined analysis. Int J Epidemiol 2012; 41:126-39; PMID:20846947; http://dx.doi.org/10.1093/ije/dyq154
- Mostofsky E, Schwartz J, Coull BA, Koutrakis P, Wellenius GA, Suh HH, Gold DR, Mittleman MA. Modeling the association between particle constituents of air pollution and health outcomes. Am J Epidemiol 2012; 176:317-26; PMID:22850792; http://dx.doi.org/10.1093/aje/kws018
- Houseman EA, Accomando WP, Koestler DC, Christensen BC, Marsit CJ, Nelson HH, Wiencke JK, Kelsey KT. DNA methylation arrays as surrogate measures of cell mixture distribution. BMC Bioinformatics 2012; 13:86; PMID:22568884; http://dx.doi.org/10.1186/1471-2105-13-86
- Aryee MJ, Jaffe AE, Corrada-Bravo H, Ladd-Acosta C, Feinberg AP, Hansen KD, Irizarry RA. Minfi: a flexible and comprehensive Bioconductor package for the analysis of Infinium DNA methylation microarrays. Bioinformatics (Oxford, England) 2014; 30:1363-9; PMID:24478339; http://dx.doi.org/10.1093/bioinformatics/btu049
- Reinius LE, Acevedo N, Joerink M, Pershagen G, Dahlen SE, Greco D, Soderhall C, Scheynius A, Kere J. Differential DNA methylation in purified human blood cells: implications for cell lineage and studies on disease susceptibility. PLoS One 2012; 7:e41361; PMID:22848472; http://dx.doi.org/10.1371/journal.pone.0041361
- Huang da W, Sherman BT, Lempicki RA. Systematic and integrative analysis of large gene lists using DAVID bioinformatics resources. Nat Protoc 2009; 4:44-57; PMID:19131956; http://dx.doi.org/10.1038/nprot.2008.211
- Huang da W, Sherman BT, Lempicki RA. Bioinformatics enrichment tools: paths toward the comprehensive functional analysis of large gene lists. Nucleic Acids Res 2009; 37:1-13; PMID:19033363; http://dx.doi.org/10.1093/nar/gkn923
- Li M, Kao E, Gao X, Sandig H, Limmer K, Pavon-Eternod M, Jones TE, Landry S, Pan T, Weitzman MD, et al. Codon-usage-based inhibition of HIV protein synthesis by human schlafen 11. Nature 2012; 491:125-8; PMID:23000900; http://dx.doi.org/10.1038/nature11433
- Hartigan JA, Hartigan PM. The Dip Test of Unimodality. Ann Statist 1985; 13:70-84; http://dx.doi.org/10.1214/aos/1176346577
- Chen YA, Lemire M, Choufani S, Butcher DT, Grafodatskaya D, Zanke BW, Gallinger S, Hudson TJ, Weksberg R. Discovery of cross-reactive probes and polymorphic CpGs in the Illumina Infinium HumanMethylation450 microarray. Epigenetics 2013; 8:203-9; PMID:23314698; http://dx.doi.org/10.4161/epi.23470
- Abecasis GR, Auton A, Brooks LD, DePristo MA, Durbin RM, Handsaker RE, Kang HM, Marth GT, McVean GA. An integrated map of genetic variation from 1,092 human genomes. Nature 2012; 491:56-65; PMID:23128226; http://dx.doi.org/10.1038/nature11632
- R Core Team. R: A language and environment for statistical computing. R Foundation for Statistical Computing, Vienna, Austria. URL http://www.R-project.org/. 2014
- Kanehisa M, Goto S, Kawashima S, Nakaya A. The KEGG databases at GenomeNet. Nucleic Acids Res 2002; 30:42-6; PMID:11752249; http://dx.doi.org/10.1093/nar/30.1.42
- Benjamini Y, Hochberg Y. Controlling the False Discovery Rate: A Practical and Powerful Approach to Multiple Testing. J Royal Statist Soc Series B (Methodological) 1995; 57:289-300
- Geiger A, Cooper J. Overview of Airborne Metals Regulations, Exposure Limits, Health Effects, and Contemporary Research. 2010
- Baccarelli A, Wright RO, Bollati V, Tarantini L, Litonjua AA, Suh HH, Zanobetti A, Sparrow D, Vokonas PS, Schwartz J. Rapid DNA methylation changes after exposure to traffic particles. Am J Respir Crit Care Med 2009; 179:572-8; PMID:19136372; http://dx.doi.org/10.1164/rccm.200807-1097OC
- Geserick P, Kaiser F, Klemm U, Kaufmann SH, Zerrahn J. Modulation of T cell development and activation by novel members of the Schlafen (slfn) gene family harbouring an RNA helicase-like motif. Inter Immunol 2004; 16:1535-48; PMID:15351786; http://dx.doi.org/10.1093/intimm/dxh155
- Schwarz DA, Katayama CD, Hedrick SM. Schlafen, a new family of growth regulatory genes that affect thymocyte development. Immunity 1998; 9:657-68; PMID:9846487; http://dx.doi.org/10.1016/S1074-7613(00)80663-9
- Zoppoli G, Regairaz M, Leo E, Reinhold WC, Varma S, Ballestrero A, Doroshow JH, Pommier Y. Putative DNA/RNA helicase Schlafen-11 (SLFN11) sensitizes cancer cells to DNA-damaging agents. Proc Natl Acad Sci 2012; 109:15030-5; PMID:22927417; http://dx.doi.org/10.1073/pnas.1205943109
- Hou L, Barupal J, Zhang W, Zheng Y, Liu L, Zhang X, Dou C, McCracken JP, Diaz A, Motta V, et al. Particulate Air Pollution Exposure and Expression of Viral and Human MicroRNAs in Blood: The Beijing Truck Driver Air Pollution Study. Environ Health Perspect 2015; 124(3):344-50; PMID:26068961; http://dx.doi.org/10.1289/ehp.1408519
- Hao R, He J, Liu X, Gao G, Liu D, Cui L, Yu G, Yu W, Chen Y, Guo D. Inhibition of hepatitis B virus gene expression and replication by hepatocyte nuclear factor 6. J Virol 2015; 89:4345-55; PMID:25653429; http://dx.doi.org/10.1128/JVI.03094-14
- El azreq MA, Bourgoin SG. Cytohesin-1 regulates human blood neutrophil adhesion to endothelial cells through beta2 integrin activation. Mol Immunol 2011; 48:1408-16; PMID:21511340; http://dx.doi.org/10.1016/j.molimm.2011.03.018
- Kolanus W. Guanine nucleotide exchange factors of the cytohesin family and their roles in signal transduction. Immunol Rev 2007; 218:102-13; PMID:17624947; http://dx.doi.org/10.1111/j.1600-065X.2007.00542.x
- Boyer D, Quintanilla R, Lee-Fruman KK. Regulation of catalytic activity of S6 kinase 2 during cell cycle. Mol Cell Biochem 2008; 307:59-64; PMID:17786541; http://dx.doi.org/10.1007/s11010-007-9584-5
- Graub R, Lancero H, Pedersen A, Chu M, Padmanabhan K, Xu XQ, Spitz P, Chalkley R, Burlingame AL, Stokoe D, et al. Cell cycle-dependent phosphorylation of human CDC5 regulates RNA processing. Cell cycle (Georgetown, Tex) 2008; 7:1795-803; PMID:18583928; http://dx.doi.org/10.4161/cc.7.12.6017
- Mu R, Wang YB, Wu M, Yang Y, Song W, Li T, Zhang WN, Tan B, Li AL, Wang N, et al. Depletion of pre-mRNA splicing factor Cdc5L inhibits mitotic progression and triggers mitotic catastrophe. Cell death & disease 2014; 5:e1151; PMID:24675469; http://dx.doi.org/10.1038/cddis.2014.117
- Sun Q, Yue P, Deiuliis JA, Lumeng CN, Kampfrath T, Mikolaj MB, Cai Y, Ostrowski MC, Lu B, Parthasarathy S, et al. Ambient air pollution exaggerates adipose inflammation and insulin resistance in a mouse model of diet-induced obesity. Circulation 2009; 119:538-46; PMID:19153269; http://dx.doi.org/10.1161/CIRCULATIONAHA.108.799015
- Xu X, Liu C, Xu Z, Tzan K, Zhong M, Wang A, Lippmann M, Chen LC, Rajagopalan S, Sun Q. Long-term exposure to ambient fine particulate pollution induces insulin resistance and mitochondrial alteration in adipose tissue. Toxicol Sci 2011; 124:88-98; PMID:21873646; http://dx.doi.org/10.1093/toxsci/kfr211
- Weinmayr G, Hennig F, Fuks K, Nonnemacher M, Jakobs H, Mohlenkamp S, Erbel R, Jockel KH, Hoffmann B, Moebus S. Long-term exposure to fine particulate matter and incidence of type 2 diabetes mellitus in a cohort study: effects of total and traffic-specific air pollution. Environ Health 2015; 14:53; PMID:26087770; http://dx.doi.org/10.1186/s12940-015-0031-x
- Carmona JJ, Sofer T, Hutchinson J, Cantone L, Coull B, Maity A, Vokonas P, Lin X, Schwartz J, Baccarelli AA. Short-term airborne particulate matter exposure alters the epigenetic landscape of human genes associated with the mitogen-activated protein kinase network: a cross-sectional study. Environ Health 2014; 13:94; PMID:25395096; http://dx.doi.org/10.1186/1476-069X-13-94
- Dai L, Bind MA, Koutrakis P, Coull BA, Sparrow D, Vokonas PS, Schwartz JD. Fine particles, genetic pathways, and markers of inflammation and endothelial dysfunction: Analysis on particulate species and sources. J Expo Sci Environ Epidemiol 2016; 26(4):415-21; PMID:26732377; http://dx.doi.org/10.1038/jes.2015.83
- Dai L, Koutrakis P, Coull BA, Sparrow D, Vokonas PS, Schwartz JD. Use of the Adaptive LASSO Method to Identify PM Components Associated with Blood Pressure in Elderly Men: The Veterans Affairs Normative Aging Study. Environ Health Perspect 2015; 124(1):120-5; PMID:26090776; http://dx.doi.org/10.1289/ehp.1409021
- Harper KN, Peters BA, Gamble MV. Batch effects and pathway analysis: 2 potential perils in cancer studies involving DNA methylation array analysis. Cancer Epidemiol Biomarkers Prev 2013; 22:1052-60; http://dx.doi.org/10.1158/1055-9965.EPI-13-0114
- National Reserch Council. Research Priorities for Airborne Particulate Matter: IV. Continuing Research Progress Washington, DC: National Research Council 2004