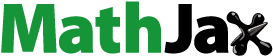
ABSTRACT
This study evaluated the hypothesis that prenatal maternal socioeconomic status (SES) adversity is associated with DNA methylation in the placenta. SES adversity was defined by the presence of, as well as a summative count of, four factors: less than college education, single marital status, food and nutritional service assistance, and public health insurance. Epigenome-wide DNA methylation was assessed using the Illumina EPIC array in 426 placentas from a sample of infants born < 28 weeks of gestation from the Extremely Low Gestational Age Newborn cohort. Associations between SES adversity and DNA methylation were assessed with robust linear regressions adjusted for covariates and controlled the false discovery rate at < 10%. We also examined whether such associations were sex specific. Indicators of SES adversity were associated with differential methylation at 33 CpG sites. Of the 33 identified CpG sites, 19 (57.6%) displayed increased methylation, and 14 (42.4%) displayed decreased methylation in association with at least one of the SES adversity factors. Sex differences were observed in DNA methylation associated with summative SES score; in which placentas derived from female pregnancies showed more robust differential CpG methylation than placentas from male pregnancies. Maternal SES adversity was associated with differential methylation of genes with key role in gene transcription and placental function, potentially altering immunity and stress response. Further investigation is needed to evaluate the role of epigenetic differences in mediating the association between maternal socioeconomic status during pregnancy and later life health outcomes in children.
Introduction
Socioeconomic status (SES) plays a critical role in the enrichment of the environment of a mother and her developing child by shaping access to various basic needs [Citation1], including healthcare, income, and nutrition. Lower SES is related to adverse pregnancy-related health outcomes [Citation2,Citation3], including maternal morbidity, preterm birth, and a wide array of cognitive and emotional outcomes in children. The origins of these adverse outcomes appear to precede birth, continue into adulthood [Citation4,Citation5], and persist across generations [Citation6].
Socioeconomic adversity is a chronic stressor exposing individuals to environmental, psychological, and behavioural factors that increase demands on biological regulatory systems. Thus, SES adversity is thought to lead to a biological embedding of dysregulated physiological homeostasis as well as dysregulated stress and inflammatory responses [Citation7,Citation8]. The specific biologic mechanisms by which prenatal SES is linked to biological changes in the offspring, however, are not well understood.
One key biological mechanism of interest is epigenetic modification via DNA methylation, the addition of a methyl group to a cytosine (-CH3) in the context of CpG dinucleotides. The addition of these methyl groups can affect gene activity and phenotype yet do not alter the DNA sequence. Two pathways by which low SES might influence DNA methylation are: (1) oxidative stress induction – an imbalance between reactive oxygen species (i.e., free radicals) – antioxidant defences that could influence the enzymatic processes that control CpG methylation; and (2) pro-inflammatory induction that could affect the abundance and binding of transcription factors and thus subsequent DNA methylation patterns [Citation9–Citation11].
SES has been associated with alterations in DNA methylation of stress- and inflammation-related genes in peripheral blood lymphocytes [Citation12,Citation13]. In a candidate-gene study of placentas, maternal education, prenatal tobacco exposure, and SES summative risk score (sum of the SES adverse factors: low education, living in poverty, single mother, crowded dwelling, prenatal tobacco use) were associated with differential methylation of a key regulatory enzymes in cortisol hormone signalling [Citation14].
A meta-analysis suggested that the association between SES factors, such as educational attainment, and DNA methylation could be confounded by maternal smoking exposure [Citation15]. However, while controlling for maternal smoking and other covariates, a recent epigenome-wide study found that DNA methylation in cord blood and blood collected during adolescence (20 CpG sites) was associated with maternal education [Citation16]. Furthermore, sexual-dimorphic patterns in DNA methylation were observed, with greater CpG methylation in blood derived from females, as compared to males [Citation16].
The placenta serves as the interface between the mother and foetus and facilitates the transfer of nutrients, water, oxygen, and immune mediators to the developing foetus. In addition, the placenta senses and transduces signals from the environment to the foetus and is involved in the regulation of genes and hormone production in response to stressful exposures [Citation17,Citation18]. Previous research has demonstrated that placental methylation is linked to maternal exposure to various environmental factors [Citation19,Citation20], including socioeconomic adversity [Citation14]. Accordingly, we posit that the placental epigenome can serve as a ‘recording’ of intrauterine environmental adversity and the resultant alterations in biological pathways [Citation17]. Moreover, epigenetic changes are linked to birth outcomes as well as outcomes later in life [Citation18,Citation21,Citation22]; specifically, placental methylation is influenced by psychosocial distress, such as socioeconomic adversity, during pregnancy [Citation17] and is linked to both maternal and child health outcomes [Citation21], suggesting sex-specific patterns [Citation14,Citation23].
In this study, we hypothesized that prenatal SES adversity factors would be associated with placental epigenome-wide DNA methylation. Our goal was to identify placental epigenome-wide DNA methylation patterns associated with indicators of SES, while controlling for potential cofounders, such as smoking exposure. We also examined whether associations between prenatal SES adversity and placental DNA methylation differ in the placentas derived from male or female pregnancies.
Results
For the current study, 426 subjects from the Extremely Low Gestational Age Newborns (ELGAN) cohort were selected based on the availability of placental samples with data on DNA methylation. As summarized in , maternal characteristics of the study sample were largely similar to those in the ELGAN cohort from which the sample was derived. Mothers were on average 29.4 years old and 62.1% were white. At birth, the average gestational age was 25.6 weeks, 61% of the mothers had a singleton pregnancy, and 52.3% of the infants were male. Regarding SES adversity factors, 22.5% of the mothers were single, 13.3% had less than high school education, 34% had public insurance, and 20% were receiving food stamps via supplemental nutrition assistance. In the sample, most mothers (82.6%) had at least two of the four SES adverse factors, while 9.9% had none or only one adverse factor. shows the associations among the SES factors.
Table 1. Sample characteristics.
Table 2. Association among socioeconomic factorsa.
In placental epigenome-wide CpG-by-CpG methylation analysis with false discovery rate (FDR) < 0.1, methylation at 33 CpG sites across the genome, corresponding to 21 genes, were associated with SES factors, in models adjusted for maternal age, race, infant sex, birth weight, gestational age, acute inflammation of the placenta chorion, and second-hand smoking exposure (). These results were largely consistent in the models without adjustment for smoking exposure, therefore we used the final model with smoking for statistical inference (Supplemental File 2 shows the top 50 CpG sites for the two models for each SES factors).
Figure 1. Manhattan plots of CpG methylation loci associated with SES factors. (a) Summative score, (b) marital status, (c) education level, (d) food security, and (e) insurance status. The x-axis represents the genomic location of the individual CpG locis and the y-axis represent the -log10(p value) associated with socioeconomic factors, adjusted for maternal age, race, infant sex, birth weight, gestational age, smoking exposure, placental inflammation, and cell-mixture; FDR threshold at 10% (bottom) and 5% (top) lines have been added to the plot, respectively. QQ plots (right side of the figure) here plot theoretical quantiles of the t-distribution on the x-axis against the empirical modified t-statistics estimated using the empirical Bayes procedure.
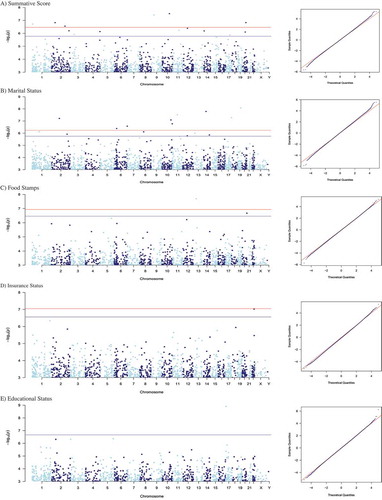
Of these 33 CpG sites identified, 14 were associated with the summative SES score, 15 were associated with marital status, two were associated with being on supplemental nutrition assistance, one was associated with educational level, and one was associated with health insurance status (). The majority of the CpG sites (19; 57.6%) displayed increased methylation in association with one of the SES factors, and 14 (42.4%) displayed decreased methylation in association with one of the SES factors.
Table 3. Epigenome-wide results of the association between DNA methylation and socioeconomic factors.
Among the five most statistically significant CpG sites, four were associated with marital status (cg21075783 [logFC = −0.91, p = 8.28E-09]; cg14194715 [logFC = −0.38, p = 2.99E-08]; cg15555622 [logFC = −0.30, p = 1.64E-08]; and cg07980716 [logFC = 0.45, p = 5.25E-08]). Of these five CpG sites, only cg14194715 and cg07980716 were annotated to genes that were located in the bodies of the protein coding genes: FRY Microtubule Binding Protein (FRY), and the Apoptosis Inducing Factor Mitochondria Associated 1 (AIFM1), respectively. Two CpG sites displaying decreased methylation within the Chromosome 15 Open Reading Frame 26 (C15orf26) gene were associated with summative SES score (cg26546864 [logFC = −0.67, p = 3.78E-08], and cg09236163 [logFC = – 0.64, p = 5.76E-07]).
As a secondary approach, we conducted differentially methylated regional analysis, but found no significant associations between differential methylated regions relative to maternal SES factors at FDR < 0.1.
Sex-stratified results
In models stratified by the sex of the pregnancy with FDR < 0.01, we observed robust differences in autosomes DNA methylation between placentas derived from female pregnancies (27 CpG sites, 19 genes) and male pregnancies (2 CpG sites) in association with summative SES score (Supplemental File 3, Table S1, Figures S1–S5). Among the female-derived placentas, the most statistically significant relationship was observed at cg06064918 (logFC = −1.65, p = 9.06E-28) and cg10052190 (logFC = −2.84, p = 1.01E-20) which are within the protein coding Chromosome 16 Open Reading Frame 52 (C16orf52) gene. Of note, three CpG sites (cg15895819, cg20860638, cg05134500) within the melanotransferrin gene (MFI2) showed statistically significant relationships to summative SES score. Similar to the non-stratified analysis, differentially methylated regional analysis did not identify differentially methylated regions relative to SES indicators.
In both sex-stratified and non-stratified analyses, cg26546864 within the TSS1500 region of the C15orf26 gene in chromosome 15 and cg11173636 (not annotated to a gene) within chromosome 10 were associated with the summative SES adversity score.
Functional enrichment results
Further analysis using Ingenuity Pathways Analysis (IPA) revealed canonical pathways and networks (Supplemental File 3, Table S2, Figures S6 and S7) associated with differentially methylated genes. For the 21 methylated genes related to SES, top canonical pathways and network are involved in biological functions including cellular development, growth and proliferation, muscular and haematological system development and function, and immune cell trafficking. For the 22 differentially methylated genes in placentas derived from female pregnancies, top canonical pathways and network have biological functions in cell cycle, embryonic development, gene expression, and energy production.
Discussion
In this study, we set out to characterize the relationship between in utero exposure to socioeconomic adversity and DNA methylation patterning in the placenta. Based on the impact of SES on oxidative stress and inflammation cellular processes, we hypothesized that SES would be associated with epigenome-wide DNA methylation across multiple CpG sites. To our knowledge, this is among the first studies to explore the association between SES factors and placental DNA methylation using an epigenome-wide approach. Changes in DNA methylation in the placenta have been linked to later life health outcomes [Citation24,Citation25], suggesting it may serve as sentinel response system to environmental stressors and exposures. In this study, a total of 33 CpG sites (representing 21 genes) were identified that were associated with either a summative SES adversity score or individual component exposures, including marital status, maternal education, and supplemental nutrition assistance. Additionally, sex differences were observed in DNA methylation associated with summative adversity score. Specifically, the placentas derived from female pregnancies showed more differentially methylated CpG sites than the placentas from male pregnancies. Taken together, these findings suggest that socioeconomic adversity (independent of second-hand smoke exposure) is associated with imprints on the epigenome and may be linked to biological embedding of SES adversity, which could affect long-term child outcomes [Citation26].
SES adversity is associated with many health outcomes, including neurodevelopmental, and neurobehavioral disorders in children as well as poorer adult health status and shorter life expectancy [Citation27–Citation33]. In the present analysis, summative adversity score and single-mother family were the SES exposures most often associated with placental DNA methylation. This finding underscores that although SES factors are often related, they might not exert similar biological effects as they combine and accumulate together with innate and acquired biological functions over the life-course [Citation1,Citation34]. This is consistent with other studies reporting difference in associations between one or more SES factors and DNA methylation in placenta and other tissues [Citation4,Citation12,Citation14].
The probes most significantly associated with SES factors belong to the FRY (cg14194715) and AIFM1 (cg07980716) genes. The FRY gene plays a critical role in structural integrity during mitosis, in the developing mammalian cortex and the pathogenesis of congenital neurodevelopmental disorders [Citation35], which aligns with the gene ontology that suggests role in cell morphogenesis and neuron projection development [Citation36]. An important paralog of this gene is FRY Like Transcription Coactivator (FRYL), which is expressed in a wide spectrum of tissues, with high steady-state levels of expression in the placenta, brain, and colon. In an in vivo mice study, Fryl was associated with abnormal kidney development and function leading to death of most mice soon after birth. The authors posited that Fryl deficient newborn mice affects their ability to eliminate detrimental metabolites that can be effectively removed from the foetus through foetal–placental exchange system before parturition [Citation37]. Fryl has also been associated with functional genes clusters (zinc finger, DNA-binding, regulation of transcription) with roles in placentation, trophoblast invasion and/or preeclampsia risk [Citation38,Citation39].
The AIFM1 gene function as a regulator of apoptosis (programmed cell death), an essential feature of normal placental development [Citation40]. Future studies should explore the role of AIFM1 in stress-related function within the placenta [Citation41] and its association to child health. Furthermore, AIFM1 deficiency leads to severe mitochondrial dysfunction, causing muscle atrophy and neurodegeneration in model organisms as well as in humans [Citation42], and has been related to mitochondrial disease [Citation43], motor neuron disease [Citation44], as well as severe encephalopathy in infants [Citation43,Citation45].
In sex-stratified analysis, more robust effects on DNA methylation were observed in the placentas derived from female pregnancies than placentas from male pregnancies. This is in contrast to a study of the placenta that showed that the association between prenatal SES adversity and placental methylation of a candidate gene (HSD11B2) was more robust for male-derived placentas than for female-derived placentas [Citation14]. Similar to our findings in the placenta, two previous studies found childhood SES risk to be more strongly associated with DNA methylation in peripheral blood and/or cord blood from young adult females than males [Citation16,Citation46]. Sex differences in the placenta, its relationship to SES stressors and the functional consequences are still not well understood. Elucidating the sex-specific mechanisms involved in transplacental signals could lead to novel insights into disease risk and resilience related to early life adversity [Citation47,Citation48]. In our study, the probes most significantly associated with female SES cumulative score belong to the protein coding C15orf52 gene (cg05064918, cg10052190), which has moderate expression in placental trophoblastic cells [Citation49]. An important paralog of C15orf52 is Coiled-Coil Domain Containing 9B (CCDC9B) gene. To our knowledge, this is the first time a study associates the C15orf52 gene with SES adversity factors; however, in genome-wide association studies [Citation50,Citation51], both of these genes have been linked to diabetes mellitus, a condition that is highly associated with SES adversity [Citation52,Citation53].
In this study, the protein coding C15orf26 gene, alias Cilia And Flagella Associated Protein 161 (CFAP161), was identified in both sex-stratified (cg26546864) and non-stratified analysis (cg26546864, cg09236163) in association with SES cumulative score. C15orf26 is highly expressed in the placenta relative to other tissues [Citation54]. In human leukocytes, methylation of CpG sites near C15orf26 was positively associated with telomere length (a marker of cellular ‘ageing’) in cell lines, but negatively associated with telomere length in whole blood cells. In another study, C15orf26 was found to be downregulated in mycobacteria infection of respiratory epithelium. Considering this evidence, future studies could investigate the role of C15orf26 in oxidative stress, which can affect developmental programming [Citation10].
Despite substantial differences in the operationalization of SES among DNA methylation studies, the Zinc Finger Protein 609 (ZNF609) was associated with single-parent family status in our study, and with low childhood SES position based on fathers’ education and household amenities in an study using whole blood DNA from adults [Citation55]. This gene, along with NIPBL and the multiprotein complex integrator, promotes cortical neuron migration during brain development by regulating the transcription of crucial genes in this process. Many genes in the zinc finger family function as transcription factors, binding specific long sequences of DNA to control gene transcription, which can play a role in immunity and stress response [Citation56], placental function [Citation57], and methylation of zinc finger genes related to in-utero stress can lead to effects in the offspring epigenome [Citation56].
In addition, a number of other relevant genes were identified as being differentially methylated as a function of SES, including genes linked to psychiatric disorders (Zinc Finger Protein 833, Pseudogene; Family With Sequence Similarity 103, Member A1; and Discs Large Associated Protein 2) [Citation58–Citation61], nervous system development (Cadherin 4; and Ras Homolog Family Member U) [Citation62–Citation64], immune regulation (Down-Regulator Of Transcription 1) [Citation65,Citation66], and metabolic function (Protein Phosphatase 2 Regulatory Subunit Bdelta) [Citation67]. These findings are in line with previous literature showing that psychiatric disorders and immunosuppression is commonly observed in individual with low SES [Citation68–Citation72], as well as in individuals exposed to early-life SES adversity with significant down-regulation of glucocorticoid-related genes in blood [Citation73] and placenta [Citation14], which regulates the secretion of cortisol and transduces its anti-inflammatory actions in the immune system. In the placenta, this mechanism could potentially protect the developing foetus from the deleterious effects of high levels of stress hormones [Citation14].
One possible explanation for the lack of significant findings in the differentially methylated regional analyses is that the effect on SES on the epigenome is diffused throughout the genome, as evidence by the CpG-by-CpG results. Furthermore, the functional enrichment results revealed pathways highly consistent with the gene functions described in this discussion, such as embryonic development, gene expression, cellular growth and proliferation, and immune function.
When interpreting the results of this study, several factors should be considered. Tissue-specific patterns of CpG methylation [Citation74] in the placenta may not be similar to other tissues, and thus future comparison between tissues will not be straight forward. Further, because the ELGAN cohort consists only of preterm births, these findings may not be generalizable to full-term cohorts. Finally, placental RNA or protein is not currently available to functionally validate the expression levels in response to increases in CpG methylation. Future studies should integrate mRNA and protein expression with CpG methylation and environmental exposure data to gain further insight on how CpG methylation in the placenta impacts gene expression levels and relates to socioeconomic adversity. Similarly, access to exposure data for environmental contaminants (e.g., water and air quality) was not currently available for study. Finally, we view the multidimensional definition of SES as a relative strength of this study, but note that the relative weighting of these variables in the model may have influenced findings. Further studies should examine relative contributions of such variables to targeted outcomes and across different samples of children.
In summary, findings in this study suggest that in utero exposure to SES adversity has the potential to alter placental DNA methylation and thereby influence developmental programming, with subsequent influence on neurodevelopmental and neurobehavioral outcomes of the offspring. This study is among the first to explore the effects of SES adversity in relation to the epigenome in the placenta. This work contributes to a growing body of literature showing that adverse social environment is associated with altered placental CpG methylation, which should be further explored for its role in mediating later life health outcomes in children.
Methods
Study Sample
This study used data from the Extremely Low Gestational Age Newborns (ELGAN) study, which enrolled infants born < 28 weeks of gestation during 2002–2004, in 5 states and 14 hospitals in the United States [Citation75]. The broad goal of the ELGAN study is to understand the risk of neurodevelopmental disorders in extremely preterm children. Details regarding the recruitment of ELGAN participants have been discussed elsewhere [Citation75]. Briefly, mothers provided informed consent following admission to the hospital, before birth, or immediately following birth. The Institutional Review Board at each of the 14 participating ELGAN sites approved the study procedures [Citation75].
Placenta tissue collection
Upon expulsion, placentas were placed into a sterile exam basin and taken to a nearby room where biopsies of the placentas were collected as previously described [Citation76]. Briefly, a tissue sample from the foetal side of the placenta was collected by applying traction to the chorion and the underlying trophoblast tissue and cutting a sample out at the base of this tissue structure. The tissue sample was placed into a cryo-vial that was immediately submerged in liquid nitrogen and was stored at −80°C until processing.
DNA extraction and assessment of epigenome-wide DNA methylation
A small subsample of placental tissue (~0.2 g) was cut from the frozen biopsy sample and rinsed with sterile 1 X PBS to wash away any residual blood. Samples were then homogenized in Buffer RLT with β-mercaptoethanol (Qiagen, Valencia CA). An AllPrep DNA/RNA/miRNA Universal Kit (Qiagen, Valencia CA) was utilized to extract DNA and RNA greater than 18 nucleotides in length, according to the manufacturer’s instructions.
To perform epigenome-wide DNA methylation measurements, extracted DNA was bisulphate-converted using the EZ DNA methylation kit (Zymo Research, Irvine, CA), followed by quantification using the Infinium MethylationEPIC BeadChip (Illumina, San Diego, CA) that measures over 850,000 CpG loci at a single nucleotide resolution. Samples were randomly allocated to different plates and chips to minimize confounding. Following approach and recommendations previously described [Citation77,Citation78], DNA methylation data was imported into R for pre-processing using minfi package [Citation79]. Quality control was performed at the sample level, excluding samples that failed (n = 3) and technical duplicates (n = 3); there was no mismatch on genotype or sex; 426 samples were retained for subsequent analyses. Functional normalization was performed with a preliminary step of normal-exponential out-of-band (noob) correction method [Citation80] for background subtraction and dye normalization, followed by the typical functional normalization method with the top two principal components of the control matrix [Citation81,Citation82]. Quality control was performed on individual probes by computing a detection p value and excluded 806 (0.09%) probes with non-significant detection (p > 0.01) for 5% or more of the samples. A total of 856,832 CpG sites were included in the final analyses. Lastly, the ComBat function was used from the sva package to adjust for batch effects from sample plate [Citation83]. The data were visualized using density distributions at all processing steps. Each probe measured the average methylation level at a single CpG site. Methylation levels were calculated and expressed as β values (β = intensity of the methylated allele (M))/(intensity of the unmethylated allele (U) + intensity of the methylated allele (M) + 100). β values were logit transformed to M values for statistical analyses [Citation84].
Prenatal socioeconomic adversity
Maternal education, marital status, eligibility for public health insurance (i.e., Medicaid), and supplemental nutrition assistance as indicated by eligibility to receive food stamps, were used as variables reflecting socioeconomic adversity. A research assistant collected these data at enrolment in the ELGAN study via a structured interview of the mother. In addition, a summative risk score was created to assess the overall risk of experiencing multiple socioeconomic adversities during pregnancy. These SES indicators are related to each other but are not interchangeable. Each contributes to different material and social resources, and differs in their potential roles as having an effect on health status [Citation3]. Moreover, the combination of multiple low SES indicators can potentially result in a cumulative effect on health status [Citation14]. Thus, for this study a risk score was derived based on a sum of the number of the four SES adversity indicators (education [less then < 12 years of education], health insurance [public health insurance], marital status [single], supplemental nutrition assistance [receiving food stamp or similar]), ranging from 0 to 4. Higher scores reflect greater prenatal socioeconomic adversity. For the analyses presented here, SES summative risk score was dichotomized as ≤ 1 vs ≥ 2 to explore its cumulative effects. This cut-off was chosen based on previous work relating SES to placental CpG methylation [Citation14,Citation85], thus allowing us to make potential comparisons in the future.
Statistical analysis
Demographic and clinical characteristics of the 426 participants for analyses are reported using means, SD, or proportions. For the SES indicators, association, and related p values are presented. For each of the SES indicators, CpG-by-CpG analyses were performed by fitting robust linear regression models for each CpG site adjusted for covariates with DNA methylation as the response variable on the M value scale using the SES indicator as the main predictor. Robust linear regression was used to protect against potential heteroscedasticity [Citation78].
Maternal age, race, infant sex, birth weight, gestational age (GA), and acute inflammation of the placenta chorion were included as a priori-selected covariates based on their potential to confound associations among prenatal socioeconomic adversity and DNA methylation. Gestational age (GA) estimates were based on a hierarchy of the quality of available information: dates of embryo retrieval or intrauterine insemination or foetal ultrasound before the 14th week (62%) followed by foetal ultrasound at 14 or more weeks (29%), last menstrual period without foetal ultrasound (7%), and GA recorded in the neonatal intensive care unit log (1%). Race was dichotomized as White and not-White based on the distribution of race/ethnicity in the sample. Maternal age and birth weight z scores were treated as continuous. To reduce the possibility that methylation levels were affected by infiltration of inflammatory cells within the placenta, acute inflammation status of the chorion/decidua was used as an adjustment factor in all models. A trained pathologist examined the chorion/decidua: acute inflammation was graded as 0 (no inflammation), 1 (a single focus of 5–10 neutrophils), 2 (several small foci or a single focus of >10 neutrophils), 3 (numerous large or confluent foci), or 4 (necrosis), with grades of ≥ 2 considered indicative of moderate-to-severe acute inflammation [Citation86]. Furthermore, to control for cell-type heterogeneity, the top 10 component variables derived from surrogate variable analysis (SmartSVA package) were selected, a reference-free method that efficiently estimates cell-type mixture in heterogeneous tissues using iteratively re-weighted least squares [Citation87–Citation89].
Second-hand tobacco smoke exposure, ascertained by maternal interview, was used as the measure of prenatal smoke exposure because pregnant women often underreport first-hand smoking [Citation90,Citation91]. Missing covariates, both continuous and categorical, were simultaneously imputed using a random forest trained on observed values of the data matrix, assessing for complex interactions and non-linear relations (missForest package) [Citation92]. Imputation was assessed with an out-of-bag imputation error estimate.
Robust linear regression models were fit to address our question of whether maternal smoking exposure would explain potential associations between SES indicators and DNA methylation. In these models, test statistics were modified using the Phipson’s robust empirical Bayes procedure, shrinking probe-wise sample variances towards a common value and controlling for test-statistic inflation [Citation93]. First, models adjusted for maternal age, race, infant sex, birth weight, GA, acute inflammation of the placenta chorion, and the first 10 principal components estimated using SmartSVA as proxy for placenta cellular heterogeneity, without controlling for smoking status. Second, smoking exposure was added to the model to explore whether maternal smoking exposure might account for any relationship between SES factors and DNA methylation. Overall, these models were largely consistent, and we used the final model with smoking for statistical inference. Statistically significant associations were identified based on Benjamini-Hochberg FDR q-values using a significance level of < 0.1 [Citation94].
Sex-specific associations were assessed via stratification of adjusted robust linear regression models, including second-hand smoke exposure, and excluding sex of the infant as covariates. Additionally, in the sex-stratified analysis, we only used the autosomes. Because our sample size is reduced by half for sex stratification analysis, we adopted a conservative FDR of < 0.01 to determine statistical significance. Manhattan plots and QQ-plots were used to report results. QQ-plots here plot theoretical quantiles of the t-distribution on the x-axis against the empirical modified t-statistics estimated using the empirical Bayes procedure, showing potential test-statistic inflation at tails of the inferential distribution. The interpretation of these QQ-plots is equivalent to those that plot theoretical and sample p values. Traditional inflation factors are not interpretable in this empirical Bayes setting, often underrepresenting inflation in test statistics.
As secondary analysis, we tested for differentially methylated regions in relationship to SES factors using the DMRcate with an FDR < 0.1. All analysis was performed using R, version 3.4.3 [Citation95].
Ingenuity pathway analysis
In order to examine the higher-level system biological functions and processes, canonical pathways, and networks among the differentially methylated genes were assessed using Ingenuity Pathway Analysis (IPA) (Ingenuity Systems®, Redwood City, CA, USA). A data set containing the genes identified in DNA methylation analysis was mapped using IPA to generate putative networks based on the manually curated knowledge database of pathway interactions extracted from the literature. The gene network was generated using both direct and indirect relationships/connectivity. These networks were ranked by scores that measured the probability that the genes were included in the network by chance alone.
Supplemental Material
Download Zip (6.6 MB)Disclosure statement
No potential conflict of interest was reported by the authors.
Supplementary material
Supplemental data for this article can be accessed here.
Additional information
Funding
References
- Braveman PA, Cubbin C, Egerter S, et al. Socioeconomic status in health research: one size does not fit all. JAMA. 2005;294(22):2879–2888.
- Chen E, Miller GE. Socioeconomic status and health: mediating and moderating factors. Annu Rev Clin Psychol. 2013;9:723–749. PubMed PMID: 23245339; eng.
- Wolfe B, Evans W, Seeman TE. The biological consequences of socioeconomic inequalities. New York: Russell Sage Foundation; 2012.
- Blumenshine P, Egerter S, Barclay CJ, et al. Socioeconomic disparities in adverse birth outcomes: a systematic review. Am J Prev Med. 2010 Sep;39(3):263–272. PubMed PMID: 20709259; eng.
- Bradley RH, Corwyn RF. Socioeconomic status and child development. Annu Rev Psychol. 2002;53:371–399. PubMed PMID: 11752490; eng.
- Power C, Hypponen E, Smith GD. Socioeconomic position in childhood and early adult life and risk of mortality: a prospective study of the mothers of the 1958 British birth cohort. Am J Public Health. 2005 Aug;95(8):1396–1402. PubMed PMID: 15985645; PubMed Central PMCID: PMCPMC1449372.
- Ehrlich KB, Ross KM, Chen E, et al. Testing the biological embedding hypothesis: is early life adversity associated with a later proinflammatory phenotype? Dev Psychopathol. 2016;28(4pt2):1273–1283. PubMed PMID: 27691981.
- Kim P, Evans GW, Chen E, et al. How socioeconomic disadvantages get under the skin and into the brain to influence health development across the lifespan. In: Halfon N, Forrest CB, Lerner RM, et al., editors. Handbook of life course health development. Cham: Springer International Publishing; 2018. 463–497.
- Janicki-Deverts D, Cohen S, Matthews KA, et al. Socioeconomic status, antioxidant micronutrients, and correlates of oxidative damage: the Coronary Artery Risk Development in Young Adults (CARDIA) study. Psychosom Med. 2009 Jun;71(5):541–548. PubMed PMID: 19414620; PubMed Central PMCID: PMCPMC3925505. eng.
- Menezo YJ, Silvestris E, Dale B, et al. Oxidative stress and alterations in DNA methylation: two sides of the same coin in reproduction. Reprod Biomed Online. 2016 Dec;33(6):668–683. PubMed PMID: 27742259; eng.
- Muscatell KA, Brosso SN, Humphreys KL. Socioeconomic status and inflammation: a meta-analysis. Mol Psychiatry. 2018. DOI:10.1038/s41380-018-0259-2. PubMed PMID: Muscatell2018.
- Stringhini S, Polidoro S, Sacerdote C, et al. Life-course socioeconomic status and DNA methylation of genes regulating inflammation. Int J Epidemiol. 2015 Aug;44(4):1320–1330. PubMed PMID: 25889032; eng.
- Needham BL, Smith JA, Zhao W, et al. Life course socioeconomic status and DNA methylation in genes related to stress reactivity and inflammation: the multi-ethnic study of atherosclerosis. Epigenetics. 2015;10(10):958–969. PubMed PMID: 26295359; PubMed Central PMCID: PMCPMC4844216. eng.
- Appleton AA, Armstrong DA, Lesseur C, et al. Patterning in placental 11-B hydroxysteroid dehydrogenase methylation according to prenatal socioeconomic adversity. PLoS One. 2013;8(9):e74691.
- Karlsson Linner R, Marioni RE, Rietveld CA, et al. An epigenome-wide association study meta-analysis of educational attainment. Mol Psychiatry. 2017 Dec;22(12):1680–1690. PubMed PMID: 29086770; eng.
- Alfano R, Guida F, Galobardes B, et al. Socioeconomic position during pregnancy and DNA methylation signatures at three stages across early life: epigenome-wide association studies in the ALSPAC birth cohort. Int J Epidemiol. 2018;48(1):30–44.
- Palma-Gudiel H, Cirera F, Crispi F, et al. The impact of prenatal insults on the human placental epigenome: A systematic review. Neurotoxicol Teratol. 2018 Jan 4. DOI:10.1016/j.ntt.2018.01.001. PubMed PMID: 29307795; eng.
- Burton GJ, Fowden AL, Thornburg KL. Placental origins of chronic disease. Physiol Rev. 2016 Oct;96(4):1509–1565. PubMed PMID: 27604528; eng.
- Robinson WP, Price EM. The human placental methylome. Cold Spring Harb Perspect Med. 2015;55:a023044–a023044. PubMed PMID: 25722473.
- Martin EM, Fry RC. Environmental influences on the epigenome: exposure- associated DNA methylation in human populations. Annu Rev Public Health. 2018 Apr 1;39:309–333.
- Lester BM, Conradt E, Marsit CJ. Are epigenetic changes in the intrauterine environment related to newborn neurobehavior? Epigenomics. 2014 Apr;6(2):175–178. PubMed PMID: 24811786; PubMed Central PMCID: PMCPMC4407197.
- Paquette AGL BM, Lesseur C, Armstrong DA, et al. Placental epigenetic patterning of glucocorticoid response genes is associated with infant neurodevelopment [Research article]. Epigenomics. 2015;7(5):767–779.
- Martin E, Smeester L, Bommarito PA, et al. Sexual epigenetic dimorphism in the human placenta: implications for susceptibility during the prenatal period. Epigenomics. 2017 Mar;9(3):267–278. PubMed PMID: 28234023; PubMed Central PMCID: PMCPMC5331919. eng.
- Meakin CJ, Martin EM, Santos HP Jr., et al. Placental CpG methylation of HPA-axis genes is associated with cognitive impairment at age 10 among children born extremely preterm. Horm Behav. 2018 May;101:29–35. PubMed PMID: 29477804; eng.
- Lester BM, Marsit CJ. Epigenetic mechanisms in the placenta related to infant neurodevelopment. Epigenomics. 2018 Mar 1;10(3):321–333. PubMed PMID: 29381081; eng.
- Nelson CA. Hazards to early development: the biological embedding of early life adversity. Neuron. 2017 Oct;96(2):262–266. PubMed PMID: 29024653; eng.
- Osler M, Andersen AM, Batty GD, et al. Relation between early life socioeconomic position and all cause mortality in two generations. A longitudinal study of Danish men born in 1953 and their parents. J Epidemiol Community Health. 2005 Jan;59(1):38–41. PubMed PMID: 15598724; PubMed Central PMCID: PMCPMC1763354. eng.
- Poulton R, Caspi A, Milne BJ, et al. Association between children‘s experience of socioeconomic disadvantage and adult health: a life-course study. Lancet. 2002 Nov 23;360(9346):1640–1645. PubMed PMID: 12457787; PubMed Central PMCID: PMCPMC3752775. eng.
- Christensen DL, Schieve LA, Devine O, et al. Socioeconomic status, child enrichment factors, and cognitive performance among preschool-age children: results from the follow-up of growth and development experiences study. Res Dev Disabil. 2014 Jul;35(7):1789–1801. PubMed PMID: 24679548; PubMed Central PMCID: PMCPMC4997613. eng.
- Piccolo LR, Merz EC, He X, et al. Age-related differences in cortical thickness vary by socioeconomic status. PLoS One. 2016;11(9):e0162511. PubMed PMID: 27644039; PubMed Central PMCID: PMCPMC5028041. eng.
- Shaked D, Katzel LI, Seliger SL, et al. Dorsolateral prefrontal cortex volume as a mediator between socioeconomic status and executive function. Neuropsychology. 2018 Nov;32(8):985–995. PubMed PMID: 30211609; PubMed Central PMCID: PMCPMC6234054. eng.
- Arrhenius B, Gyllenberg D, Chudal R, et al. Social risk factors for speech, scholastic and coordination disorders: a nationwide register-based study. BMC Public Health. 2018 Jun 15;18(1):739. PubMed PMID: 29902994; PubMed Central PMCID: PMCPMC6002992. eng.
- Chin-Lun Hung G, Hahn J, Alamiri B, et al. Socioeconomic disadvantage and neural development from infancy through early childhood. Int J Epidemiol. 2015 Dec;44(6):1889–1899. PubMed PMID: 26675752; PubMed Central PMCID: PMCPMC4715254. eng.
- Farah MJ. The neuroscience of socioeconomic status: correlates, causes, and consequences. Neuron. 2017 Sep;96(1):56–71. PubMed PMID: 28957676; eng.
- Sakai D, Dixon J, Mj D, et al. Mammalian neurogenesis requires Treacle-Plk1 for precise control of spindle orientation, mitotic progression, and maintenance of neural progenitor cells. PLoS Genet. 2012;8(3):e1002566–e1002566. PubMed PMID: 22479190.
- Gaudet P, Livstone MS, Lewis SE, et al. Phylogenetic-based propagation of functional annotations within the Gene Ontology consortium. Brief Bioinform. 2011 Sep;12(5):449–462. PubMed PMID: 21873635; PubMed Central PMCID: PMCPMC3178059. eng.
- Byun Y-S, Kim E-K, Araki K, et al. Fryl deficiency is associated with defective kidney development and function in mice. Exp Biol Med. 2018;243(5):408–417. PubMed PMID: 29409347; eng.
- Crosley EJ, Elliot MG, Christians JK, et al. Placental invasion, preeclampsia risk and adaptive molecular evolution at the origin of the great apes: evidence from genome-wide analyses. Placenta. 2013 Feb;34(2):127–132. PubMed PMID: 23266291; eng.
- Perez-Garcia V, Fineberg E, Wilson R, et al. Placentation defects are highly prevalent in embryonic lethal mouse mutants. Nature. 2018 Mar;555(7697):463–468. PubMed PMID: 29539633; PubMed Central PMCID: PMCPMC5866719. eng.
- Sharp AN, Heazell AE, Crocker IP, et al. Placental apoptosis in health and disease. Am J Reprod Immunol. 2010 Sep;64(3):159–169. PubMed PMID: 20367628; PubMed Central PMCID: PMCPMC3025811. eng.
- Das M, Fau XB, Lin L, et al. Phosphatidylserine efflux and intercellular fusion in a BeWo model of human villous cytotrophoblast. Placenta. 2004;25(5):396–407. eng.
- Bano D, Prehn JHM. Apoptosis-Inducing Factor (AIF) in physiology and disease: the tale of a repented natural born killer. EBio Medicine. 2018 Apr;30:29–37. PubMed PMID: 29605508; PubMed Central PMCID: PMCPMC5952348. eng.
- Morton SU, Prabhu SP, Lidov HGW, et al. AIFM1 mutation presenting with fatal encephalomyopathy and mitochondrial disease in an infant. Cold Spring Harb Mol Case Stud. 2017;3(2):a001560–a001560. PubMed PMID: 28299359.
- Diodato D, Tasca G, Verrigni D, et al. A novel AIFM1 mutation expands the phenotype to an infantile motor neuron disease. Eur J Human Genet. 2016;24(3):463–466. PubMed PMID: 26173962.
- Berger I, Ben-Neriah Z, Dor-Wolman T, et al. Early prenatal ventriculomegaly due to an AIFM1 mutation identified by linkage analysis and whole exome sequencing. Mol Genet Metab. 2011 Dec;104(4):517–520. PubMed PMID: 22019070; eng.
- Beach SR, Dogan MV, Brody GH, et al. Differential impact of cumulative SES risk on methylation of protein-protein interaction pathways as a function of SLC6A4 genetic variation in African American young adults. Biol Psychol. 2014 Feb;96:28–34. PubMed PMID: 24192273; PubMed Central PMCID: PMCPMC3946695. eng.
- Bale TL. The placenta and neurodevelopment: sex differences in prenatal vulnerability. Dialogues Clin Neurosci. 2016;18(4): 459–464. PubMed PMID: 28179817; eng.
- DiPietro JA, Voegtline KM. The gestational foundation of sex differences in development and vulnerability. Neuroscience. 2017;342:4–20. PubMed PMID: 26232714; eng.
- Uhlen M, Fagerberg L, Hallstrom BM, et al. Proteomics. Tissue-based map of the human proteome. Science. 2015 Jan 23;347(6220):1260419. PubMed PMID: 25613900; eng.
- MacArthur J, Bowler E, Cerezo M, et al. The new NHGRI-EBI catalog of published genome-wide association studies (GWAS catalog). Nucleic Acids Res. 2017;45(D1):D896–D901. PubMed PMID: 27899670; eng.
- Imamura M, Takahashi A, Yamauchi T, et al. Genome-wide association studies in the Japanese population identify seven novel loci for type 2 diabetes. Nat Commun. 2016 Jan 28;7:10531. PubMed PMID: 26818947; PubMed Central PMCID: PMCPMC4738362. eng.
- Stringhini S, Zaninotto P, Kumari M, et al. Lifecourse socioeconomic status and type 2 diabetes: the role of chronic inflammation in the English longitudinal study of ageing [Article]. Sci Rep. 2016 Apr 22 6:24780. https://www.nature.com/articles/srep24780#supplementary-information
- Volaco A, Cavalcanti AM, Filho RP, et al. Socioeconomic status: the missing link between obesity and diabetes mellitus? Curr Diabetes Rev. 2018;14(4):321–326. PubMed PMID: 28637406; eng.
- Fagerberg L, Hallstrom BM, Oksvold P, et al. Analysis of the human tissue-specific expression by genome-wide integration of transcriptomics and antibody-based proteomics. Mol Cell Proteomics. 2014 Feb;13(2):397–406. PubMed PMID: 24309898; PubMed Central PMCID: PMCPMC3916642. eng.
- Borghol N, Suderman M, McArdle W, et al. Associations with early-life socio-economic position in adult DNA methylation. Int J Epidemiol. 2012;41(1):62–74. PubMed PMID: 22422449; eng.
- Fedotova AA, Bonchuk AN, Mogila VA, et al. C2H2 zinc finger proteins: the largest but poorly explored family of higher eukaryotic transcription factors. Acta Naturae. 2017;9(2):47–58. Apr-Jun. PubMed PMID: 28740726; PubMed Central PMCID: PMCPMC5509000. eng.
- Wen H, Chen L, He J, et al. MicroRNA expression profiles and networks in placentas complicated with selective intrauterine growth restriction. Mol Med Rep. 2017;16(5):6650–6673. PubMed PMID: 28901463; eng.
- Luciano M, Huffman JE, Arias-Vásquez A, et al. Genome-wide association uncovers shared genetic effects among personality traits and mood states. Am J Med Genet B Neuropsychiatr Genet. 2012 Sep;159B(6):684–695. PubMed PMID: 22628180; PubMed Central PMCID: PMCPMC3795298. eng.
- Zhou Y, Lutz PE, Wang YC, et al. Global long non-coding RNA expression in the rostral anterior cingulate cortex of depressed suicides. Transl Psychiatry. 2018 Oct;8(1):224. PubMed PMID: 30337518; PubMed Central PMCID: PMCPMC6193959. eng.
- Lin M, Pedrosa E, Hrabovsky A, et al. Integrative transcriptome network analysis of iPSC-derived neurons from schizophrenia and schizoaffective disorder patients with 22q11.2 deletion. BMC Syst Biol. 2016 Nov;10(1):105. PubMed PMID: 27846841; PubMed Central PMCID: PMCPMC5111260. eng.
- Jiang-Xie LF, Liao HM, Chen CH, et al. Autism-associated gene Dlgap2 mutant mice demonstrate exacerbated aggressive behaviors and orbitofrontal cortex deficits. Mol Autism. 2014;5:32. PubMed PMID: 25071926; PubMed Central PMCID: PMCPMC4113140. eng.
- Kimura Y, Matsunami H, Inoue T, et al. Cadherin-11 expressed in association with mesenchymal morphogenesis in the head, somite, and limb bud of early mouse embryos. Dev Biol. 1995 May;169(1):347–358. PubMed PMID: 7750650; eng.
- Hertel N, Redies C. Absence of layer-specific cadherin expression profiles in the neocortex of the reeler mutant mouse. Cereb Cortex. 2011 May;21(5):1105–1117. . PubMed PMID: 20847152; eng.
- Faure S, Fort P. Atypical RhoV and RhoU GTPases control development of the neural crest. PubMed PMID: 26555387 Small GTPases. 2015;64:174–177.
- Hsu SF, Su WC, Jeng KS, et al. A host susceptibility gene, DR1, facilitates influenza A virus replication by suppressing host innate immunity and enhancing viral RNA replication. J Virol. 2015 Apr;89(7):3671–3682. PubMed PMID: 25589657; PubMed Central PMCID: PMCPMC4403386. eng.
- Zhang S, Li J, Yang Y, et al. Up-regulation of microRNA-203 in influenza A virus infection inhibits viral replication by targeting DR1. Sci Rep. 2018 May;8(1):6797. PubMed PMID: 29717211; PubMed Central PMCID: PMCPMC5931597. eng.
- Ramos-Lopez O, Riezu-Boj JI, Milagro FI, et al. Dopamine gene methylation patterns are associated with obesity markers and carbohydrate intake. Brain Behav. 2018 Aug;8(8):e01017. PubMed PMID: 29998543; PubMed Central PMCID: PMCPMC6085894. eng.
- Yang YC, Gerken K, Schorpp K, et al. Early-life socioeconomic status and adult physiological functioning: a life course examination of biosocial mechanisms. Biodemography Soc Biol. 2017;63(2):87–103. PubMed PMID: 28521624; PubMed Central PMCID: PMCPMC5439296. eng.
- Azad MB, Lissitsyn Y, Miller GE, et al. Influence of socioeconomic status trajectories on innate immune responsiveness in children. PLoS One. 2012;7(6):e38669. PubMed PMID: 22685596; PubMed Central PMCID: PMCPMC3369855. eng.
- Dowd JB, Aiello AE. Socioeconomic differentials in immune response. Epidemiology. 2009 Nov;20(6):902–908. PubMed PMID: 19797966; PubMed Central PMCID: PMCPMC2765221. eng.
- Chen E, Fisher EB, Bacharier LB, et al. Socioeconomic status, stress, and immune markers in adolescents with asthma. Psychosom Med. 2003;65(6):984–992. Nov-Dec. PubMed PMID: 14645776; eng.
- Guintivano J, Sullivan PF, Stuebe AM, et al. Adverse life events, psychiatric history, and biological predictors of postpartum depression in an ethnically diverse sample of postpartum women. Psychol Med. 2017 Sep 27:1–14. DOI: 10.1017/s0033291717002641. PubMed PMID: 28950923; eng.
- Miller GE, Chen E, Fok AK, et al. Low early-life social class leaves a biological residue manifested by decreased glucocorticoid and increased proinflammatory signaling. Proc Natl Acad Sci U S A. 2009 Aug;106(34):14716–14721. PubMed PMID: 19617551; PubMed Central PMCID: PMCPMC2732821. eng.
- Ghosh S, Yates AJ, Fruhwald MC, et al. Tissue specific DNA methylation of CpG islands in normal human adult somatic tissues distinguishes neural from non-neural tissues. Epigenetics. 2010 Aug 16;5(6):527–538. PubMed PMID: 20505344; PubMed Central PMCID: PMCPMC3322498.
- O‘Shea TM, Allred EN, Dammann O, et al. The ELGAN study of the brain and related disorders in extremely low gestational age newborns. Early Hum Dev. 2009 Nov;85(11):719–725. PubMed PMID: 19765918; PubMed Central PMCID: PMCPMC2801579. eng.
- Onderdonk AB, Delaney ML, DuBois AM, et al. Detection of bacteria in placental tissues obtained from extremely low gestational age neonates. Am J Obstet Gynecol. 2008 Jan;198(1):110 e1–7. PubMed PMID: 18166321.
- Cardenas A, Gagné-Ouellet V, Allard C, et al. Placental DNA methylation adaptation to maternal glycemic response in pregnancy. Diabetes. 2018 Aug;67(8):1673–1683. PubMed PMID: 29752424; eng.
- Teschendorff AE, Zheng SC. Cell-type deconvolution in epigenome-wide association studies: a review and recommendations. Epigenomics. 2017 May;9(5):757–768. PubMed PMID: 28517979; eng.
- Aryee MJ, Jaffe AE, Corrada-Bravo H, et al. Minfi: a flexible and comprehensive Bioconductor package for the analysis of Infinium DNA methylation microarrays. Bioinformatics. 2014 May 15;30(10):1363–1369. PubMed PMID: 24478339; PubMed Central PMCID: PMCPMC4016708.
- Triche TJ Jr., Weisenberger DJ, Van Den Berg D, et al. Low-level processing of illumina infinium DNA methylation beadarrays. Nucleic Acids Res. 2013 Apr;41(7):e90. PubMed PMID: 23476028; PubMed Central PMCID: PMCPMC3627582.
- Fortin JP, Labbe A, Lemire M, et al. Functional normalization of 450k methylation array data improves replication in large cancer studies. Genome Biol. 2014 Dec;15(12):503. PubMed PMID: 25599564; PubMed Central PMCID: PMCPMC4283580. eng.
- Fortin JP, Triche TJ, Hansen KD. Preprocessing, normalization and integration of the illumina humanmethylationEPIC array with minfi. Bioinformatics. 2017 Feb;33(4):558–560. PubMed PMID: 28035024; PubMed Central PMCID: PMCPMC5408810. eng.
- Johnson WE, Li C, Rabinovic A. Adjusting batch effects in microarray expression data using empirical Bayes methods. Biostatistics. 2007 Jan;8(1):118–127. PubMed PMID: 16632515; eng.
- Du P, Zhang X, Huang CC, et al. Comparison of Beta-value and M-value methods for quantifying methylation levels by microarray analysis. BMC Bioinformatics. 2010 Nov;11:587. PubMed PMID: 21118553; PubMed Central PMCID: PMCPMC3012676. eng.
- Beach SR, Lei MK, Brody GH, et al. Parenting, socioeconomic status risk, and later young adult health: exploration of opposing indirect effects via DNA methylation. Child Dev. 2016;87(1):111–121. Jan-Feb. DOI: PubMed PMID: 26822447; PubMed Central PMCID: PMCPMC4733891. eng.
- Hecht JL, Fau OA, Delaney M, et al. Characterization of chorioamnionitis in 2nd-trimester C-section placentas and correlation with microorganism recovery from subamniotic tissues. Pediatr Dev Pathol. 2008;11:15–22, (1093-5266 (Print)). eng
- Leek JT, Johnson WE, Parker HS, et al. The sva package for removing batch effects and other unwanted variation in high-throughput experiments. Bioinformatics. 2012 Mar 15;28(6):882–883. PubMed PMID: 22257669; PubMed Central PMCID: PMCPMC3307112. eng.
- Leek JT, Storey JD. Capturing heterogeneity in gene expression studies by surrogate variable analysis. PLoS Genet. 2007;3. DOI:10.1371/journal.pgen.0030161.
- Chen J, Behnam E, Huang J, et al. Fast and robust adjustment of cell mixtures in epigenome-wide association studies with SmartSVA. BMC Genomics. 2017 May 26;18(1):413. .
- Hawkins SS, Dacey C, Gennaro S, et al. Secondhand smoke exposure among nonsmoking pregnant women in New York city. Nicotine Tob Res. 2014;16(8):1079–1084. PubMed PMID: 24642590.
- Shipton D, Tappin DM, Vadiveloo T, et al. Reliability of self reported smoking status by pregnant women for estimating smoking prevalence: a retrospective, cross sectional study. BMJ (Clin Res Ed). 2009;339:b4347–b4347. PubMed PMID: 19875845.
- Stekhoven DJ, Bühlmann P. MissForest–non-parametric missing value imputation for mixed-type data. Bioinformatics. 2012 Jan;28(1):112–118. PubMed PMID: 22039212; eng.
- Phipson B, Lee S, Majewski IJ, et al. Robust Hyperparameter Estimation Protects Against Hypervariable Genes And Improves Power To Detect Differential Expression. Ann Appl Stat. 2016;10(2):946–963. PubMed PMID: 28367255.
- Benjamini Y, Hochberg Y. Controlling the false discovery rate: a practical and powerful approach to multiple testing. J Royal Stat Soc Ser B (Methodological). 1995;57(1):289–300.
- Team RC. R: A language and environment for statistical computing. Vienna, Austria: R Foundation for Statistical Computing; 2013.