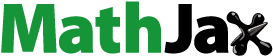
ABSTRACT
Endocrine-disrupting compounds are associated with altered epigenetic regulation and adverse health outcomes, although inconsistent results suggest that people have varied responses to the same exposure. Interpersonal variation in response to environmental exposures is not identified using standard, population-based methods. However, methods that capture an individual’s response, such as analyzing stochastic epigenetic mutations (SEMs), may capture currently missed effects of environmental exposure. To test whether polybrominated biphenyl (PBB) was associated with SEMs, DNA methylation was measured using Illumina’s MethylationEPIC array in PBB-exposed individuals, and SEMs were identified. Association was tested using a linear regression with robust sandwich variance estimators, controlling for age, sex, lipids, and cell types. The number of SEMs was variable (range: 119–18,309), and positively associated with age (p = 1.23e-17), but not with sex (p = 0.97). PBBs and SEMs were only positively associated in people who were older when they were exposed (p = 0.02 vs. p = 0.91). Many subjects had SEMs enriched in biological pathways, particularly in pathways involved with xenobiotic metabolism and endocrine function. Higher number of SEMs was also associated with higher age acceleration (intrinsic: p = 1.70e-3; extrinsic: p = 3.59e-11), indicating that SEMs may be associated with age-related health problems. Finding an association between environmental contaminants and higher SEMs may provide insight into individual differences in response to environmental contaminants, as well as into the biological mechanism behind SEM formation. Furthermore, these results suggest that people may be particularly vulnerable to epigenetic dysregulation from environmental exposures as they age.
Introduction
Endocrine-disrupting compounds (EDCs) are a class of chemicals that interfere with the endocrine system by disrupting the synthesis, binding, or transport of hormones [Citation1]. They are common in the industrialized world and are present in pesticides, personal care products, electronics, fabrics, and household dust [Citation2,Citation3]. In human populations, increased exposure to EDCs has been linked to reproductive, hormonal, and developmental health problems [Citation4–Citation8]. However, studies of the health effects of EDC exposure have reported inconsistent results, with some studies finding an association between the same EDC and the same health outcome, and others not finding an association [Citation9–Citation11]. While some of this inconsistency is due to differences in study design, population, specific exposures, and dose, it is also known that there are differences in how individuals respond to and metabolize xenobiotics [Citation12]. Therefore, it is reasonable to expect interindividual differences in the health consequences even among people with similar chemical exposures.
Exposure to EDCs has also been linked to epigenetic variation, both in cell lines and human cohorts, and these variations in epigenetic features have been suggested to be potential mediators between exposure to EDCs and the development of adverse health outcomes [Citation13–Citation19]. However, previous studies on the effect of EDCs on DNA methylation have relied on differences in either mean global DNA methylation differences (as measured by methylation of Alu, LINE, or other repetitive elements) or in the mean methylation at a particular site in the genome. For an association to be detected in these types of studies, the differences in methylation levels have to be shared by a large proportion of the cohort. While this approach is useful and effective and has found associations between exposure to EDCs, particular biological pathways, and health outcomes, it does not fully explain why people are susceptible to different health problems from the same environmental exposure.
It is possible that, in addition to affecting epigenetic marks in a way shared by a population, EDCs can also act randomly throughout the genome, altering different epigenetic marks in susceptible people and promoting distinct health outcomes. If this were so, these epigenetic differences would be missed by traditional analysis methods. Evaluation of rare stochastic epigenetic mutations (SEMs) would provide a measure of random differences in an individual. SEMs are defined as an individual having an extreme methylation value at a site when compared to the rest of the population. SEMs, by nature, are not shared among a population and do not have a substantial influence on the mean methylation level. However, despite their stochasticity, SEMs have been linked to cancer progression, ageing, and age-dependent X-inactivation skewing [Citation20,Citation21]. Therefore, they may be an important mediator between environmental exposures and health outcomes that could help explain the interpersonal variation in response to EDC exposure.
To investigate the association between exposure to environmental EDCs and SEMs, we utilized data collected as part of the Michigan PBB Registry. The Michigan PBB Registry includes approximately 7,000 individuals who were exposed to an EDC when an agricultural accident in the 1970s introduced polybrominated biphenyl (PBB) into the food supply. Individuals in this cohort were exposed to high levels of PBB during the nearly year-long period when contaminated food was distributed. Forty years later, a majority of participants still have higher PBB blood levels than 95% of the general United States [Citation22]. Previous studies in this cohort have linked PBB exposure to earlier age of menarche, genitourinary conditions in males, thyroid dysfunction, and increased risk for certain types of cancer, particularly among those were exposed to PBB at a younger age [Citation23–Citation27]. Additionally, previous epigenetic research has found that higher PBB exposure is associated with methylation proportion at 1890 CpGs across the genome, and the effect that PBB has on the epigenome is suggestive of estrogen signalling and immune dysfunction [Citation28]. However, it is not clear whether environmental stimuli, like PBB, would act randomly on the epigenome and thus associate with the number of SEMs. It is also not known if SEMs would depend on the age when they were first exposed to PBB or if they are sex-specific (like other previous research on the health effects of PBB), and it is not known if SEM count would associate with other age-related health outcomes like epigenetic age acceleration measures.
Results
Study population demographics
The study population was composed of 658 people, many of who were highly exposed to PBB (range: 0.01–236.73 ppb; ). There were more female participants than male participants in this population (381 v. 277; p = 6.87e-05). A majority of the cohort (97%) was of White/Non-Hispanic ancestry, which is representative of the population of rural Michigan in the 1970s. Higher levels of PBB were associated with both being male (ln(PBB) in men vs women: −0.99 vs. −0.36, p = 4.54e-07) and older age (r = 0.25, p = 3.96e-11). PBB level and total lipid levels were not correlated in this cohort (r = −0.07, p = 0.06). Additionally, age of exposure was highly correlated with current age (r = 0.98; p < 2.2e-16), because most of the study population was exposed during the contamination event. Half of this study population was exposed before age 13.
Table 1. Characteristics of the members of the Michigan PBB Registry with epigenetic data.
SEM calculation and characteristics
SEM values were highly variable in this cohort, with a range of 119 to 18,309, and were not normally distributed (Figure S1A). Therefore, the natural log of the number of SEMs was used for all regression analyses (Figure S1B). SEMs were present in 12.75% of probes (Figure S2). In the participants who had multiple samples, the SEM calls were highly correlated within the longitudinal pair, and not correlated with the other samples (r = 0.55–0.77; p < 2.2e-16; ), indicating that SEM calls can be replicated within individuals. The number of SEMs in these sample pairs also increased between sampling for a majority of participants (mean difference in SEMs between the later time point and the earlier time point: 22.14 ± 47.64).
Figure 1. SEMs can be replicated in longitudinal repeats. Because DNA methylation levels were measured in longitudinal repeats for 14 individuals, with the first sample taken in 2004, and the second sample taken between 2012 and 2015 (designated below by the Subject ID followed by 1 or 2 to indicate the first or second sample), SEMs were calculated in both of these samples to see if they could be replicated. The SEM calls were highly correlated within the longitudinal pairs (r = 0.55–0.77) and not correlated within samples that were non-longitudinal pairs (r = 0.00–0.10), indicating that SEMs are not just the result of technical error, and can be replicated. In a majority of individuals (64.28%), the number of SEMs increased between samples (mean: 22.1447.64).
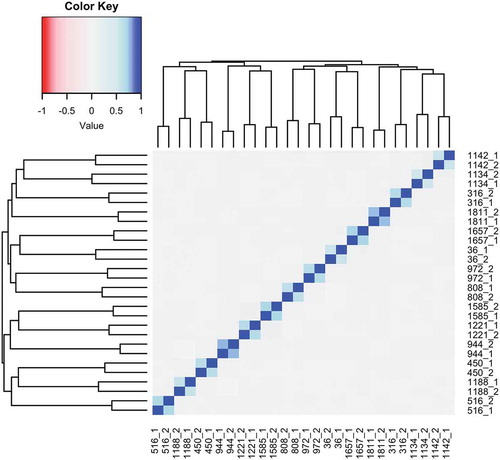
Similar to other SEM studies [Citation20], SEMs were positively associated with current age, both when unadjusted (z = 7.11; p = 1.15e-12) and when adjusted for sex and cell type composition (z = 8.54; p = 1.23e-17) (Figure S3). Both the mean and the variance in the number of SEMs were higher in people who were older when they were exposed to PBB (Figure S4). Higher proportions of CD8T cells, B cells, monocytes and NK cells were associated with an increased number of SEMs while a higher proportion of CD4T cells and granulocytes were associated with decreased number of SEMs. While age was also associated with many of these covariates, the association between these covariates and number of SEMs is independent of their association with age (Table S1).
Higher number SEMs associates with higher PBB level
Current PBB levels had a positive, but non-significant association with the number of SEMs, once age, sex, lipid level, and cell type proportion were adjusted for (z = 1.57; p = 0.11; Figure S5). This association was largely consistent when the number of SEMs and each congener of PBB were analyzed individually (p = 0.003–0.16; Figure S6). However, because SEMs were more common in older individuals, and many PBB-associated health problems have been specific to people exposed at younger ages [Citation9], we also tested whether PBB and age of exposure interacted to predict SEM count. PBB exposure occurred primarily during a 10-month period in 1973, making it possible to estimate the age of exposure to PBBs. Further analysis showed that there was a significant interaction between age at exposure to PBB and current PBB level to predict the number of SEMs (z = 2.27; p = 0.02). Stratifying the analysis by median age of exposure showed that there was an association between PBB level and SEM count, but only in the participants who were older when they were exposed to PBB (t = 2.22, p = 0.02 vs. z = 0.10, p = 0.91; ).
Figure 2. Higher SEM count associates with higher exposure to PBB in those exposed when they were older. The interaction between age of exposure and current total PBB level is associated with the number of SEMs (z = 2.27; p = 0.02). The association between total PBB and SEMs was only significant in participants who were exposed when they were older (black triangles, solid line) (z = 2.22; p = 0.02). For participants exposed to PBB when they were younger, the association between PBB and SEM count was not significant (grey circles, dashed line) (t = 0.20; p = 0.91). Figure was plotted on a log10 scale for ease of interpretation, but the statistical analysis was done as described in methods.
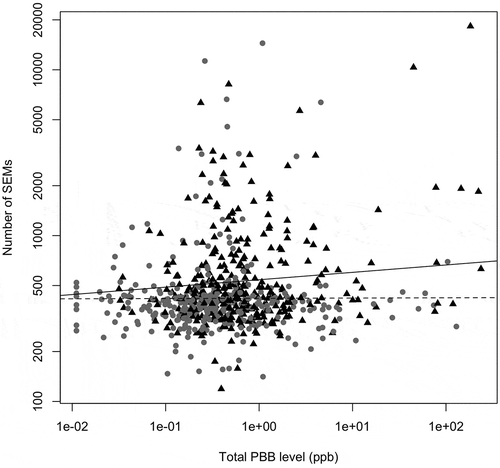
SEMs by sex
SEM count was not different between men and women, once age and cell type proportions were adjusted for (z = −0.03, p = 0.97, Figure S7). There was also no interaction between sex and PBB level to predict SEM count (z = 1.06, p = 0.28). When the population was stratified by sex, the association between PBB and number of SEMs was non-significant in both males (z = 1.23, p = 0.21) and females (z = 0.63, p = 0.52).
SEMs are enriched in biological pathways
To test whether SEMs were more likely to be in certain biological pathways, and thus identify possible biological mechanisms behind SEM formation, an enrichment test was conducted with biological pathways defined by KEGG on the probes with SEMs. Of the 331 pathways tested, the CpGs with the most SEMs were significantly enriched in 94 of them (FDR < 0.05; Table S2). Of these 94, 55.3% are directly related to signalling pathways, and many others are related to cell cycle regulation and autophagy.
Next, to test whether the SEMs were randomly distributed across the genome of each participant or if some participants had clusters of SEMs in particular biological pathways, enrichment tests were conducted with biological pathways defined by KEGG. Of the 658 participants, 129 participants had SEMs enriched in at least one KEGG pathway, while 529 participants had no enrichment of their SEMs in any pathway tested. Participants whose SEMs were enriched in any KEGG pathway had a higher number of SEMs (geometric mean: 783.67 vs. 423.16; p-value < 2.2e-16), and a higher level of PBB (geometric mean: 0.68 vs. 0.44 ppb; p-value = 0.03) compared to the participants who had no enrichment (Figure S8). Men and women were equally likely to have pathway enrichment (p = 0.22). Participants whose SEMs were enriched in at least one KEGG pathway were also older compared to those whose SEMs were not enriched in any KEGG pathway (mean: 57.68 vs. 53.45 years; p-value = 0.002).
Of the 331 KEGG pathways tested, 85 pathways were enriched in at least one participant (Table S3). A majority (51.7%) were only enriched in one person, emphasizing the interpersonal variation of SEMs. However, there were 10 pathways that were enriched in more than 30 of the 129 participants (Figure S9). All of the pathways that were enriched in more than 30 participants were involved in endocrine function or xenobiotic metabolism. However, only one of these pathways was more likely to be enriched in people with high PBB level (compared to the median) (). Many of these pathways were more likely to occur in male participants compared to female participants (), indicating that SEMs formation and susceptibility may be under sex-specific influences. Participants with a higher number of SEMs (compared to the median) were not more likely to have enrichment of the common biological pathways (p = 0.42–1; Table S4), suggesting that the association between higher PBB exposure or sex and enrichment in these KEGG pathways was not due to the association between higher PBB exposure or sex and higher SEM exposure. These common pathways were not enriched for the pathways found enriched in the probes with SEMs (OR: 0.30, 95% CI: 0.01–1.69, p = 0.19).
Table 2. People in the Michigan PBB Registry have SEMs enriched in biological pathways related to xenobiotic metabolism and hormone regulation.
SEM count associates with other well-characterized biomarkers of age
To test for potential health outcomes associated with higher SEM count, we tested the association between SEM count and two measures of age acceleration. Age acceleration was chosen because it associates with many health conditions and lifestyle factors [Citation29–Citation42], and because it is known from previous research that SEM count associates with age and age-related conditions [Citation20]. SEM count was positively associated with both intrinsic age acceleration (z = 3.13, p = 1.70e-3, )) and extrinsic age acceleration (z = 6.61, p = 3.59e-11, )).
Figure 3. Higher number of SEMs is associated with age acceleration. The number of SEMs is positively associated with two measures of age acceleration (intrinsic: z = 3.13, p = 1.70e-3, Part A; extrinsic: z = 6.61, p = 3.59e-11, Part B), even when adjusted for age, sex, and estimated cell type proportions. Figure was plotted on a log10 scale for ease of interpretation, but the statistical analysis was done as described in methods.
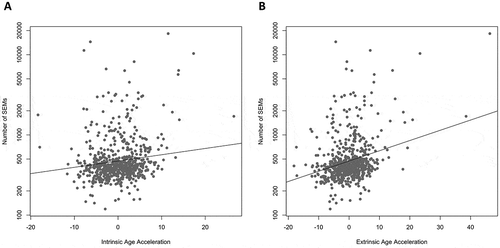
Discussion
While the association between environmental contaminants, including PBB, and DNA methylation levels shared by a population has been demonstrated before [Citation13–Citation15,Citation28,Citation43–Citation46], this is the first study to show that increased exposure to endocrine-disrupting compounds associates with an increased number of stochastic epigenetic mutations in individuals. This study utilized samples from the Michigan PBB Registry, a cohort of people who were highly exposed to PBB due to an agricultural accident and still have PBB levels well above the national average. Increased level of PBB associated with increased number of SEMs in people who were exposed when they were older, even when controlling for age, sex, lipid levels, and estimated cell type proportions. These results suggest that the number of random SEMs throughout a person’s genome may depend not only on age, as has been shown before, but also on exposure to environmental contaminants [Citation20].
The finding that PBB and SEM count only associated in the people who were exposed when they were older was the opposite of what was expected, given that many of the health consequences associated with PBB exposure, like earlier age of menarche, increased risk of spontaneous abortions, and offspring with genitourinary conditions, were in participants exposed in utero or in early childhood [Citation23,Citation24,Citation47]. This could suggest that the mechanisms of SEM formation and the mechanisms for endocrine-related health problems are distinct and that populations exposed to different developmental time-points may differ in their risk for each. Additionally, this could be a reason that there are fewer health problems statistically associated with PBB exposure in people exposed as adults since each person may have different epigenetic marks affected and thus different health outcomes [Citation9].
Because age at exposure and current age are highly correlated, finding an association with SEM count in older people could also suggest that people are more vulnerable to SEM formation from environmental exposure as they age. If vulnerability increases with age, people who were exposed when they were younger may become more susceptible to health conditions from environmental exposures as they age and gain more SEMs. This would indicate that some of the consequences of environmental contaminants like PBB would not be seen for decades and would be difficult to identify with standard epidemiological approaches. Unfortunately, while this cohort is unique in that the age when they were exposed to PBB can be estimated because the age at exposure and their current age are so correlated, these two possibilities cannot be separated.
We also analyzed whether any of the participants’ SEMs were enriched in any biological pathway or whether they were all randomly distributed throughout the genome. While a majority of participants did not have any biological pathway enriched, 19.6% of participants had SEMs enriched in at least one biological pathway. Participants with higher numbers of SEMs and higher PBB were more likely to have their SEMs enriched in at least one biological pathway. While pathways related to immune function, xenobiotic metabolism, and estrogen signalling have been suggested by previous epigenetic studies in this population [Citation28], and many of the pathways enriched in this analysis are related to xenobiotic metabolism and hormone signalling, most of these 85 pathways enriched in participants have not previously been implicated in epigenetic analyses in these participants. These participants may be more likely to have adverse health effects since the epigenetic dysregulation accumulated within a biological pathway. It is also possible that the extreme difference in epigenetic regulation in certain biological pathways is compensatory. This would indicate that these participants are upregulating certain biological pathways in response to PBB. However, given the diversity of pathways affected in the participants, both of these possibilities are difficult to test with standard statistical methods.
There were several people who had SEMs enriched in biological pathways related to xenobiotic metabolism and endocrine function, even though most of these pathways were not more likely to have high SEM formation. We found that people with higher levels of PBB compared to the median were only more likely to have SEMs in one biological pathway (neuroactive ligand–receptor interaction), and people with higher numbers of SEMs were not more likely to have SEMs in any of these biological pathways. Enrichment of these pathways in multiple participants of the Michigan PBB Registry (especially since they are the only pathways enriched in multiple participants) is of interest because cytochrome 450 was observed to be upregulated in rats after they were exposed to PBB [Citation48–Citation50], and altered steroid hormone levels and endocrine disruption was found to be associated with PBB exposure, both in animal models [Citation51–Citation53] and in epidemiological studies [Citation23,Citation24,Citation54,Citation55]. Even though there was no enrichment of these pathways in people with increased exposure compared to other people in this study, part of this could be because of the extremely high PBB exposure in this population (median exposure is 27 times higher than the national average), thus making it a comparison of high exposure to higher exposure [Citation22]. Because most people in this study have high PBB exposure, it is still possible that these pathways are upregulated following exposure to PBB, and this upregulation makes them more vulnerable to epigenetic mutations. While it has not been tested whether SEMs are more likely to form in active regions of the genome, these pathways have been associated with PBB exposure previously. Enrichment of biological pathways among our results could support the presence of an underlying biological mechanism by which SEMs contribute to health problems.
While SEMs were enriched in some biological pathways in multiple people, it is important to note that a majority of SEMs are randomly dispersed in different genomic locations in different people. Not all participants had the same pathways enriched, with only 129 of the 658 participants having enrichment of SEMs in any biological pathway and 44 of the 85 enriched pathways only being present in one person. The diversity and number of pathways enriched may help explain the individual susceptibility to different diseases following exposure to PBB, where individuals with epimutations in one biological pathway would have different health outcomes than individuals with epimutations in other biological pathways.
Beyond associations with environmental exposure, this study investigated associations with SEM formation and with sex. We found that SEM count, the association of PBB with SEM count, and the odds for enrichment of any biological pathways did not differ by sex. However, the enrichment of SEM in those common pathways involved in xenobiotic metabolism and hormonal regulation was more likely to occur in men compared to women. This could indicate distinct mechanisms behind SEM formation for men and women. It could also suggest that men may be more vulnerable to epigenetic dysregulation following exposure to PBB. While sex-specific epigenetic effects have not been studied with PBB exposure, epigenetic studies of the structurally related polychlorinated biphenyl (PCB) in other populations have reported that men are more vulnerable to epigenetic effects from EDC exposure [Citation56].
We found that the CpGs with SEMs called were more likely to be in biological pathways directly involved with signalling, cell cycle regulation, and autophagy. This could indicate areas of the genome that are active and involved in signal transduction and cell division are more likely to have an error in their epigenetic regulation that leads to an SEM. While this would be consistent with the finding of xenobiotic metabolism and hormone signalling pathways enriched in members of the Michigan PBB Registry (since these pathways are expected to be upregulated following exposure to PBB), again, this has not directly been tested. More research is needed to investigate whether these pathways replicate in other studies of SEMs as well as to test mechanisms behind SEM formation.
This study also found that an increased number of SEMs was associated with age acceleration (as measured by both intrinsic age acceleration and extrinsic age acceleration) [Citation34]. This is the first report of the association between SEM count and more established measures of age acceleration, but it is consistent given that SEM count is associated with older age and age-related health problems like cancer [Citation20,Citation21]. This could also mean that the same mechanisms that lead to the ageing process also lead to SEM formation. Additionally, because age acceleration has been associated with a myriad of adverse health outcomes, such as cancer, cardiovascular disease, and all-cause mortality [Citation36,Citation39,Citation57,Citation58], this could also indicate that an increase in SEM count from environmental exposure to EDCs could increase the risk for health problems later in life. However, more research is needed to test whether this association between SEM count and age acceleration replicates in other cohorts, as well as to test whether increased SEM count leads to increased age acceleration, or if an increase in age acceleration leads to more epigenetic dysregulation.
The findings from this study should be interpreted in light of its limitations. First, DNA methylation was only measured in blood samples, and therefore, it is not known if environmental exposure would associate with increased SEM count in other tissues. Also, it is not known if population-level exposure to other endocrine-disrupting compounds would also associate with number of SEMs, because only PBB levels were tested. Finally, while other studies have found an association between number of SEMs and health conditions, such as X-inactivation skewing and cancer progression [Citation20,Citation21], there were not enough participants with SEMs enriched in the same biological pathways for this to be directly tested in this cohort. Therefore, it is not known whether SEMs would mediate health conditions associated with PBB exposure in this cohort.
However, this study does have several strengths. We were able to implement a very stringent quality control pipeline by both removing probes with potential SNPs and implementing a lower detection p-value so that we could be more confident in our SEM calls. Additionally, because exposure to PBB occurred as part of a unique environmental contamination event, the age at which people were first exposed to PBB can be inferred from the available data, which allowed for the interaction between age at exposure and PBB level to be tested. In addition, in this study population, we were able to show that technical replicates were highly correlated, that the longitudinal pairs were highly correlated with themselves and uncorrelated to other samples, and that SEM calls in multiple tissues from the same person had very low correlation – supporting that SEMs are biological, epigenetic mutations that can be replicated and are not just the result of technical noise or underlying genetic variation. We were also able to replicate other studies finding that SEM count had a strong, positive association with increasing age, further supporting that these SEMs are biological, epigenetic mutations.
In conclusion, PBB exposure level is associated with increased number of SEMs, but only in people exposed when they were older. To our knowledge, this is the first report of environmental exposures associating with the number of rare, random, epigenetic mutations. It is also the first report of these SEMs being enriched in many biological pathways, especially in male participants. In addition, we found that SEMs are more common in signalling pathways, which may give insight into how SEMs are formed and which regions are more susceptible to SEM formation. We also found that SEM count is associated with increased age acceleration, which has not been shown before in previous studies. These SEMs may be important for understanding the variety of adverse health outcomes that are associated with EDC exposure and may mediate downstream effects on an individual’s health risks. More studies are warranted to test whether the adverse health outcomes reported by this population associate with the epigenetic mutations in their genome, and whether other environmental exposures associate with the formation of SEMs in particular biological pathways.
Methods
Participant selection
Participants were selected from the Michigan PBB Registry. The Michigan PBB Registry was started by the Michigan Department of Community Health (MDCH) after the cause of the agricultural accident was identified as PBB. The MDCH recruited individuals that lived on farms quarantined because of PBB contamination, obtained food from quarantined farms, and chemical workers and their families. This registry has recently been transferred to Emory University and has continued to enroll participants, collect biological samples, and survey health outcomes (http://pbbregistry.emory.edu/). For the current analyses, participants were selected from the registry if they met the following criteria: 1) they were exposed to PBB prior to being 50 years old, 2) they had a recent (2004-present) buffy coat or whole blood sample available for DNA extraction, and 3) they had current PBB and lipid level measurements in their serum. Informed consent was obtained from each individual before participation and study protocols were approved by the Institutional Review Board at Emory University. A total of 666 participants met these criteria and were selected for this study, including 14 participants with blood samples from two time points.
Exposure assessment
Two hundred and nine possible congeners of PBB exist and are defined based on the number and position of the bromine molecules around the biphenyl rings [Citation59]. In the technical mixture of PBBs that was added to the food supply in Michigan, the primary congener was PBB-153 [Citation59–Citation61]. Exposure to four congeners of PBB (PBB-153, PBB-101, PBB-77, and PBB-180) was previously assessed in members of this registry using gas chromatography-tandem mass spectrometry [Citation62]. The limits of detection (LOD) were 2 pg/mL for PBB-153, 4.5 pg/mL for PBB-77, 3.9 pg/mL for PBB-101, and 5.6 pg/mL for PBB-180. The extraction recovery ranged from 83.2% to 99.2%. The accuracy ranged from 89% to 119% and the precision ranged from 2.8% to 8.5%.
For the purposes of this study, the value for congeners below the LOD in a sample was imputed as the LOD divided by the square root of 2 [Citation63]. The congeners were then summed to give a total PBB value per person. This was transformed using a natural log so that the distribution was less skewed. For analyses using single congeners, if the congener was detected in more than 90% of the samples, then the transformed exposure level was used in analyses. If it was detected in fewer than 90% of samples, the exposure was dichotomized for analyses (detected in sample or not).
Lipid measurement
An Abnova Triglyceride Quantification Assay Kit (Abnova Corporation) was used to measure the total triglyceride content in serum, and a Caymen Cholesterol Assay Kit (Caymen Chemical Company) was used to measure total cholesterol content in serum. Both were done according to manufacturer’s instructions. Total lipid amount was calculated based on these components as described elsewhere [Citation64,Citation65].
MethylationEPIC array
Peripheral blood samples were collected from participants as part of the ongoing Michigan PBB Registry between 2004 and 2015. Blood was spun at 3,000 rpm to separate the plasma from the buffy coat. Buffy coats were aliquoted and stored at −80°C. DNA was extracted from buffy coat samples using the QIAamp DNA Blood Mini Kit (QIAGEN, Hilden, Germany). DNA was extracted from the same samples the exposure levels were assessed on in order to limit confounding.
Methylation levels were measured from these DNA samples at >850,000 sites using the Infinium MethylationEPIC BeadChip (Illumina, San Diego, CA) [Citation66]. Briefly, 1 µg of DNA from participants’ buffy coats was bisulfite converted, amplified, fragmented, and hybridized to the BeadChip array according to the manufacturer’s instructions. Samples were randomized into 8 plates and 88 chips by age, sex, and PBB level in order to limit confounding. DNA from a stable lymphoblast line was used as a technical replicate, which was included randomly on the plates and chips (one replicate per half plate) so that technical replicates occurred in multiple positions on the chips. Samples with low signal intensity (detection p-value >1e-10) or missing data in >10% of probes, and probes with <3 beads or missing data in >10% of samples were removed using the wateRmelon package [Citation67,Citation68]. Two samples and 21,103 probes were removed for missing data. Forty-four thousand two hundred and ten previously identified cross-reactive probes, 19,681 probes on the X or Y chromosomes, and 18,100 probes with a common SNP within 5 base pairs of the assessed CpG (MAF > 1% in European populations from the Thousand Genomes Project) were also removed from the dataset [Citation69,Citation70]. To remove potential SNPs present in this population but not marked in the Thousand Genomes Project, probes with gaps (5%) in their methylation proportions in samples were identified using Gaphunter and also dropped [Citation71]. Dye-bias correction and normalization were performed using ssNOOB, and ComBAT was used to adjust for chip-to-chip batch effects [Citation72,Citation73]. Six samples that were mismatched for self-reported sex, genetically identical to other samples, or had a higher number of Mendelian errors were removed. This resulted in a final dataset of 672 samples (from 658 participants, with 14 participants having two samples) and 769,042 probes. For each individual sample at each probe, the methylation proportion (β) at that site was calculated from the methylated (M) and unmethylated (U) signal as β = M/(U + M). Cell type proportion was estimated for each sample using Houseman’s method for estimating cell type proportion with methylation signals from CpGs that are distinct in blood cell types [Citation74]. This data is publicly available at NCBI Gene Expression Omnibus (GSE116339).
SEM calculation
Samples were considered to have an SEM at a locus if their methylation level at that locus was either greater than three times the interquartile range above the third quartile or less than three times the interquartile range below the first quartile (either < or . This definition of SEMs is consistent with the approach described and validated by Gentilini and colleagues [Citation20,Citation21,Citation75]. To do this, the IQR for each of the 769,042 probes that passed QC was calculated. Then, a new data matrix was annotated to determine whether each sample’s methylation proportion was an extreme outlier for each probe. The count of all the SEMs per sample was then calculated from the new data matrix. SEMs were also identified and counted in 15 technical replicates from cell lines derived from a single CEPH sample to assess the level of technical noise that could be due to batch effects (Figure S10). Additionally, to investigate the potential effect that genetic variant could have on SEM calls, SEMs were calculated in a publicly available dataset (GSE78743) [Citation76], that contained 148 samples (11 different tissues taken from 16 different people) (Figure S11).
DNA methylation age
Two measures of DNA methylation age were calculated. Intrinsic age was calculated as the linear combination of the beta values of 353 CpGs that have been previously shown to predict chronological age in multiple tissues by Horvath [Citation34]. Extrinsic age was calculated similarly, but from 71 CpGs that have previously shown to predict chronological age in whole blood by Hannum, et. al [Citation77]. Extrinsic age acceleration was designed for use in blood samples, and so may not be well suited for other tissues. Intrinsic age acceleration, because it was developed in multiple tissues, is independent of age-related changes in blood cell composition. For this study, intrinsic and extrinsic age were calculated from the background-corrected beta values using Horvath’s publically available online calculator (https://dnamage.genetics.ucla.edu/home). Both measures first calculate a DNA methylation-based age, and then calculate the age acceleration from the residuals of the regression of DNA methylation age on chronological age.
Statistical analysis
The similarity between SEM calls called in the longitudinal pairs, the technical replicates, and the multiple tissues from the same people was assessed using a Pearson’s correlation coefficient for the presence/absence of all loci considered as part of these analyses. Linear regression models were used to determine the association between PBB serum level (as the independent variable) and the total sum of SEMs for a participant (as the dependent variable) in an analysis of the most recent blood sample from the 658 participants. Age, sex, total lipid levels, and estimated cell type proportions were included as covariates [Citation78]. Because the number of SEMs was more variable in participants who were older or had higher exposure to PBBs, robust sandwich variance estimators were calculated for each of the cross-sectional analyses. Interaction between PBB and age of exposure was evaluated by the inclusion of an interaction term (age at exposure × total PBB level) in the model with main effects for PBB level, current age, sex, total lipid level, and cell type estimates. The study population was then stratified into participants who were exposed when they were younger (age of exposure less than the median age of exposure) and participants who were exposed when they were older (age of exposure greater than the median age of exposure). The association between current PBB level and SEM count was then tested in each subset separately, with the same covariates as before. As a sensitivity analysis, the association between each of the four detected PBB congeners was also assessed using the same covariates. The association between SEM count and sex was assessed with the same model as above, but with just age and cell types as a covariate. Interaction between PBB and sex was evaluated by the inclusion of an interaction term (sex × total PBB level) to the model with PBB level, current age, sex, total lipid level, and cell type estimates. The study population was then stratified into male participants and female participants, and the association between PBB and SEM count was assessed in each sex separately, with age, lipid levels, and cell type proportions as covariates. The association between each age acceleration measure was also assessed via the same model, with age, sex, and estimated cell type proportions as covariates.
Enrichment analysis
MissMethyl was used to assess whether an individual’s SEMs were enriched in particular biological pathways (defined by KEGG) or were randomly distributed throughout the genome [Citation79]. This analysis was conducted in each sample separately. The false discovery rate (FDR) was controlled at 5% in each analysis. For pathways identified in more than 30 participants, a chi-square test was used to determine if the participants with the highest levels of PBB (based on a median split), highest number of SEMs (based on a median split) or who were male were more likely to have each of the common biological pathways enriched. Additionally, using MissMethyl, we assessed whether the probes with SEMs were enriched in any biological pathways.
Supplemental Material
Download MS Word (4.7 MB)Acknowledgments
We are grateful to the members of the Michigan PBB Registry for their participation and engagement with research studies over the past 40 years, to the Michigan Department of Health and Human Services which had the foresight to create the Registry, and to our community partners (PBB Citizens Advisory Board, Pine River Superfund Citizen Advisory Group, and the Mid-Michigan District Health Department) who continue to provide guidance and insight to the Michigan PBB Research.
Disclosure statement
No potential conflict of interest was reported by the authors.
Supplementary Material
Supplemental data for this article can be accessed here.
Additional information
Funding
References
- Bergman A, Heindel JJ, Jobling S, et al. editors. State of the science of endocrine disrupting chemicals. Geneva, Switzerland: World Health Organization; 2012.
- Porta M, Pumarega J, Gasull M, et al. Contamination from endocrine disrupters of the general population at low and high concentrations. Vitam Horm. 2014;94:167–192. PubMed PMID: 24388190.
- Frederiksen M, Vorkamp K, Thomsen M, et al. Human internal and external exposure to PBDEs – a review of levels and sources. Int J Hyg Environ Health. 2009 Mar;212(2):109–134. PubMed PMID: 18554980.
- Crain DA, Janssen SJ, Edwards TM, et al. Female reproductive disorders: the roles of endocrine-disrupting compounds and developmental timing. Fertil Steril. 2008 Oct;90(4):911–940. PubMed PMID: 18929049; PubMed Central PMCID: PMCPMC4086418.
- Scsukova S, Rollerova E, Bujnakova Mlynarcikova A. Impact of endocrine disrupting chemicals on onset and development of female reproductive disorders and hormone-related cancer. Reprod Biol. 2016 Dec;16(4):243–254. . PubMed PMID: 27692877.
- Sifakis S, Androutsopoulos VP, Tsatsakis AM, et al. Human exposure to endocrine disrupting chemicals: effects on the male and female reproductive systems. Environ Toxicol Pharmacol. 2017 Apr;51:56–70. PubMed PMID: 28292651.
- Zawatski W, Lee MM. Male pubertal development: are endocrine-disrupting compounds shifting the norms? PubMed PMID: 23709001 J Endocrinol. 2013;2182:R1–12.
- Maqbool F, Mostafalou S, Bahadar H, et al. Review of endocrine disorders associated with environmental toxicants and possible involved mechanisms. Life Sci. 2016 Jan 15;145:265–273. PubMed PMID: 26497928.
- Curtis SW, Conneely KN, Marder ME, et al. Intergenerational effects of endocrine-disrupting compounds: a review of the Michigan polybrominated biphenyl registry. Epigenomics. 2018 Jun;10(6):845–858. PubMed PMID: 29888951; eng.
- Lee DH. Evidence of the possible harm of endocrine-disrupting chemicals in humans: ongoing debates and key issues. Endocrinol Metab (Seoul). 2018 Mar;33(1):44–52. . PubMed PMID: 29589387; PubMed Central PMCID: PMCPMC5874194.
- Minguez-Alarcon L, Gaskins AJ. Female exposure to endocrine disrupting chemicals and fecundity: a review. Curr Opin Obstet Gynecol. 2017 Aug;29(4):202–211. . PubMed PMID: 28557831; PubMed Central PMCID: PMCPMC5551432.
- Tracy TS, Chaudhry AS, Prasad B, et al. Interindividual variability in cytochrome P450-mediated drug metabolism. Drug Metab Dispos. 2016 Mar;44(3):343–351. PubMed PMID: 26681736; PubMed Central PMCID: PMCPMC4767386.
- Rusiecki JA, Baccarelli A, Bollati V, et al. Global DNA hypomethylation is associated with high serum-persistent organic pollutants in Greenlandic Inuit. Environ Health Perspect. 2008 Nov;116(11):1547–1552. PubMed PMID: 19057709; PubMed Central PMCID: PMCPMC2592276.
- Kim KY, Kim DS, Lee SK, et al. Association of low-dose exposure to persistent organic pollutants with global DNA hypomethylation in healthy Koreans. Environ Health Perspect. 2010 Mar;118(3):370–374. PubMed PMID: 20064773; PubMed Central PMCID: PMCPMC2854765.
- Lind L, Penell J, Luttropp K, et al. Global DNA hypermethylation is associated with high serum levels of persistent organic pollutants in an elderly population. Environ Int. 2013 Sep;59:456–461. PubMed PMID: 23933504.
- Marsit CJ. Influence of environmental exposure on human epigenetic regulation. J Exp Biol. 2015 Jan 01;218(Pt 1):71–79. . PubMed PMID: 25568453; PubMed Central PMCID: PMCPMC4286705.
- Weng YI, Hsu PY, Liyanarachchi S, et al. Epigenetic influences of low-dose bisphenol A in primary human breast epithelial cells. Toxicol Appl Pharmacol. 2010 Oct 15;248(2):111–121. PubMed PMID: 20678512; PubMed Central PMCID: PMCPMC2946518.
- Kappil MA, Li Q, Li A, et al. In utero exposures to environmental organic pollutants disrupt epigenetic marks linked to fetoplacental development. Environ Epigenet. 2016 Mar;2(1). PubMed PMID: 27308065; PubMed Central PMCID: PMCPMC4905724. doi:10.1093/eep/dvv013.
- Liu D, Perkins JT, Petriello MC, et al. Exposure to coplanar PCBs induces endothelial cell inflammation through epigenetic regulation of NF-kappaB subunit p65. Toxicol Appl Pharmacol. 2015 Dec 15;289(3):457–465. PubMed PMID: 26519613; PubMed Central PMCID: PMCPMC4662647.
- Gentilini D, Garagnani P, Pisoni S, et al. Stochastic epigenetic mutations (DNA methylation) increase exponentially in human aging and correlate with X chromosome inactivation skewing in females. Aging (Albany NY). 2015 Aug;7(8):568–578. PubMed PMID: 26342808; PubMed Central PMCID: PMCPMC4586102.
- Gentilini D, Scala S, Gaudenzi G, et al. Epigenome-wide association study in hepatocellular carcinoma: identification of stochastic epigenetic mutations through an innovative statistical approach. Oncotarget. 2017 Jun 27;8(26):41890–41902. PubMed PMID: 28514750; PubMed Central PMCID: PMCPMC5522036.
- Sjodin A, Wong LY, Jones RS, et al. Serum concentrations of polybrominated diphenyl ethers (PBDEs) and polybrominated biphenyl (PBB) in the United States population: 2003–2004. Environ Sci Technol. 2008 Feb 15;42(4):1377–1384. PubMed PMID: 18351120.
- Blanck HM, Marcus M, Tolbert PE, et al. Age at menarche and tanner stage in girls exposed in utero and postnatally to polybrominated biphenyl. Epidemiology. 2000 Nov;11(6):641–647. PubMed PMID: 11055623.
- Small CM, DeCaro JJ, Terrell ML, et al. Maternal exposure to a brominated flame retardant and genitourinary conditions in male offspring. Environ Health Perspect. 2009 Jul;117(7):1175–1179. PubMed PMID: 19654930; PubMed Central PMCID: PMCPMC2717147.
- Jacobson MH, Darrow LA, Barr DB, et al. Serum Polybrominated Biphenyls (PBBs) and Polychlorinated Biphenyls (PCBs) and Thyroid Function among Michigan Adults Several Decades after the 1973–1974 PBB Contamination of Livestock Feed. Environ Health Perspect. 2017 Sep 26;125(9):097020. PubMed PMID: 28953452; PubMed Central PMCID: PMCPMC Journal - In Process. eng. .
- Bahn AK, Mills JL, Snyder PJ, et al. Hypothyroidism in workers exposed to polybrominated biphenyls. N Engl J Med. 1980 Jan 3;302(1):31–33. PubMed PMID: 6243165.
- Hoque A, Sigurdson AJ, Burau KD, et al. Cancer among a Michigan cohort exposed to polybrominated biphenyls in 1973. Epidemiology. 1998 Jul;9(4):373–378. PubMed PMID: 9647899.
- Curtis SW, Cobb DO, Kilaru V, et al. Exposure to polybrominated biphenyl (PBB) associates with genome-wide DNA methylation differences in peripheral blood. Epigenetics. 2019 Jan 24:1–15. PubMed PMID: 30676242.
- Ambatipudi S, Horvath S, Perrier F, et al. DNA methylome analysis identifies accelerated epigenetic ageing associated with postmenopausal breast cancer susceptibility. Eur J Cancer. 2017 Apr;75:299–307. PubMed PMID: 28259012; PubMed Central PMCID: PMCPMC5512160.
- Binder AM, Corvalan C, Mericq V, et al. Faster ticking rate of the epigenetic clock is associated with faster pubertal development in girls. Epigenetics. 2018;13(1):85–94. PubMed PMID: 29235933; PubMed Central PMCID: PMCPMC5836971.
- Demanelis K, Virani S, Colacino JA, et al. Cadmium exposure and age-associated DNA methylation changes in non-smoking women from northern Thailand. Environ Epigenet. 2017 May;3(2):dvx006. PubMed PMID: 29492308; PubMed Central PMCID: PMCPMC5804546.
- Durso DF, Bacalini MG, Sala C, et al. Acceleration of leukocytes’ epigenetic age as an early tumor and sex-specific marker of breast and colorectal cancer. Oncotarget. 2017 Apr 4;8(14):23237–23245. PubMed PMID: 28423572; PubMed Central PMCID: PMCPMC5410300.
- Hofstatter EW, Horvath S, Dalela D, et al. Increased epigenetic age in normal breast tissue from luminal breast cancer patients. Clin Epigenetics. 2018 Aug 29;10(1):112. PubMed PMID: 30157950; PubMed Central PMCID: PMCPMC6114717.
- Horvath S. DNA methylation age of human tissues and cell types. PubMed PMID: 24138928; PubMed Central PMCID: PMCPMC4015143 Genome Biol. 2013;1410:R115.
- Horvath S, Erhart W, Brosch M, et al. Obesity accelerates epigenetic aging of human liver. Proc Natl Acad Sci U S A. 2014 Oct 28;111(43):15538–15543. PubMed PMID: 25313081; PubMed Central PMCID: PMCPMC4217403.
- Horvath S, Langfelder P, Kwak S, et al. Huntington’s disease accelerates epigenetic aging of human brain and disrupts DNA methylation levels. Aging (Albany NY). 2016 Jul;8(7):1485–1512. PubMed PMID: 27479945; PubMed Central PMCID: PMCPMC4993344.
- Horvath S, Raj K. DNA methylation-based biomarkers and the epigenetic clock theory of ageing. Nat Rev Genet. 2018 Jun;19(6):371–384. PubMed PMID: 29643443.
- Irvin MR, Aslibekyan S, Do A, et al. Metabolic and inflammatory biomarkers are associated with epigenetic aging acceleration estimates in the GOLDN study. Clin Epigenetics. 2018;10:56. PubMed PMID: 29713391; PubMed Central PMCID: PMCPMC5907301.
- Kresovich JK, Xu Z, O’Brien KM, et al. Methylation-based biological age and breast cancer risk. J Natl Cancer Inst. 2019 Feb 22 PubMed PMID: 30794318. doi:10.1093/jnci/djz020.
- Levine ME, Hosgood HD, Chen B, et al. DNA methylation age of blood predicts future onset of lung cancer in the women’s health initiative. Aging (Albany NY). 2015 Sep;7(9):690–700. PubMed PMID: 26411804; PubMed Central PMCID: PMCPMC4600626.
- Levine ME, Lu AT, Bennett DA, et al. Epigenetic age of the pre-frontal cortex is associated with neuritic plaques, amyloid load, and Alzheimer’s disease related cognitive functioning. Aging (Albany NY). 2015 Dec;7(12):1198–1211. PubMed PMID: 26684672; PubMed Central PMCID: PMCPMC4712342.
- Levine ME, Lu AT, Chen BH, et al. Menopause accelerates biological aging. Proc Natl Acad Sci U S A. 2016 Aug 16;113(33):9327–9332. PubMed PMID: 27457926; PubMed Central PMCID: PMCPMC4995944.
- Everson TM, Punshon T, Jackson BP, et al. Cadmium-associated differential methylation throughout the placental genome: epigenome-wide association study of two U.S. Birth Cohorts. Environ Health Perspect. 2018 Jan 22;126(1):017010. PubMed PMID: 29373860.
- Green BB, Karagas MR, Punshon T, et al. Epigenome-wide assessment of DNA methylation in the placenta and arsenic exposure in the New Hampshire birth cohort study (USA). Environ Health Perspect. 2016 Aug;124(8):1253–1260. PubMed PMID: 26771251; PubMed Central PMCID: PMCPMC4977055.
- Fei DL, Koestler DC, Li Z, et al. Association between In Utero arsenic exposure, placental gene expression, and infant birth weight: a US birth cohort study. Environ Health. 2013 Jul 16;12:58. PubMed PMID: 23866971; PubMed Central PMCID: PMCPMC3733767.
- Cardenas A, Koestler DC, Houseman EA, et al. Differential DNA methylation in umbilical cord blood of infants exposed to mercury and arsenic in utero. Epigenetics. 2015;10(6):508–515. PubMed PMID: 25923418; PubMed Central PMCID: PMCPMC4622995.
- Small CM, Murray D, Terrell ML, et al. Reproductive outcomes among women exposed to a brominated flame retardant in utero. Arch Environ Occup Health. 2011;66(4):201–208. PubMed PMID: 22014192; PubMed Central PMCID: PMCPMC3964180.
- McCormack KM, Melrose P, Rickert DE, et al. Concomitant dietary exposure to polychlorinated biphenyls and polybrominated biphenyls: tissue distribution and arylhydrocarbon hydroxylase activity in lactating rats. Toxicol Appl Pharmacol. 1979 Jan;47(1):95–104. PubMed PMID: 218325.
- Dent JG, McCormack KM, Rickert DE, et al. Mixed function oxidase activities in lactating rats and their offspring following dietary exposure to polybrominated biphenyls. Toxicol Appl Pharmacol. 1978 Dec;46(3):727–735. PubMed PMID: 218324.
- Dent JG. Characteristics of cytochrome P-450 and mixed function oxidase enzymes following treatment with PBBs. PubMed PMID: 209991; PubMed Central PMCID: PMCPMC1637476. Environ Health Perspect. 1978 Apr;23:301–307
- McCormack KM, Lepper LF, Wilson DM, et al. Biochemical and physiological sequelae to perinatal exposure to polybrominated biphenyls: a multigeneration study in rats. Toxicol Appl Pharmacol. 1981 Jun 30;59(2):300–313. PubMed PMID: 6266078.
- Arneric SP, McCormack KM, Braselton WE Jr., et al. Altered metabolism of progesterone by hepatic microsomes from rats following dietary exposure to polybrominated biphenyls. Toxicol Appl Pharmacol. 1980 Jun 30;54(2):187–196. PubMed PMID: 6252660.
- Newton JF, Braselton WE Jr., Lepper LF, et al. Effects of polybrominated biphenyls on metabolism of testosterone by rat hepatic microsomes. Toxicol Appl Pharmacol. 1982 Mar 30;63(1):142–149. PubMed PMID: 6280342.
- Small CM, Cheslack-Postava K, Terrell M, et al. Risk of spontaneous abortion among women exposed to polybrominated biphenyls. Environ Res. 2007 Oct;105(2):247–255. PubMed PMID: 17239850; PubMed Central PMCID: PMCPMC2237897.
- Terrell ML, Rosenblatt KA, Wirth J, et al. Breast cancer among women in Michigan following exposure to brominated flame retardants. Occup Environ Med. 2016 Aug;73(8):564–567. PubMed PMID: 27312402.
- Georgiadis P, Gavriil M, Rantakokko P, et al. DNA methylation profiling implicates exposure to PCBs in the pathogenesis of B-cell chronic lymphocytic leukemia. Environ Int. 2019 May;126:24–36. PubMed PMID: 30776747.
- Lind L, Ingelsson E, Sundstrom J, et al. Methylation-based estimated biological age and cardiovascular disease. Eur J Clin Invest. 2018 Feb;48(2). PubMed PMID: 29231988. doi:10.1111/eci.12872.
- Marioni RE, Shah S, McRae AF, et al. DNA methylation age of blood predicts all-cause mortality in later life. Genome Biol. 2015 Jan 30;16:25. PubMed PMID: 25633388; PubMed Central PMCID: PMCPMC4350614.
- Safe S. Polychlorinated biphenyls (PCBs) and polybrominated biphenyls (PBBs): biochemistry, toxicology, and mechanism of action. PubMed PMID: 6091997; eng Crit Rev Toxicol. 1984;134:319–395.
- Fries GF. The PBB episode in Michigan: an overall appraisal. PubMed PMID: 3002722 Crit Rev Toxicol. 1985;162:105–156.
- Kay K. Polybrominated biphenyls (PBB) environmental contamination in Michigan, 1973–1976. Environ Res. 1977 Feb;13(1):74–93. PubMed PMID: 191251.
- Marder ME, Panuwet P, Hunter RE, et al. Quantification of polybrominated and polychlorinated biphenyls in human matrices by isotope-dilution gas chromatography-tandem mass spectrometry. J Anal Toxicol. 2016 Sep;40(7):511–518. PubMed PMID: 27445313; PubMed Central PMCID: PMCPMC4986627.
- Helsel DR. Less than obvious – statistical treatment of data below the detection limit. Environ Sci Technol. 1990 Dec 01;24(12):1766–1774. .
- Phillips DL, Pirkle JL, Burse VW, et al. Chlorinated hydrocarbon levels in human serum: effects of fasting and feeding. Arch Environ Contam Toxicol. 1989 Jul–Aug;18(4):495–500. PubMed PMID: 2505694.
- Bernert JT, Turner WE, Patterson DG Jr., et al. Calculation of serum “total lipid” concentrations for the adjustment of persistent organohalogen toxicant measurements in human samples. Chemosphere. 2007 Jun;68(5):824–831. . PubMed PMID: 17408721.
- Pidsley R, Zotenko E, Peters TJ, et al. Critical evaluation of the illumina methylation EPIC BeadChip microarray for whole-genome DNA methylation profiling [journal article]. Genome Biol. 2016 October 07;17(1):208. .
- Heiss JA, Just AC. Improved filtering of DNA methylation microarray data by detection p values and its impact on downstream analyses. Clin Epigenetics. 2019 Jan 24;11(1):15. PubMed PMID: 30678737; PubMed Central PMCID: PMCPMC6346546.
- Pidsley R, CC YW, Volta M, et al. A data-driven approach to preprocessing Illumina 450K methylation array data. BMC Genomics. 2013 May 1;14:293. PubMed PMID: 23631413; PubMed Central PMCID: PMCPMC3769145.
- Genomes Project C, Auton A, Brooks LD, et al. A global reference for human genetic variation. Nature 2015 Oct 1;526(7571):68–74. . PubMed PMID: 26432245; PubMed Central PMCID: PMCPMC4750478.
- McCartney DL, Walker RM, Morris SW, et al. Identification of polymorphic and off-target probe binding sites on the illumina infinium methylationEPIC BeadChip. Genom Data. 2016 Sep;9:22–24. PubMed PMID: 27330998; PubMed Central PMCID: PMCPMC4909830.
- Andrews SV, Ladd-Acosta C, Feinberg AP, et al. “Gap hunting” to characterize clustered probe signals in illumina methylation array data. Epigenetics Chromatin. 2016;9:56. PubMed PMID: 27980682; PubMed Central PMCID: PMCPMC5142147.
- Sun Z, Chai HS, Wu Y, et al. Batch effect correction for genome-wide methylation data with illumina infinium platform. BMC Med Genomics. 2011 Dec 16;4:84. PubMed PMID: 22171553; PubMed Central PMCID: PMCPMC3265417.
- Fortin JP, Triche TJ Jr., Hansen KD. Preprocessing, normalization and integration of the illumina humanMethylationEPIC array with minfi. Bioinformatics. 2017 Feb 15;33(4):558–560. . PubMed PMID: 28035024; PubMed Central PMCID: PMCPMC5408810.
- Houseman EA, Accomando WP, Koestler DC, et al. DNA methylation arrays as surrogate measures of cell mixture distribution. BMC Bioinformatics. 2012 May 08;13:86. PubMed PMID: 22568884; PubMed Central PMCID: PMCPMC3532182.
- Gentilini D, Somigliana E, Pagliardini L, et al. Multifactorial analysis of the stochastic epigenetic variability in cord blood confirmed an impact of common behavioral and environmental factors but not of in vitro conception. Clin Epigenetics. 2018;10:77. PubMed PMID: 29930742; PubMed Central PMCID: PMCPMC5994106.
- Tobi EW, Slieker RC, Luijk R, et al. DNA methylation as a mediator of the association between prenatal adversity and risk factors for metabolic disease in adulthood. Sci Adv. 2018 Jan;4(1):eaao4364. PubMed PMID: 29399631; PubMed Central PMCID: PMCPMC5792223.
- Hannum G, Guinney J, Zhao L, et al. Genome-wide methylation profiles reveal quantitative views of human aging rates. Mol Cell. 2013 Jan 24;49(2):359–367. PubMed PMID: 23177740; PubMed Central PMCID: PMCPMC3780611.
- Schisterman EF, Whitcomb BW, Louis GM, et al. Lipid adjustment in the analysis of environmental contaminants and human health risks. Environ Health Perspect. 2005 Jul;113(7):853–857. PubMed PMID: 16002372; PubMed Central PMCID: PMCPMC1257645.
- Phipson B, Maksimovic J, Oshlack A. missMethyl: an R package for analyzing data from Illumina’s HumanMethylation450 platform. Bioinformatics. 2016 Jan 15;32(2):286–288. . PubMed PMID: 26424855.