ABSTRACT
Various pathogens use differing strategies to evade host immune response including modulating the host’s epigenome. Here, we investigate if EVs secreted from P. aeruginosa alter DNA methylation in human lung macrophages, thereby potentially contributing to a dysfunctional innate immune response. Using a genome-wide DNA methylation approach, we demonstrate that P. aeruginosa EVs alter certain host cell DNA methylation patterns. We identified 1,185 differentially methylated CpGs (FDR < 0.05), which were significantly enriched for distal DNA regulatory elements including enhancer regions and DNase hypersensitive sites. Notably, all but one of the 1,185 differentially methylated CpGs were hypomethylated in association with EV exposure. Significantly hypomethylated CpGs tracked to genes including AXL, CFB and CCL23. Gene expression analysis identified 310 genes exhibiting significantly altered expression 48 hours post P. aeruginosa EV treatment, with 75 different genes upregulated and 235 genes downregulated. Some CpGs associated with cytokines such as CSF3 displayed strong negative correlations between DNA methylation and gene expression. Our infection model illustrates how secreted products (EVs) from bacteria can alter DNA methylation of the host epigenome. Changes in DNA methylation in distal DNA regulatory regions in turn can modulate cellular gene expression and potential downstream cellular processes.
Background
Extracellular vesicles (EVs) are circular lipid bilayer particles, 20–1000 nm in diameter, secreted by nearly all known eukaryotic and prokaryotic cell types [Citation1]. EVs include eukaryotic exosomes (20–150 nm) and microvesicles (100–1000 nm), which contain a variety of macromolecules including RNA, DNA and proteins, as well as outer membrane vesicles (OMVs) (20–500 nm) that contain virulence and other factors [Citation1]. EVs transport these virulence factors to facilitate infection and can trigger inflammatory responses in host cells [Citation2].
Multiple studies have characterized the contents of P. aeruginosa extracellular vesicles. Some examples of proteins include OprF, OprG, IcmP and LasA which have roles in antibiotic resistance, proteolysis and bacteria-host interactions [Citation3]. P. aeruginosa EVs also carry immune modulatory components like liposaccharide (LPS) that may affect cells that express pattern recognition receptors [Citation1,Citation4]. After being released from the bacteria, EVs from other Gram-negative bacteria can exist in natural environments like water drains, soil, and house dust [Citation1]. Furthermore, Gram-negative EVs are pathogenic on their own outside of the live bacteria [Citation5,Citation6]. To better understand the mechanism behind pathogenic effects of bacteria in the lung, it is important to study the effects of isolated EVs on host immune cells. Such studies will contribute to understanding the bacterial effects of diseases such as cystic fibrosis (CF), in which pathogenic species like P. aeruginosa deliver virulence factors by secreting EVs into the lung and accelerates decline the pulmonary function in patients [Citation7–11].
Although some work has been done to understand the impact of bacterial EVs in gene expression of host cells, not as much has been done to discern epigenetic responses to bacterial EVs. Epigenetics is the study of heritable changes in gene function caused by mechanisms other than changes in the underlying DNA sequence [Citation12]. One of the most widely studied epigenetic modifications is DNA methylation of cytosine at CpG dinucleotides [Citation13]. CpG islands, regions of dense CpG contents, are predominantly located in promoter regions of genes [Citation14,Citation15]. Methylation of CpG regions regulates gene expression by recruiting proteins involved in gene repression or by inhibiting the binding of transcription factor(s) to DNA [Citation16]. DNA methylation controls methylation of histone H3K4 at regulatory regions, where hypomethylated regulatory regions such as promoters and putative enhancers have enriched with H3K4me3 marks thereby increasing active transcription [Citation17]. Additionally, DNA methylation at enhancer regions can present bivalent chromatin states as it can co-exist with active H3K27ac [Citation18]. When DNA methylation marks decrease, the H3K27ac marks are also reduced, decreasing active transcription [Citation18]. The role of DNA methylation and its loss at DNase hypersensitive sites (DHS) is less studied. However, it is evident that DHS regions are depleted in areas of dense CpGs [Citation19]. Historically, alterations in DNA methylation have been attributed to chronic or longer-term environmental exposures, however, recent work has begun to investigate whether shorter-term exposures also result in DNA methylation changes.
Recent studies have investigated how pathogens can modulate host epigenome, specifically the DNA methylation landscape of cells of the innate immune system. Sinclair et al. have shown genome-wide DNA methylation changes in Anaplasma phagocytophilum-infected human neutrophils [Citation20]. Cizmeci et al. demonstrated altered DNA methylation associated with Burkholderia pseudomallei infection in macrophages [Citation21]. Pacis et al. in an infection model of Mycobacterium tuberculosis in human dendritic cells [Citation22], provide evidence of demethylation of distal DNA regulatory elements in genes, some of which regulate the activation of key immune transcription factors. However, epigenetic effects of EVs from P. aeruginosa, a common type of bacteria that causes infections, has not yet been studied to our knowledge. Therefore, the goal of this study is to assess whether EVs isolated from P. aeruginosa alter DNA methylation in human lung macrophages thereby potentially modulating the ability of macrophages to mount a proper immune response to the infection.
Results
Subject characteristics and EV characterization
Bronchoalveolar lavage (BAL) fluid was obtained from the right upper lobe of healthy subjects (n = 8) and a pure population of lung macrophages was isolated as previously described [Citation23,Citation24]. A cohort of five females and three males was used for DNA methylation analysis, whereas four females and three males were used in the gene expression analysis, due to lack of RNA availability from one of the original female subjects. Mean age of the study population was 27.1 years old. Subject characteristics are shown in .
Table 1.. Subject characteristics
As the mucoid phenotype of the P. aeruginosa is the strain associated with increased morbidity and mortality, EVs from clinically-driven mucoid strains were used for exposure [Citation25]. Isolation of EVs was done via affinity resin as described in the Supplemental Methods. Negative stain transmission electron microscopy was used for visualization of EVs (Supplementary Figure 1a). Additionally, nanoparticle tracking analysis was used for particle counting and validation of particle size. EV particle size ranged from 20 to 600 nm. A representative trace confirming particle size from the NanoSight NS300 instrument is shown in Supplemental Figure 1b. To characterize the contents of P. aeruginosa EVs, we performed mass spectrometry on isolated EVs. They were found to contain virulence factors such as LasB and CbpD and protease associated proteins such as PrpL and LasA. Full list of proteins identified from mass spectrometry from P. aeruginosa EVs can be found in Supplementary Table 1.
Differential methylation with P. aeruginosa EV treatment
Unsupervised hierarchical clustering of top 10,000 most variable CpGs resulted in clustering by subject rather than by treatment (Supplementary Figure 2). Clustering by subjects is not unexpected to observe genetic effects even after dropping known SNP-associated array probes as treatment effects of P. aeruginosa EVs may not affect majority of the CpGs analysed.
To observe the effects of P. aeruginosa EV treatment on DNA methylation of lung macrophages, we compared methylation status of DNA from matched control lung macrophages and P. aeruginosa EV treated macrophages. We identified 1,185 CpGs with differential methylation in association with EV treatment (FDR < 0.05, )). Some of the most differentially methylated CpGs include those associated with genes such as BCAT1, CNIH3, and YPEL2. Remarkably, all but one of the significantly differentially methylated CpGs were hypomethylated in treated lung macrophages compared to the controls. The only hypermethylated CpG was associated with gene EVL. Full list of differentially methylated CpGs and their annotations are provided in Supplementary Table 2.
Figure 1. P. aeruginosa EVs alter DNA methylation in human lung macrophages
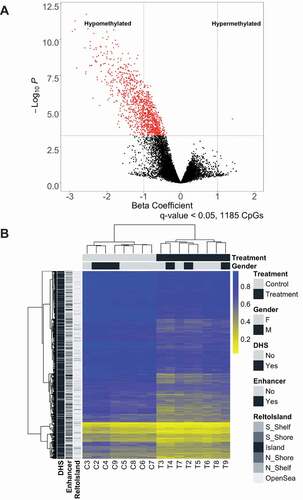
As expected, supervised hierarchical clustering of the 1,185 CpG with differential methylation indicated a clear distinction between control and P. aeruginosa EV treated lung macrophages ()). To better understand where the differentially methylated CpGs were located, we conducted Mantel-Haenszel odds ratio enrichment tests for genomic contexts of the 1,185 CpGs. Interestingly, differentially methylated CpGs were significantly enriched in DNase hypersensitive sites (DHS) (OR = 8.91, 95% CI = 7.04–11.41) and Fantom5 enhancer regions (OR = 7.91, 95% CI = 6.95–8.98) ()). In addition, differentially methylated CpGs were significantly depleted for gene promoter regions (OR = 0.29, 95% CI = 0.24–0.36). Based on the observed depletion of CpGs at promoter regions, the differentially methylated CpGs were expectedly significantly enriched in the open sea region (OR = 5.61, 95% CI = 4.61–6.88) in relation to known CpG island locations ()). Similar to the CpG probes used in analysis, the differentially methylated CpGs were most enriched in regions within 200 base pairs (bps) upstream of the transcription start site (TSS) (Supplementary Figure 3). However, the CpGs were enriched in regions 1–2 kbps downstream from the TSS compared to the analysed CpGs, which corresponds to the enrichment of differentially methylated CpGs at gene body sites (OR = 1.34, 95% CI = 1.19–1.51) and introns (OR = 1.94, CI = 1.72–2.18).
Figure 2. Differentially methylated CpGs are enriched in enhancers and DNase hypersensitive sites. Enrichment of genomic context (a) and relation to CpG island (b) of differentially methylated CpGs compared to CpGs used in analysis as determined by odds ratio and their 95% confidence intervals from the Mantel-Haenszel test plotted on a log2 scale, adjusted for Illumina probe types. Odds ratio of enrichment at CpG Islands is not represented as none of the differentially methylated were located in CpG islands
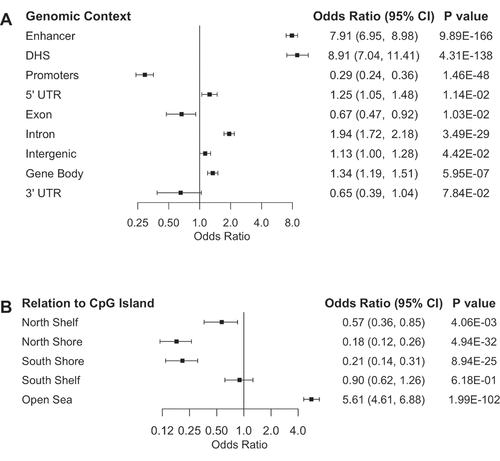
Differential gene expression with P. aeruginosa EV treatment
To further understand effects of P. aeruginosa EV treatment on the lung macrophages, we measured gene expression with the NanoString nCounter human v3 Cancer/Immune RNA expression assay. To expand on studies that have measured targeted gene expression of inflammatory response to P. aeruginosa EVs, our studies were designed to broaden the scope of the genes measured [Citation1]. Of 541 genes used in differential gene expression analysis, a majority (310) of genes were significantly differentially expressed in association with P. aeruginosa EV treatment ()). There were 3-fold more downregulated genes (235 genes) in the EV-treated lung macrophages compared to upregulated genes (75 genes). Hierarchical clustering of all 541 genes used in downstream analysis indicated a distinction between control lung macrophages and lung macrophages treated with EVs ()).
Figure 3. P. aeruginosa EVs alter gene expression in human lung macrophages
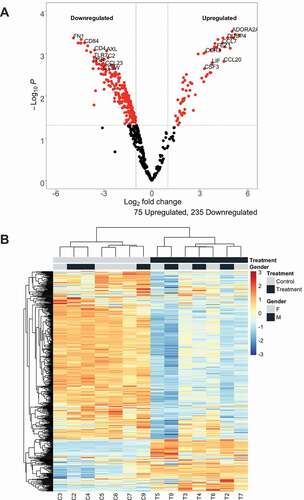
Figure 4. Hypomethylated CpGs related to CSF3 is negatively associated with increased CSF3 expression. Correlation between methylation beta values of CSF3-associated CpGs and gene expression (a–d). Correlations calculated based on Spearman’s rank correlation method. Pseudomonas aeruginosa extracellular vesicle treated lung macrophages coloured blue. Control lung macrophages coloured red. Linear regression line and 95% confidence interval indicated by the blue line and grey bands, respectively. (e) Representation of location of hypomethylated CpGs as well as other unchanged CSF3-associated CpGs in relation to CSF3 gene. Exons numbered in white. 845bps indicates distance from the furthest differentially methylated CpG to the transcription start site
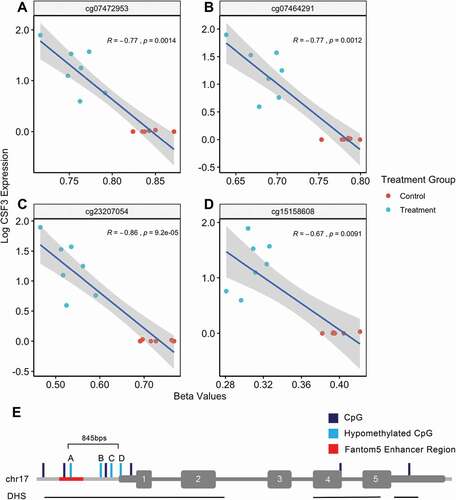
Figure 5. Hypomethylation of AXL, CD46 and MME is positively associated with gene expression. CpGs with positive correlation between methylation beta values and respective gene expression levels (a–d). Pseudomonas aeruginosa extracellular vesicle treated lung macrophages coloured blue. Control lung macrophages coloured red. Linear regression line and 95% confidence interval indicated by the blue line and grey bands, respectively
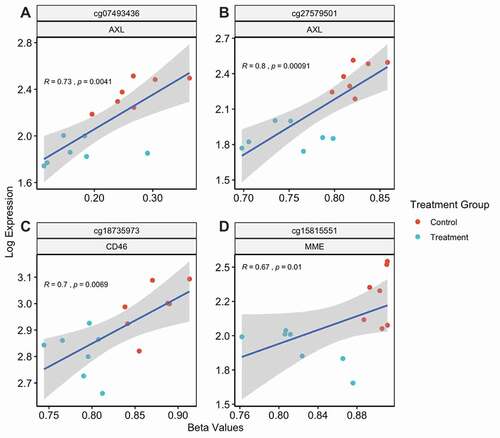
Top downregulated genes included genes that encode cell membrane proteins such as FN1, TREM2, CD22, and CR1. The most highly upregulated genes included genes that encode for cytokines and chemokines such as PPBP, IL19, IL6, and CXCL6. We conducted gene ontology analysis for both downregulated and upregulated genes to specifically assess what biological processes were associated with directional changes in gene expression. Strikingly, the downregulated genes were associated with biological processes related to regulation of viral processes and MHC class II presentation (Supplementary Figure 4a). Upregulated genes were found to be associated with biological processes regulating white blood cell migration or chemotaxis (Supplementary ). A full list of differentially expressed genes (FDR < 0.05) can be found in Supplementary Table 3.
To gain a deeper understanding of the biological function of these differentially methylated CpGs, we assessed gene ontologies of nearby genes of these CpG sites. Because not all CpGs were annotated to a specific gene, we used the Genomic Regions Enrichment Annotations Tool (GREAT) to predict the functions of the non-coding regions using their nearby genes [Citation26]. Given the relative purity of cell type in our samples, significantly hypomethylated CpGs were expectedly associated with genes involved in active immune response biological processes such as wound healing, positive regulation of angiogenesis and haemopoiesis (Supplementary ). Wound healing involved in inflammatory response biological process had the highest fold enrichment relative to other biological processes. Further GREAT analysis indicated that these hypomethylated CpGs were associated with cellular components such as PI3K complex and focal adhesion as well as molecular functions such as MAP kinase activity and histone acetyltransferase binding (Supplementary Figure 6A, 6B). Interestingly, gene ontology analysis of 735 genes annotated to differentially methylated CpGs revealed that the CpGs were enriched in positive regulation of phosphorylation and signal transduction processes (Supplementary Figure 7).
Correlation between changes in methylation and changes in gene expression
We next sought to assess the potential relationship between CpG hypomethylation and downstream gene expression in lung macrophages treated with P. aeruginosa EVs. The 1,185 differentially methylated CpGs tracked to 565 genes. Of these 565 genes, 38 unique genes had matching NanoString gene expression data. As some CpGs tracked to the same genes on the NanoString gene expression panel, we were able to assess correlations between 57 CpGs and their respective gene expression levels. 31 of the 57 CpGs showed significant negative correlation and four CpGs positive correlation between methylation and gene expression, respectively, while 22 did not have significant correlation at the 0.05 significance level. Complete results for the correlation of gene expression versus DNA methylation is available in Supplementary Table 4.
One particular gene that is relevant to macrophages, CSF3, had four associated CpGs that were hypomethylated. CSF3 encodes a cytokine that is essential in controlling differentiation and function of granulocytes [Citation27]. Spearman rank correlation indicated that hypomethylation was significantly associated with increased expression of CSF3 for all four CpGs which are located upstream of the transcription start site (all P < 0.01, ). The CpG site (cg07472953) farthest upstream of the transcription start site was still within 1.5kb, and also tracked to a Fantom5 enhancer region (). The other three hypomethylated CpGs were the only CpGs measured on the array platform within 200 bps of the transcription start site. All four hypomethylated CpGs were located within the same DHS and were not in a CpG-dense CpG island. Full annotation of all CSF3 associated CpGs are included in Supplementary Table 5.
Among the remaining CpGs tested, we observed four CpGs with positive correlations between methylation and gene expression at three different genes. Decreased methylation of CpGs related to P. aeruginosa EV treatment was significantly associated with decreased gene expression levels of AXL, MME, and CD46 (). All four CpGs tracked to the gene body regions of these genes, and the observed association of gene body hypermethylation with increased gene expression is consistent with prior literature [Citation28–30]. Three CpGs except cg15815551 () were located in either shelf or shore region in relation to CpG island. Full annotation of these CpGs are highlighted in light green in Supplementary Table 2.
Discussion
The goal of this study was to determine if EVs from P. aeruginosa could alter the host epigenome. Although prior work has examined epigenomic alterations related to bacterial infection [Citation20–22], our study addresses a gap in understanding the impact of P. aeruginosa EV exposure on host immune cell epigenetic gene regulation. Using human lung macrophages and P. aeruginosa extracellular vesicles as an in vitro model, we demonstrate that macrophages exposed to P. aeruginosa EVs exhibit decreased DNA methylation at known gene regulatory elements and that these changes are associated with altered gene expression.
We observed a broadly consistent pattern of DNA hypomethylation associated with P. aeruginosa EV exposure that tracked to cis-regulatory regions of biological processes important in active immune function such as wound healing and haematopoiesis. What was more interesting is that the ontology of only the genes annotated to CpGs revealed that genes with active phosphorylation processes were enriched. This suggests that the lung macrophages activate innate immune pathways in response to P. aeruginosa EVs. Additionally, the hypomethylated CpGs were located in distal regulatory elements such as enhancers and DNase hypersensitive sites and depleted in promoter regions. It is possible that hypomethylation of these distal regulatory elements may be changing the chromatin conformation to poise the lung macrophages to be prepared for active transcription, but not directly controlling active transcription. Few CpGs in HDAC9 and TET2, genes important in transcriptional regulation, were hypomethylated. It suggests EVs may potentially activate master epigenetic regulatory genes to induce a response in the lung macrophages. The significant correlations observed in a majority of the assessed relationships between methylation and gene expression indicate the possibility of epigenetic mechanisms contributing to modulation of immune reponses to P. aeruginosa EVs in lung macrophages.
Our investigation is consistent with the study of Pacis et al. demonstrating DNA methylation changes in response to bacterial infection (i.e. M. tuberculosis) in both human dendritic cells and monocyte-derived macrophages in several key observations [Citation31]. First, our results overwhelmingly demonstrate DNA hypomethylation in our EV infection model. Second, our study shows significant enrichment of differentially methylated CpGs in enhancer regions. And finally, differential methylation was noted to overlap in numerous genes in both studies, including: NFKB1, CREB5, and BCL2.
NFKB1, CREB5, and BCL2 have important functions in innate immunity in response to infections. In macrophages, the NF-κB family has essential pro-inflammatory functions in response to infections. NF-κB transcription factors induce large number of cytokines and chemokines such as IL-1β and IL-6 [Citation32]. We observed hypomethylation of CpG in the 5ʹ UTR region of NFKB1 and substantial increase in expression of IL1B and IL6. NF-κB also regulates BCL2 to promote cell survival. The CREB family is also involved in anti-apoptotic cell survival in macrophages through NF-κB-dependent pathway [Citation33]. Hypomethylation of these critical genes may contribute to survival of macrophages in response to pathogens like M. tuberculosis and P. aeruginosa. Further investigation is needed to validate the involvement of these genes in an active P. aeruginosa infection.
However, not all studies focusing on host DNA methylation changes in the setting of infection demonstrate consistent results. This suggests that methylation events may be pathogen-specific. While Cizmeci et al. observed DNA hypomethylation in a human macrophage cell line upon exposure to Burkholderia pseudomallei consistent with our results, the work of Marr et al. demonstrates DNA hypermethylation events associated with Leishmania donovani infection of THP-1 macrophages [Citation21,Citation34,Citation35–39]. Additional infection models and testing of alternate disease-related pathogens or pathogen-derived secreted products are required to better understand DNA alterations in host-pathogen interactions.
P. aeruginosa infection in cystic fibrosis patients is common and leads to poor patient outcomes. Understanding the molecular mechanisms underlying immune response to this opportunistic pathogen could reveal novel strategies to combat chronic infections. Our study reports epigenetic and transcriptional reprogramming of lung macrophages from exposure to P. aeruginosa extracellular vesicles. Future work examining epigenetic reprogramming of lung macrophages in CF patients with active P. aeruginosa infection as well as other CF pathogens, either singularly or co-colonized is needed to validate our lung infection model observations. With further studies in immune response to bacterial pathogens in CF, opportunities to develop better options for preventing and treating infections in CF and other immunocompromised patients may be gleaned.
Conclusions
In this study, we have demonstrated extensive epigenetic changes in DNA methylation, primarily hypomethylation in enhancer and DNase hypersensitive regions and gene body regions in host lung macrophages upon exposure to P. aeruginosa EVs. Consistent with changes in these distal DNA regulatory elements, we observed coinciding changes in gene expression. These results are consistent with an immune evasion strategy employed by a prokaryote in a host-pathogen interaction, potentially leading to an altered innate immune response.
Methods
This study was approved by the Dartmouth Hitchcock Institutional Review Board (#22,781). All subjects were healthy non-smokers. Eight volunteer subjects, all Caucasian, with a mean age of 27.1 (range 23–31, 3 males and 5 females) were included in the study and specimens from 7 subjects were amenable for use in the gene expression portion of this study (3 males and 4 females).
Bronchoalveolar lavage and macrophage isolation
Subjects underwent flexible bronchoscopy following local anaesthesia with lidocaine to the posterior pharynx and intravenous sedation. A bronchoscope was inserted trans-orally and advanced through the vocal cords. Bronchoalveolar lavage (BAL) fluid was obtained from tertiary airways via rinses with 20 ml of sterile saline followed by 10 ml of air and repeated for a total of 5 times per airway. Lung macrophages were isolated as previously described [Citation23,Citation40].
Experimental design
Right upper lobe (RUL) lung macrophages were used in this study, as RUL is the predominant lung lobe infected in CF patients and our previous work has shown that regional differences occur in lung macrophages [Citation24,Citation41]. Cells were plated in 6-well tissue culture dishes seeded at 1 × 106 cells per well. Cells were allowed to set down for 60 minutes. Lung macrophages were allowed to set down for 60 minutes. Cells were then incubated in the presence or absence (control) of P.aeruginosa EVs at a ratio of 100:1 (EVs:cell) per Cecil et al., for up to 48 hours. We have used the 48-h time point to simulate chronic infection [Citation42].
The P. aeruginosa clinical isolate strain used to generate EVs in this study is designated DH1137 and was a gift from Dr. Deborah Hogan, Department of Microbiology and Immunology, Geisel School of Medicine at Dartmouth. The isolate was originally described as strain NC-AMT0101-2 by Smith et al [Citation43]. Additionally, P. aeruginosa strain NC-AMT0101-2 has been characterized as LasR sufficient and mucoid phenotype by the work of Hoffman et al [Citation44]. For EV isolation and characterization details see Appendix 1: Supplemental methods.
DNA methylation array
Epigenome-wide DNA methylation profiling was performed via the Infinium Methylation EPIC Bead Chips (Illumina Inc., San Diego, CA) to measure methylation at more than 850,000 CpG sites as previously described [Citation45]. Briefly, DNA was extracted from bronchoalveolar lavage-derived cells via Qiagen (Germantown, MD) DNeasy Blood and Tissue Kit. DNA was quantitated on a Qubit 3.0 Fluorometer (Life Technologies, Carlsbad, CA). Bisulphite conversion of DNA was carried out with the Zymo EZ DNA methylation kit (Zymo Research, Irvine, CA) and EPIC array hybridization and scanning were performed at the University of Southern California Molecular Genomics Core.
DNA methylation array data processing
Raw intensity data files (IDATs) from the MethylationEPIC BeadChips were processed by the minfi R/Bioconductor analysis pipeline (version 1.30) annotation file version ilm10b4.hg19 [Citation46]. 78,379 cross reactive and SNP-associated probes annotated by Zhou, et al and 19,064 probes on sex chromosomes were excluded [Citation47]. The quality control was performed using ENmix R package [Citation48]. 9,432 probes those failing to meet a detection P-value of 0.00001 in ≥ 10% samples, were excluded. After all exclusions, 759,003 CpGs were remained for analysis. The filtered data was then normalized using preprocessFunnorm in minfi to remove any unwanted technical variation.
Annotations of CpGs such as genomic context or relation to CpG Island were provided in the Illumina EPIC B4 manifest file. The ‘enhancer’ context was defined by having a FANTOM5 enhancer record. ‘DNase hypersensitive site’ context was defined by having a record in the ‘DNase_Hypersensitive_NAME’ in the annotation. ‘Gene body’ transcriptional context was defined by having a ‘Body’ in the UCSC_RefGene_Group. Likewise, ‘3ʹ UTR’ and ‘5ʹ UTR’ were defined by having ‘UTR3’ and ‘UTR5’, respectively, in the UCSC_RefGene_Group. ‘Promoter’, ‘Intergenic’, ‘Intron’ and ‘Exon’ genomic contexts were defined by finding overlapping genomic regions of the CpGs and each context using the ilm10b4.hg19 annotation. Relation to CpG Island were defined by the ‘Relation_to_UCSC_CpG_Island’ in the Illumina EPIC annotation file. If no record of relation to the CpG island was indicated, the CpG was considered to be in the ‘Open Sea’ region.
Differential methylation analysis
Based on the prior analysis of distribution of the methylation beta value variance for the processed CpGs, we selected 115,556 CpGs with variance greater than 0.001 for downstream analyses. Differential methylation analysis of Illumina EPIC data was conducted with M-values (log2 ratio of beta value) using limma (v3.40.6) and q value (v2.16.0) R packages in R (v3.6.1) [Citation49,Citation50]. Differentially methylated CpGs were identified by fitting the M-values into a linear mixed-effects model adjusted for age, sex, repeat measures from single subject. The repeated measures were accounted for by calculating correlation coefficient by blocking by subject using the duplicateCorrelation function in limma. The correlation coefficient was found to be 0.857. The differentially methylated CpGs were deemed to be significant if the Q-value (p-values adjusted for false discovery rate) was under the threshold value of 0.05.
Differentially methylated CpGs were compared to the 115,556 CpGs for enrichment tests of genomic context using Mantel-Haenszel test, adjusting for Illumina EPIC array probe types. Functional significance of the CpGs were analysed with Genomic Regions Enrichment of Annotations Tool (GREAT) [Citation26]. Distance of differentially methylated CpGs to the nearest transcriptional start sites (TSS) were annotated with TxDb.Hsapiens.UCSC.hg19.knownGene annotation file using the ChIPpeakAnno (v3.18.2) [Citation51].
Gene expression quantification
RNA was isolated via RNeasy mini kit (Qiagen, Germantown, MD) as outlined in manufacturer’s instructions, and quantitated on a Qubit 3.0 Fluorometer (Life Technologies, Carlsbad, CA). The digital multiplexed NanoString nCounter human v3 Cancer/Immune RNA expression assay (NanoString Technologies, Seattle, WA) was performed according to manufacturer’s instructions with total RNA. Briefly, 200ng total RNA was assayed by overnight hybridization (65°C) with nCounter gene-specific reporter and capture probes. Following a 20-hour hybridization, reporter and capture probes are washed away using the automated nCounter sample prep station and probe/target complexes are aligned and immobilized in the nCounter Cartridge. Cartridges are then placed in the nCounter digital analyser for data collection. nSolver Analysis software (NanoString) (v3.0) was used for data analysis including background correction by subtracting the mean of the six negative controls included on the NanoString platform and normalization using the average geometric mean of the thirty housekeeping genes included in the assay.
Gene expression analysis
Differential gene expression analysis of Nanostring data was conducted using edgeR (v3.26.8) and limma (v3.40.6) packages in R (v3.6.1) [Citation49,Citation52]. The normalized data from Nanostring were scaled to counts per million (cpm). 541 genes with greater than 10cpm in at least 2 samples were used for downstream analysis. Likelihood ratio tests were conducted after fitting the gene expression data to a negative binomial generalized linear model. 310 genes were determined as differentially expressed based on its likelihood ratio test FDR value threshold of 0.05. Gene ontology analysis was conducted with Over Representation Analysis of biological processes gene ontology through WebGestalt (Web-based Gene SeT AnaLysis Toolkit) 2017 [Citation53].
Abbreviations:
EV: Extracellular vesicle; CF: Cystic fibrosis; NTA: Nanoparticle tracking analysis; BAL: Bronchoalveolar lavage
Authors’ contributions
DAA and AA designed the study. JAD, DLM, and DSA provided clinical samples. DAA and HFH performed the experimental work. MKL performed data analysis with input from BCC. MKL and DAA wrote the manuscript. All authors approved the final version of the manuscript.
Ethics approval and consent to participate
This study was approved by the Dartmouth Hitchcock Institutional Review Board (#22781).
Supplemental Material
Download Zip (379.4 KB)Acknowledgments
We thank the CF Translational Research Core at Dartmouth for the assistance of subject enrollment and clinical sample collection.
Data availability statement
DNA methylation array data has been deposited into Gene Expression Omnibus under accession number GSE142801. A token for access by reviewers is available by contacting the corresponding author. All other data used in this study is available upon request from the corresponding author.
Disclosure statement
The authors state that there are no conflicts of interest (financial nor personal) associated with this work.
Funding
This work is supported by the National Institutes of Health (NIH) R01HL122372 (AA) and National Institutes of Health (NIH) R01CA216265 (BCC). Subject enrollment and clinical sample collection were achieved with the assistance of the CF Translational Research Core at Dartmouth, which is jointly funded by the National Institutes of Health (P30DK117469 to Dean Madden) and Cystic Fibrosis Foundation Research Development Program (STANTO19R0 to Bruce Stanton).
Supplementary material
Supplemental data for this article can be accessed here.
Additional information
Funding
References
- Kim JH, Lee J, Park J, et al. Gram-negative and gram-positive bacterial extracellular vesicles. In: Seminars in cell and developmental biology. Vol. 40. Elsevier; Amsterdam, Netherlands: 2015. p. 97–104.
- Ellis TN, Kuehn MJ. Virulence and immunomodulatory roles of bacterial outer membrane vesicles. Microbiol Mol Biol Rev. 2010 Mar 1; 74(1):81–94.
- Choi D-S, Kim D-K, Choi SJ, et al. Proteomic analysis of outer membrane vesicles derived from Pseudomonas aeruginosa. Proteomics. 2011;11(16):3424–3429. Aug.
- Ellis TN, Leiman SA, Kuehn MJ. Naturally produced outer membrane vesicles from Pseudomonas aeruginosa elicit a potent innate immune response via combined sensing of both lipopolysaccharide and protein components. Infect Immun. 2010;78(9):3822–3831. Sep.
- Park K-S, Lee J, Jang SC, et al. Pulmonary inflammation induced by bacteria-free outer membrane vesicles from pseudomonas aeruginosa. Am J Respir Cell Mol Biol. 2013;49(4):637–645. Oct.
- Lee JC, Lee EJ, Lee JH, et al. Klebsiella pneumoniae secretes outer membrane vesicles that induce the innate immune response. FEMS Microbiol Lett. 2012;331(1):17–24. Jun.
- McGuigan L, Callaghan M. The evolving dynamics of the microbial community in the cystic fibrosis lung. Environ Microbiol. 2015;17(1):16–28. Jan.
- Malhotra S, Hayes D, Wozniak DJ. Cystic fibrosis and pseudomonas aeruginosa: the host-microbe interface. In: Clinical microbiology reviews. Vol. 32. American Society for Microbiology;. Washington DC: Jo-Anne H. Young. 2019. e00138-18.
- Bomberger JM, MacEachran DP, Coutermarsh BA, et al. Long-distance delivery of bacterial virulence factors by pseudomonas aeruginosa outer membrane vesicles. Ausubel FM, editor. PLoS Pathog. 2009 Apr 10;5(4):e1000382.
- Koeppen K, Hampton TH, Jarek M, et al. A novel mechanism of host-pathogen interaction through srna in bacterial outer membrane vesicles. Whiteley M, editor. PLOS Pathog. 2016 Jun 13;12(6):e1005672.
- Renelli M, Matias V, Lo RY, et al. DNA-containing membrane vesicles of pseudomonas aeruginosa PAO1 and their genetic transformation potential. Microbiology. 2004;150(7):2161–2169.
- Wu CT, Morris JR. Genes, genetics, and epigenetics: A correspondence. Science. 2001;293:1103–1105.
- Marsit CJ, Brummel SS, Kacanek D, et al. Infant peripheral blood repetitive element hypomethylation associated with antiretroviral therapy in utero. Epigenetics. 2015 Jan 1 10;(8)708–716.
- Nishiyama R, Ito M, Yamaguchi Y, et al. A chloroplast-resident DNA methyltransferase is responsible for hypermethylation of chloroplast genes in chlamydomonas maternal gametes. Proc Natl Acad Sci U S A. 2002 Apr 30; 99(9):5925–5930.
- Feng S, Cokus SJ, Zhang X, et al. Conservation and divergence of methylation patterning in plants and animals. Proc Natl Acad Sci U S A. 2010 May 11 107;(19)8689–8694.
- Moore LD, Le T, Fan G. DNA methylation and its basic function. Neuropsychopharmacol. 2013;38:23–38.
- Sharifi-Zarchi A, Gerovska D, Adachi K, et al. DNA methylation regulates discrimination of enhancers from promoters through a H3K4me1-H3K4me3 seesaw mechanism. BMC Genomics. 2017 Dec 12;18(1). DOI:https://doi.org/10.1186/s12864-017-4353-7
- Charlet J, Duymich CE, Lay FD, et al. Bivalent regions of cytosine methylation and H3K27 acetylation suggest an active role for DNA methylation at enhancers. Mol Cell. 2016 May 5 62;(3)422–431.
- He Y, Carrillo JA, Luo J, et al. Genome-wide mapping of DNase I hypersensitive sites and association analysis with gene expression in MSB1 cells. Front Genet. 2014 Oct 13;5. DOI:https://doi.org/10.3389/fgene.2014.00308
- Sinclair SHG, Yegnasubramanian S, Dumler JS. Global DNA methylation changes and differential gene expression in Anaplasma phagocytophilum-infected human neutrophils. Clin Epigenetics. 2015 Dec 29; 7(1):77.
- Cizmeci D, Dempster EL, Champion OL, et al. Mapping epigenetic changes to the host cell genome induced by burkholderia pseudomallei reveals pathogen-specific and pathogen-generic signatures of infection. Sci Rep. 2016 Aug 3;6: DOI:https://doi.org/10.1038/srep30861
- Pacis A, Tailleux L, Morin AM, et al. Bacterial infection remodels the DNA methylation landscape of human dendritic cells. Genome Res. 2015 Dec 1 25;(12)1801–1811.
- Bessich JL, Nymon AB, Moulton LA, et al. Low levels of insulin-like growth Factor-1 contribute to alveolar macrophage dysfunction in cystic fibrosis. J Immunol. 2013 Jul 1; 191(1):378–385.
- Armstrong DA, Chen Y, Dessaint JA, et al. DNA methylation changes in regional lung macrophages are associated with metabolic differences. ImmunoHorizons. 2019 Jul 1 3;(7)274–281.
- Pritt B, O’brien L, Winn W. Mucoid Pseudomonas in cystic fibrosis. Am J Clin Pathol [Internet]. 2007 [cited 2020 Feb 7];128:32–34. Available from: https://academic.oup.com/ajcp/article-abstract/128/1/32/1759840
- McLean CY, Bristor D, Hiller M, et al. GREAT improves functional interpretation of cis-regulatory regions. Nat Biotechnol. 2010;28(5):495–501. May.
- Brown GR, Hem V, Katz KS, et al. Gene: A gene-centered information resource at NCBI. Nucleic Acids Res. 2015 Jan 28 43;(D1)D36–42.
- Jones PA. Functions of DNA methylation: islands, start sites, gene bodies and beyond. Nat Rev Genet. 2012;13:484–492.
- Jjingo D, Conley AB, Yi SV, et al. On the presence and role of human gene-body DNA methylation. Oncotarget. 2012;3(4):462–474.
- Maunakea AK, Nagarajan RP, Bilenky M, et al. Conserved role of intragenic DNA methylation in regulating alternative promoters. Nature. 2010 Jul 8 466;(7303)253–257.
- Pacis A, Mailhot-Léonard F, Tailleux L, et al. Gene activation precedes DNA demethylation in response to infection in human dendritic cells. Proc Natl Acad Sci U S A. [2019 Apr 2];116(14):6938–6943.
- Liu T, Zhang L, Joo D, et al. NF-κB signaling in inflammation. Nat Publ Gr. 2017;2.
- Wen AY, Sakamoto KM, Miller LS. The role of the transcription factor CREB in immune function hhs public access. J Immunol. 2010;185(11):6413–6419.
- Marr AK, MacIsaac JL, Jiang R, et al. Leishmania donovani infection causes distinct epigenetic dna methylation changes in host macrophages. PLoS Pathog. 2014 Oct 1;10(10). https://doi.org/10.1371/journal.ppat.1004419
- Katakura F, Nishiya K, Wentzel AS, et al. Paralogs of common carp granulocyte colony-stimulating factor (G-CSF) have different functions regarding development, trafficking and activation of neutrophils. Front Immunol. 2019 Feb 19;10: DOI:https://doi.org/10.3389/fimmu.2019.00255
- Lemke G. How macrophages deal with death. Nat Rev Immunol. 2019 Sep 24; 19(9):539–549.
- Wong SS, Sun NN, Fastje CD, et al. Role of neprilysin in airway inflammation induced by diesel exhaust emissions. Res Rep Health Eff Inst. 2011 Jun;(159):3–40.
- Barilla-LaBarca ML, Liszewski MK, Lambris JD, et al. Role of membrane cofactor protein (CD46) in regulation of C4b and C3b deposited on cells. J Immunol. 2002 Jun 15; 168(12):6298–6304.
- Wang X, Zhang D, Sjölinder M, et al. CD46 accelerates macrophage-mediated host susceptibility to meningococcal sepsis in a murine model. Eur J Immunol. 2017 Jan;47(1):119–130.
- Chen Y, Armstrong DA, Salas LA, et al. Genome-wide DNA methylation profiling shows a distinct epigenetic signature associated with lung macrophages in cystic fibrosis. Clin Epigenetics. 2018 Dec 10 10;(1)152.
- Armstrong DA, Nymon AB, Ringelberg CS, et al. Pulmonary microRNA profiling: implications in upper lobe predominant lung disease. Clin Epigenetics. 2017 Dec 30 9;(1)56.
- Cecil JD, O’Brien-Simpson NM, Lenzo JC, et al.Outer membrane vesicles prime and activate macrophage inflammasomes and cytokine secretion in vitro and in vivo.Front Immunol.8: 2017 Aug 25
- Smith EE, Buckley DG, Wu Z, et al. Genetic adaptation by Pseudomonas aeruginosa to the airways of cystic fibrosis patients. Proc Natl Acad Sci U S A. 2006 May 30 103;(22)8487–8492.
- Hoffman LR, Richardson AR, Houston LS, et al. Nutrient availability as a mechanism for selection of antibiotic tolerant Pseudomonas aeruginosa within the CF airway. PLoS Pathog. 2010 Jan;6(1): DOI:https://doi.org/10.1371/journal.ppat.1000712
- Kling T, Wenger A, Beck S, et al. Validation of the methylationEPIC beadChip for fresh-frozen and formalin-fixed paraffin-embedded tumours. Clin Epigenetics. 2017 Apr 4;91. https://doi.org/10.1186/s13148-017-0333-7
- Aryee MJ, Jaffe AE, Corrada-Bravo H, et al. Minfi: A flexible and comprehensive bioconductor package for the analysis of infinium DNA methylation microarrays. Bioinformatics. [2014 May 15];30(10):1363–1369.
- Zhou W, Laird PW, Shen H. Comprehensive characterization, annotation and innovative use of Infinium DNA methylation BeadChip probes. Nucleic Acids Res. 2017 Feb 28;45(4):e22.
- Xu Z, Niu L, Li L, et al. ENmix: a novel background correction method for Illumina HumanMethylation450 BeadChip. Nucleic Acids Res. 2015;44(3):20.
- Ritchie ME, Phipson B, Wu D, et al. limma powers differential expression analyses for RNA-sequencing and microarray studies. Nucleic Acids Res. 2015 Apr 20 43;(7)e47.
- Storey JD, Bass AJ, Dabney A, et al. qvalue: Q-value estimation for false discovery rate control. [Internet]. R Package. 2019;2(18.0): Available from: http://github.com/jdstorey/qvalue
- Zhu LJ, Gazin C, Lawson ND, et al. ChIPpeakAnno: a Bioconductor package to annotate ChIP-seq and ChIP-chip data. BMC Bioinformatics. 2010 Dec 11 11;(1)237.
- Robinson MD, McCarthy DJ, Smyth GK. edgeR: A Bioconductor package for differential expression analysis of digital gene expression data. Bioinformatics. 2009 Jan 1; 26(1):139–140.
- Wang J, Vasaikar S, Shi Z, et al., A more comprehensive, powerful, flexible and interactive gene set enrichment analysis toolkit.Nucleic Acids Res. 2017 [2017 Jul 3];45(W1):W130–7.