ABSTRACT
Exposure to metals increases risk for pregnancy complications. Extracellular vesicle (EV) miRNA contribute to maternal-foetal communication and are dysregulated in pregnancy complications. However, metal impacts on maternal circulating EV miRNA during pregnancy are unknown. Our objective was to investigate the impact of multiple metal exposures on EV miRNA in maternal circulation during pregnancy in the MADRES Study. Associations between urinary concentrations of nine metals and 106 EV miRNA in maternal plasma during pregnancy were investigated using robust linear regression (N = 231). Primary analyses focused on metal-miRNA associations in early pregnancy (median: 12.3 weeks gestation). In secondary analyses, we investigated associations with late pregnancy miRNA counts (median: 31.8 weeks gestation) in a subset of participants (N = 184) with paired measures. MiRNA associated with three or more metals (PFDR<0.05) were further investigated using Bayesian Kernel Machine Regression (BKMR), an environmental mixture method. Thirty-five miRNA were associated (PFDR<0.05) with at least one metal in early pregnancy. One association (an inverse association between cobalt and miR–150-5p) remained statistically significant when evaluating late pregnancy miRNA counts. Eight miRNA (miR–302b-3p, miR–199a-5p, miR–188-5p, miR–138-5p, miR–212-3p, miR–608, miR–1272, miR–19b-3p) were associated with three metals (barium, mercury, and thallium) in early pregnancy, and their predicted target genes were enriched in pathways important for placental development. Results were consistent when using BKMR. Early pregnancy exposure to barium, mercury, and thallium may have short-term impacts on a common set of EV miRNA which target pathways important for placental development.
Introduction
Most individuals in the United States are exposed simultaneously to multiple metals[Citation1], with major sources including diet and drinking water, tobacco smoke, air pollution, and certain cosmetics [Citation2–9]. Toxic and essential metal exposures during pregnancy have been associated with a variety of pregnancy complications and adverse birth outcomes, including hypertensive disorders of pregnancy, gestational diabetes mellitus, foetal growth restriction, and preterm birth [Citation10–18]. Although the mechanisms underlying these effects are largely unknown, growing evidence suggests that epigenetic dysregulation, including alterations in microRNA (miRNA), may play an important role in metals toxicity [Citation19–29].
MiRNA are small non-coding RNA that posttranscriptionally regulate gene expression by directly degrading or inhibiting the translation of messenger RNA [Citation30]. Perturbations in miRNA can have widespread impacts on gene expression and disease development [Citation30], as most miRNA inhibit multiple messenger RNA targets and collectively regulate more than 60% of human protein coding genes [Citation30]. In addition to impacting gene expression in the tissues from which they are derived, miRNA can be packaged into extracellular vesicles (EVs) and released into circulation, facilitating intercellular communication [Citation31–33]. EV concentrations are higher in the circulation of pregnant compared with non-pregnant women and increase during gestation, with a large fraction originating in the placenta [Citation34–36]. Maternal circulating EV miRNA are therefore hypothesized to facilitate maternal-foetal communication during pregnancy, and dysregulation of EV miRNA has been associated with pregnancy complications and adverse birth outcomes [Citation37–44].
Metal-induced alterations in miRNA profiles have been reported both for maternal and foetal tissues, including the placenta, cord blood, and cervix [Citation26–29], and a growing number of in vitro studies have demonstrated that certain metals alter the release of EVs and their cargo [Citation45–53]. However, population-based studies evaluating metal impacts on EV miRNA are lacking. The current study investigated the impact of prenatal exposure to multiple toxic and essential metals on maternal circulating EV miRNA in the Maternal and Developmental Risks from Environmental and Social Stressors (MADRES) study, a pregnancy cohort of predominately lower income Hispanic women in Los Angeles, California.
Materials and methods
Study participants
MADRES is an ongoing prospective pregnancy cohort, which has been described in detail previously [Citation54]. Briefly, participants are recruited from four prenatal care providers in Los Angeles, California, which include two community health clinics, one county hospital prenatal clinic, and one private obstetrics and gynecology practice. Most of these clinics serve predominantly lower income Hispanic populations. Women are eligible to participate in MADRES if they are 18 years of age or older, can speak English or Spanish fluently, and their pregnancy is at less than 30 weeks’ gestation at the time of recruitment. Exclusion criteria include: 1) multiple gestation; 2) current incarceration; 3) HIV positive status; and 4) having a physical, mental, or cognitive disability that would prevent participation in the study or the ability to provide informed consent. Informed consent was obtained from all participants at study entry, and the study was approved by the University of Southern California’s Institutional Review Board. The current study focused on 231 mother-infant pairs who had urinary metals and plasma EV miRNA measured in early pregnancy (median gestational age (GA): 12.3 weeks) and complete covariate information.
Urinary metals
Maternal spot urine samples were collected from MADRES participants in 90-mL sterile specimen containers during their first (median GA: 12.3 weeks) and third (median GA: 31.8 weeks) study visits. Samples were transported on ice to the laboratory within 1 hour, where they were aliquoted and stored at −80°C in 2 mL sterile cryovials (VWR). Methods for urinary metals assessment in MADRES have been described in detail previously [Citation13,Citation14]. Briefly, a multi-metals panel was measured by inductively coupled plasma mass spectrometry at NSF International, which is affiliated with the University of Michigan Children’s Health Exposure Analysis Resource laboratory hub. The Centers for Disease Control and Prevention method 3018.3 was used, with modifications for the expanded metals panel and the Thermo Scientific iCAP™ RQ instrument (serial number RQ0029). The current study focused on nine metals that can be measured reliably in urine [Citation55–63], which were above the limits of detection (LOD) for ≥60% of participants: antimony (Sb), barium (Ba), cadmium (Cd), cobalt (Co), mercury (Hg), molybdenum (Mo), nickel (Ni), thallium (Tl), and tin (Sn). Values below the LOD were set to the LOD/√2. To account for urine dilution, urinary metal concentrations were adjusted for specific gravity measured by a refractometer (Itago), as described previously [Citation13,Citation14]. Given that placentation begins in early pregnancy and depends on maternal-foetal crosstalk [Citation64], we focused on early pregnancy urinary metal concentrations, hypothesizing that this would be a particularly vulnerable window of exposure.
Plasma EV miRNA
Methods for extracting and profiling EV miRNA have been described in detail previously [Citation43,Citation65]. Briefly, blood samples were collected from MADRES participants at their first (median GA: 12.3 weeks) and third (median GA: 31.8 weeks) study visits in EDTA vacutainers. Samples were transported on ice and processed within two hours. Plasma aliquots were stored at −80°C. The Qiagen ExoRNeasy kit was used to extract EV RNA from 500 µl of plasma according to the manufacturer’s instructions [Citation66], with a second addition of 90 µL chloroform and an additional round of phase separation. Purified RNA aliquots were frozen at −80°C. Extracted plasma EV miRNA were quantified by BioAnalyzer using a small RNA kit (Agilent Technologies, Inc. USA). Samples with a minimum concentration of 100 pg/µL and a visible peak between 10 and 24 nucleotides were used for miRNA profiling. Approximately 800 miRNA were profiled using the NanoString nCounter Human v3 miRNA expression assay (Nanostring Technologies, Inc.). A single lot of miRNA probes was used for all samples. Raw Nanostring RCC files were deposited in the NCBI Gene Expression Omnibus and are publicly available through GEO Series accession number GSE168788. Post-quantification quality control and normalization of the miRNA data was conducted using the NanoStringNorm R Package [Citation67]. Samples with positive normalization factors>6 were excluded from analyses. Given our hypothesis that early pregnancy is a particularly important window for maternal-foetal communication, primary analyses focused on early pregnancy EV miRNA measures. However, late pregnancy EV miRNA were also evaluated in secondary analyses for a subset of participants who had paired measures in the third trimester (N = 184).
Covariates
The following variables were hypothesized potential confounders or precision variables and were therefore considered for all statistical models: maternal age, pre-pregnancy BMI, maternal ethnicity, maternal place of birth, foetal sex, recruitment site, GA at sample collection, pregnancy order, nausea during pregnancy, prenatal vitamin use, maternal smoking status, and maternal second-hand smoke exposure during the pregnancy. Information on maternal pre-pregnancy weight, ethnicity, place of birth, pregnancy order, nausea, supplement use, smoking status, and second-hand smoke exposure were determined by questionnaires, administered during pregnancy in English or Spanish depending on the preferred language of the participant. Maternal standing height was measured twice using a stadiometer (Perspectives Enterprises Model PE-AIM-101). Pre-pregnancy BMI was calculated using the participant’s self-reported pre-pregnancy weight and measured height values. Information on foetal sex was abstracted from the maternal medical records. Information on maternal ethnicity and birthplace was used to create one variable with three categories: non-Hispanic, Hispanic born in the United States, and Hispanic born outside the United States. Information on maternal smoking status and second-hand smoke exposure during the pregnancy were combined to create a binary variable reflecting any or no tobacco smoke exposure during the pregnancy.
Statistical analyses
We hypothesized that metals would likely have short-term impacts on EV miRNA and that early pregnancy would be a particularly vulnerable window of exposure. Primary analyses therefore focused on early pregnancy measures of both urinary metals and EV miRNA. However, to investigate whether associations identified in early pregnancy persisted into late pregnancy, we ran secondary analyses using late pregnancy measures of these miRNA in a subset of participants with paired EV miRNA measures available in the third trimester (N = 184). Statistical analyses were conducted in R (version 4.0.3)[Citation68]. Sample-specific normalization of raw miRNA counts was performed using the NanoStringNorm R Package (version 1.2.1.1)[Citation67], as described previously [Citation43,Citation65]. Of the 800 miRNA measured by the NanoString nCounter platform, 106 were above the sample-specific background levels (mean + 1.5 SD of negative controls) for ≥60% of participants in early pregnancy and were therefore retained for statistical analyses.
Given that the major determinants of EV miRNA in maternal circulation during pregnancy are largely unknown, we first ran a principal component analysis (PCA) for early pregnancy measures of the 106 EV miRNA that met the detection threshold criteria for inclusion in statistical analyses, using the ‘stats’ package in R. The top 10 miRNA principal components (miR PCs) explained 79% of the variance in the data (Figure S1). Associations between hypothesized confounders and precision variables and these 10 miR PCs were evaluated using Spearman correlations for continuous variables and Kruskal-Wallis and Wilcoxon rank sum tests for categorical and binary variables, respectively. Continuous variables of interest included maternal age, pre-pregnancy BMI, nausea severity score in early pregnancy, and the GA at sample collection. Categorical and binary variables of interest included recruitment site, maternal education, ethnicity by birthplace, prenatal vitamin use in early pregnancy, pregnancy order, foetal sex, tobacco smoke exposure during pregnancy, and laboratory batch. Variables associated with any of the top 10 miR PCs with a P ≤ 0.05 were included in statistical models.
Urinary metal concentrations and miRNA counts were log-transformed to reduce the influence of extreme values. Associations between urinary metals and miRNA counts were evaluated using robust linear regression, with separate models run for each metal-miRNA pair. Final models were adjusted for maternal age, pre-pregnancy BMI, recruitment site, maternal ethnicity by birthplace, foetal sex, GA at sample collection, and laboratory batch. False discovery rate-adjusted p-values (PFDR) <0.05 were considered statistically significant. We also evaluated whether results remained statistically significant after a more conservative Bonferroni correction. Bonferroni-significant results were further investigated using generalized additive models (GAMs) to ensure that the linearity assumption for the robust linear regression models was met and to identify potential influential points using the ‘mgcv’ package in R [Citation69]. GAMs were plotted using the ‘visreg’ package in R [Citation70]. Two participants had extremely low concentrations of urinary Mo (<1 μg/L) which were highly influential and therefore excluded from models evaluating Mo as the exposure of interest. Volcano plots were created for each metal using the ‘EnhancedVolcano’ package in R[Citation71]. To visualize the number of unique versus common miRNA associated with each metal, an UpSet plot was created using the ‘UpsetR’ package in R [Citation72].
Many of the urinary metals were significantly correlated with each other (). For miRNA associated with three or more metals in robust linear regression models, we therefore conducted secondary analyses using Bayesian Kernel Machine Regression (BKMR), an environmental mixture modelling approach [Citation73]. This method can distinguish which mixture components are associated with an outcome, accounting for the correlations between them. We obtained 100,000 posterior samples of model parameters using a Markov chain Monte Carlo sampler and the default noninformative priors specified in the ‘bkmr’ R package [Citation74]. Model convergence was inspected using trace plots.
Figure 1. Spearman correlations between metal pairs (N = 231). Blue shades indicate positive correlations, while red shades indicate negative correlations. Stronger correlations are indicated by darker shades, and the correlation coefficients are overlaid on the plot. Blank boxes indicate correlations that were not statistically significant (P ≥ 0.05). Abbreviations: Ba, barium; Cd, cadmium; Co, cobalt; Hg, mercury; Mo, molybdenum; Ni, nickel; Sb, antimony; Sn, tin; Tl, thallium.
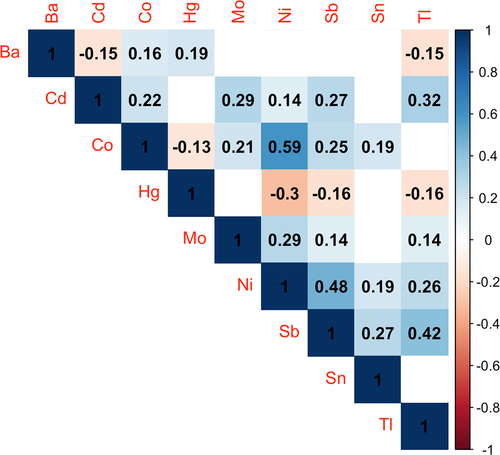
Predicted target genes of the miRNA associated with three or more metals were identified using mirDIP, which uses data from 30 different sources to generate an integrated miRNA-target interaction prediction score [Citation75]. These scores are assigned confidence classes based on the quality of data supporting the interactions [Citation75]. We restricted to genes with scores in the ‘very high confidence’ category and investigated whether these predicted targets were significantly enriched (PBonferroni<0.05) in biological pathways using pathDIP4, which integrates information from 24 different databases [Citation76]. We restricted to pathways that were statistically significant across at least two databases and investigated whether any of these pathways were commonly identified for multiple miRNA. MiRNet 2.0 was used to visualize miRNA-target gene interactions [Citation77].
Results
Participant characteristics
Characteristics of the study participants are shown in . The majority (85.3%) of participants were Hispanic. The median maternal age was 28.2, and the median pre-pregnancy BMI was 28.0 kg/m2. There were slightly more women carrying male (52.4%) compared with female (47.6%) foetuses. Participants included in the current study were generally similar to individuals who were excluded, although there was a higher percentage of Hispanic participants, as they were prioritized for EV miRNA measures, and participants included in the study had attained less education on average (Table S1). Urinary metal concentrations for this population have been described previously [Citation13,Citation14] and are shown for the current study subset in Table S2. Pairwise correlations between urinary metals are shown in . The majority of metals were positively correlated with each other.
Table 1. Participant characteristics (N = 231).
Metal-miRNA associations
Four of the nine metals investigated were significantly (PFDR<0.05) associated with one or more miRNA in early pregnancy (, ,Table S3). Ba was associated with 20 miRNA, Co with one miRNA, Hg with 23 miRNA, and Tl with 16 miRNA (, , Table S3). After a more conservative Bonferroni correction (PBonferroni<0.05), four of the 20 miRNA associated with Ba, eight of the 23 miRNA associated with Hg, three of the 16 miRNA associated with Tl, and the one miRNA associated with Co remained statistically significant (, Table S3). GAMs did not identify any influential points and confirmed that metal-miRNA associations were linear (Figure S2). Cd, Mo, Ni, Sb, and Sn were not significantly associated with any of the miRNA after multiple testing correction. Of the 35 unique miRNA associated with one or more metal in early pregnancy, 30 are expressed in the placenta, and a subset of 13 are very highly expressed (Table S3) [Citation78]. One of these highly expressed miRNA (miR–1323) is a member of the placenta-specific C19MC cluster [Citation78].
Figure 2. Volcano plots showing associations between early pregnancy urinary concentrations of barium (a), cobalt (b), mercury (c), and thallium (d) and the 106 EV miRNA detectable in ≥60% of early pregnancy maternal plasma samples. Results are from robust linear regression models, which were adjusted for maternal age, pre-pregnancy BMI, recruitment site, maternal ethnicity by birthplace, foetal sex, gestational age at sample collection, and laboratory batch. Points above the dashed line indicate miRNA with a PFDR<0.05. MiRNA that remained significantly associated with the specified metal after a more conservative Bonferroni correction (PBonferroni<0.05) are annotated. Associations for cadmium, molybdenum, nickel, antimony, and tin are not shown, as they did not remain statistically significant after multiple testing correction.
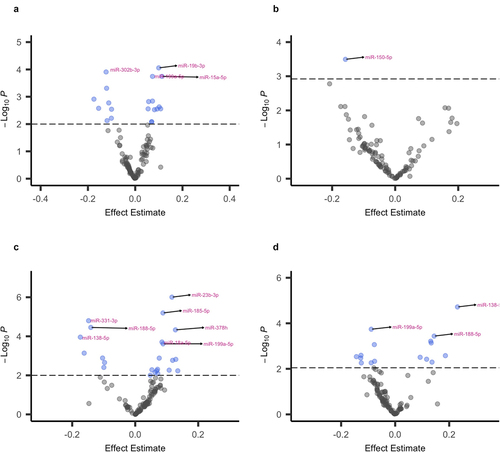
Figure 3. UpSet plot showing the number of unique and common miRNA associated with each metal after multiple testing correction (PFDR<0.05). The total number of significant (PFDR<0.05) hits (set size) for each metal is shown on the left side of the plot. The total number of unique and common hits are shown with barplots on the right side of the plot. Single dots indicate unique hits for the specified metal, while multiple linked dots indicate common hits for the specified metals.
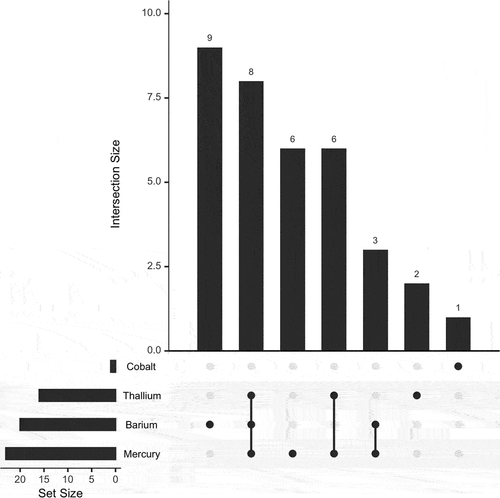
Eight miRNA (miR–302b-3p, miR–199a-5p, miR–188-5p, miR–138-5p, miR–212-3p, miR–608, miR–1272, miR–19b-3p) were significantly associated with three different metals (Ba, Hg, Tl) in early pregnancy (, ). Ba and Hg were inversely associated with miR–138-5p, miR–1272, miR–188-5p, miR–212-3p, miR–608, and miR–302b-3p and were positively associated with miR–19b-3p and miR–199a-5p (). For all eight miRNA, associations were in the opposite direction for Tl (). The patterns of associations between these metals and miRNA were consistent when Ba, Hg, and Tl were evaluated simultaneously in one model using BKMR (Figures S3 and S4). The eight miRNA were significantly correlated with each other (Figure S5). The six miRNA that were inversely associated with Ba and Hg but positively with Tl (miR–138-5p, miR–1272, miR–188-5p, miR–212-3p, miR–608, miR–302b-3p) were positively correlated with each other and had high loadings for miR PC 2 (Table S4, Figure S5). The two miRNA that were positively associated with Ba and Hg but inversely with Tl (miR–19b-3p, miR–199a-5p) were positively correlated with each other and had high loadings for miR PCs 1 and 3 (Table S4, Figure S5). These two sets of miRNA were inversely correlated with each other (Figure S5).
Table 2. Associations between barium, mercury, and thallium and the eight common miRNA that remained statistically significant after multiple testing correction.
To investigate whether associations between the 35 miRNA associated with metal exposures in early pregnancy persisted across gestation, we evaluated the same metal-miRNA associations using late pregnancy measures of these miRNA for a subset of participants (N = 184) with paired measures in the third trimester. For all 35 miRNA, early pregnancy measures were significantly correlated (P < 0.05) with late pregnancy measures, with Spearman correlations ranging from moderate (rho = 0.31 for miR–18a-5p) to high (rho = 0.71 for miR–138-5p) (Table S5). Associations between early pregnancy concentrations of metals and early pregnancy measures of these 35 miRNA were very similar in this smaller subset of participants with paired miRNA measures in early and late pregnancy (Table S6). However, only one association (the inverse association between Co and miR–150-5p) remained statistically significant after multiple testing correction, with a somewhat stronger effect estimate, when evaluating late pregnancy miRNA counts (β: −0.20, 95% CI: −0.31, −0.09 compared with β: −0.16, 95% CI: −0.24, −0.07 for early pregnancy miRNA counts) (Table S7).
Gene set enrichment analyses for target genes
PathDIP4 identified 17, seven, four, 14, 35, 23, and 29 Bonferroni-significant pathways, respectively, for seven of the eight miRNA associated with three or more metals in early pregnancy: miR–302b-3p, miR–199a-5p, miR–188-5p, miR–138-5p, miR–212-3p, miR–608, and miR–19b-3p (Table S8). Ten of the significant pathways identified were common to three or more miRNA, and the EGFR pathway was common to seven of the eight miRNA (). MiRNA-target gene interactions for these seven miRNA are shown in , highlighting genes involved in the EGFR pathway.
Table 3. Bonferroni-significant pathways identified for three or more of the miRNA commonly associated with barium, mercury, and thallium.
Figure 4. MiRNA-gene networks for the seven miRNA associated with barium, mercury, and thallium, which are predicted to target genes enriched in the EGFR pathway. MiRNA nodes are shown in light blue and predicted target genes are shown in pink. Genes involved in the EGFR pathway are highlighted in yellow and are annotated.
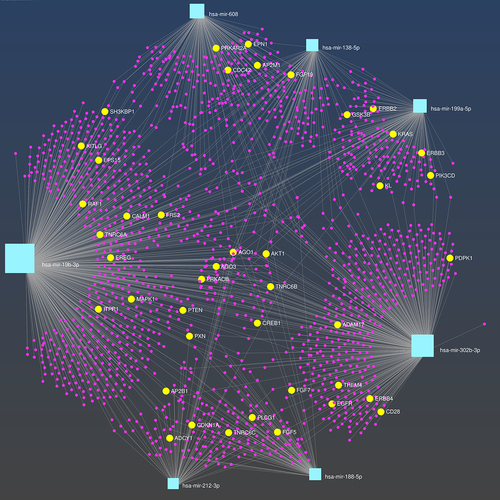
Discussion
During pregnancy, EVs and their miRNA cargo are bidirectionally transferred between the mother and foetus, facilitating maternal-foetal communication during this critical period [Citation79]. Dysregulation of EV miRNA has been implicated in a growing number of pregnancy complications and adverse birth outcomes. However, impacts of environmental contaminants on EV miRNA are largely unknown. In a cohort of predominately lower income Hispanic women in Los Angeles, we identified 35 unique EV miRNA that were associated with urinary metals in early pregnancy. Eight of these miRNA (miR–302b-3p, miR–199a-5p, miR–188-5p, miR–138-5p, miR–212-3p, miR–608, miR–1272, miR–19b-3p) were significantly associated with three different metals (Ba, Hg, and Tl). These associations were consistent in single metal analyses and when using an environmental mixture modeling method, which suggests that all three metals impact this common set of miRNA. With the exception of an inverse association identified between Co and miR–150-5p, metal-miRNA associations did not persist into late pregnancy.
A growing number of in vitro studies have demonstrated that metals impact the release of EVs from a variety of cell types and alter EV cargo [Citation45–53]. Prior epidemiological studies have also reported metal impacts on miRNA in diverse biofluids and tissue types, including the placenta [Citation26,Citation28,Citation29,Citation80–89]. However, to our knowledge, this is the first epidemiological study to investigate metal impacts on EV miRNA. Of the four metals associated with maternal circulating EV miRNA in the current study, Hg has been studied most extensively, although few studies have investigated its impacts on miRNA expression. One small study (N = 60) of pregnant women in Mexico City evaluated maternal toenail Hg concentrations in relation to cervical miRNA in mid-pregnancy [Citation29]. They identified significant associations with 17 miRNA, including inverse associations with miR–203a-5p and miR–23b-3p [Citation29]. In contrast, we observed positive associations between maternal Hg and these miRNA. A small study (N = 43) in the National Children’s Study also reported associations between Hg concentrations and multiple miRNA in the placenta, including several members of the let-7 family [Citation82], but none of these miRNA were significantly associated with urinary Hg in the current study. These discrepancies may be due to our evaluation of miRNA in maternal circulating EVs, rather than in cervical or placental tissue, or the different Hg biomarkers utilized which may represent different forms of Hg (e.g., inorganic/elemental Hg versus methylHg). A third very small study (N = 30) reported two miRNA (miR–92a and miR–486) that were upregulated in the plasma of women occupationally exposed to Hg [Citation90]. However, neither miRNA met the detection thresholds for inclusion in our analyses. Although Ba and Tl are also extremely toxic and have been associated with adverse pregnancy and birth outcomes [Citation14,Citation91–97], we are unaware of prior studies investigating their impacts on miRNA expression. Interestingly, the patterns of association for Ba and maternal circulating EV miRNA were very similar to those for Hg. Although this pair of metals was positively and significantly correlated with each other, their associations with the eight common miRNA persisted in an environmental mixture model, which suggests that their effects may be independent. In contrast, associations between Tl and these miRNA were consistently in the opposite direction. While surprising, given that Tl is also very toxic, one potential explanation may be unmeasured confounding from diet, as contaminated vegetable consumption is a major source of Tl exposure [Citation98].
Interestingly, the majority of metal-miRNA associations were restricted to the early pregnancy period. When comparing early pregnancy metal associations with miRNA measures in late pregnancy, only one association (an inverse association between Co and miR–150-5p) was similar to that observed in early pregnancy and remained statistically significant after correcting for multiple testing. These findings suggest that metal impacts on maternal circulating EV miRNA are largely transient. However, these short-term effects may have important consequences, as maternal-foetal communication in early pregnancy is vital for the establishment and maintenance of pregnancy, including successful placentation [Citation99]. Consistent with this, we found that predicted target genes of seven of the eight common miRNA associated with Ba, Hg, and Tl were significantly enriched in the EGFR pathway, which regulates placental development and plays important roles in endometrial function in early pregnancy [Citation100–102]. Predicted target genes of five of the eight common miRNA were also significantly enriched in the VEGF pathway, which is critical for placental vasculogenesis [Citation103]. Dysregulation of both pathways has been implicated in the development of pregnancy complications, including preeclampsia and intrauterine growth restriction [Citation100,Citation103,Citation104]. Collectively, our findings suggest that Ba, Hg, and Tl may impact a common set of miRNA which target pathways critical for normal placental development and other key biological processes that are essential in early pregnancy.
During pregnancy, it has been estimated that approximately 20% of EVs in maternal circulation are released from the placenta [Citation36]. The majority of maternal circulating EV miRNA that were associated with metal exposures in MADRES are expressed in the placenta, and 13 of these are very highly expressed [Citation78]. It is therefore possible that some of these miRNA originate in the placenta, which is sensitive to metal exposures [Citation26,Citation27,Citation105]. However, given that only one of the metal-associated miRNA (miR–1323) is placenta-specific [Citation78], we cannot rule out the possibility that other miRNA may have been released from maternal tissues. Although the origins of maternal circulating EVs have not been well-studied in pregnant women, haematopoietic cells and adipose tissue are estimated to contribute to the largest fraction of circulating EVs among non-pregnant adults and may also be major sources of circulating EVs during pregnancy [Citation106].
In contrast with the large number of maternal circulating EV miRNA associated with Ba, Hg, and Tl, the only significant association identified for Co was an inverse association with miR–150-5p. A previous study reported that this miRNA is upregulated in placental tissue of women with preeclampsia [Citation107]. First trimester circulating levels of miR–150-5p have also been associated with an increased risk for preterm birth [Citation108]. The inverse association between Co and miR–150-5p may therefore indicate a possible protective role for this essential metal during pregnancy. Interestingly, the five other metals evaluated (Cd, Mo, Ni, Sb, Sn) were not significantly associated with any of the EV miRNA after correcting for multiple testing. Although a small number of epidemiological studies have investigated these metals in relation to miRNA and identified significant associations, they focused on men who were exposed occupationally to much higher levels [Citation83–85]. Urinary metal concentrations in MADRES were generally low. For example, urinary Cd, Co, Ni, Sb, and Sn concentrations were, on average, similar to or lower than concentrations reported for pregnant women in the U.S. National Health and Nutrition Examination Survey (NHANES) as well as other populations both in the U.S. and abroad [Citation12,Citation109–112]. In contrast, Ba and Hg concentrations were higher on average in MADRES compared with pregnant women in NHANES and pregnancy cohorts located in other parts of the U.S., including Boston and Puerto Rico [Citation12,Citation109,Citation110]. These higher exposure levels may be one explanation for why Ba and Hg were associated with multiple EV miRNA in maternal circulation, while others such as Cd were not.
The current study was strengthened by our investigation of multiple metals, including several understudied metals of concern; the use of an environmental mixture modeling approach to simultaneously evaluate multiple correlated metals; and our extensive profiling of miRNA in maternal circulating EVs. Our sample size (N = 231) was also large compared with previous studies, which allowed us to comprehensively adjust for potential confounders and account for multiple testing. A novel aspect of our study was the availability of paired miRNA measures for a subset of participants in early and late pregnancy. This provided us with the opportunity to investigate whether metal-miRNA relationships are transient or persist across pregnancy. Another strength was our focus on a population that is underrepresented in epigenetic research that has a high prevalence of both multipollutant exposures and pregnancy complications. There are also several important limitations that need to be considered. First, given the unique characteristics of the MADRES cohort, findings may not be generalizable to other populations. Additionally, some of the metals evaluated (e.g., Co) have short half-lives, thus urinary concentrations may reflect very recent exposures. Furthermore, diet may be an important confounder for some of the metals evaluated, but detailed dietary data was not available for most MADRES participants in early pregnancy. It is also important to acknowledge that we measured total EVs in maternal circulation, which reflect EVs derived from different cells and tissues. Although 20% of EVs in maternal circulation during pregnancy are estimated to originate in the placenta, the remaining fraction reflect a heterogenous mixture of EVs released from multiple tissues [Citation36,Citation106]. Future studies which can distinguish EV subpopulations based on tissue origin will therefore be critical for improving understanding of the impacts of metals and other environmental contaminants on maternal circulating EV miRNA during pregnancy. Follow-up studies using experimental models will also be important for validating and better understanding the downstream effects of these metal-miRNA associations on predicted target genes and pathways.
In conclusion, we identified multiple EV miRNA in maternal circulation that are associated with metals in early pregnancy, including a common set of miRNA that may be impacted by multiple metals which target pathways important for placental development. With the exception of the inverse association identified between Co and miR–150-5p, metal-miRNA associations did not persist into late pregnancy. This suggests that metal impacts on maternal circulating EV miRNA in pregnancy are largely transient, although these short-term alterations may still have important downstream effects, given the critical roles that EV miRNA play in facilitating maternal-foetal communication in early pregnancy. To our knowledge this is the first study to investigate metal impacts on maternal circulating EV miRNA during pregnancy. Given the growing number of studies demonstrating links between maternal circulating miRNA and pregnancy complications, and the high prevalence of metal co-exposures, additional studies on this topic are greatly needed.
Supplemental Material
Download Zip (2.8 MB)Acknowledgments
We thank the MADRES participants, the study staff, and our community clinic partners for their many contributions to this work.
Disclosure statement
No potential conflicts of interest were reported by the authors.
Supplementary material
Supplemental data for this article can be accessed here.
Additional information
Funding
References
- Shim YK, Lewin MD, Ruiz P, et al. Prevalence and associated demographic characteristics of exposure to multiple metals and their species in human populations: the United States NHANES, 2007-2012. J Toxicol Environ Health A. 2017;80(9):502–512.
- Borowska S, Brzóska MM. Metals in cosmetics: implications for human health. J Appl Toxicol. 2015;35(6):551–572.
- Centers for Disease Control and Prevention (CDC). Mercury exposure among household users and nonusers of skin-lightening creams produced in Mexico - California and Virginia, 2010. MMWR Morb Mortal Wkly Rep. 2012;61:33–36.
- Jandacka D, Durcanska D, Bujdos M. The contribution of road traffic to particulate matter and metals in air pollution in the vicinity of an urban road. Transp Res D Transp Environ. 2017;50:397–408.
- Caruso RV, O’Connor RJ, Stephens WE, et al. Toxic metal concentrations in cigarettes obtained from U.S. smokers in 2009: results from the International Tobacco Control (ITC) United States survey cohort. Int J Environ Res Public Health. 2013;11(1):202–217.
- Hoover JH, Coker E, Barney Y, et al. Spatial clustering of metal and metalloid mixtures in unregulated water sources on the Navajo Nation - Arizona, New Mexico, and Utah, USA. Sci Total Environ. 2018;633:1667–1678.
- Patel AI, Hecht CE, Cradock A, et al. Drinking water in the United States: Implications of water safety, access, and consumption. Annu Rev Nutr. 2020;40(1):345–373.
- Wilson D. Arsenic consumption in the United States. J Environ Health. 2015;78:8–14.
- Davis MA, Gilbert-Diamond D, Karagas MR, et al. A dietary-wide association study (DWAS) of environmental metal exposure in US children and adults. PLoS One. 2014;9(9):e104768.
- Kahn LG, Trasande L. Environmental Toxicant Exposure and Hypertensive Disorders of Pregnancy: recent Findings. Curr Hypertens Rep. 2018;20(10):87.
- Khoshhali M, Rafiei N, Farajzadegan Z, et al. Maternal exposure to cadmium and fetal growth: a systematic review and meta-analysis. Biol Trace Elem Res. 2020;195(1):9–19.
- Kim SS, Meeker JD, Carroll R, et al. Urinary trace metals individually and in mixtures in association with preterm birth. Environ Int. 2018;121:582–590.
- Howe CG, Claus Henn B, Eckel SP, et al. Prenatal Metal Mixtures and Birth Weight for Gestational Age in a Predominately Lower-Income Hispanic Pregnancy Cohort in Los Angeles. Environ Health Perspect. 2020;128(11):117001.
- Howe CG, Claus Henn B, Farzan SF, et al. Prenatal metal mixtures and fetal size in mid-pregnancy in the MADRES study. Environ Res. 2021;196:110388.
- Liu T, Zhang M, Guallar E, et al. Trace Minerals, Heavy Metals, and Preeclampsia: findings from the Boston Birth Cohort. J Am Heart Assoc. 2019;8(16):e012436.
- Wang Y, Wang K, Han T, et al. Exposure to multiple metals and prevalence for preeclampsia in Taiyuan, China. Environ Int. 2020;145:106098.
- Wang X, Gao D, Zhang G, et al. Exposure to multiple metals in early pregnancy and gestational diabetes mellitus: a prospective cohort study. Environ Int. 2020;135:105370.
- Liu W, Zhang B, Huang Z, et al. Cadmium Body Burden and Gestational Diabetes Mellitus: a Prospective Study. Environ Health Perspect. 2018;126(2):027006.
- Gu S, Dai J, Qu T, et al. Emerging Roles of MicroRNAs and Long Noncoding RNAs in Cadmium Toxicity. Biol Trace Elem Res. 2020;195(2):481–490.
- Martin EM, Fry RC. Environmental Influences on the Epigenome: exposure- Associated DNA Methylation in Human Populations. Annu Rev Public Health. 2018;39(1):309–333.
- Bommarito PA, Martin E, Fry RC. Effects of prenatal exposure to endocrine disruptors and toxic metals on the fetal epigenome. Epigenomics. 2017;9(3):333–350.
- Xiao L, Zan G, Feng X, et al. The associations of multiple metals mixture with accelerated DNA methylation aging. Environ Pollut. 2021;269:116230.
- Vidal AC, Semenova V, Darrah T, et al. Maternal cadmium, iron and zinc levels, DNA methylation and birth weight. BMC Pharmacol Toxicol. 2015;16:20.
- Kippler M, Engström K, Mlakar SJ, et al. Sex-specific effects of early life cadmium exposure on DNA methylation and implications for birth weight. Epigenetics. 2013;8(5):494–503.
- Wallace DR, Taalab YM, Heinze S, et al. Toxic-Metal-Induced Alteration in miRNA Expression Profile as a Proposed Mechanism for Disease Development. Cells. 2020;9(4):901.
- Li Q, Kappil MA, Li A, et al. Exploring the associations between microRNA expression profiles and environmental pollutants in human placenta from the National Children’s Study (NCS). Epigenetics. 2015;10(9):793–802.
- Rahman ML, Liang L, Valeri L, et al. Regulation of birthweight by placenta-derived miRNAs: evidence from an arsenic-exposed birth cohort in Bangladesh. Epigenetics. 2018;13(6):573–590.
- Rager JE, Bailey KA, Smeester L, et al. Prenatal arsenic exposure and the epigenome: altered microRNAs associated with innate and adaptive immune signaling in newborn cord blood. Environ Mol Mutagen. 2014;55:196–208.
- Sanders AP, Burris HH, Just AC, et al. Altered miRNA expression in the cervix during pregnancy associated with lead and mercury exposure. Epigenomics. 2015;7(6):885–896.
- Catalanotto C, Cogoni C, Zardo G. MicroRNA in Control of Gene Expression: an Overview of Nuclear Functions. Int J Mol Sci. 2016;17(10):1712.
- Zhang J, Li S, Li L, et al. Exosome and Exosomal MicroRNA: trafficking, Sorting, and Function. Genomics Proteomics Bioinformatics. 2015;13(1):17–24.
- O’Brien K, Breyne K, Ughetto S, et al. RNA delivery by extracellular vesicles in mammalian cells and its applications. Nat Rev Mol Cell Biol. 2020;21(10):585–606.
- Fabbri M. MicroRNAs and miRceptors: a new mechanism of action for intercellular communication. Philos Trans R Soc Lond B Biol Sci. 2018;373(1737):20160486.
- Sarker S, Scholz-Romero K, Perez A, et al. Placenta-derived exosomes continuously increase in maternal circulation over the first trimester of pregnancy. J Transl Med. 2014;12:204.
- Salomon C, Torres MJ, Kobayashi M, et al. A Gestational Profile of Placental Exosomes in Maternal Plasma and Their Effects on Endothelial Cell Migration. PLoS One. 2014;9(6):e98667.
- Elfeky O, Longo S, Lai A, et al. Influence of maternal BMI on the exosomal profile during gestation and their role on maternal systemic inflammation. Placenta. 2017;50:60–69.
- Gillet V, Ouellet A, Stepanov Y, et al. miRNA Profiles in Extracellular Vesicles From Serum Early in Pregnancies Complicated by Gestational Diabetes Mellitus. J Clin Endocrinol Metab. 2019;104(11):5157–5169.
- Li H, Ouyang Y, Sadovsky E, et al. Unique microRNA Signals in Plasma Exosomes from Pregnancies Complicated by Preeclampsia. Hypertension. 2020;75(3):762–771.
- Hromadnikova I, Dvorakova L, Kotlabova K, et al. The Prediction of Gestational Hypertension, Preeclampsia and Fetal Growth Restriction via the First Trimester Screening of Plasma Exosomal C19MC microRNAs. Int J Mol Sci. 2019;20(12):2972.
- Salomon C, Guanzon D, Scholz-Romero K, et al. Placental Exosomes as Early Biomarker of Preeclampsia: potential Role of Exosomal MicroRNAs Across Gestation. J Clin Endocrinol Metab. 2017;102(9):3182–3194.
- Menon R, Debnath C, Lai A, et al. Garbhini Study Team. Circulating Exosomal miRNA Profile During Term and Preterm Birth Pregnancies: a Longitudinal Study. Endocrinology. 2019;160(2):249–275.
- Fallen S, Baxter D, Wu X, et al. Extracellular vesicle RNA s reflect placenta dysfunction and are a biomarker source for preterm labour. J Cell Mol Med. 2018;22(5):2760–2773.
- Howe CG, Foley HB, Kennedy EM, et al. Extracellular Vesicle MicroRNA in Early versus Late Pregnancy with Birth Outcomes in the MADRES Study. Epigenetics. 2021;1–17. DOI:10.1080/15592294.2021.1899887.
- Rodosthenous RS, Burris HH, Sanders AP, et al. Second trimester extracellular microRNAs in maternal blood and fetal growth: an exploratory study. Epigenetics. 2017;12(9):804–810.
- Li J, Xue J, Ling M, et al. MicroRNA-15b in extracellular vesicles from arsenite-treated macrophages promotes the progression of hepatocellular carcinomas by blocking the LATS1-mediated Hippo pathway. Cancer Lett. 2021;497:1–17.
- Ngalame NNO, Luz AL, Makia N, et al. Arsenic Alters Exosome Quantity and Cargo to Mediate Stem Cell Recruitment Into a Cancer Stem Cell-Like Phenotype. Toxicol Sci. 2018;165(1):40–49.
- Xu Y, Luo F, Liu Y, et al. Exosomal miR–21 derived from arsenite-transformed human bronchial epithelial cells promotes cell proliferation associated with arsenite carcinogenesis. Arch Toxicol. 2015;89(7):1071–1082.
- Dai X, Chen C, Yang Q, et al. Exosomal circRNA_100284 from arsenite-transformed cells, via microRNA-217 regulation of EZH2, is involved in the malignant transformation of human hepatic cells by accelerating the cell cycle and promoting cell proliferation. Cell Death Dis. 2018;9(5):454.
- Dai X, Chen C, Xue J, et al. Exosomal MALAT1 derived from hepatic cells is involved in the activation of hepatic stellate cells via miRNA-26b in fibrosis induced by arsenite. Toxicol Lett. 2019;316:73–84.
- Chen C, Luo F, Liu X, et al. NF-kB-regulated exosomal miR–155 promotes the inflammation associated with arsenite carcinogenesis. Cancer Lett. 2017;388:21–33.
- Gao M, Dong Z, Sun J, et al. Liver-derived exosome-laden lncRNA MT1DP aggravates cadmium-induced nephrotoxicity. Environ Pollut. 2020;258:113717.
- Harischandra DS, Ghaisas S, Rokad D, et al. Environmental neurotoxicant manganese regulates exosome-mediated extracellular miRNAs in cell culture model of Parkinson’s disease: relevance to α-synuclein misfolding in metal neurotoxicity. Neurotoxicology. 2018;64:267–277.
- Harischandra DS, Rokad D, Neal ML, et al. Manganese promotes the aggregation and prion-like cell-to-cell exosomal transmission of α-synuclein. Sci Signal. 2019;12(572):eaau4543.
- Bastain TM, Chavez T, Habre R, et al. Study Design, Protocol and Profile of the Maternal And Developmental Risks from Environmental and Social Stressors (MADRES) Pregnancy Cohort: a Prospective Cohort Study in Predominantly Low-Income Hispanic Women in Urban Los Angeles. BMC Pregnancy Childbirth. 2019;19(1):189.
- Kravchenko J, Darrah TH, Miller RK, et al. A review of the health impacts of barium from natural and anthropogenic exposure. Environ Geochem Health. 2014;36(4):797–814.
- Vacchi-Suzzi C, Kruse D, Harrington J, et al. Is Urinary Cadmium a Biomarker of Long-term Exposure in Humans? A Review. Curr Environ Health Rep. 2016;3(4):450–458.
- Faroon O, Afroz S. Toxicological Profile for Cobalt. Agency for Toxic Substances and Disease Registry. 2004.
- Branco V, Caito S, Farina M, et al. Biomarkers of mercury toxicity: past, present, and future trends. J Toxicol Environ Health B Crit Rev. 2017;20(3):119–154.
- Todd G, Keith S, Faroon O, et al. Toxicological Profile for Molybdenum. ATSDR. 2020.
- Fay M. Toxicological Profile for Nickel. ATSDR. 2005.
- ATSDR. Toxicological Profile for Antimony and its Compounds. ATSDR. 2019.
- Harper C. Toxicological Profile for Tin and Tin Compounds. ATSDR. 2005.
- Sturini M, Maraschi F, Cucca L, et al. Toxicological Profile for Thallium. ATSDR. 2002.
- Sun T, Gonzalez TL, Deng N, et al. Sexually Dimorphic Crosstalk at the Maternal-Fetal Interface. J Clin Endocrinol Metab. 2020;105(12):e4831-e4847.
- Foley HB, Howe CG, Eckel SP, et al. Extracellular vesicle-enriched miRNA profiles across pregnancy in the MADRES cohort. PLoS One. 2021;16(5):e0251259.
- Enderle D, Spiel A, Coticchia CM, et al. Characterization of RNA from Exosomes and Other Extracellular Vesicles Isolated by a Novel Spin Column-Based Method. PLoS ONE. 2015;10(8):e0136133.
- Waggott D, Chu K, Yin S, et al. NanoStringNorm: an extensible R package for the pre-processing of NanoString mRNA and miRNA data. Bioinformatics. 2012;28(11):1546–1548.
- R Core Team.R: a language and environment for statistical computing [Internet].R Fonudation for Statistical Computing:Vienna (Austria) 2017. Available from https://www.R-project.org/
- Wood SN. Generalized additive models: an introduction with R. Second edition ed. Boca Raton: CRC Press/Taylor & Francis Group; 2017.
- Breheny P, Burchett W. Visualization of Regression Models Using visreg. 2013.
- Blighe K, Rana S, Turkes E, et al. EnhancedVolcano: publication-ready volcano plots with enhanced colouring and labeling [Internet]. Bioconductor version: Release (3.13); 2021 [cited 2021 Jun 3]. Available from: https://bioconductor.org/packages/EnhancedVolcano/
- Conway JR, Lex A, Gehlenborg N. UpSetR: an R package for the visualization of intersecting sets and their properties. Bioinformatics. 2017;33(18):2938–2940.
- Bobb JF, Valeri L, Claus Henn B, et al. Bayesian kernel machine regression for estimating the health effects of multi-pollutant mixtures. Biostatistics. 2015;16(3):493–508.
- Bobb JF, Claus Henn B, Valeri L, et al. Statistical software for analyzing the health effects of multiple concurrent exposures via Bayesian kernel machine regression. Environ Health. 2018;17(1):67.
- Tokar T, Pastrello C, Rossos AEM, et al. mirDIP 4.1-integrative database of human microRNA target predictions. Nucleic Acids Res. 2018;46(D1):D360–70.
- Rahmati S, Abovsky M, Pastrello C, et al. pathDIP 4: an extended pathway annotations and enrichment analysis resource for human, model organisms and domesticated species. Nucleic Acids Res. 2020;48:D479–88.
- Chang L, Zhou G, Soufan O, et al. miRNet 2.0: network-based visual analytics for miRNA functional analysis and systems biology. Nucleic Acids Res. 2020;48(W1):W244–51.
- Gonzalez TL, Eisman LE, Joshi NV, et al. High-throughput miRNA sequencing of the human placenta: expression throughout gestation. Epigenomics. 2021;13(13):995–1012.
- Czernek L, Düchler M. Exosomes as Messengers between Mother and Fetus in Pregnancy. Int J Mol Sci. 2020;21(12):4264.
- Guo X, Yang Q, Zhang W, et al. Associations of blood levels of trace elements and heavy metals with metabolic syndrome in Chinese male adults with microRNA as mediators involved. Environ Pollut. 2019;248:66–73.
- Kong APS, Xiao K, Choi KC, et al. Associations between microRNA (miR–21, 126, 155 and 221), albuminuria and heavy metals in Hong Kong Chinese adolescents. Clin Chim Acta. 2012;413(13–14):1053–1057.
- Dioni L, Sucato S, Motta V, et al. Urinary chromium is associated with changes in leukocyte miRNA expression in obese subjects. Eur J Clin Nutr. 2017;71(1):142–148.
- Bollati V, Marinelli B, Apostoli P, et al. Exposure to metal-rich particulate matter modifies the expression of candidate microRNAs in peripheral blood leukocytes. Environ Health Perspect. 2010;118(6):763–768.
- Deng Q, Dai X, Feng W, et al. Co-exposure to metals and polycyclic aromatic hydrocarbons, microRNA expression, and early health damage in coke oven workers. Environ Int. 2019;122:369–380.
- Amrani I, Haddam N, Garat A, et al. Exposure to metal fumes and circulating miRNAs in Algerian welders. Int Arch Occup Environ Health. 2020;93(5):553–561.
- Beck R, Bommarito P, Douillet C, et al. Circulating miRNAs associated with arsenic exposure. Environ Sci Technol. 2018;52(24):14487–14495.
- Chen QY, Shen S, Sun H, et al. PBMC gene expression profiles of female Bangladeshi adults chronically exposed to arsenic-contaminated drinking water. Environ Pollut. 2020;259:113672.
- Ruíz-Vera T, Ochoa-Martínez ÁC, Zarazúa S, et al. Circulating miRNA-126, −145 and −155 levels in Mexican women exposed to inorganic arsenic via drinking water. Environ Toxicol Pharmacol. 2019;67:79–86.
- Pérez-Vázquez MS, Ochoa-Martínez ÁC, Ruíz-vera T, et al. Evaluation of epigenetic alterations (miR–126 and miR–155 expression levels) in Mexican children exposed to inorganic arsenic via drinking water. Environ Sci Pollut Res Int. 2017;24(36):28036–28045.
- Ding E, Zhao Q, Bai Y, et al. Plasma microRNAs expression profile in female workers occupationally exposed to mercury. J Thorac Dis. 2016;8(5):833–841.
- Goodrich JM, Ingle ME, Domino SE, et al. First trimester maternal exposures to endocrine disrupting chemicals and metals and fetal size in the Michigan Mother-Infant Pairs study. J Dev Orig Health Dis. 2019;10(4):447–458.
- Bermúdez L, García-Vicent C, López J, et al. Assessment of ten trace elements in umbilical cord blood and maternal blood: association with birth weight. J Transl Med. 2015;13(1):291.
- Jiang Y, Xia W, Zhang B, et al. Predictors of thallium exposure and its relation with preterm birth. Environ Pollut. 2018;233:971–976.
- Xia W, Du X, Zheng T, et al. A Case-Control Study of Prenatal Thallium Exposure and Low Birth Weight in China. Environ Health Perspect. 2016;124(1):164–169.
- Hu JMY, Arbuckle TE, Janssen P, et al. Prenatal exposure to endocrine disrupting chemical mixtures and infant birth weight: a Bayesian analysis using kernel machine regression. Environ Res. 2021;195:110749.
- Zhang -Q-Q, Li J-H, Wang Y-D, et al. Association between maternal thallium exposure and risk of gestational diabetes mellitus: evidence from a birth cohort study. Chemosphere. 2021;270:128637.
- Zhu B, Liang C, Yan S, et al. Association between serum thallium in early pregnancy and risk of gestational diabetes mellitus: the Ma’anshan birth cohort study. J Trace Elem Med Biol. 2019;52:151–156.
- Peter ALJ, Viraraghavan T. Thallium: a review of public health and environmental concerns. Environ Int. 2005;31(4):493–501.
- Lash GE. Molecular Cross-Talk at the Feto–Maternal Interface.Cold Spring Harb Perspect Med. 2015. Available from https://www.ncbi.nlm.nih.gov/pmc/articles/PMC4665040/
- Hastie R, Brownfoot FC, Pritchard N, et al. EGFR (Epidermal Growth Factor Receptor) Signaling and the Mitochondria Regulate sFlt-1 (Soluble FMS-Like Tyrosine Kinase-1) Secretion. Hypertension. 2019;73(3):659–670.
- Large MJ, Wetendorf M, Lanz RB, et al. The epidermal growth factor receptor critically regulates endometrial function during early pregnancy. PLoS Genet. 2014;10(6):e1004451.
- Evain-Brion D, Alsat E, Roulier S, et al. Placental Epidermal Growth Factor Receptors: from Physiology to Pathology. Pediatr Res. 1993;33:S7–8.
- Umapathy A, Chamley LW, James JL. Reconciling the distinct roles of angiogenic/anti-angiogenic factors in the placenta and maternal circulation of normal and pathological pregnancies. Angiogenesis. 2020;23(2):105–117.
- Armant DR, Fritz R, Kilburn BA, et al. Reduced expression of the epidermal growth factor signaling system in preeclampsia. Placenta. 2015;36(3):270–278.
- Deyssenroth MA, Gennings C, Liu SH, et al. Intrauterine multi-metal exposure is associated with reduced fetal growth through modulation of the placental gene network. Environ Int. 2018;120:373–381.
- Li Y, He X, Li Q, et al. EV-origin: enumerating the tissue-cellular origin of circulating extracellular vesicles using exLR profile. Comput Struct Biotechnol J. 2020;18:2851–2859.
- Zeng Y, Wei L, Lali MS, et al. miR–150-5p mediates extravillous trophoblast cell migration and angiogenesis functions by regulating VEGF and MMP9. Placenta. 2020;93:94–100.
- Cook J, Bennett PR, Kim SH, et al. First Trimester Circulating MicroRNA Biomarkers Predictive of Subsequent Preterm Delivery and Cervical Shortening. Sci Rep. 2019;9(1):5861.
- Watson CV, Lewin M, Ragin-Wilson A, et al. Characterization of trace elements exposure in pregnant women in the United States, NHANES 1999-2016. Environ Res. 2020;183:109208.
- Ashrap P, Watkins DJ, Mukherjee B, et al. Predictors of urinary and blood Metal(loid) concentrations among pregnant women in Northern Puerto Rico. Environ Res. 2020;183:109178.
- Howe CG, Margetaki K, Vafeiadi M, et al. Prenatal metal mixtures and child blood pressure in the Rhea mother-child cohort in Greece. Environ Health. Internet 2021; 20(1). Available from https://www.ncbi.nlm.nih.gov/pmc/articles/PMC7789252/
- Lewis RC, Meeker JD, Basu N, et al. Urinary metal concentrations among mothers and children in a Mexico City birth cohort study. Int J Hyg Environ Health. 2018;221:609–615.