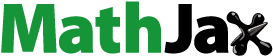
ABSTRACT
The role of DNA methylation and its interplay with gene expression in the susceptibility to pancreatic cancer (PanC) remains largely unexplored. To fill in this gap, we conducted an integrative two-phase epigenome-wide association study (EWAS) of PanC using genomic DNA from 44 cases and 556 controls (20 local controls and 536 public controls in the Framingham Heart Study) in phase 1 and 23 cases and 22 controls in phase 2. We validated the findings using pre-diagnostic blood samples from 13 cases and 26 controls in the Women’s Health Initiative (WHI) Study. We further examined gene expression in peripheral leukocytes of 47 cases and 31 controls involved in the methylation study using RNA sequencing and performed bidirectional Mendelian randomization (MR) analysis using existing single nucleotide polymorphism (SNP) data. In phase 1, we identified 2776 significantly differentially methylated CpG sites (DMPs) and 154 significantly differentially methylated regions (DMRs). In phase 2, we validated six DMPs (in or near AIM2, DGKA, STK39, and TNFSF8) and three DMRs (in or near nc886, LY6G5C, and HLA-DPB1). The DMR near nc886 was further validated in the WHI samples (P = 6.69 × 10−5). MR analysis suggested that the CpG sites cg00308130 and cg16684184 for nc886 and cg16875057 for STK39 were causally related to PanC susceptibility and that PanC influenced methylation of cg15354065 for DGKA. This first integrative EWAS of PanC provides novel insights into the role of DNA methylation and its interplay with SNPs and gene expression in the disease susceptibility.
Introduction
Pancreatic cancer (PanC) is the fourth leading cause of cancer-related deaths in both men and women in the United States, with 60,430 estimated new cases and 48,220 estimated deaths in 2021 [Citation1]. The poor prognosis of PanC is largely because of a lack of effective early detection tools and treatment strategies. The majority of PanC is caused by the interplay of genetic and environmental factors. Despite advances in the understanding of genetic variants, lifestyle factors, and gene-environment interactions [Citation2–9], much of the interindividual variation in PanC susceptibility remains unexplained by measurable lifestyle and genetic factors [Citation7,Citation9]. At the molecular level, DNA methylation, one of the most frequent and well-characterized epigenetic modifications, reflects a wide range of genetic influences and environmental exposures [Citation10]. In contrast to genetic variants, DNA methylation is reversible and therefore an appealing target for new treatment strategies. Hypermethylation, particularly that in promoter regions that leads to transcriptional gene silencing or repressed gene expression, and global hypomethylation, which is thought to contribute to chromosomal instability and activate the expression of proto-oncogenes, are both frequently observed in human cancers [Citation11].
Although researchers have well-studied aberrations of DNA methylation in pancreatic tumours in The Cancer Genome Atlas (TCGA) and the International Cancer Genome Consortium projects [Citation12,Citation13], whether and, if so, how changes in DNA methylation influence susceptibility to PanC are unclear. A previous study showed a significant association of DNA methylation profiles in leukocytes with risk of PanC [Citation14]. These investigators measured the DNA methylation status of up to 1505 CpG sites and found significant differences in methylation profiles in 110 CpG sites with a false discovery rate less than 0.05 between PanC cases and non-cancer controls. A recent nested case–control study identified two CpG sites to be associated with PanC risk in pre-diagnostic blood samples among 50 preselected CpG sites in association with inflammatory blood markers [Citation15]. These findings strongly support using leukocyte DNA from blood for PanC biomarker discovery.
With advances in technology, the genome-wide methylation status can be measured using Infinium MethylationEPIC BeadChip, which covers about 850,000 CpG sites. Epigenome-wide association studies (EWASs) for several human cancers have been reported, including colon [Citation16], breast [Citation17], lung [Citation18], and more recently, PanC [Citation19]. However, the potential causal role of DNA methylation in influencing PanC susceptibility and the functional consequences of PanC-associated differential methylation on gene expression have yet to be investigated. To fill this knowledge gap, as illustrated in , we performed an integrative analysis of EWAS, RNA-sequencing and single nucleotide polymorphism (SNP) data from a genome-wide association study (GWAS) to identify PanC-associated CpG sites and regions, as well as their potential causal roles in PanC susceptibility and functional effects on gene expression. Our integrative EWAS design is in line with recent EWAS of complex traits such as blood pressure [Citation20] and body mass index (BMI) [Citation21]. In our two-phase integrative EWAS, we innovatively leveraged 536 public controls in the Framingham Heart Study (FHS) to supplement the 44 cases and 20 controls collected at the University of Texas MD Anderson Cancer Center in phase 1, followed by validation in 23 cases and 22 controls from MD Anderson and the pre-diagnostic blood samples from 13 cases and 26 controls in the Women’s Health Initiative (WHI) in phase 2. Furthermore, we employed state-of-the-art bidirectional Mendelian randomization (MR) analysis to identify CpG sites causally related to PanC susceptibility, as well as triangular association analysis of DNA methylation, gene expression and PanC risk to characterize the functional effects of PanC-associated CpG sites and regions on gene expression. This first integrative EWAS of PanC provides novel insights into the potential roles of DNA methylation in PanC susceptibility.
Materials and methods
Study population
MD Anderson (MDA). The MDA study participants were drawn from a case–control study of PanC conducted from 2000 to 2009 [Citation22,Citation23]. The cases were patients with pathologically confirmed pancreatic ductal adenocarcinoma. The controls were the spouses of cancer patients at MDA, excluding those of patients with PanC, gastrointestinal cancer, or smoking-related cancers (e.g., lung cancer, head and neck cancer). Information on the participants’ risk factors and medical history was collected via personal interviews, and blood samples were collected from all study participants. All selected participants were of European descent, free of diabetes, and had BMI in the normal range (18–25 kg/m2) at the time of recruitment. The cases and controls were frequency-matched according to age (±5 years), sex, and smoking status (ever versus never). Forty-four cases and 20 controls were selected for the phase 1 discovery study, and 23 cases and 22 controls were selected for the phase 2 validation study.
FHS. To increase the study power of the phase 1 study in a cost-effective way, we included additional publicly available data of healthy controls from the FHS offspring cohort. Unrelated individuals were identified based on pedigree information, and 536 qualified participants in the FHS were selected based on age (±5 years of the age range of MDA discovery study participants), diabetic status (negative), and BMI (controlled at the normal range of 18–25 kg/m2) reported for Exam 8, collected during 2005–2008. Diabetic status was determined using data reported in Exam 7, as this information in Exam 8 was not released at the time of our research. We performed power analysis of EWAS with 44 MDA cases and varying number of combined controls from MDA and FHS by a two-sample t-test at a significance level α = 1.2 × 10−7, the Bonferroni correction for 400,000 CpG sites as in the phase 1 discovery study. As shown in Figure S1, while the combined over 550 controls achieved >80% of power for CpG sites of large effect size with standardized mean difference of at least 1, the power was less than 5% for CpG sites of moderate effect size, e.g., a standardized mean difference of 0.5. Without a priori knowledge about the effect size distribution in the EWAS for PanC, we included all qualified FHS control samples in the phase 1 discovery study.
WHI. To further control the problem of reverse causation, data from a prospective study, the WHI, were used for verification of our findings. The WHI cohort consisted of healthy postmenopausal women 50–79 years old at the time of recruitment (1994–1998) with their blood collected at the initial time point of the study. As recorded in the data, 13 subjects were later diagnosed with incident PanC during 0.8–17.0 years of follow-up. Twenty-six healthy individuals who were free of cancer until the last recorded follow-up examination (2010) were selected for our study based on frequency matching according to race, diabetic status, age (±5 years), BMI, and smoking status (ever versus never).
Ethics approval
This study was approved by the University of Texas MD Anderson Cancer Center Institutional Review Board (Houston, Texas, USA).
DNA methylation profiling
For the MDA study, peripheral leukocytes were isolated and stored at −80°C before DNA isolation. DNA was extracted using a DNA purification kit (Promega, Madison, WI, USA) and the profiling of DNA methylation was conducted using Infinium MethylationEPIC BeadChip (Illumina, San Diego, CA, USA) at more than 850,000 CpG sites across the genome. For the FHS study, the DNA methylation profiles of the FHS offspring samples were collected from peripheral blood samples during Exam 8 (2005–2008), and measured using an Infinium HumanMethylation450 (450 K) BeadChip array (Illumina) at more than 480,000 CpG sites. The WHI study used DNA from peripheral blood samples collected at the time of recruitment, and methylation status was determined using the same platform as in the FHS study.
Data preprocessing and quality control
In phase 1, raw IDAT files from the MDA and FHS studies were jointly called for common DNA methylation CpG sites, which was followed by a normalization procedure using the functional normalization method in the R package Minfi [Citation24–26]. The detection P value, an indicator of the quality of the signal, was calculated to compare the total signal (methylated and unmethylated) for each probe with the background signal level in each individual. The CpG sites that failed (P > 0.01) in more than 10% of the total samples were filtered out. We excluded the probes on the sex chromosomes, the probes for target polymorphic CpGs that overlap with known SNPs, and the cross-reactive probes [Citation27,Citation28].
To remove the batch effects, ComBat was applied to the normalized data, with the FHS cohort adjusted based on the MD Anderson control group as the reference [Citation29]. Also, principal component analysis was conducted to provide evidence supporting the removal of between-cohort batch effects (Figure S2). 400,985 CpG sites of 600 individuals were retained for the downstream analysis in phase 1.
For the phase 2 MDA study and the WHI study for validation, the raw IDAT files were, respectively, preprocessed with the same quality control procedures, data normalization, and probe filtering as described for the phase 1 MDA study. For the phase 2 MDA study, 790,980 CpG sites of 45 individuals were available. For the WHI study, 430,471 CpG sites of 39 individuals were available for downstream analysis.
Identification and validation of the differentially methylated CpG sites (DMP)
For the discovery study (phase 1), probe-wise analysis for detecting differential DNA methylation probes was conducted using limma [Citation30]. The association of CpG sites with the risk of PanC was estimated using linear regression models with adjustment for age, sex, and smoking status. Modified t-statistics using the empirical Bayesian methodology and corresponding P values were obtained for each CpG site. Bonferroni correction was used to adjust for multiple hypothesis testing. The same covariates and methods were applied in the replication study with the phase 2 MDA cohort. All analyses were performed using the R computing language (version 3.4.1) [Citation31].
Identification and validation of differentially methylated regions (DMR)
The associations of methylation regions with PanC risk were tested independently in the discovery (N = 600) and replication (N = 45) phases in the MDA cohorts and the WHI cohort (N = 39) using the ‘bumphunter’ method [Citation24,Citation32] with adjustment for age, sex, and smoking status in the two MDA cohorts and race, diabetic status, age, BMI, and smoking status in the WHI cohort. Data-driven clusters (i.e., the methylation regions) were identified, with at least seven CpG sites included in each cluster and fewer than 500 base pairs between CpG sites within one cluster. At each CpG site, a linear model with methylation level as the dependent variable and disease status as the independent variable adjusted for the covariates described above was fitted. A Loess curve was used to smooth the summary statistics for the CpG sites. The cluster for which the smoothed estimate passed the cut-off threshold (99% of all estimates) was classified as a candidate methylation region. Then, each methylation region was assessed using 2,000 permutations to determine the P value of the candidate regions. Differentially methylated regions (DMRs) with P value less than 0.05 in both the discovery and replication data sets were defined as significant DMRs.
mRNA expression in the MDA cohort
DNA methylation in the promoter region is known to repress the target gene’s expression by impeding the binding of transcription factors and modifying chromatin structures, while DNA methylation in the gene body has been reported to be positively correlated with gene expression [Citation33]. To explore the influence of the PanC-associated CpG sites and regions on gene expression, RNA-sequencing was conducted in 78 MDA study subjects who had frozen lymphocytes available for RNA extraction, consisting of 29 cases and 13 controls in phase 1 and 18 cases and 18 controls in phase 2. Total RNA was extracted from the lymphocytes, and mRNA expression was measured using RNA sequencing at the Next-Generation Sequencing Core lab at MDA. The normalized fragment per kilobase of transcript per million value was used for downstream analysis. Logistic regression adjusted for age, sex, and smoking status was performed to examine the associations between gene transcription levels and PanC.
Correlations between DNA methylation and gene expression
Correlations between DNA methylation and gene expression (GE) data were analysed for the 47 cases and 31 controls using the nonparametric Kendall method. The annotations for CpG sites mapping to the University of California, Santa Cruz Genome Browser (UCSC) gene names (GRCH37/hg19) were provided by Illumina. This also included information on chromosomes, positions, regulatory features, enhancer regions, DNase I hypersensitivity sites, relationships with CpG Islands, and CpG Island names [Citation34,Citation35]. In addition, the correlations between DNA methylation and GE were analysed for the FHS data set to further corroborate the results of the MD Anderson study. GE profiling in the FHS cohort (N = 1,700) using fasting peripheral whole blood samples was analysed on the GeneChip Human Exon 1.0 ST platform (Affymetrix, Santa Cruz, CA, USA), details of which were described previously [Citation21,Citation36,Citation37].
Bidirectional MR
Next, causal inference of methylation levels with PanC was performed using a bidirectional MR approach with methylation quantitative trait loci (mQTLs) as the instrumental variables (IVs).
Forward MR (DNA methylation → PanC) was conducted using a two-sample inverse-variance weighted (IVW) method with summary statistics from a large public data study [Citation38]. The IVs and their summary statistics were drawn from the Accessible Resource for Integrative Epigenomic Studies (ARIES) mQTL database [Citation39]. The IVs were the cis-mQTLs within ±500 kb of the replicated significant CpG sites. SNPs in linkage disequilibrium with r2 values greater than 0.3 were pruned out. The associations of IVs with PanC status were evaluated using SNP data on the MDA study subjects in the Pancreatic Cancer Case-Control Consortium (PanC4) GWAS [Citation7]. The genotype data were imputed based on the 1000 Genomes platform using the Michigan Imputation Server. In total, 1,109 individuals (611 cases and 498 controls) who passed quality control measures as defined in [Citation7] were examined. The pleiotropic effect of the IVs was assessed using the heterogeneity test to ensure the validity of the IVW estimated MR [Citation40].
Reverse MR (PanC → DNA methylation) was performed to determine whether PanC causes changes in DNA methylation levels. The IV for PanC was assembled as an additive weighted genetic risk score (GRS) from the 27 significant SNPs identified in the most recent meta-analysis of GWAS conducted by the Pancreatic Cancer Cohort Consortium (PanScan) and PanC4 [Citation8]. The SNPs were extracted following the same procedure used in the forward MR, and the associations between the GRS and methylation levels were examined in 109 MDA study subjects with both GWAS and EWAS data. Direct association testing between the methylation levels and the GRS was performed to circumvent the difficulties of validating the MR exclusion restriction assumptions for a binary exposure, i.e., the disease status [Citation41–43].
Results
Characteristics of the study population
The frequency distribution of the characteristics of all the study participants in phases 1 and 2 is shown in . The MDA cases and controls were well matched by sex, age, and smoking status. The combined MDA and FHS controls in phase 1 had a higher proportion of female participants (P = 0.01), older individuals (P = 0.01), and non-smokers (P < 0.001) than did the cases.
Table 1. Individual characteristics for discovery and replication case–control studies.
Identification of differentially methylated CpG sites
In phase 1, we identified 2776 significant differentially methylated CpG sites (DMPs) after the Bonferroni correction (P < 0.05/400,985 = 1.2 × 10−7). In phase 2, 6 of 2774 CpG sites (2 CpG sites were absent from the phase 2 methylation data after quality control) were successfully replicated in association with PanC susceptibility based on a stringent multiple-testing threshold with P values less than 0.05/2774 = 1.8 × 10−5 (, ). The six replicated CpG sites were hypomethylated in PanC cases. Three CpG sites (cg27661394, cg10780778, and cg14113958) were in both the enhancer regions and DNase I hypersensitivity sites. One CpG site (cg16875057) was in the enhancer region, and another (cg15354065) was in the DNase I hypersensitivity sites. None of the CpG sites was co-hybridized to alternate sequences or target repetitive sequences, and none were polymorphic CpGs that overlapped known SNPs. cg10636246, cg15354065, and cg16875057 were located near the transcription start site of AIM2, 5ʹ-untranslated region (UTR) of DGKA, and enhancer region of the STK39 gene, respectively, according to UCSC annotation (). In addition, cg14113958 was located near the 3ʹ-UTR of TNFSF8.
Table 2. Top differentially methylated CpG sites in the discovery and replication samples.
Figure 2. Volcano plots of all CpG sites for the discovery phase 1 (left) and replication phase 2 (right) in the two-phase MD Anderson study. The blue dots indicate the six successfully replicated DMPs. The x-axis represents the log2 fold change: Log2FC =, with
representing the mean M values of each group. The y-axis represents the – log10 P value. The P values are from the EWAS. The discovery phase had a total of 400,985 CpGs, and the replication phase had a total of 790,980 CpGs. All successfully replicated CpG sites were hypomethylated in pancreatic cancer cases.
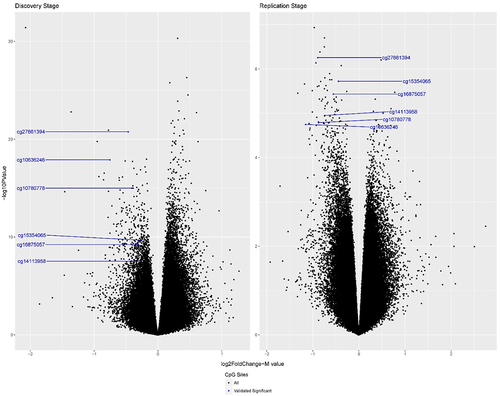
Identification of DMRs
In phase 1, we discovered 154 significant DMRs (P < 0.05), three of which were replicated in phase 2 ( and Supplementary Table S1, ). The three replicated DMRs consisted of one on chromosome 5 near gene nc886 (denoted DMR1) and two on chromosome 6: one near LY6G5C (DMR2) and the other near HLA-DPB1 (DMR3). In phase 1, DMR1 contained 14 CpG sites: 6 in the CpG Island (chr5:135,416,204–135,416,475), 6 within 0–2 kb upstream of the CpG Island (N shore), and 2 within 0–2 kb downstream from the CpG Island (S shore). The CpG Island was located at the transcription start site of nc886 (TSS200, TSS1500) and in the DNase I hypersensitivity region (chr5:135,415,705–135,416,015). DMR2 contained 19 CpG sites in the CpG Island (chr6:31,650,648–31,651,292) located at the transcription start site of LY6G5C (TSS200, TSS1500) and in the DNase I hypersensitivity region (chr6:31,650,845–31,651,375). DMR3 contained 12 CpG sites in the CpG Island (chr6:33,048,416–33,048,814) located at the gene body of HLA-DPB1 and in the DNase I hypersensitivity region (chr6:33,048,360–33,049,175). DMR1 and DMR3 were hypomethylated and DMR2 was hypermethylated in PanC cases.
Table 3. Top differentially methylated regions in the discovery and replication samples.
Validation in the WHI prospective study
In the prospective WHI study, we discovered 15 DMRs using the bumphunter method (P value < 0.05). One of these DMRs, which is located on chromosome 5 near gene nc886, overlapped one validated DMR in the MDA study. It contains 19 CpG sites, including two CpG Islands (chr5:135,415,069–135,415,307 and chr5:135,416,204–135,416,475), one of which is the same CpG Island previously discovered in the MDA study. Notably, this DMR was hypomethylated in the MDA cases but hypermethylated in the WHI cases (β = 0.107, p = 6.69 × 10−5, bumphunter P value = 3.24 × 10−4).
Associations of GE with replicated DMPs and DMRs
To further assess the potential functional effect of DNA methylation changes, we analysed the association of GE with methylation levels in the same whole blood samples. We analysed four genes (AIM2, DGKA, STK39, and TNFSF8) in the replicated DMPs and two genes (HLA-DPB1 and LY6G5C) in the replicated DMRs (Supplementary Table S2). Because the expression values of nc886 using RNA sequencing were filtered out by quality control, we used the expression level for the nc886 downstream target gene EIF2AK2 as a surrogate for that for nc886 in this analysis. We used the CpG sites in the largest DMRs discovered in the phase 1 and 2 and WHI cohorts, which were 17 CpG sites from DMR1, 19 CpG sites from DMR2, and 17 CpG sites from DMR3.
Significant correlations between GE level and DNA methylation level were observed in 5 CpG sites (MDA cases), 1 CpG site (MDA controls), and 13 CpG sites (FHS data) in DMR1 with EIF2AK2; 16 CpG sites (MDA controls), and 18 CpG sites (FHS data) in DMR2 with LY6G5C; 2 CpG sites (MDA cases), 10 CpG sites (MDA controls), and 16 CpG sites (FHS data) in DMR3 with HLA-DPB1; 4 CpG sites (MDA cases), 5 CpG sites (MDA controls), and 16 CpG sites (FHS data) in DMR3 with HLA-DPA1. The expression levels of AIM2, DGKA, STK39, and TNFSF8, genes near the validated DMPs, showed significant correlations with DNA methylation levels in the large FHS dataset.
After assessing the associations between GE and PanC status, we identified EIF2AK2, HLA-DPB1, and HLA-DPA1 to be nominally significant, which demonstrated triangular associations for DMR1 (near nc886) and DMR3 (near HLA-DPB1). Five CpG sites near nc886 were negatively correlated with the EIF2AK2 expression levels in PanC cases (), whereas the EIF2AK2 expression levels were significantly lower in PanC cases than in controls after adjusting for age, sex, and smoking status (β = – 0.130, SD = 0.055, P = 0.017) ()). In contrast, the EIF2AK2 expression level was positively associated with the methylation levels for the nc886 CpG sites among healthy individuals based on the FHS data (N = 1,700). The CpG sites in DMR3 had one or two cis-located genes (HLA-DPA1 and HLA-DPB1), the transcription levels for which were positively associated with methylation levels and negatively associated with PanC status after adjusting for age, sex, and smoking status (HLA-DPB1: β = – 0.012, SD = 0.005, P = 0.031; HLA-DPA1: β = – 0.020, SD = 0.010, P = 0.039) ()(d)). This analysis demonstrated that the majority of the DMPs and the CpG sites within the DMRs were located in cis-genes with low transcriptional activity. This finding is in accordance with the results of several studies of the association between GE analysis and DNA methylation [Citation20,Citation44].
Table 4. Association between nc866 methylation and EIF2AK2 expression in pancreatic cancer cases and controls.
Bidirectional MR
Forward MR. We tested the forward causality using independent mQTLs as the IVs found in the ARIES mQTL database for five CpG sites (cg10636246, cg15354065, cg16875057, cg00308130, and cg16684184) among the validated DMPs and CpGs within validated DMRs. We used the inverse-variance weighted (IVW) method to assess the forward causality of DNA methylation on PanC and discovered three significant causal CpG sites (cg16875057, cg00308130, and cg16684184). cg16875057 is one of the validated DMPs near STK39, and cg00308130 and cg16684184 are both in the DMR near nc886 (). Heterogeneity testing of the IVs demonstrated no significance, which indicated no pleiotropic effect in the analysis.
Table 5. Results of bi-directional Mendelian randomization.
Reverse MR. We used the additive weighted genetic risk score (GRS) of 27 known PanC loci [Citation8] as the IV in conducting the reverse causality tests for the five CpG sites subjected to forward causality testing. We examined the directional association in the combined 109 MD Anderson samples (64 in phase 1 and 45 in phase 2). We found no reverse association for the three significant forward causal CpG sites. Only cg15354065, located at the 5ʹ-UTR of DGKA, had a significant reverse association with PanC susceptibility (P = 0.012).
Discussion
In this two-phase integrative EWAS of PanC leveraging public control data on peripheral blood samples, we discovered and validated six DMPs (cg27661394, cg10780778, cg14113958, cg10636246, cg15354065, and cg16875057) and three DMRs (nc886, LY6G5C, and HLA-DPB1). An external validation using pre-diagnostic blood samples drawn from the WHI prospective cohort further confirmed the differential methylation of CpG sites near or in nc886. Furthermore, we discovered significant triangular associations among PanC disease status, gene expression, and methylation levels for nc886 and HLA-DPB1. MR tests additionally showed forward causal effects of DMP cg16875057 and DMR nc886 (cg00308130, cg16684184) on PanC susceptibility. This first integrative EWAS of PanC provides novel insights into the potential roles of DNA methylation in PanC susceptibility.
One of the major findings in the present study is the identification of nc886 DNA methylation as a susceptibility marker for PanC. nc886 (or VTRNA2-1) produces an RNA polymerase III transcripts and acts as a direct inhibitor of EIF2AK2. EIF2AK2 is known to encode PKR in humans [Citation45]. PKR is a major regulator of central cellular processes, including mRNA translation, transcriptional control, apoptosis regulation, and cell proliferation. nc886 is implicated to have a tumour-suppressing role for several human malignancies [Citation46–48]. In addition to its PKR-regulatory activity, nc886 suppresses the microRNA pathway by binding to Dicer [Citation49]. In the present study, nc886 was hypomethylated in blood cells of MDA cases. In contrast, it was hypermethylated in pre-diagnostic blood samples obtained from the cancer patients in the WHI study and in another previously reported prospective study (18). Furthermore, the nc886 methylation status was associated with the level of transcription of EIF2AK2, and we identified a forward causal relationship of nc886 region methylation with PanC using summary statistics from a large public study (PanC4 Consortium and AIRES mQTL database). These observations support potential value of nc886 methylation as a susceptibility marker for PanC. Reduced expression of nc886 induced by gene hypermethylation could lead to overexpression of EIF2AK2, which in turn can contribute to tumour development. However, the hypomethylated nc886 in MDA cases might suggest a reverse causality phenomenon. To investigate whether there is any causal relationship of nc886 methylation with PanC, we conducted MR test using SNP data. We identified cg00308130 (p = 0.034) and cg16684184 (p = 0.004) in nc886 with forward causal relationship with PanC. The contradictory observations on nc886 methylation status in pre – and after diagnostic blood samples may have different implications regarding the role of the gene on aetiology vs disease progression. Therefore, it is important to establish a susceptibility marker for detecting PanC using pre-diagnostic blood samples in a prospective cohort study.
The other potential methylation markers for PanC identified in our 2-phase study were six DMPs (in or near AIM2, DGKA, STK39, and TNFSF8) and two DMRs (in or near LY6G5C and HLA-DPB1). Collectively, in the discovery and validation study of 109 MDA and 1700 FHS samples, we found significant correlations of transcription levels with DNA methylation for three genes (AIM2, DGKA, and TNFSF8) as well as one gene (STK39) with a marginally significant association with PanC. AIM2, an inflammasome receptor for DNA, is known to play an important role in the human innate immune response by activating inflammatory cascades [Citation50,Citation51]. AIM2 is also one of the most extensively validated and widely studied markers of peripheral inflammation found in blood [Citation52,Citation53]. It is a member of the IFI20X/IFI16 family that plays a putative role in tumorigenic reversion and may control cell proliferation. We observed hypomethylated AIM2 in blood cells from PanC patients, who have increased expression of AIM2 and elevated levels of C-reactive protein as demonstrated in other studies [Citation54–56]. Indeed, the FHS data demonstrated a significant negative association between AIM2 methylation and GE. In addition, the MR test suggested that methylation near AIM2 is robustly associated with PanC status. DGKA is a lipid kinase that regulates the lipid second messengers diacylglycerol and phosphatidic acid in the plasma membrane, which affects many important oncogenic proteins and pathways in cancer cells [Citation57]. Decreased methylation of DGKA has been associated with increased radiation-induced fibrosis [Citation58]. TNFSF8 is a cytokine with pleiotropic biologic activities. STK39 functions in the cellular stress response pathway. MR test identified cg16875057 (p = 0.050) in STK39, with forward causal relationship and cg15354065 (p = 0.012) in DGKA with reverse causal relationship and cg27661394 (p = 0.066) and cg10780778 (p = 0.051) of marginally downstream effect. Although some of our observations were not replicated in the WHI samples, the strong association of methylation and GE, the biological relevance of the genes in oncogenesis, and the supporting MR evidence suggest potential values of these markers in PanC susceptibility. Consistent with findings from two previous prospective studies s [Citation15,Citation19], our observations support a mechanistic link of genes regulating the immune response, inflammatory response, and lipid second messenger pathway and PanC.
One of the replicated DMRs on chromosome 6 had one or two cis-located genes (HLA-DPA1 and/or HLA-DPB1) where gene transcription levels were positively associated with methylation levels and negatively associated with PanC status. HLA-DPA1/B1 is a class II major histocompatibility complex that plays a central role in the immune system by presenting peptides derived from extracellular proteins to T-cell receptor. Polymorphic variations in the HLA gene regions have been associated with risk of several human cancer types [Citation59–63]. Our data support further investigation on the role of these genes in PanC susceptibility.
We further compared our discovered DMRs with those identified to be associated with PanC in a recently published study using pre-diagnostic leukocyte DNA methylation profiling [Citation19]. In the latter, no significant DMP was discovered. We found that among the 76 DMRs that were identified using the combined discovery and validation samples, there were 6 regions that had an overlap with our discovered DMRs in phase I with P value less than 0.05 (Supplementary Table S3). These overlapping DMRs include one on chromosome 17 near gene MFSD6L (hypomethylated in PanC cases), the DMR near RNF39 (hypermethylated in PanC cases), and one on chromosome 20 near gene BLCAP and NNAT (hypomethylated in PanC cases). Those corroborated findings suggest that DMRs are more robust and likely to be replicated than DMPs in EWAS and our multi-phase strategy leveraging a large number of external controls is powerful and effective.
This study has several strengths. One is the two-stage design of the EWAS to boost the statistical power while guarding against false-positive findings. Another is the inclusion of multiple data types, such as GE data obtained using RNA sequencing and SNP data, to further corroborate the association of DNA methylation with PanC, to assess the functional consequences of methylation changes, and to characterize the directional relationships among methylation in peripheral blood, GE, and pancreatic cancer risk. The major limitation of our study is the small sample size and thus limited statistical power in analysing a large number of markers. To overcome this limitation, we leveraged the publicly available FHS cohort study methylation data in our discovery phase. We also took advantage of the existing data from the prospective WHI cohort in the independent validation study. A limitation of leveraging public controls is potentially inflated type I error rate likely due to residual batch effects (Figure S3). However, our results were not affected by inflated P values because we ranked the CpG sites to identify the most differentially methylated ones and our strict replication standards using an independent MDA (phase 2) guarded against false-positive findings among the identified CpGs. Another limitation is that our analyses were based on DNA methylation and gene expression profiling in whole blood from pre – or post-diagnosis pancreatic cases and controls; however, it is yet unknown whether similar findings are applicable to the pancreas tissue due to the difficulty in obtaining DNA methylation and gene expression data from normal pancreas. It is noted that the TCGA study has only collected genomic data from pancreatic tumours and a few matched nearby normal tissues, rather than normal pancreas from healthy individuals [Citation12].
We examined the forward causal relationships of three CpGs out of the six replicated CpGs and two CpGs in nc886 based on our knowledge of cis-regulated mQTLs from the ARIES study [Citation39]. The advantage of using IVs from an independent external database over using the IVs assessed from the same cohort for the MR analysis is to prevent the winner’s curse [Citation43] which may result in bias away from the null. We assessed the reverse causal relationship of the six replicated CpGs with PanC based on the additive weighted genetic risk score from 27 SNPs [Citation8] after linkage disequilibrium-based pruning. Because the exposure variable, PanC status, was a binary variable that could easily violate the exclusion restriction assumption, we directly tested for an association between the genetic risk score and the outcome as suggested by previous literature [Citation41–43]. The advantage is that the hypothesis testing result is more conservative; the disadvantage is that we were only able to test whether there exists a causal effect without estimate of the magnitude and direction.
In conclusion, our findings suggest that changes in DNA methylation in whole blood are likely to influence the susceptibility to PanC. Information on DNA methylation may provide insight into the complex interplay among genetic, epigenetic, and environmental factors underlying the risk of PanC, leading to better preventive and therapeutic interventions for this disease.
Supplemental Material
Download Zip (975.7 KB)Acknowledgments
This research was supported by National Institutes of Health (NIH) grant R01CA169122 and the University Cancer Foundation via the Institutional Research Grant program at the University of Texas MD Anderson Cancer Center. P.W. was partially supported by NIH grants R01HL116720, P30CA016672 and P50CA217674. J.S. was supported by Cancer Prevention and Research Institute of Texas (CPRIT) Core Facility Support Award RP170002. We would like to thank Mr. Donald Norwood from the Research Medical Library at MD Anderson Cancer Center for editorial assistance. The authors acknowledge the Texas Advanced Computing Center at The University of Texas at Austin for providing computing resources. The FHS is conducted and supported by the National Heart, Lung, and Blood Institute (NHLBI) in collaboration with Boston University (contract numbers N01-HC-25195, HHSN268201500001I, and 75N92019D00031). This manuscript was not prepared in collaboration with investigators in the FHS and does not necessarily reflect the opinions or views of the FHS, Boston University, or the NHLBI. The WHI program is funded by the NHLBI, NIH, and U.S. Department of Health and Human Services through contract numbers N01WH22110, 24152, 32100-2, 32105-6, 32108-9, 32111-13, 32115, 32118-32119, 32122, 42107-26, 42129-32, and 44221. This manuscript was not prepared in collaboration with investigators of the WHI, has not been reviewed and/or approved by the WHI, and does not necessarily reflect the opinions of the WHI investigators or the NHLBI.
Data availability statement
The data sets used for the analyses described in this manuscript were obtained from dbGaP at https://www.ncbi.nlm.n ih.gov/gap/through accession numbers phs000007, phs000200 and phs000648.
Disclosure statement
No potential conflicts of interest were disclosed.
Supplementary material
Supplemental data for this article can be accessed here
Additional information
Funding
References
- Henley SJ, Ward, EM, Scott, S, et al. Annual report to the nation on the status of cancer, part I: National cancer statistics. Cancer. 2020;126(10):2225–2249.
- Amundadottir L, Kraft, P, Stolzenberg-Solomon, RZ, et al. Genome-wide association study identifies variants in the ABO locus associated with susceptibility to pancreatic cancer. Nat Genet. 2009;41(9):986–990.
- Petersen GM, Amundadottir, L, Fuchs, CS, et al. A genome-wide association study identifies pancreatic cancer susceptibility loci on chromosomes 13q22.1, 1q32.1 and 5p15.33. Nat Genet. 2010;42(3):224–228.
- Wolpin BM, Rizzato, C, Kraft, P, et al. Genome-wide association study identifies multiple susceptibility loci for pancreatic cancer. Nat Genet. 2014;46(9):994–1000.
- Tang H, Wei, P, Duell, EJ, et al. Axonal guidance signaling pathway interacting with smoking in modifying the risk of pancreatic cancer: a gene- and pathway-based interaction analysis of GWAS data. Carcinogenesis. 2014;35(5):1039–1045.
- Tang H, Wei, P, Duell, EJ, et al. Genes-environment interactions in obesity- and diabetes-associated pancreatic cancer: a GWAS data analysis. Cancer Epidemiol Biomarkers Prev. 2014;23(1):98–106.
- Childs EJ, Mocci, E, Campa, D, et al. Common variation at 2p13.3, 3q29, 7p13 and 17q25.1 associated with susceptibility to pancreatic cancer. Nat Genet. 2015;47(8):911–916.
- Klein AP, Wolpin , BM, Risch, HA, et al. Genome-wide meta-analysis identifies five new susceptibility loci for pancreatic cancer. Nat Commun. 2018;9(1):556.
- Tang HW, Jiang, L, Stolzenberg-Solomon, RZ, et al. Genome-wide gene-diabetes and gene-obesity interaction scan in 8,255 cases and 11,900 controls from panscan and PanC4 consortia. Cancer Epidemiol Biomarkers Prev. 2020;29(9):1784–1791.
- van Dongen J, Nivard, MG, Willemsen, G, et al. Genetic and environmental influences interact with age and sex in shaping the human methylome. Nat Commun. 2016;7:11115.
- van Kempen PMW, Noorlag, R, Braunius, WW, et al. Differences in methylation profiles between HPV-positive and HPV-negative oropharynx squamous cell carcinoma: a systematic review. Epigenetics. 2014;9(2):194–203.
- Bailey P, Chang, DK, Nones, K, et al. Genomic analyses identify molecular subtypes of pancreatic cancer. Nature. 2016;531(7592):47–52.
- Wang Z, Wei P. IMIX: a multivariate mixture model approach to association analysis through multi-omics data integration. Bioinformatics. 2021;36(22–23):5439–5447.
- Pedersen KS, Bamlet, WR, Oberg, AL, et al. Leukocyte DNA methylation signature differentiates pancreatic cancer patients from healthy controls. PLoS ONE. 2011;6(3):e18223.
- Michaud DS, Ruan, M, Koestler, DC, et al. DNA methylation-derived immune cell profiles, CpG markers of inflammation, and pancreatic cancer risk. Cancer Epidemiol Biomarkers Prev. 2020;29(8):1577–1585.
- Heiss JA, Brenner H. Epigenome-wide discovery and evaluation of leukocyte DNA methylation markers for the detection of colorectal cancer in a screening setting. Clin Epigenetics. 2017;9:24.
- van Veldhoven K, Polidoro, S, Baglietto, L, et al. Epigenome-wide association study reveals decreased average methylation levels years before breast cancer diagnosis. Clin Epigenetics. 2015;7:67.
- Battram T, Richmond, RC, Baglietto, L, et al. Appraising the causal relevance of DNA methylation for risk of lung cancer. Int J Epidemiol. 2019;48(5):1493–1504.
- Michaud DS, Ruan , M, Koestler, DC, et al. Epigenome-wide association study using prediagnostic bloods identifies new genomic regions associated with pancreatic cancer risk. JNCI Cancer Spectr. 2020;4(5): kaa041.
- Richard MA, Huan, T, Ligthart, S, et al. DNA methylation analysis identifies loci for blood pressure regulation. Am J Hum Genet. 2017;101(6):888–902.
- Mendelson MM, Marioni , RE, Joehanes, R, et al. Association of body mass index with DNA methylation and gene expression in blood cells and relations to cardiometabolic disease: a mendelian randomization approach. PLoS Med. 2017;14(1):e1002215.
- Hassan MM, Bondy , ML, Wolff, RA, et al. Risk factors for pancreatic cancer: case-control study. Am J Gastroenterol. 2007;102(12):2696–2707.
- Li D, Morris, JS, Liu, J, et al. Body mass index and risk, age of onset, and survival in patients with pancreatic cancer. JAMA. 2009;301(24):2553–2562.
- Aryee MJ, Jaffe, AE, Corrada-Bravo, H, et al. Minfi: a flexible and comprehensive Bioconductor package for the analysis of Infinium DNA methylation microarrays. Bioinformatics. 2014;30(10):1363–1369.
- Fortin JP, Labbe, A, Lemire, M, et al. Functional normalization of 450k methylation array data improves replication in large cancer studies. Genome Biol. 2014;15(12):503.
- Fortin JP, Triche TJ Jr., Hansen KD. Preprocessing, normalization and integration of the Illumina HumanMethylationEPIC array with minfi. Bioinformatics. 2017;33(4):558–560.
- Chen YA, Lemire , M, Choufani, S, et al. Discovery of cross-reactive probes and polymorphic CpGs in the Illumina Infinium HumanMethylation450 microarray. Epigenetics. 2013;8(2):203–209.
- Maksimovic J, Phipson B, Oshlack A. A cross-package Bioconductor workflow for analysing methylation array data. F1000Res. 2016;5:1281.
- Leek JT, Parker, HS, Fertig, EJ, et al. sva: Surrogate variable analysis. R package; 2019. https://bioconductor.org/packages/release/bioc/html/sva.html.
- Ritchie ME, Phipson, B, Wu, D, et al. limma powers differential expression analyses for RNA-sequencing and microarray studies. Nucleic Acids Res. 2015;43(7):e47.
- R Development Core Team. R: a language and environment for statistical computing. Vienna Austria: R Foundation for Statistical Computing; 2014.
- Jaffe AE, Murakam, P, Lee, H, et al. Bump hunting to identify differentially methylated regions in epigenetic epidemiology studies. Int J Epidemiol. 2012;41(1):200–209.
- Zemach A, McDaniel , IE, Silva, P, et al. Genome-wide evolutionary analysis of eukaryotic DNA methylation. Science. 2010;328(5980):916–919.
- Hansen KD. A manifest package for Illumina’s EPIC methylation arrays. R package; 2016. https://bitbucket.com/kasperdanielhansen/Illumina_EPIC.
- Hansen KD. IlluminaHumanMethylationEPICanno.ilm10b2.hg19: Annotation for Illumina’s EPIC methylation arrays. R package; 2016. https://bitbucket.com/kasperdanielhansen/Illumina_EPIC.
- Joehanes R, Johnson , AD, Barb, JJ, et al. Gene expression analysis of whole blood, peripheral blood mononuclear cells, and lymphoblastoid cell lines from the framingham heart study. Physiol Genomics. 2012;44(1):59–75.
- Lin H, Yin, X, Xie, Z, et al. Methylome-wide association study of atrial fibrillation in framingham heart study. Vol. 7. Scientific reports; 2017. p. 40377-40377.
- Burgess S, Butterworth A, Thompson SG. Mendelian randomization analysis with multiple genetic variants using summarized data. Genet Epidemiol. 2013;37(7):658–665.
- Gaunt TR, Butterworth , A, Thompson, SG, et al. Systematic identification of genetic influences on methylation across the human life course. Genome Biol. 2016;17:61.
- Greco MF, Minelli , C, Sheehan, NA, et al. Detecting pleiotropy in Mendelian randomisation studies with summary data and a continuous outcome. Stat Med. 2015;34(21):2926–2940.
- VanderWeele TJ, Tchetgen, EJ, Cornelis, M, Kraft, P, et al. Methodological challenges in mendelian randomization. Epidemiology. 2014;25(3):427–435.
- Katan MB. Apolipoprotein E isoforms, serum cholesterol, and cancer. 1986. Int J Epidemiol. 2004;33(1):9.
- Burgess S, Labrecque JA. Mendelian randomization with a binary exposure variable: interpretation and presentation of causal estimates. Eur J Epidemiol. 2018;33(10):947–952.
- Tserel L, Kolde , R, Limbach, M, et al. Age-related profiling of DNA methylation in CD8+ T cells reveals changes in immune response and transcriptional regulator genes. Sci Rep. 2015;5:13107.
- Lee K, Kunkeaw , N, Jeon, SHH, et al. Precursor miR-886, a novel noncoding RNA repressed in cancer, associates with PKR and modulates its activity. Rna. 2011;17(6):1076–1089.
- Lee YS. A novel type of non-coding RNA, nc886, implicated in tumor sensing and suppression. Genomics Inform. 2015;13(2):26–30.
- Kunkeaw N, Jeon, SH, Lee, K, et al. Cell death/proliferation roles for nc886, a non-coding RNA, in the protein kinase R pathway in cholangiocarcinoma. Oncogene. 2013;32(32):3722–3731.
- Fort RS, Matho, C, Geraldo, MV, et al. Nc886 is epigenetically repressed in prostate cancer and acts as a tumor suppressor through the inhibition of cell growth. BMC Cancer. 2018;18(1):127.
- Ahn JH, Lee, HS, Lee, JS, et al. nc886 is induced by TGF-beta and suppresses the microRNA pathway in ovarian cancer. Nat Commun. 2018;9(1):1166.
- Martinon F, Tschopp J. Inflammatory caspases and inflammasomes: master switches of inflammation. Cell Death Differ. 2007;14(1):10–22.
- Hornung V, Ablasser , A, Charrel-Dennis, M, et al. AIM2 recognizes cytosolic dsDNA and forms a caspase-1-activating inflammasome with ASC. Nature. 2009;458(7237):514–518.
- Miller MW, Maniates, H, Wolf, EJ, et al. CRP polymorphisms and DNA methylation of the AIM2 gene influence associations between trauma exposure, PTSD, and C-reactive protein. Vol. 67. Brain Behav Immun; 2018. p. 194–202.
- Ligthart S, Marzi , C, Aslibekyan, S, et al. DNA methylation signatures of chronic low-grade inflammation are associated with complex diseases. Genome Biol. 2016;17(1):255.
- Sharma BR, Karki R, Kanneganti T-D. Role of AIM2 inflammasome in inflammatory diseases, cancer and infection. Eur J Immunol. 2019;49(11):1998–2011.
- Sollie S, Michaud , DS, Sarker, D, et al. Chronic inflammation markers are associated with risk of pancreatic cancer in the Swedish AMORIS cohort study. BMC Cancer. 2019;19(1):858.
- Szkandera J, Stotz , M, Absenger, G, et al. Validation of C-reactive protein levels as a prognostic indicator for survival in a large cohort of pancreatic cancer patients. Br J Cancer. 2014;110(1):183–188.
- Purow B. Molecular pathways: Targeting diacylglycerol kinase alpha in cancer. Clin Cancer Res. 2015;21(22):5008–5012.
- Weigel C, Veldwijk , M, Oakes, C, et al. Epigenetic regulation of diacylglycerol kinase alpha promotes radiation-induced fibrosis. Nat Commun. 2016;7:10893.
- Jia M, Han, J, Hang, D, et al. HLA-DP is the cervical cancer susceptibility loci among women infected by high-risk human papillomavirus: potential implication for triage of human papillomavirus-positive women. Tumour Biol. 2016;37(6):8019–8025.
- Zhang Q, Yin, J, Zhang, Y, et al. HLA-DP polymorphisms affect the outcomes of chronic hepatitis B virus infections, possibly through interacting with viral mutations. J Virol. 2013;87(22):12176–12186.
- Lan Q, Hsiung , CA, Matsuo, K, et al. Genome-wide association analysis identifies new lung cancer susceptibility loci in never-smoking women in Asia. Nat Genet. 2012;44(12):1330–1335.
- Michailidou K, Lindstrom , S, Dennis, J, et al. Association analysis identifies 65 new breast cancer risk loci. Nature. 2017;551(7678):92–94.
- Shiraishi K, Okada , Y, Takahashi, A, et al. Association of variations in HLA class II and other loci with susceptibility to EGFR-mutated lung adenocarcinoma. Nat Commun. 2016;7:12451.