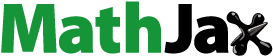
ABSTRACT
The decision to engage in lung cancer screening (LCS) necessitates weighing benefits versus harms. Previously, clinicians in the United States have used the PLCOM2012 algorithm to guide LCS decision-making. However, that formula contains race and gender-based variables. Previously, using data from a European study, Bojesen and colleagues have suggested that cg05575921 methylation could guide decision-making. To test this hypothesis in a more diverse American population, we examined DNA and clinical data from 3081 subjects from the National Lung Screening Trial (NLST) study. Using survival analysis, we found a simple linear predictor consisting of age, pack-year consumption and cg05575921, to have the best predictive power among several alternatives (AUC = 0.66). Results showed that the highest quartile of risk was more than 2-fold more likely to develop lung cancer than those in the lowest quartile. Race, ethnicity, and gender had no effect on prediction with both cg05575921 and pack years contributing equally (both p < 0.003) to risk prediction. Current smokers had considerably lower methylation than former smokers (46% vs 67%; p < 0.001) with the average methylation of those who quit approaching 80% after 25 years of cessation. Finally, current male smokers had lower mean cg05575921 percentage than female smokers (46% vs 49%; p < 0.001). We conclude that cg05575921 (along with age and pack years) can be used to guide LCS decision-making, and additional studies might focus on how best to use methylation to inform decision-making.
Introduction
Lung cancer is the leading type of cancer death in the United States [Citation1]. The overall 5-year survival for patients diagnosed with lung cancer is only 20%, largely because nearly 60% of cancers are diagnosed at a late stage [Citation2]. In contrast, when diagnosed early, the 5-year survival for patients with lung cancer is around 56%. Hence, there are strong rationale developing methods for early diagnosis of lung cancer.
Because smoking accounts for 90% of lung cancer mortality, screening efforts have targeted heavy smokers [Citation3]. Clinical trials have demonstrated that screening heavy smokers with low-dose CT (LDCT), though not chest radiographs or sputum cytology, can reduce lung cancer mortality [Citation4,Citation5]. The National Lung Screening Trial (NLST) enrolled 53,454 US smokers, adults ages 55 to 74 with a 30-pack-year (PY) smoking history who were either current smokers or had quit within the previous 15 years [Citation4]. The NSLT found that three rounds of screening with LDCT scan reduced lung cancer mortality by 16% after nearly 7 years of follow-up compared to screening with chest radiographs [Citation4,Citation5]. In 2020, the European NELSON trial investigators presented data at the World Conference on Lung Cancer showing that LDCT screening reduced lung cancer mortality by 24% for men and 33% for women over a 10-year follow-up [Citation6].
Recognizing the value of LDCT screening, the United States Preventative Services Task Force (USPSTF) has recently updated their prior guidance to recommend annual screening for all patients between the ages of 50 to 80 with at least 20 PY history of smoking and either currently smoking or within 15 years of having quit smoking.” [Citation7] Critically, in both their current and prior (2014) guidelines, the USPSTF recommended a counselling visit prior to screening because the screening decision is considered very complex, requiring patients to balance an absolute survival benefit of about three in 1,000 against a number of potential harms [Citation7,Citation8]. According to the USPSTF, these harms include ‘false-positive results leading to unnecessary tests and invasive procedures, overdiagnosis, radiation-induced cancer, incidental findings, and increases in distress or anxiety.’ [Citation7] These potential harms are not imaginary. The overall false-positive rate for LDCT was 23.3%, and in three rounds of screening nearly 40% of NLST subjects had at least one abnormal scan with 96% of these abnormal scans having false positives [Citation5,Citation9]. This high false-positive rate can have additional adverse consequences because most abnormal scans are followed by further imaging studies. Based on data from an Italian COSMOS cohort, a modelling study estimated one radiation-induced lung cancer death for every 2500 people who were screened over a period of 10–20 years [Citation10].
Morbidity and mortality from unnecessary diagnostic testing are also a major concern. In the NLST, 2.7% of the subjects with a false-positive test result underwent invasive diagnostic testing. Trial sites, which were predominantly academic medical centres, performed these diagnostic procedures with a high degree of safety. Still, nearly 10% resulted in major complications [Citation11]. Sadly, this clinical trial experience underestimated the real-world clinical outcomes of screening. A 2019 study by Shih and colleagues of a nationally representative sample of 344,510 patients showed that the actual rate of complications was 23% and median costs of minor, intermediate and major complications was $5573, $19,470 and $57,893, respectively [Citation12].
Other risks of screening are harder to quantify. But the apprehension of those contemplating screening and the fear of lung cancer are very real. The suicide rate of lung cancer patients is 4 times that of the general population risk and is significantly higher than those with breast, colon or prostate cancer [Citation13].
In order to better inform patients and clinicians, investigators have developed a number of predictive algorithms for guiding LDCT decision-making. Unfortunately, these metrics do not perform well and often rely on potentially biased ethnicity and gender variables. As a result, in 2021, the USPSTF called for ‘research to identify biomarkers that can accurately identify persons at high risk.’
Fortunately, advances in epigenetics may have already identified that new biomarker. In 2012, our group discovered that methylation status at cg05575921, a cytosine-guanine (CpG) site in the aryl hydrocarbon receptor repressor (AHRR), as being highly predictive of smoking status [Citation14]. Since that time, over 100 studies have confirmed those findings, and further shown that it is associated with lung cancer, can be used to assess the number of cigarettes smoked and guide smoking cessation therapy [Citation15–19]. Most critically to the current study, in 2017, using data from the Copenhagen City Study, Bojesen and colleagues showed that cg05575921 methylation predicted which smokers would benefit from LDCT screening[Citation20]. Recently, they have refined those prior findings and shown that ‘adding cg05575921 methylation on top of current eligibility criteria for lung cancer screening improves the specificity of lung cancer screening’ [Citation21].
In this study, we examine the relationship of cg05575921 methylation to risk for lung cancer and test whether its inclusion into risk estimation algorithms can improve the prediction of lung cancer in NLST subjects.
Methods
The clinical data and biomaterial in this study are from the National Lung Screening Trial (NLST). The NLST is a National Cancer Institute (NCI) sponsored trial that was jointly conducted by 33 Lung Cancer Screening Centers and the American College of Radiology Imaging Network (ACRIN). A full description of the study design, rationale and methods is available elsewhere [Citation22,Citation23].
For this study, we analysed the DNA and clinical materials from 3081. The clinical data were provided by the NCI Cancer Data Access System (CDAS). The DNA material was provided by the Eastern Cooperative Oncology Group-American College of Radiology Imaging Network (ECOG-ACRIN; https://ecog-acrin.org/). The procedures carried out in this study were approved by the NCI National Clinical Trials Network Core Correlative Sciences Committee.
Determinations of cg05575921 methylation were conducted by personnel blinded to case status. In brief, 1 µg of DNA was shipped from the ECOG-ACRIN repository at MD Anderson Cancer Center in 12 separate shipments. After receipt, the DNA was plated into 96 well plates containing at least one blank and one methylation sensitive DNA control. The DNA was then bisulphite converted using an Epitect Fast 96 DNA Bisulphite Conversion kit (Qiagen, Germantown MD) used according to manufacturer’s direction.
After elution, cg05575921 methylation was determined using the Smoke Signature assay from Behavioural Diagnostics (Coralville IA) using our previously described methods [Citation24,Citation25]. In brief, a 3 µl aliquot of each bisulphite-converted sample from above was pre-amplified using a proprietary 2X preamplification mixture, diluted 1:3000 with molecular-grade water, and partitioned into ~1.5 nl droplets using an automated droplet generator. DNA amplicons contained within these droplets were then PCR amplified using proprietary primer probe sets from Behavioural Diagnostics (Coralville, IA) and universal digital PCR reagents from Bio-Rad (Carlsbad, CA). The number of droplets containing amplicons with at least one ‘C’ allele (methylated CpG residue), one ‘T’ allele (unmethylated CpG residue) or neither allele was then determined using Bio-Rad QX-200 droplet reader and proprietary Quantisoft software. Percent methylation and 95% confidence interval (CI) estimates of the mean for each sample were calculated using the software by fitting the observed ratios to a Poisson distribution. Samples whose 95% exceeded 3% were excluded from the study. Average intraplate and interplate values of the methylation sensitive DNA standard were 31.0 ± 1.6% and 31.5 ± 1.4%, respectively.
Data Analysis
Analysis of the time from randomization to the event of lung cancer (screen or non-screen detected) was carried out using the Royston-Parmar survival model, where
is the cumulative hazard,
is the baseline cumulative hazard,
is age at screening, and
is a covariate, possibly a dummy variable (e.g., gender) [Citation26]. Time (t) is years from randomization, and age is separated from the other covariates in the formula because it was included in all models to account for left-truncation (different ages at study entry) [Citation27]. A key feature of the model is that the log baseline cumulative hazard is a function of cubic splines,
, with K knots and weights
. Maximum likelihood was used for parameter estimation, and model selection for both
and
was based on the Akaike Information Criteria (AIC) using the difference in AIC (ΔAIC) and AIC weights (AICwt) [Citation28]. The covariates were cg05575921 (Cg055), PY, smoking status at screening (Status, 0 = former, 1 = current), and the set of demographic variables, gender, ethnicity, race, and education. The demographic variables were categorical (see ), and dummy coding was used to represent their effects. Fifteen models were estimated: four models of the single effects (e.g., Cg055 alone), six models including two effects (e.g., Cg055 and PY), four models including three effects, and the full model (Cg055, PY, Status, demographic dummy variables). Kaplan–Meier plots were used to assess consistency with the proportional hazards assumption, which was deemed to be acceptable but not perfect. In addition, there was reasonable agreement between the Kaplan–Meier cumulative hazard and the spline-based approximation. The R package flexsurv was used for the survival analysis [Citation29]. Predictive performance was estimated with the time-dependent area under the Receiver Operating Characteristic curve (AUC) and the Brier score using bootstrap cross-validation as implemented in the riskRegression package [Citation30]. Additional analysis examined the relationship of cg05575921 with other variables. Generalized additive models (GAM) (or regularized spline models) were used to account for possible non-linear trends using mgcv[Citation31]. An approximate F-test was computed to test the null hypothesis that the trend line was flat (i.e., a horizontal line), along with an adjusted R2 statistic and the percentage of deviance explained. Finally, means and proportions for demographic comparisons of continuous and categorical data were performed with ANOVA F-tests and chi-squared tests, respectively [Citation32].
Table 1. Clinical and demographic characteristics of NLST subjects by gender; count or mean (SD).
Results
The clinical and demographic data for the 3081 subjects for whom reliable cg05575921 and survival time data were obtained are given in . These data include all of the parameters used by Tammemagi and colleagues in their PLCOM2012 prediction formula [Citation33]. Overall, the sample was 59% male with both males and females subjects having an average age of just over 61 years of age. The subjects were largely White with only 4% of the subjects being of African American Ancestry. The average level of education was relatively low with only 33% of the subjects having completed a Bachelor’s degree or higher. The vast majority of male subjects (74%) reported being married or living as married. In contrast, only 53% of female subjects reported having a similar status (X2(1) = 138.7, p < .001). On average, the subjects were modestly overweight with both males and females having an average BMI of approximately 28. Women reported a personal cancer history more than five times more frequently than men (6.5% vs 1.3%; X2(1) = 50.5, p < .001). However, the rates of cancer in their immediate families were similar (males, 23%, vs females, 24%; X2(1) = .5, p = .467). Finally, only 7% of these relatively heavily smoking subjects reported a personal history of COPD (7% for males and 8% for females, X2(1) = 2.9, p = .09) [Citation34].
The middle of contains a summary of the key variables used in our biomarker analyses. Cg05575921 mean percentage methylation in current male smokers was lower than methylation in current female smokers (46.1% ± 14.1 vs 49.3% ± 13.7; F(1, 1492) = 20.4, p < .001). Similarly, despite having similar periods of self-reported quitting (7.5 ± 5.1 yrs vs 6.9 ± 4.7 yrs) males who reported quitting smoking prior to evaluation also had significantly lower mean cg05575921 methylation than females (65.6% ± 12.6 vs 69.2% ± 11.0; F(1, 1585) = 34.2, p < .001). Female subjects also had lower current daily cigarette consumption (29.1 ± 11.3 vs 26.5 ± 9.8 cigs/day; F(1, 3079) = 44.0, p < .001); and pack-year history (58.5 ± 24.5 PY vs 51.6 ± 19.8 PY; F(1, 3079) = 68.0, p < .001) than male subjects.
Finally, the bottom summarizes the number of the key lung cancer-related outcome variables. Overall, females had a similar rate of lung cancer during the three annual LDCT exams and the follow-up period as male subjects (4.6% for males and 4.1% for females, X2(1) = .2, p = .622).
Because the smoking induced cg05575921 demethylation regresses as a function of smoking cessation, the relationship between cg05575921 methylation with current cigarette and pack-year consumption histories was determined for current smokers only (see ). In these currently smoking subjects, the relationship between current daily cigarette consumption and cg05575921 levels was slightly different from a flat line for males (GAM adj. R2 = .009, trend line test p = .012, and deviance explained = 1.162%), but not for females (GAM adj. R2 = .007, trend line test p = .084, and deviance explained = 1.140%). The relationship between cg05575921 methylation with pack year consumption history () was a bit stronger for male subjects (GAM adj. R2 = .039, trend line test p < .001, and deviance explained = 4.786%), and also for females (GAM adj. R2 = .017, trend line test p = .017, and deviance explained = 2.017%).
Figure 1. The relationship between cg05575921 methylation and smoking intensity (cigarettes per day) in currently smoking subjects with males at top (N = 840, trend p = 0.012, adj R-sq = 0.009, deviance explained = 1.162%) and females at bottom (N = 654, trend p = 0.084, adj R-sq = 0.007, deviance explained = 1.140%).
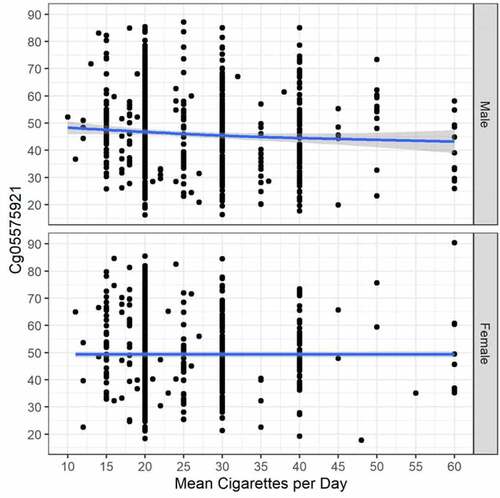
Figure 2. The relationship between cg05575921 methylation and pack year consumption in currently smoking subjects with males at top (N = 840, trend p < .001, adj R-sq = 0.039, deviance explained = 4.786%), and females at bottom (N = 654, trend p = 0.002, adj R-sq = 0.017, deviance explained = 2.017%).
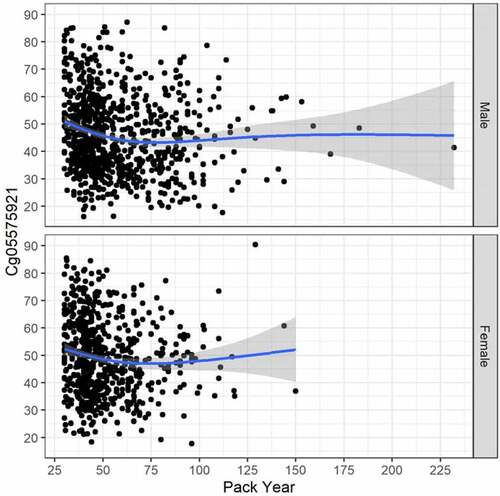
To better understand the reversion of cg05575921 methylation in those who quit smoking, we analysed the relationship of current cg05575921 methylation to years of smoking cessation in the 1535 subjects who reported that they quit smoking. As demonstrates, in these heavy smokers, average cg05575921 slowly increases as the time of cessation increases with gradually approaching 80% methylation after approximately 25 years (GAM adj. R2 = .164, trend line test p < .001, and deviance explained = 16.741%).
Modelling
The bottom of indicates that there were 135 subjects who developed cancer and 2946 censored subjects (4.4% events). Results of the survival model selection are shown in . As can be seen in the table, the best fitting model was Model 5 that had the predictors of age (which was in every model), cg05575921, and PY (AICwt = 0.613). The second-best model added smoking status (former/current) (AICwt = 0.303), and the third best model had only age and cg05575921 (AICwt = 0.029). Thus, the three best fitting models all had age and cg05575921 as predictors and together accounted for AICwt = .95. Parameter estimates for the best fitting Model 5 are shown in (the boundary and interior knots of log(year) used for the baseline cumulative hazard are shown in the table note). The gamma parameters are the weights of the cubic spline terms and the effects of the predictors are listed in the last three rows. The table indicates that as cg05575921 decreases the log cumulative hazard increases, whereas as age and PY increase the log cumulative hazard also increases. None of the predictor confidence intervals (L95% to U95%) cover 0, and age has the strongest effect (Z = 4.53), followed by PY (Z = 3.05) and Cg05575921 (Z = −2.99). AUC was computed for the quantiles of time and increased from a low of .567 (95% CI = [.357, .750]) at less than a year from randomization, to a high of .656 [.600, .709] at 5.4 years. Likewise, the Brier score increased over time, from a low of .004 [.002, .008] to a high of .038 [.031, .048], with all values being only slightly smaller than for the null model.
Table 2. Survival model selection results.
Table 3. Parameter estimates for best model.
shows the time-varying AUC (95% CIs) for the top five fitting models (in the order of AIC fit, Model 5, 11, 1, 2, 6; see ). The times were the quantiles of the event times (in years). The figure indicates that Model 2 (Age, PY) had the best short-term predictive power but diminished over time. In contrast, Model 5 (Age, Cg055, PY) had the greatest long-term predictive power (at 4.5 and 5.4 years). The CIs were overlapping for all estimates due to the small number of events (4.4%).
Figure 4. The time-varying AUC (95% CIs) for the top five fitting models (in order of AIC fit, Model 5, 11, 1, 2, 6; see ). The times were the quantiles of the event times (in years) .
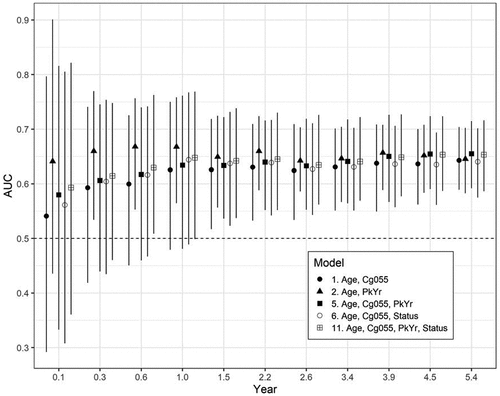
An illustration of the effects of cg05575921 and PY is shown in . The figure shows the cumulative hazard as a function of year on study, cg05575921 and PY. The 25th and 75th percentiles of the two variables are considered, and age is fixed at the sample mean value. The upper right-hand panel shows that the increase in risk is fastest for the combination of the larger PY (= 66) and smaller Cg05575921 (= 45%). In contrast, the risk increases at the slowest rate in the bottom left-hand panel for the smaller PY (= 40) and the larger cg05575921 (= 71%).
Figure 5. Cumulative hazard (95% confidence ribbons) as a function of year on study, pack years consumption (PY), and Cg05575921. Values for the latter two are set at the 25th and 75th percentiles, and age is set to the mean of the sample. Curves are estimated based on the best fitting survival model () .
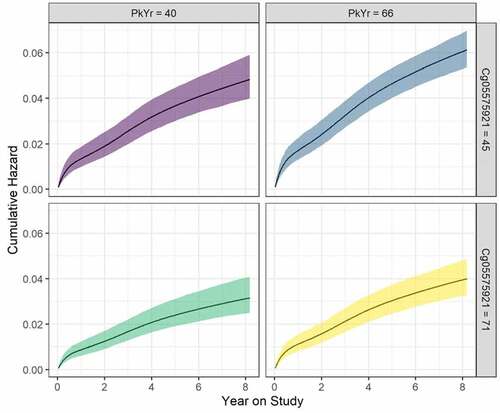
Discussion
In summary, we confirm prior findings that cg05575921 methylation can help to guide LDCT decision-making. A model with cg05575921 methylation, age, and tobacco pack-year consumption significantly predicted the risk of lung cancer during 8-year follow-up of 3081 National Lung Screening Trial participants in the low-dose CT screening arm. Current smokers and men had much lower methylation than former smokers and women, respectively. These results confirmed the predictive value of cg05575921 methylation for developing lung cancer, as shown in the Copenhagen City Heart Study. Danish investigators suggested that the biomarker could potentially identify persons most likely to benefit from lung cancer screening. We were able to demonstrate the discriminant power of cg05575921 methylation in a more diverse population of high-risk persons who were actually undergoing lung cancer screening.
While these findings are particularly promising, given the more recent findings by Copenhagen City Heart Study consortium (Jacobsen et al., 2022) [Citation21], there is still considerable work to be performed before these results can be fully transformed into a clinical test. First and foremost, the age range and the consumption range of the test should be expanded to address all of those thought to need screening by the USPSTF. Second, and perhaps more obviously given the well-publicized concerns with the prior algorithms [Citation35], special attention must be given to broadening the diversity of individuals examined to include African Americans, Asians and Native Americans.
Broadening the age range is an obvious next step given the recent recommendations by the USPSTF to offer LDCT testing to those as young as 50 years old and with as little as 20 PY of consumption. However, this recent set of recommendations is based on current testing technologies. If LDCT techniques become more sensitive or specific, or the cost of their administration decreases, it may be prudent to consider using LDCT screening in those who are younger or those with lower smoking consumption histories. Therefore, further expansion of the subject pool to include those who are younger than 50 or have smoked less than 20 PY may be advisable.
In contrast to the prior study by Jacobsen, our analyses include African American participants. Even so, the numbers of African Americans in this study are low with the numbers needed to fully compare the predictive capacity of the approach in Blacks versus Whites being considerable. Furthermore, the number of other underrepresented populations, such as Asians is even lower. Therefore, although the results are promising, any conclusions about the absence of significant racial effects must be considered tentative. Furthermore, although we have previously shown that the assay works equally well for African Americans in classifying smoking status [Citation18,Citation25], these studies do not address the question of relative risk for cancer. This is particularly relevant for African Americans. As a result of being targeted by the tobacco industry, African Americans have a higher rate of smoking menthol laden cigarettes [Citation36,Citation37]. If menthol or other related compounds themselves are major carcinogens, this could be an issue for this approach because menthol, for example, is metabolized by the CYP2A6 pathway, which is not regulated by AHRR [Citation38,Citation39].
The strength of the current approach is the use of reference free methylation sensitive dPCR (MSdPCR). Although qPCR is sufficient for most applications, it is well known that measurement error can arise from alterations in well-to-well amplification rate variation or from non-linearities when comparing the results to external standards [Citation40]. In contrast, MSdPCR is relatively immune to both of those issues and provides confidence intervals of the putative value [Citation24]. These improvements will provide more certainty to the laboratory assessments and may provide additional confidence to both clinicians and patients, thereby hastening clinical adoption.
We did not find a robust relationship between the number of cigarettes smoked per day and cg05575921 in the active smokers. This was expected. Previous studies have shown a plateauing of the dose response at cg05575921 after 15 cigarettes per day [Citation16,Citation18,Citation41]. In this study, there were only a handful of subjects in the NLST who were currently smoking ten or fewer cigarettes per day and, because one had to have 30 PY of smoking in order to qualify for the NLST study (i.e., at least 20 cigs per day times 30 years), it is very likely that these lower levels were changes from their prior patterns of consumption. Therefore, the current findings are very consistent with the prior findings and suggest that the pattern of reversion at cg05575921 to smoking cessation for heavy smokers is biphasic with large initial changes followed by a more gradual rate of remethylation [Citation16,Citation18,Citation41].
The differences in cg05575921 levels between actively smoking male and female subjects are of extreme interest. In theory, cg05575921 should be a good indicator of polyaromatic hydrocarbon (PAH) consumption. The mechanism through which this occurs is well understood. Reductions in cg05575921 methylation are associated with increases in AHRR transcription, and theoretically, production of the AHRR product [Citation14,Citation42–44]. The AHRR product then functions as a decoy receptor that competes with the aryl hydrocarbon receptor (AHR) for dimerization with the aryl hydrocarbon receptor translocator [Citation45,Citation46]. By controlling the numbers of AHR/ARNT heterodimers, cells control the activity of the xenobiotic pathway, which among other things metabolizes PAH, but not nicotine which is metabolized by a different set of cytochromes. Therefore, cg05575921 levels in peripheral WBC should be a good indicator of bloodstream PAH levels.
However, the current data supports the proposition that PAH levels in actively smoking male NLST subjects are higher than those in female subjects. This is consistent with findings by Chen and associates who have shown that levels of cotinine, a metabolite of nicotine that is not processed via the xenobiotic pathway, are also higher in male versus female smokers (313.5 ng/mL vs. 255.8 ng/ml) [Citation47]. Whereas it is true that in the NLST LDCT arm (n = 26,638), men (678) and women (638) had similar rates of cancer per 100,000 PY (men vs women; relative rate 1.06 (94–1.20) [Citation11]; per cigarette, female smokers inhale less PAH and nicotine than male smokers [Citation48]. Therefore, after adjustment for size, it appears that women may smoke less than men yet have the same rate of lung cancer as men. Further in-depth examination of this subject is in order.
These NLST data put our prior understanding of the relationship between smoking cessation and re-methylation of the cg05575921 locus on a firm foundation. This understanding will be crucial if DNA methylation is to be used like a ‘hemoglobin A1c’ for guiding smoking cessation [Citation17]. Unfortunately, these NLST data are not perfect with disadvantages including that time of cessation relies on patient recall and that the cessation was not biochemically confirmed. In addition, time is denoted only in integer values of years with no finer gradation for more recent cessation. Still, using a crude linear model, these data suggest that after about 25 years, cg05575921 methylation returns to normal levels (i.e., above 80%). This fits well with the data from Kato and associates [Citation16]. However, we know that the reversion of methylation is not linear with methylation of heavy smokers (starting methylation below 55%) increasing an average of 11% over the first 90 days of cotinine verified smoking cessation while those quitters with less intense smoking habits (starting methylation >55%) reverting only 5% over the first 90 days [Citation17]. Therefore, based on these and prior studies, we believe that it is likely that the initial reversion curve of methylation is steep with more gradual increases in methylation after 1 year of cessation as the values approach the population norm for non-smokers (86.6 ± 2.9%) [Citation18]. Studies to better refine this reversion curve could lead to improved contingency management-based methods for guiding smoking cessation therapy [Citation49].
Given the groundbreaking prior work by Jacobsen and colleagues from the Copenhagen City Consortium, we believe that further studies to better refine the risk for cancer using this type of approach in larger samples are needed. Other promising biomarkers, such as radiomic features extracted from LDCT data [Citation50–52], could be assistive in conjunction with cg05575921 methylation to improve lung cancer prediction performance in the future. Using radiomic feature extraction for risk prediction refinement is attractive as no additional sample collection, testing or cost is incurred to utilize this data captured in the LDCT screening scan. In addition, we believe that clinical trials to understand how to best employ these tests in clinical practice would be beneficial. In that regard, we note that recent findings suggest that after controlling for other factors, African Americans are significantly less likely to undertake LDCT screening than Whites [Citation53]. By deciphering the reasons for the decreased uptake of potentially lifesaving imaging in African Americans and other marginalized groups, we may be able to increase the rate of screening engagement for all individuals with the lessons learned being potentially applicable for optimizing the use of other preventative screening measures.
In summary, we confirm and extend prior findings from Jacobsen and colleagues by reporting that a simple metric consisting of age, cg05575921 and PY history predicts risk for lung cancer in the NLST population. We suggest that further studies to speed clinical translation, including optimizing cost-effectiveness and tools for communicating results to both patients and clinicians, are in order.
Disclosure statement
Dr. Philibert is the Chief Executive Officer of Behavioural Diagnostics and inventor on a number of granted and pending patent applications with respect to both tobacco consumption related to the material discussed herein. The use of cg05575921 status to determine smoking status is protected by US Patents 8,637,652 and 9,273,358.
Data availability statement
The genome-wide data included in this manuscript were prepared with funding from the United States National Institutes of Health (NIH). They are not yet publicly available.
Additional information
Funding
References
- Centers for Disease Control and Prevention, Centres for Disease Control. Annual Smoking-Attributable Mortality, Years of Potential Life Lost, and Productivity Losses— United States, 1997-2001. Morb Mortal Wkly. 1997–2001;54625–628:2005.
- Husmann L, Stolzmann P. Staging, restaging and response evaluation of non-small-cell lung cancer. In: Hodler J, von Schulthess GK, Kubik-Huch RA, editors. Diseases of the chest and heart 2015–2018: diagnostic imaging and interventional techniques. Milano: Springer Milan; 2015. p. 183–188.
- Alberg AJ, Brock MV, Ford JG. Epidemiology of lung cancer: diagnosis and management of lung cancer: American college of chest physicians evidence-based clinical practice guidelines. Chest. 2013;143(5 Suppl):e1S–e29S.
- The National Lung Screening Trial Research Team. Reduced lung-cancer mortality with low-dose computed tomographic screening. N Engl J Med. 2011;365(5):395–409.
- The National Lung Screening Trial Research Team. Results of initial low-dose computed tomographic screening for lung cancer. N Engl J Med. 2013;368(21):1980–1991.
- de Koning HJ, van der Aalst CM, de Jong PA. Reduced lung-cancer mortality with volume CT screening in a randomized trial. N Engl J Med. 2020;382(503–513):503–513.
- Krist AH. Screening for lung cancer: US preventive services task force recommendation statement. JAMA. 2021;325(10):962–970.
- Moyer VA. Screening for lung cancer: U.S. preventive services task force recommendation statement. Ann Intern Med. 2014;160(5):330–338.
- Aberle DR, Adams AM, Berg CD, National Lung Screening Trial Research Team. Reduced lung-cancer mortality with low-dose computed tomographic screening. N Engl J Med. 2011;365:395–409.
- Rampinelli C, De Marco P, Origgi D. Exposure to low dose computed tomography for lung cancer screening and risk of cancer: secondary analysis of trial data and risk-benefit analysis. BMJ. 2017;356. DOI:10.1136/bmj.j347.
- Pinsky PF, Gierada DS, Hocking W. National Lung Screening Trial findings by age: medicare-eligible versus under-65 population. Ann Intern Med. 2014;161(627–633):627.
- Huo J, Xu Y, and Sheu T. Complication rates and downstream medical costs associated with invasive diagnostic procedures for lung abnormalities in the community setting complications and medical costs associated with diagnostic procedures for lung abnormalities complications and medical costs associated with diagnostic procedures for lung abnormalities. JAMA Internal Medicine. 2019;179(3): 324–332.
- Rahouma M, Kamel M, Abouarab A. Lung cancer patients have the highest malignancy-associated suicide rate in USA: a population-based analysis. Ecancermedicalscience. 2018;12. DOI:10.3332/ecancer.2018.859.
- Monick MM, Beach SRH, Plume J. Coordinated changes in AHRR methylation in lymphoblasts and pulmonary macrophages from smokers. Am J Med Genet B Neuropsychiatr Genet. 2012;159B(141–151):141–151.
- Gao X, Jia M, Zhang Y. DNA methylation changes of whole blood cells in response to active smoking exposure in adults: a systematic review of DNA methylation studies. Clin Epigenetics. 2015;7(113). DOI:10.1186/s13148-015-0148-3
- Takeuchi F, Takano, K, and Yamamoto, M, et al. Clinical implication of smoking-related aryl-hydrocarbon receptor repressor (AHRR) hypomethylation in Japanese adults. Circ J. 2022;86(6):986–992.
- Philibert R, Mills JA, Long JD. The reversion of cg05575921 methylation in smoking cessation: a potential tool for incentivizing healthy ageing. Genes (Basel). 2020;11(1415):1415.
- Dawes K, Andersen A, Reimer R. The relationship of smoking to cg05575921 methylation in blood and saliva DNA samples from several studies. Sci Rep. 2021;11(21627). DOI:10.1038/s41598-021-01088-7.
- Zhang Y, Elgizouli M, Schöttker B. Smoking-associated DNA methylation markers predict lung cancer incidence. Clin Epigenetics. 2016;8(127). DOI:10.1186/s13148-016-0292-4.
- Bojesen SE, Timpson N, Relton C. AHRR (cg05575921) hypomethylation marks smoking behaviour, morbidity and mortality. Thorax. 2017;72(7):646–653.
- Jacobsen KK, Schnohr P, Jensen GB. AHRR (cg05575921) Methylation Safely Improves Specificity of Lung Cancer Screening Eligibility Criteria: A Cohort Study. Cancer Epidemiol Prev Biomarkers. 2022;31(4):758–765.
- Hillman BJ. Economic, legal, and ethical rationales for the ACRIN national lung screening trial of CT screening for lung cancer. Acad Radiol. 2003;10(3):349–350.
- National Lung Screening Trial Research Team. The national lung screening trial: overview and study design. Radiology. 2011;258(1):243–253.
- Philibert R, Dogan M, Noel A. Dose response and prediction characteristics of a methylation sensitive digital PCR assay for cigarette consumption in adults. Front Genet. 2018;9. DOI:10.3389/fgene.2018.00137.
- Dawes K, Andersen A, Papworth E. Refinement of cg05575921 demethylation response in nascent smoking. Clin Epigenetics. 2020;12:1–11.
- Royston P, Parmar MK. Flexible parametric proportional‐hazards and proportional‐odds models for censored survival data, with application to prognostic modelling and estimation of treatment effects. Stat Med. 2002;21(15):2175–2197.
- Pencina MJ, Larson MG, D’Agostino RB. Choice of time scale and its effect on significance of predictors in longitudinal studies. Stat Med. 2007;26(1343–1359):1343–1359.
- Anderson DR. Model based inference in the life sciences: a primer on evidence. New York: Springer; 2008.
- Jackson CH. flexsurv: a Platform for Parametric Survival Modelling in R. J Stat Softw. 2016;70(8). DOI:10.18637/jss.v070.i08
- Lazic SE. Medical risk prediction models: with ties to machine learning. J R Stat Soc Ser A. 2022;185(1):425–425.
- Wood SN. Generalized additive models: an introduction with R. New York: Chapman and Hall/CRC; 2006.
- Fleiss JL. Statistical methods for rates and proportions. New York NY: John Wiley & Sons Inc; 1981.
- Tammemägi MC, Katki HA, Hocking WG. Selection criteria for lung-cancer screening. N Engl J Med. 2013;368(728–736):728–736.
- Wheaton AG, Liu Y, Croft JB. Chronic obstructive pulmonary disease and smoking status—United States, 2017. Morbidity Mortality Weekly Rep. 2019;68(24):533.
- Vyas DA, Eisenstein LG, and Jones DS. Hidden in plain sight — reconsidering the use of race correction in clinical algorithms. N Engl J Med. 2020;383(9):874–882.
- Caraballo RS, Asman K. Epidemiology of menthol cigarette use in the United States. Tob Induc Dis. 2011;9(S1):S1
- Gardiner PS. The African Americanization of menthol cigarette use in the United States. Nicotine Tob Res. 2004;6(1):S55–S65.
- Miyazawa M, Marumoto S, and Takahashi T. Metabolism of (+)- and (-)-menthols by CYP2A6 in human liver microsomes. J Oleo Sci. 2011;60(3):127–132.
- Jabba SV, and Jordt S-E. Risk analysis for the carcinogen pulegone in mint- and menthol-flavoured e-cigarettes and smokeless tobacco products. JAMA Intern Med. 2019;179(12):1721.
- Taylor SC, Nadeau K, Abbasi M. The ultimate qPCR experiment: producing publication quality, reproducible data the first time. Trends Biotechnol. 2019;37(7):761–774.
- Zhang Y, Florath I, Saum K-U. Self-reported smoking, serum cotinine, and blood DNA methylation. Environ Res. 2016;146:395–403.
- Zeilinger S, Kühnel B, Klopp N. Tobacco smoking leads to extensive genome-wide changes in DNA methylation. PLoS One. 2013;8(e63812):e63812.
- Shenker NS, Polidoro, S, and van Veldhoven, K. Epigenome-wide association study in the European prospective investigation into cancer and nutrition (EPIC-turin) identifies novel genetic loci associated with smoking. Hum Mol Genet. 2012;22(5): 843–851.
- Stueve TR, Li W-Q, Shi J. Epigenome-wide analysis of DNA methylation in lung tissue shows concordance with blood studies and identifies tobacco smoke-inducible enhancers. Hum Mol Genet. 2017;26(3014–3027):3014–3027.
- Philibert RA, Beach S, and Brody GH. The DNA methylation signature of smoking: an archetype for the identification of biomarkers for behavioural illness. Genes motiv use subst. 2014;61:109–127.
- Sakurai S, Shimizu T, Ohto U. The crystal structure of the AhRR/ARNT heterodimer reveals the structural basis of the repression of AhR-mediated transcription. J Biol Chem. 2017;292(17609–17,616):17609–17,616.
- Chen A, Krebs, N, and Zhu, J. Sex/gender differences in cotinine levels among daily smokers in the Pennsylvania adult smoking study. J Women’s Health. 2017;26(11):1222–1230.
- Melikian AA, Djordjevic M, Hosey J. Gender Differences Relative to Smoking Behaviour and Emissions of Toxins From Mainstream Cigarette Smoke. Nicotine Tob Res. 2007;9(3):377–387.
- Halpern SD, French B, and Small DS. Randomized trial of four financial-incentive programs for smoking cessation. NEJM. 2015;372(22):2108–2117.
- Uthoff J, Stephens MJ, and Newell JD. Machine learning approach for distinguishing malignant and benign lung nodules utilizing standardized perinodular parenchymal features from CT. Med Phys. 2019;46(7):3207–3216.
- Gillies RJ, and Schabath MB. Radiomics improves cancer screening and early detection. Cancer Epidemiol Biomarkers Prev. 2020;29(12):2556–2567.
- Khawaja A, Bartholmai BJ, and Rajagopalan S. Do we need to see to believe?-radiomics for lung nodule classification and lung cancer risk stratification. J Thorac Dis. 2020;12(6):3303–3316.
- Li J, Stults C, and Liang S-Y. Adherence to provider referrals for lung cancer screening with low dose computed tomography before and during the COVID-19 pandemic. Soc Res Nicotine Tob Res. S2–104 (Baltimore, MD). 2022;22.