ABSTRACT
Breast cancer is the most common cancer diagnosed in women and is often treated with chemotherapy. Although previous studies have demonstrated increasing biological age in patients who receive chemotherapy, evaluation of this association with DNA methylation-based markers of biological ageing may provide novel insight into the role of chemotherapy on the ageing process. We therefore sought to investigate the association between chemotherapy and markers of biological ageing as estimated from DNA methylation in women with breast cancer. DNA methylation profiling was performed on peripheral blood collected from 18 patients before and after the first cycle of chemotherapy using the Infinium HumanMethylation450 BeadChip. Six markers of biological age acceleration were estimated from DNA methylation levels. Multiple linear regression analyses were performed to evaluate the association between each metric of biological age acceleration and chemotherapy. After adjusting for chronological age and race, intrinsic epigenetic age acceleration (p = 0.041), extrinsic epigenetic age acceleration (p = 0.050), PhenoAge acceleration (p = 0.001), GrimAge acceleration (p < 0.001), and DunedinPACE (p = 0.006) were significantly higher and telomere length (p = 0.027) was significantly lower following the first cycle of chemotherapy compared to before treatment initiation. These results demonstrate greater biological ageing as estimated from DNA methylation following chemotherapy in women with breast cancer. Our findings illustrate that cytotoxic therapies may modulate the ageing process among breast cancer patients and may also have implications for age-related health conditions in cancer survivors.
Introduction
Breast cancer is the most common malignancy in adult women, accounting for approximately 10% of newly diagnosed cancer in women each year and is the second leading cause of malignancy-related deaths in women worldwide [Citation1]. While the incidence of breast cancer in many Westernized countries decreased in the early 2000’s following a decline in hormone replacement therapy, the incidence of breast cancer has been increasing by approximately 0.3% each year over the past two decades, likely contributing to increasing obesity and declining birth rates [Citation2]. Despite the continuing increase in breast cancer incidence, mortality from breast cancer has declined over the past three decades, which has partially been attributed to earlier detection of cancer through mammography and advancements in treatment [Citation3]. Patients who receive breast cancer treatment, however, experience a greater burden of age-related health conditions, and identifying factors associated with these conditions may aid in tertiary preventative strategies to reduce such treatment-related conditions [Citation4,Citation5]. Given the growing cancer survivor and ageing populations, examining patients who received breast cancer treatment may provide novel insights into the effect treatment has on the ageing process.
Increasing chronological age is one of the most significant risk factors for chronic disease and malignancy [Citation6]. Numerous markers of biological ageing have been identified, capturing different aspects of the ageing process [Citation7]. DNA methylation, one of the several epigenetic regulators, has previously demonstrated to capture age-related changes to the epigenome and correlates with both chronological age and age-related diseases [Citation8]. Additionally, the difference between chronological age and these markers of biological ageing is used to estimate epigenetic age acceleration, where positive values indicate accelerated ageing and vice versa. Several DNA methylation measures of biological age have been developed, including metrics by Horvath (intrinsic epigenetic age), Hannum (extrinsic epigenetic age), Levine (PhenoAge), Lu (GrimAge), Lu, (telomere length), and Belsky (DunedinPACE), and have been associated with numerous age-related health conditions [Citation9–14]. Additionally, these metrics of biological age have been associated with breast cancer, illustrating the utility of these biomarkers in breast cancer risk assessment and survival, and may have implications to serve as biomarkers during and after cancer treatment [Citation15–18].
Chemotherapy is a mainstay treatment for breast cancer. While effective in treating cancer, chemotherapy has been suggested to contribute to the ageing process and age-related health conditions [Citation19]. Moreover, aberrations in markers of ageing have been observed in women who received chemotherapy [Citation20,Citation21]. Specifically, anthracyclines (e.g., doxorubicin) and alkylating agents (e.g., cyclophosphamide) have been shown to induce epigenetic alterations, telomere attrition, stem cell exhaustion, and cellular senescence [Citation22]. Breast cancer patients treated with doxorubicin and cyclophosphamide were also observed to have an increase in p16INK4a, equating to an approximate 15-year increase in chronological age [Citation23]. Additionally, a previous study observed increased biological ageing among patients who underwent adjuvant radiation and chemotherapy, although the effect of chemotherapy on biological ageing independent of radiation remains limited [Citation24]. Further examination of the impact breast cancer treatment, both separately and in combination with other therapies, on biological ageing may aid in identifying less gerontogenic treatment regimens, and subsequently lessen age-related sequelae in breast cancer survivors. Therefore, the purpose of this study is to investigate the effects of chemotherapy on DNA methylation-based biological age among women with breast cancer.
Methods
Patient samples
The following analyses were conducted using a publicly available dataset, which has previously been published [Citation25]. Briefly, a total of 19 patients with breast cancer who underwent chemotherapy treatment at the Winthrop P. Rockefeller Cancer Institute at the University of Arkansas for Medical Sciences were enrolled. Study participants were female patients with stage I, II, or III and had either non-triple negative or triple negative breast cancer with no prior history of chemotherapy or radiotherapy. Patients were treated with a combination of doxorubicin (60 mg/m2) with cyclophosphamide (600 mg/m2) for 4 cycles every 2 weeks. Two blood samples were collected from each patient, one prior to the initiation of chemotherapy and one after the first cycle. Peripheral blood mononuclear cells were isolated, and DNA was extracted from these cells. Written and informed consent was obtained from all patients.
DNA methylation profiling
Patient blood samples underwent DNA methylation profiling using the Infinium HumanMethylation450 BeadChip. Raw intensity data were imported into the R package ChAMP and further processed using default settings [Citation26]. Beta-Mixture Quantile (BMIQ) normalization was applied to normalize the array data, and COMBAT was used to correct for batch effects, with resulting beta values made publicly available (37 samples available) [Citation27]. The R package ENmix was used to further perform quality control [Citation28]. Samples or CpGs with measurements >5% missing and extreme outliers (defined as less than the 25th percentile minus 3 × interquartile range (IQR) or greater than the 75th percentile plus 3 × IQR) were set to missing (4 samples).
Biological age calculation
Five principal component-based markers of biological ageing [Citation29] were estimated using DNA methylation levels: intrinsic epigenetic age acceleration (IEAA), extrinsic epigenetic age acceleration (EEAA), PhenoAge acceleration (PhenoAA), GrimAge acceleration (GrimAA), and telomere length attrition (TLA). IEAA was estimated from 353 CpG probes and has been associated with cell-intrinsic ageing [Citation9]. EEAA was estimated using 71 CpG probes and has been associated with immunological ageing [Citation10]. PhenoAA was estimated from 513 CpG probes and has been associated with clinical measures [Citation11]. GrimAA was estimated using 1,030 CpG probes and has been associated with lifespan [Citation12]. TLA was estimated using 140 CpG probes and has been associated with telomere length as measured by Southern blot [Citation13]. DunedinPACE (Pace of Ageing Calculated from the Epigenome) was estimated via the R package DunedinPACE using 173 CpG probes and has been associated with a longitudinal pace of ageing [Citation14]. Acceleration of the markers of biological ageing was estimated as the residual from a linear model of chronological age on each biological metric of ageing combining samples before and after chemotherapy.
Statistical analysis
To examine the association between chemotherapy (independent variable) and each metric of biological age (dependent variables), multiple linear regression was performed. To adjust for confounding, chronological age at blood sample collection and race were included as covariates in the regression models. Beta coefficients represent the mean change in biological ageing among the study sample post-chemotherapy treatment (intervention samples) compared to pre-chemotherapy treatment (control samples). As blood cell composition changes with age [Citation30], the Houseman method was used to estimate the proportion of CD4 T cells, CD8 T cells, natural killer cells, B cells, granulocytes, and monocytes for each sample and were included as covariates during regression analyses [Citation31]. To avoid model overfitting, monocyte proportion was excluded during analysis as this cell type was the least associated with chronological age. Interaction and stratified analyses were conducted by chronological age and race. Stochastic epigenetic mutations (SEMs), defined as DNA methylation levels that exceed 3 × IQR below the 25th percentile (hypomethylated SEMs) and 3 × IQR above the 75th percentile (hypermethylated SEMs), were estimated using SEMdetectR [Citation32] and multiple linear regression analyses were performed to investigate the association between chemotherapy treatment and hypomethylated, hypermethylated, and total SEMs. Principal component analysis (PCA) of the biological age metrics and covariates was conducted to examine whether the included variables discriminated between samples collected before and after the first cycle of chemotherapy and to assess the directionality of the effects of each variable relative to chemotherapy. Associations were considered significant if p ≤ 0.05. All statistical analyses were performed using SAS Studio (SAS Institute, Inc., Cary, NC, USA). PCA was conducted using the R package factoextra (v1.0.7) and graphing was performed using R (4.2.1) [Citation33].
Results
Sample characteristics
presents the descriptive characteristics for study participants by chemotherapy status. Overall, the average age of patients was 52.7 years with 77.8% of study participants identifying as White prior to treatment initiation. Additionally, study participants exhibited significantly increased epigenetic age acceleration for all ageing metrics after the first cycle of chemotherapy compared to before chemotherapy initiation, i.e., IEAA (p = 0.038), EEAA (p = 0.041), PhenoAA (p = 0.001), GrimAA (p < 0.001), and DunedinPACE (p = 0.007) were significantly higher and TLA (p = 0.024) was significantly lower, following the first cycle of chemotherapy. Zero correlation was observed for most of the markers of biological age acceleration and chronological age (Supplemental Figure S1).
Table 1. Study sample characteristics by chemotherapy.
Markers of biological ageing and chemotherapy
presents the multiple linear regression results for the association between the markers of biological ageing and chemotherapy. After adjusting for chronological age and race, chemotherapy treatment was significantly associated with IEAA (p = 0.041), EEAA (p = 0.050), PhenoAA (p = 0.001), GrimAA (p < 0.001), TLA (p = 0.027), and DunedinPACE (p = 0.006). Specifically, the first cycle of chemotherapy was associated with a 3.56-year [95% CI: 0.15, 6.97], 5.26-year [95% CI: 0.01, 10.51], 8.63-year [95% CI: 3.79, 13.47], and 4.68-year [95% CI: 2.27, 7.10] higher IEAA, EEAA, PhenoAA, and GrimAA compared to before chemotherapy initiation, respectively. Additionally, chemotherapy treatment was associated with a 0.17 [95% CI: −0.32, −0.02] lower telomere length and a 15% [95% CI: 0.05, 0.25] faster pace of biological age relative to chronological age via DunedinPACE. presents the pairwise correlation between the GrimAge surrogate biomarkers of blood plasma proteins and chemotherapy. Among the eight blood plasma protein surrogates, growth differentiation factor 15, tissue inhibitor metalloproteinase 1, and adrenomedullin were the biomarkers most correlated with chemotherapy, respectively. Adjustment for cell-type proportion yielded consistent findings as without adjustment except the association between chemotherapy and DunedinPACE was non-significant (Supplemental Table S1). Additionally, although no significant interactions were observed between chemotherapy and either chronological age or race, older patients exhibited greater biological ageing via IEAA, EEAA, and PhenoAA compared to younger patients, and White patients experienced greater biological ageing for all metrics compared to Black patients (Supplemental Tables S2–3). No significant associations were observed between chemotherapy treatment and hypomethylated, hypermethylated, and total SEMs (Supplemental Table S4).
Figure 1. Correlation estimates between the GrimAge surrogate biomarkers of blood plasma proteins and chemotherapy.
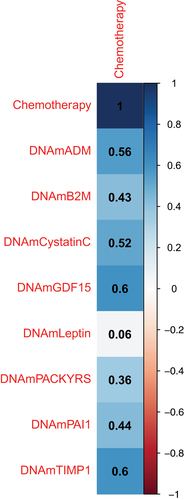
Table 2. Multiple linear regression results for the association between markers of biological ageing and chemotherapy.
presents the principal component analysis for the association between the markers of biological ageing and chemotherapy. The biological ageing metrics and covariates demonstrate a modest separation by chemotherapy status along the first principal component, with a majority of patients prior to chemotherapy initiation exhibiting negative values compared to positive values following the first cycle of chemotherapy (). Evaluation of the contribution of each variable demonstrates that markers of biological ageing exhibited consistent directionality towards participants following the first cycle of chemotherapy as exhibited by positive values along the first principal component, except for TLA which demonstrated directionality towards before chemotherapy initiation . Additionally, chronological age and race demonstrated orthogonal directionality relative to the biological age metrics. Assessment of the weighted cell types of EEAA demonstrated greater abundance of exhausted T cells and plasmablasts and lower abundance of naïve T cells following the first cycle of chemotherapy (Supplemental Figure S2).
Figure 2. Principal component analysis of the association between markers of biological ageing and chemotherapy.
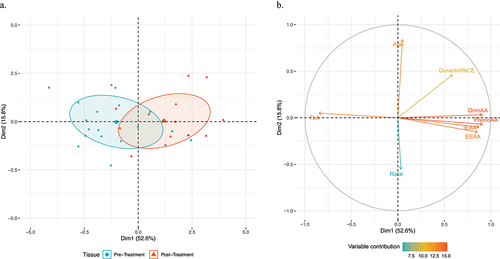
Discussion
In this study, we observed significant associations between biological ageing and chemotherapy among female participants with breast cancer. We identified IEAA, EEAA, PhenoAA, GrimAA, and DunedinPACE to be positively and TLA to be negatively associated with doxorubicin-cyclophosphamide chemotherapy. These results suggest doxorubicin-cyclophosphamide chemotherapy may induce age-related changes to the epigenome among females receiving treatment for breast cancer and may have implications for identifying therapies and strategies to aid in reducing biological ageing in breast cancer patients.
While epigenetic age acceleration has been associated with breast cancer risk, whether chemotherapy induces significant age-related changes to the epigenome remains understudied. In the current study, we observed doxorubicin-cyclophosphamide chemotherapy led to increased acceleration of all studied epigenetic age metrics. Our findings of greater ageing as assessed by EEAA, PhenoAA, and GrimAA following chemotherapy are consistent with a previous study that investigated adjuvant radiation and chemotherapy treatment and biological ageing [Citation24]. This study, however, compared women who did and did not receive chemotherapy who were also concomitantly receiving radiation treatment, limiting the ability to directly examine the effect of chemotherapy on the ageing metrics. Additionally, the majority of the findings presented in the study were significant among participants who only received radiation, suggesting radiation may contribute more to biological ageing than radiation and chemotherapy combined. Additionally, another study investigated the association between radiation, chemotherapy, and endocrine therapies and biological ageing metrics estimated years following treatment and observed associations with radiation but neither chemotherapy nor endocrine therapies, suggesting radiation treatment may similarly have age-related effects to the epigenome years after treatment [Citation34]. Our study adds to the current literature by further examining the effect of chemotherapy on biological ageing and demonstrates that chemotherapy acutely accelerates biological ageing as estimated from peripheral blood DNA methylation. By design, EEAA is positively correlated with exhausted CD8+ T cells and plasmablast cells and negatively correlated with naïve CD8+ T cells [Citation30]. T cell exhaustion denotes a hyporesponsive state of T cells with impaired cytotoxicity resulting in a failure of tumour-infiltrating T lymphocytes into the tumour microenvironment [Citation35]. While newer therapies, e.g., immunotherapy, restore exhausted T cells, chemotherapy promotes chronic local and systemic inflammation, leading to further T cell exhaustion, and this exhaustion of T cells may partially explain the observed association with EEAA [Citation36]. Similarly, PhenoAA was developed to estimate phenotypic age by leveraging nine clinical biomarkers, such as creatinine and glucose [Citation11]. Treatment with doxorubicin has been associated with elevated levels of creatinine [Citation37] and severe hyperglycaemia [Citation38], which may in part explain the association between chemotherapy and PhenoAA. Additionally, GrimAA was developed from eight surrogate biomarkers of blood plasma proteins, including growth differentiation factor 15 (GDF15) and tissue inhibitor metalloproteinase 1 (TIMP1) [Citation12]. GDF15 is a cytokine that mediates the body’s response to stress and demonstrated increased induction with doxorubicin treatment [Citation39], while TIMP1 has been associated with survival among women who received adjuvant chemotherapy [Citation40]. Interestingly, we observed a significant association between IEAA and chemotherapy that was not observed with either chemotherapy and radiation or radiation only therapy [Citation24]. Compared to the aforementioned ageing metrics, IEAA does not account for cell type proportions or clinical data and represents cellular intrinsic ageing that is thought to capture a fundamental ageing process that is largely conserved across cell types [Citation30]. This finding suggests systemic chemotherapy, independent of radiation therapy, may be associated with cellular ageing, although additional studies are warranted to explore the mechanism for this association. Overall, our results are consistent with previous findings that breast cancer treatment is associated with increased biological ageing and may have implications for vigilant surveillance of age-related health conditions among cancer survivors.
Ageing is a complex process that requires exploration of multiple interconnecting molecular pathways. In addition to the first-, i.e., IEAA and EEAA, and second-, i.e., PhenoAA and GrimAA, generation clocks, additional biological ageing metrics were developed to capture different aspects of the ageing process. Our study adds to the current literature by examining the association between TLA and DunedinPACE with chemotherapy, two ageing metrics not previously analysed [Citation24]. In comparison to T cell exhaustion, cellular senescence is due to replicative senescence (i.e., telomere shortening) and/or premature senescence (e.g., oxidative stress, DNA damaging agents, and mitogenic oncogenes) [Citation41], and chemotherapy has been demonstrated to modulate both T cell exhaustion and cellular senescence in breast cancer [Citation42]. Specifically, previous studies observed that higher markers of senescence, including p16INK4a 23 and telomerase activity [Citation20], were associated with accelerated biological ageing following treatment of breast cancer. Moreover, doxorubicin has been associated with shorter telomere length, as estimated via quantitative PCR-based, following initiation of chemotherapy treatment with subsequent lengthening, suggesting a potential reversal of telomere shortening [Citation21]. We observed DNA methylation-based telomere length to be shorter following administration of the first cycle of chemotherapy, suggesting breast cancer treatment may impact telomeres. Additionally, compared to the first- and second-generation clocks, DunedinPACE is less influenced by cohort effects and survival bias and focuses on the change in multi-organ system integrity during adult life [Citation14]. Moreover, DunedinPACE has been proposed to be a reliable measurement to evaluate the rate of ageing for interventional studies, including drug, behavioural, and environmental modifications [Citation14]. We observed greater estimates of DunedinPACE following breast cancer chemotherapy treatment, demonstrating a greater pace of ageing following pharmacological intervention for breast cancer. Several of the 19 biomarkers identified in the development of DunedinPACE have been shown to be associated with chemotherapy. For example, chemotherapy treatment has been associated with worsening of cardiorespiratory fitness and abnormal lipids, which may lead to a higher pace of ageing as estimated by DunedinPACE [Citation43,Citation44]. Moreover, studies investigating interventions to improve these biomarkers, such as exercise and diet, have shown to improve cellular senescence [Citation45,Citation46] and may potentially have implications in limiting chemotherapy induced biological ageing. Together, our results demonstrate that breast cancer chemotherapy may be associated with shortening of telomeres and a greater pace of ageing and strategies to either limit or prevent these changes, such as identifying less gerontogenic treatments or lifestyle modifications, may aid in slowing the ageing process and age-related diseases in breast cancer survivors.
This longitudinal study of women with breast cancer enabled the opportunity to evaluate chemotherapy-induced biological ageing as measured using DNA methylation. This study, however, is not without limitations. The sample size of this study was small, potentially reducing the power to detect additional associations as well as greater variability in effect estimates, as observed with wide confidence intervals. Furthermore, paired sampling data were not available in the dataset and thus, the biological age metrics were estimated combining both time points, rather than separately. Studies with larger sample sizes and paired sampling data are needed to confirm these observed associations. The number of covariates available in the dataset was limited and as such, the identified associations may be subject to residual confounding. Tumour characteristics, e.g., receptor status and stage, were also not available within the dataset. Assessment of these characteristics, either included as covariates or analysed by strata, may provide further information regarding whether chemotherapy-induced biological ageing varies by tumour characteristics. This analysis was restricted to the doxorubicin-cyclophosphamide chemotherapy regimen, and in the setting of rapid advancements in treatment, newer therapeutic modalities, e.g., immunotherapy and targeted therapy, are needed to examine whether similar associations are observed. Lastly, this study examined the acute effect of chemotherapy on biological ageing and additional studies are warranted to investigate the longitudinal effect of chemotherapy on biological ageing and subsequent age-related health sequelae.
In conclusion, we identified significant associations between chemotherapy treatment and IEAA, EEAA, PhenoAA, GrimAA, TLA, and DunedinPACE in women with breast cancer, with an acceleration in biological ageing following treatment. Our findings provide novel insights into the effect of doxorubicin-cyclophosphamide chemotherapy has on the ageing process. Breast cancer survival has improved over the past several decades due to advancements in prevention, early diagnosis, and treatment. These treatments, however, may lead to greater biological ageing and strategies to mitigate these processes may further improve survivorship and potentially, lower the risk of age-related health conditions among breast cancer survivors.
Authors’ contributions
The study was conceived and designed by DN and RC. DN generated the epigenetic age metrics, performed statistical analyses, and drafted the manuscript. RC, CV, and GH reviewed and provided comments on the final manuscript.
Ethical approval
The Institutional Review Boards at the parent institutions approved the study.
-) Supplemental Tables & figures.docx
Download MS Word (303.9 KB)Acknowledgments
We would like to thank the patients for partaking in the above research study and staff who aided in data collection and processing.
Disclosure statement
No potential conflict of interest was reported by the author(s)
Data availability statement
The dataset used in this analysis is available in NCBI’s Gene Expression Omnibus, https://www.ncbi.nlm.nih.gov/geo/query/acc.cgi?acc=GSE178887, accession number GSE178887.
Supplementary material
Supplemental data for this article can be accessed online at https://doi.org/10.1080/15592294.2024.2360160
Additional information
Funding
References
- Alkabban FM, Ferguson T. Breast cancer. Treasure Island (FL): StatPearls; 2024.
- Houghton SC, Hankinson SE. Cancer progress and priorities: breast cancer. Cancer Epidemiol Biomarkers Prev. 2021;30(5):822–9. doi: 10.1158/1055-9965.EPI-20-1193
- Giaquinto AN, Sung H, Miller KD. Breast cancer statistics, 2022. CA Cancer J Clin. 2022;72(6):524–541. doi: 10.3322/caac.21754
- Ahles TA, Root JC. Cognitive effects of cancer and cancer treatments. Annu Rev Clin Psychol. 2018;14(1):425–451. doi: 10.1146/annurev-clinpsy-050817-084903
- Alfano CM, Peng J, Andridge RR. Inflammatory cytokines and comorbidity development in breast cancer survivors versus noncancer controls: evidence for accelerated aging? J Clin Oncol. 2017;35(2):149–156. doi: 10.1200/JCO.2016.67.1883
- Franceschi C, Garagnani P, Morsiani C. The continuum of aging and age-related diseases: common mechanisms but different rates. Front Med. 2018;5:61. doi: 10.3389/fmed.2018.00061
- Colloca G, Di Capua B, Bellieni A. Biological and functional biomarkers of aging: definition, characteristics, and how they can impact everyday cancer treatment. Curr Oncol Rep. 2020;22(11):115. doi: 10.1007/s11912-020-00977-w
- Noroozi R, Ghafouri-Fard S, Pisarek A. DNA methylation-based age clocks: From age prediction to age reversion. Ageing Res Rev. 2021;68:101314. doi: 10.1016/j.arr.2021.101314
- Horvath S. DNA methylation age of human tissues and cell types. Genome Biol. 2013;14(10):R115. doi: 10.1186/gb-2013-14-10-r115
- Hannum G, Guinney J, Zhao L. Genome-wide methylation profiles reveal quantitative views of human aging rates. Mol Cell. 2013;49(2):359–367. doi: 10.1016/j.molcel.2012.10.016
- Levine ME, Lu AT, Quach A. An epigenetic biomarker of aging for lifespan and healthspan. Aging (Albany NY). 2018;10(4):573–591. doi: 10.18632/aging.101414
- Lu AT, Quach A, Wilson JG. DNA methylation GrimAge strongly predicts lifespan and healthspan. Aging (Albany NY). 2019;11(2):303–327. doi: 10.18632/aging.101684
- Lu AT, Seeboth A, Tsai PC. DNA methylation-based estimator of telomere length. Aging (Albany NY). 2019;11(16):5895–5923. doi: 10.18632/aging.102173
- Belsky DW, Caspi A, Corcoran DL. DunedinPACE, a DNA methylation biomarker of the pace of aging. Elife. 2022;11:11. doi: 10.7554/eLife.73420
- Ambatipudi S, Horvath S, Perrier F. DNA methylome analysis identifies accelerated epigenetic ageing associated with postmenopausal breast cancer susceptibility. Eur J Cancer. 2017;75:299–307. doi: 10.1016/j.ejca.2017.01.014
- Mak JKL, McMurran CE, Kuja-Halkola R. Clinical biomarker-based biological aging and risk of cancer in the UK biobank. Br J Cancer. 2023;129(1):94–103. doi: 10.1038/s41416-023-02288-w
- Kresovich JK, Xu Z, O’Brien KM. Methylation-based biological age and breast cancer risk. J Natl Cancer Inst. 2019;111:1051–1058. doi: 10.1093/jnci/djz020
- Rentscher KE, Bethea TN, Zhai W. Epigenetic aging in older breast cancer survivors and noncancer controls: preliminary findings from the thinking and living with cancer study. Cancer. 2023;129(17):2741–2753. doi: 10.1002/cncr.34818
- Guida JL, Ahles TA, Belsky D. Measuring aging and identifying aging phenotypes in cancer survivors. J Natl Cancer Inst. 2019;111(12):1245–1254. doi: 10.1093/jnci/djz136
- Scuric Z, Carroll JE, Bower JE. Biomarkers of aging associated with past treatments in breast cancer survivors. NPJ Breast Cancer. 2017;3(1):50. doi: 10.1038/s41523-017-0050-6
- Benitez-Buelga C, Sanchez-Barroso L, Gallardo M. Impact of chemotherapy on telomere length in sporadic and familial breast cancer patients. Breast Cancer Res Treat. 2015;149(2):385–394. doi: 10.1007/s10549-014-3246-6
- Cupit-Link MC, Kirkland JL, Ness KK. Biology of premature ageing in survivors of cancer. Vol. 2. European Society for Medical Oncology Open; 2017. p. e000250. doi: 10.1136/esmoopen-2017-000250
- Sanoff HK, Deal AM, Krishnamurthy J. Effect of cytotoxic chemotherapy on markers of molecular age in patients with breast cancer. J Natl Cancer Inst. 2014;106(4):dju057. doi: 10.1093/jnci/dju057
- Sehl ME, Carroll JE, Horvath S. The acute effects of adjuvant radiation and chemotherapy on peripheral blood epigenetic age in early stage breast cancer patients. NPJ Breast Cancer. 2020;6(1):23. doi: 10.1038/s41523-020-0161-3
- Bauer MA, Todorova VK, Stone A. Genome-wide DNA methylation signatures predict the early asymptomatic doxorubicin-induced cardiotoxicity in breast cancer. Cancers (Basel). 2021;13(24):6291. doi: 10.3390/cancers13246291
- Tian Y, Morris TJ, Webster AP. ChAMP: updated methylation analysis pipeline for Illumina BeadChips. Bioinformatics. 2017;33(24):3982–3984. doi: 10.1093/bioinformatics/btx513
- Teschendorff AE, Marabita F, Lechner M. A beta-mixture quantile normalization method for correcting probe design bias in Illumina Infinium 450 k DNA methylation data. Bioinformatics. 2013;29(2):189–196. doi: 10.1093/bioinformatics/bts680
- Xu Z, Niu L, Li L. ENmix: a novel background correction method for Illumina HumanMethylation450 BeadChip. Nucleic Acids Res. 2016;44(3):e20. doi: 10.1093/nar/gkv907
- Higgins-Chen AT, Thrush KL, Wang Y. A computational solution for bolstering reliability of epigenetic clocks: Implications for clinical trials and longitudinal tracking. Nat Aging. 2022;2(7):644–661. doi: 10.1038/s43587-022-00248-2
- Chen BH, Marioni RE, Colicino E. DNA methylation-based measures of biological age: meta-analysis predicting time to death. Aging (Albany NY). 2016;8(9):1844–1865. doi: 10.18632/aging.101020
- Houseman EA, Accomando WP, Koestler DC. DNA methylation arrays as surrogate measures of cell mixture distribution. BMC Bioinf. 2012;13(1):86. doi: 10.1186/1471-2105-13-86
- Markov Y, Levine M, Higgins-Chen AT. Reliable detection of stochastic epigenetic mutations and associations with cardiovascular aging. GeroScience. 2024. doi: 10.1007/s11357-024-01191-3
- R Core Team. R: a language and environment for statistical computing. Vienna, Austria: R Foundation for Statistical Computing; 2020.
- Kresovich JK, O’Brien KM, Xu Z. Changes in methylation-based aging in women who do and do not develop breast cancer. J Natl Cancer Inst. 2023;115:1329–1336. doi: 10.1093/jnci/djad117
- Jiang Y, Li Y, Zhu B. T-cell exhaustion in the tumor microenvironment. Cell Death Dis. 2015;6(6):e1792. doi: 10.1038/cddis.2015.162
- Fang L, Liu K, Liu C. Tumor accomplice: T cell exhaustion induced by chronic inflammation. Front Immunol. 2022;13:979116. doi: 10.3389/fimmu.2022.979116
- Elsherbiny NM, El-Sherbiny M. Thymoquinone attenuates doxorubicin-induced nephrotoxicity in rats: Role of Nrf2 and NOX4. Chem Biol Interact. 2014;223:102–108. doi: 10.1016/j.cbi.2014.09.015
- de Lima Junior Ea, Yamashita AS, Pimentel GD. Doxorubicin caused severe hyperglycaemia and insulin resistance, mediated by inhibition in AMPk signalling in skeletal muscle. J Cachexia Sarcopenia Muscle. 2016;7(5):615–625. doi: 10.1002/jcsm.12104
- Winter LM, Reinhardt D, Schatter A. Molecular basis of GDF15 induction and suppression by drugs in cardiomyocytes and cancer cells toward precision medicine. Sci Rep. 2023;13(1):12061. doi: 10.1038/s41598-023-38450-w
- Schrohl AS, Look MP, Meijer-van Gelder ME. Tumor tissue levels of Tissue Inhibitor of Metalloproteinases-1 (TIMP-1) and outcome following adjuvant chemotherapy in premenopausal lymph node-positive breast cancer patients: a retrospective study. BMC Cancer. 2009;9:322. doi: 10.1186/1471-2407-9-322
- Zhao Y, Shao Q, Peng G. Exhaustion and senescence: two crucial dysfunctional states of T cells in the tumor microenvironment. Cell Mol Immunol. 2020;17(1):27–35. doi: 10.1038/s41423-019-0344-8
- Rodriguez IJ, Bernal-Estevez DA, Llano-Leon M. Neoadjuvant chemotherapy modulates exhaustion of T cells in breast cancer patients. PLoS One. 2023;18(2):e0280851. doi: 10.1371/journal.pone.0280851
- Peel AB, Thomas SM, Dittus K. Cardiorespiratory fitness in breast cancer patients: a call for normative values. J Am Heart Assoc. 2014;3(1):e000432. doi: 10.1161/JAHA.113.000432
- He T, Wang C, Tan Q. Adjuvant chemotherapy-associated lipid changes in breast cancer patients: a real-word retrospective analysis. Medicine (Baltimore). 2020;99(33):e21498. doi: 10.1097/MD.0000000000021498
- Meng J, Geng Q, Jin S. Exercise protects vascular function by countering senescent cells in older adults. Front Physiol. 2023;14:1138162. doi: 10.3389/fphys.2023.1138162
- Xiang Q, Tian F, Xu J. New insight into dyslipidemia-induced cellular senescence in atherosclerosis. Biol Rev Camb Philos Soc. 2022;97(5):1844–1867. doi: 10.1111/brv.12866