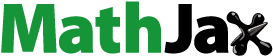
ABSTRACT
Over the past twenty-five years, per capita water use has declined in many US cities. Technological and policy changes partially explain this decline, but variables beyond the control of water managers also influence water use including external (e.g. drought) and emergent (e.g. public attention) changes. Importantly, these variables interact and the relationships between these variables and water use are non-stationary. However, many models assume fixed relationships between water use and its drivers, and limited interaction between variables. Here we present a flexible socio-hydrological approach to model how conservation strategies, and external and emergent changes, interact to influence per capita water use. We apply this approach to Las Vegas and find that marginal water rates, code changes coupled with population growth, and conservation response to water stress are the key drivers of the observed decline. Critically, modeling these strategies in absence of their connections to population growth and water stress cannot fully account for observed changes.
KEYWORDS:
1. Introduction
Over the past twenty-five years, per capita water use has declined in many US cities, as documented in both investigations of individual cities (Hester and Larson Citation2016; Ashoori, Dzombak, and Small Citation2016) and national scale analyses (Coomes et al. Citation2010; Perrone et al. Citation2015). Technological change and increasing efficiency of water fixtures and appliances contributed significantly to this decline (Suero, Mayer, and Rosenberg Citation2012; Cahill and Lund Citation2013). Further, changes in landscaping and irrigation practices decreased outdoor water use (Mini, Hogue, and Pincetl Citation2014; Sovocool, Morgan, and Bennett Citation2006), particularly in arid and semi-arid regions. However, temporal and regional variation indicates further complexity and highlights the need to consider changes outside the direct control of water managers both external (e.g. population growth and decline, economic cycles, drought) and emergent (e.g. public attention, water use behavior).
One salient example is the case of Las Vegas where average per capita water usage decreased by over 350 liters per person per day from 1990 to 2012 () (Clark County Citation2014; Bureau of Reclaimation Citation2012; King Citation2014). During this period, there are several notable trends and events related to water management in Las Vegas. First, Las Vegas experienced rapid population growth (an 120% increase) from 1990 and 2012 (Clark County Citation2014). Second, the Colorado River drought which began in the early 2000s prompted significant public attention to and policy action on water supply and demand management (SNWA Citation2005a, Citation2005b). Third, Las Vegas was significantly impacted by the Great Recession; population growth slowed and temporarily reversed, and utility revenue declined (SNWA Citation2013; Clark County Citation2014). This paper, takes the Las Vegas case as an illustrative example to address how water management strategies, and external and emergent changes interact to influence per capita water use.
Figure 1. Time series of water use and potential drivers of change: (a) annual per capita water use (LPCPD), (b) monthly per capita water use (LPCPD), (c) LVVWD service area population, (d) population density (people/km2 of developed land) of Clark County, (e) LVVWD water rates by tier, (f) LVVWD water use by sector, (g) Lake Mead water elevation, (h) percent of articles in the Las Vegas Review Journal on water issues.
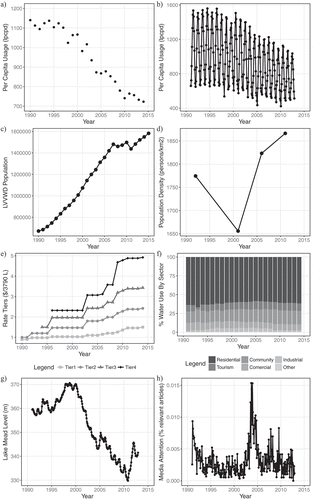
Per capita water use is influenced by many factors including: external variables (e.g. weather, economic cycles, population growth (Balling, Gober, and Jones Citation2008; Franczyk and Chang Citation2009; Hester and Larson Citation2016; Kenney et al. Citation2008)), policy decisions (e.g. building and landscape codes, water rates, conservation programs (Ahmad and Prashar Citation2010; Coomes et al. Citation2010; Dalhuisen et al. Citation2003; Inman and Jeffrey Citation2006)), socio-economic variables (Wentz and Gober Citation2007; Schleich and Hillenbrand Citation2009), demographic variables (Ashoori, Dzombak, and Small Citation2016; Willis et al. Citation2013), and consumer attitudes (Gilg and Barr Citation2006; Jorgensen, Graymore, and O’Toole Citation2009; Koutiva et al. Citation2017), perceptions (Jones et al. Citation2011; Beal, Stewart, and Fielding Citation2013; Stoutenborough and Vedlitz Citation2014), and attention (Fielding et al. Citation2012; Quesnel and Ajami Citation2017). In an assessment of per capita water use change across 43 US water utilities, Coomes et al. (Citation2010) found that while hydro-climatic and economic variables explained regional and temporary variability, the increased penetration of low-flow fixtures explained the widespread decrease in use. Critically, questions remain on how technology change (e.g. low-flow fixtures) and these other variables interact and affect water use on multiple time scales (Hester and Larson Citation2016; Zhang, Diao, and Dong Citation2013). As the aim of this study is to explain city-wide changes in water usage over multiple years, variables that drive spatial heterogeneity (e.g. household characteristics) or temporary fluctuations in consumption (e.g. weather, recessions) are not considered. While water use forecasting models consider a wide array of explanatory variables, they do not incorporate feedback effects (Billings and Jones Citation2008). The implicit assumption is that these variables, and their relationship to water consumption, are unaffected by both hydrological variability and policy decisions.
The theories and tools of economics have long been used to understand and predict the interaction between water supply and demand (Booker et al. Citation2012; Harou et al. Citation2009; Noel and Howitt Citation1982). However, water is an imperfect economic good. Water prices are rarely the result of unimpeded interaction between supply and demand; economic equilibria in water allocation is constrained by hydrology, infrastructure, and institutions (Booker et al. Citation2012). A wider view on water use is needed particularly in mature water economies with escalating costs of supply augmentation (Randall Citation1981).
Popular and political support, as well as financial resources for water conservation, tends to increase during times of water stress (Evans et al. Citation2015; Fielding et al. Citation2012; Quesnel and Ajami Citation2017). Although these measures may have been previously planned or piloted, the occurrence of water stress can, if other drivers are aligned, open a window of opportunity to implement conservation measures (Hughes, Pincetl, and Boone Citation2013; Treuer et al. Citation2017). Further, policy response to water stress beyond a specified threshold can be, as is the case in Las Vegas, codified into policy and law (SNWA Citation2005a). Additionally, evidence shows that occurrence of environmental events can, by increasing the salience of the issue, change policy makers’ and consumers’ cost benefit calculation (Bordalo, Gennaioli, and Shleifer Citation2012; Dessaint and Matray Citation2015; Hand et al. Citation2015). While some of the conservation gains, such as those due to behavioral change may be temporary, others are permanent (Russell and Fielding Citation2010; Geller, Erickson, and Buttram Citation1983).
This paper uses the case of Las Vegas as a prototypical example and seeks to understand why the decline in water use occurred and to translate this understanding into a per capita water use model. This paper addresses two questions: 1) Which variables explain the per capita water use decline in Las Vegas? 2) How can we model the interaction between policy choices and external and emergent changes? Recent work (Brelsford and Abbott Citation2017) has incorporated interactions between independent variables influencing water use in a regression model. Here we develop a set of non-linear differential equations to capture interactions between dependent and independent variables. This socio-hydrological approach also facilitates later extension to include two-way feedback between water use and relevant variables, which is increasingly recognized as important to water supply planning (Srinivasan et al. Citation2016; Gonzales and Ajami Citation2017). Average per capita water use, rather than disaggregated use, is the focus for two reasons. First, policy at the city scale is constant in space and across populations. Average per capita water use focuses the analysis on temporal variation. Second, modeling average use decreases the computational and cognitive costs of integrating a broad range of variables and processes (Rahmandad and Sterman Citation2004).
This paper is organized as follows. In the following sections, we present the case of Las Vegas, and outline our methodology, which includes a quantitative and qualitative screening analysis, differential equation model development, and multi-model comparison. Then we describe the results of the screening analysis and the variables to be included in model development. Next, we present a series of water consumption models and compare their ability to explain trends in per capita water consumption in Las Vegas. Finally, we discuss the new insights provided by this modeling approach, policy implications of the findings, and opportunities for future improvement.
2. Case description
The Las Vegas metropolitan area is selected as a case study due to the combined challenges of population growth and supply limitations, common to many cities in the arid west. Natural climate variability with periodic droughts has challenged water providers; projected climate changes and further population growth will exacerbate these challenges. The Las Vegas metropolitan area draws 90% of its water supply from Lake Mead on the Colorado River and the rest from local groundwater. The Southern Nevada Water Authority (SNWA) is the wholesale water provider that withdrawals and transports water from Lake Mead and sells it to local utilities (SNWA Citation2009a). Five local water utilities deliver water to consumers. This paper focuses on the largest of these, the Las Vegas Valley Water District (LVVWD), which serves the city of Las Vegas and nearby unincorporated areas. The initial analysis of the Las Vegas case covers the period from 1990 to 2014; model development then focuses on 1997 to 2012, the period for which monthly data for all relevant variables was available.
3. Methods
3.1 Screening analysis
As summarized above, a wide range of variables can influence per capita water use. We apply three criteria to select variables to include in a screening analysis: 1) findings from previous studies, 2) case specific context, and 3) the availability of temporal data at the scale of the LVVWD. The plausibility of these drivers is first assessed qualitatively using the case history and the series of variables described in .
Table 1. Data sources and characteristics.
For the drivers found relevant, linear regression analysis is applied to quantitatively test for and assess the strength of a relationship to per capita consumption. A multiple linear regression is run to assess which of the independent variables belong in the model. The Akaike Information Criterion (AIC) (Cavanaugh and Neath Citation2011), R-squared, Adjusted R-squared, and the F-test are used for comparison and where metrics disagree AIC was used.
3.2 Model development and assessment
The multiple hypothesis approach is continued through model development and testing with the aim of identifying the model that best balances fit and parsimony. Here the potential drivers of consumption change, retained after the screening analysis, are used to develop a series of competing mathematical models of per capita consumption. Different combinations of these variables are tested and compared using the following metrics: root mean square error (RMSE), percent bias, Nash-Sutcliff efficiency (NSE), and the coefficient of determination (R2) (Moriasi et al. Citation2007).
Not all parameters can be readily measured or reasonably estimated based on prior investigation, therefore calibration and validation are needed. A challenge of calibrating and testing models that simulate the response to infrequent phenomena, such as drought or rapid population growth, is that there may only be one or two occurrences of the phenomena in the study period. The traditional approach to calibration and validation divides the historical data set into two periods, one for each calibration and validation. Using this approach to selecting calibration and validation periods, important but infrequent phenomena, or combinations of such phenomena, may be included the calibration but not validation periods hampering the researchers’ ability to assess model validity. For example, in the Las Vegas case the periods 1997 to 2006 and 2007 to 2012 were initially selected for calibration and validation respectively. There are fundamental differences between these periods. For example, population grows rapidly in the calibration period but slows during the validation period.
Alternate year calibration is used to address this challenge and ensure that calibration and validation periods contain comparable exogenous conditions (Gowda et al. Citation2012). To implement this procedure the model is run for the full period of historic data (i.e. using the entire time series of exogenous variables such as population). Then calibration statistics, computed using only alternate years (in this case the odd numbered years), are used to select parameter values. Then, model performance is assessed by computing validation statistics using the remaining years.
4. Model development and results
4.1 Screening analysis
The case history suggests several potential drivers related to the decline in water consumption including stricter water efficiency codes, increasing population density, higher water rates, and policy and consumer response to water stress. The substantial population growth also points to the potential for socio-economic, demographic, environmental attitude or perception changes. While complete time series data was unavailable for socio-economic and demographic variables, the decadal US Census provides periodic data that can be used to check for trends. US Census data for the City of Las Vegas shows no significant changes in household size (from 2.6 in 2000 to 2.7 in 2010) (US Census Citation2000a, Citation2010a). The Census data also shows that median household income increased (from $44K in 2000 to $54K in 2010); therefore, income change cannot explain the per capita demand decrease observed (Mini, Hogue, and Pincetl Citation2015; US Census Citation2000b, Citation2010b). However, the potential for changes in economic structure (i.e. the shift away from water intensive industries) remains. Environmental value orientation and perceptions of perceived risk are hypothesized to influence water use in this case. However, data is only available on these variables for Las Vegas at one point in the study period so we were unable to incorporate these variables directly into a temporal analysis (Salvaggio et al. Citation2014). Given, the lack of evidence for socio-economic and demographic changes, and the lack of data on attitudes and perceptions, we proceed with a screening analysis of the following drivers: stricter water efficiency codes, increasing population density, higher water rates, changes in economic structure, and policy and consumer response to water stress. The plausible drivers of water use decline, including stricter water efficiency codes, increasing population density, higher water rates, changes in economic structure, and policy and consumer response to water stress, are assessed qualitatively and quantitatively.
Rules on fixture and appliance efficiency, and allowable landscape types and quantities dictate the water efficiency required in new construction or substantial renovations. During the study period, there were two substantial code changes that impacted buildings and landscapes in Las Vegas. The first was the 1993 Nevada state regulation which set efficiency standards for indoor plumbing fixtures in both residential and commercial applications (Nevada State Legislature Citation2015). There was no previous state or federal standard. (The Energy Policy Act set equivalent standards nationally and took effect in January 1994 (U.S. General Accounting Office Citation2000).) The second code change was City of Las Vegas’ landscaping ordinance limiting turf grass in new residential construction, effective January 2004. This rule prohibited turf in front lawns, restricted it in backyards and limited common space turf. Once installed, plumbing fixtures have a long life span, from 15 years for faucets to 100 years for toilets, with proper maintenance (InterNACHI Citation2016); landscape codes are only triggered by new construction. Due to the long lifespan of fixtures and focus on new landscapes, code changes alone do not quickly change water use in existing buildings. However, when coupled with rapid population growth, code changes can have a significant impact. Between 1993 when the state of Nevada fixture efficiency regulation went into effect and 2014 the LVVWD service area population grew by 107% ()) (Clark County Citation2014). In other words, at the start of 1993 0% of the existing homes were built under fixture efficiency standards, while in 2015 over 50% of the existing homes were built under fixture efficiency standards.
Population density is a product of lot size and household size. Lot size can influence water consumption in two ways. First, areas with lower population density tend to have greater yard space, which in an arid climate such as Las Vegas requires year-round irrigation (Wentz and Gober Citation2007). Second, homes also tend to have more square footage per person in low-density areas; more water is needed to clean this additional space, increasing indoor water consumption. While households with more people usually have higher total water use, they typically have lower per capita water use (Willis et al. Citation2013). The relationships between lot size and per capita water use and household size and per capita water use both indicate that higher population density leads to lower per capita water use. Population density, in people per square km of developed area, decreased 6.7% from 1992 to 2001 ()) (USGS Citation2016; Clark County Citation2014). During the same period per capita water consumption decreased by 10%, making population density change an unlikely driver.
Water consumption is relatively inelastic to changes in price in the western United States (Dalhuisen et al. Citation2003). The LVVWD has had an increasing block price structure since 1990, for both residential and commercial customers (SNWA Citation2009b). Block rate pricing introduces discontinuities and non-linearities in the relationship between demand and price; when demand crosses a block threshold there is a step change in price (Dalhuisen et al. Citation2003). When examining the relationship between demand and price at monthly increment, seasonal variations in demand introduce an additional complication. As consumer price and the quantity demanded are simultaneously determined (Olmstead, Hanemann, and Stavins Citation2007), seasonal variation in outdoor water requirements may cause some consumers to cross rate thresholds leading to the counterintuitive observation of higher (lower) demands coinciding with a higher (lower) marginal price. For this reason, an effective marginal price, which is the rate charged to the average water user based on their annual average consumption, is used in this study. ) shows all LVVWD price tiers. There are several changes in price during the study period including increases to the number of tiers in the block rate structure and rate increases for each tier ()), so it remains a plausible cause.
The population of the LVVWD service area increased by 130% from 1990 to 2014 (Clark County Citation2014). Given such a significant demographic shift, it is plausible that economic structure of Las Vegas metropolitan area transitioned during this period. For example, Hester and Larson (Citation2016) found that the decline of water intensive industries contributed to the decline in per capita water consumption in three North Carolina cities. Water use by sector (i.e. residential, commercial, industrial, etc.) for the Las Vegas Valley Water District is used to check for such a change. The percentage of total water used by each sector has not changed significantly over this period ()), making economic shifts an unlikely driver.
The final hypothesized driver of water consumption change is the policy and consumer response to water stress events. In addition to rate increases, water utilities and municipalities use regulation, incentives, and education to promote water conservation (SNWA Citation2009a). As Lake Mead is the dominate supply source for Las Vegas it is used here as a proxy for water stress. As seen in ), since the late 1980s Lake Mead levels have been primarily decreasing. This decrease is significant for three reasons. First, Lake Mead provides the majority of the storage for the Lower Basins states along the Colorado River. Second, if the elevation of Lake Mead reaches 328 m (1075 ft) shortage declaration will be triggered and both Nevada’s and Arizona’s allocations will decrease. Third, Las Vegas draws water directly from Lake Mead via two intake structures (a third is constructed but not operational) at elevations 305 m (1000 ft) and 320 m (1050 ft). Taking one of these intake structures off line due to lake levels would significantly reduce the available supply. The rate of Lake Mead declines accelerated in the early 2000s with the onset of regional drought. As stated above, the LVVWD uses regulation, incentives and education to promote water conservation. While some of these efforts date back to the early 1990s, they were intensified in 2003 due to the ongoing drought (Sovocool, Morgan, and Bennett Citation2006). This increased focus on conservation in 2003 corresponds with the beginning of a multi-year period of accelerated consumption decrease, making water stress response a plausible driver. Further, changes in water stress, as indicated by Lake Mead levels approaching shortage thresholds, correspond with increasing coverage of water issues in Las Vegas Review Journal (the major newspaper of the metropolitan area) ()). In absence of survey data, media coverage is a reasonable proxy variable for the salience of or public attention to an issue (Treuer et al. Citation2017) and is correlated with changes in water use (Quesnel and Ajami Citation2017; Gonzales and Ajami Citation2017). We hypothesize that changes in water stress drive changes in the salience of water supply for policy makers and consumers, and retain water stress as a variable for further analysis.
A multiple linear regression model, with monthly per capita consumption as the dependent variable, was developed to test plausible causes of the drop in per capita water consumption including: marginal price, population, and Lake Mead levels. Multiple linear regression was applied to different combinations of the independent variables and the best model found contained all three independent variables (lowest AIC (−2397.84), R2 = 0.79; ). To assess if the seasonal signal in the consumption data influenced the results, per capita consumption were seasonally adjusted by subtracting monthly average values. The use of seasonally adjusted consumption did not impact the results.
Table 2. Multiple linear regression results.
4.2 Model development
Based on the screening analysis, price, population growth, and water stress are used in model development. As the relationship between price and water demand is established (the price elasticity of demand equation), this model serves as a basis of comparison for further model development (Griffin Citation2006, 31). defines model variables, units, and values.
Table 3. Model variables and parameters.
Table 4. Per capita water use model comparison statistics.
A water quality model is used as an analogy to develop a mathematical model for the relationship between code change and per capita water consumption. As noted above, there were two substantial code changes impacting water efficiency: indoor fixtures in 1993 and landscaping in 2004. As the population of Las Vegas increases new homes, businesses, and public spaces are built to accommodate newcomers. Over time, an increasing percentage of the building and landscape stock meets or exceeds the new codes. Data from two studies on the efficiency of homes in Las Vegas is used to approximate the efficiency differences in building built before 1993, between 1993 and 2003 and after 2003 (Cooley et al. Citation2007; Bennett Citation2014). The relationship between average per capita consumption and population is analogous to the relationship between concentration of a conserved solute in a tank and a step change decrease in inflow concentration. Over time the tank volume will increase, the tank concentration will decrease, and the new concentration can be computed from the mass balance. Using this analogy and rearranging the equation (see Appendix A), yields the following equation for the rate of change in per capita consumption with population and code change:
Likewise, there are no existing equations for the relationship between per capita consumption and water stress, but knowledge of the physical and institutional system can inform the mathematical form. First, a portion of this consumption decrease is maintained after water stress subsides because changes to water fixtures and landscaping increase water efficiency. Second, the persistent portion of the consumption decrease will shrink as consumption approaches the current technological and cultural minimum. Third, while water stress has persisted since the early 2000s there are moments where public attention and resources are focused on the issue of water stress; at other times during the decade plus long drought public attention and resources are focused on other challenges such as balancing the utility budget. Fourth, the degree of water consumption response to a given stress level may be context specific. Finally, while Lake Mead storage is good proxy variable for local water stress, it is not directly related to per capita consumption declines or the actions that cause those declines. Changes in Lake Mead levels are linked to water stress and attention to water issues; however, not all decreases in water level generate the same response. Decreases in water levels near operational thresholds (i.e. lake levels that trigger a shift from surplus to normal conditions) lead to greater public and policy attention (see ).
These five observations inform the equation relating water stress to water consumption change. First, the salience of water supply issues, M, is defined as a variable. The salience or public attention to an issue is hard to measure, particularly retrospectively (Treuer et al. Citation2017). For this reason, media attention as measured by percent of local newspaper articles related to water issues was used as a proxy variable. The methodology used to collect and analyze the media data is detailed in (Treuer et al. Citation2017). While percent of relevant articles is not a perfect measure of the salience of water issues, it has been found to be a useful proxy in recent studies (Quesnel and Ajami Citation2017). As seen in ), the media record shows both peaks and periods of consistent low coverage. The authors hypothesize that it is the deviations from average coverage not the absolute levels of coverage that relate to a change in water salience. Therefore, the baseline media coverage is identified and removed using a graphical method analogous to the graphical method for baseflow analysis (Dunne and Leopold Citation1978). Second, a responsiveness parameter, α, is defined to account for contextual differences (Garcia, Portney, and Islam Citation2016). Third, the ratio of the minimum consumption and the current consumption is used to ensure that consumption asymptotically approaches the minimum (Garcia, Portney, and Islam Citation2016).
The model was developed at a monthly timescale to maximize use of existing data. However, per capita consumption has a strong seasonal signal which is not accounted for by the water use change predictors. The seasonal signal was added to the model by relating mean monthly air temperature to per capita use. Analogously to Nash and Barsi (Citation1983), deviations from the annual mean per capita consumption are related to observed average monthly temperature. This approach assumes that seasonal variation in use can be linearly related to the product of temperature and annual per capita consumption. (The product of temperature and annual consumption is used because the amplitude of the seasonal cycle has decreased as consumption has declined due to the reduction in outdoor water usage.) First, the deviations from the annual mean per capita consumption are computed by subtracting the twelve-month moving average of per capita consumption.
Then, a linear regression was performed to determine the relationship between the independent variable (the product of mean average monthly temperature, Tt, and average annual per capita consumption), and the dependent variable (per capita consumption deviations from the mean, MDt). The coefficient, β1, and intercept, β0, found are then used to add the seasonal signal to the consumption model. Note that the index, a, denotes the annual average per capita water usage and the index, s, denotes that seasonality of demand is incorporated. For further information on model development, please see Appendix A.
4.3 Model testing
A series of combinations of the three drivers were tested against observed water use. In addition to the price only model (Equation (1)), the performance of three models is described below: price and population growth, population growth and water stress response, and price, population growth and water stress response.
The base model (price only), captures the general trend but not the magnitude of declining per capita consumption ()) and performs poorly on all metrics (). The addition of population growth with code change to the model substantially improves performance ()) but percent bias remains around 12% for calibration and validation periods (). The third model ()) that pairs population growth with stress response without price decreases percent bias around 6% and increases NSE by 0.1. The model combining all drivers ()) is narrowly the best by RMSE and percent bias for the calibration period but, for the validation period, the third model is narrowly the best by RMSE and NSE. R2 provides an estimate of the percent variance explained by each model. Based on validation period statistics, the price only model explains 51% of the observed variance, and addition of code change and stress response increases this to 79% and 86% respectively. Goodness-of-fit statistics can be dominated by seasonality in time series with strong seasonal cycles. A seasonal trend decomposition analysis was performed and the model applied to the trend only water use time series, and verified that model fit is not dominated by seasonality. See Appendix B for further details.
5. Discussion and conclusions
The analysis shows that in the case of Las Vegas marginal price, population growth coupled with code changes, and response to water stress, explain the observed decrease in per capita water consumption, consistent with past studies (Brelsford and Abbott Citation2017). The contribution of this work is the development of a non-linear differential equation model that accounts for both variable interactions and changing relationships between water use and predictor variables. The inclusion of the three variables, water rates, building and landscape codes, and utility responsiveness to water stress, enables modelers to link water consumption scenarios to policy choices as well as to scenarios of external variables such as population and projections of water stress. The modeling approach presented enables water managers to explore the degree and timing of policy impacts under alternate projections of external and emergent changes.
The addition of population growth and stress response to the base price model substantially improved performance (). Adding just population growth to the price model increased NSE from 0.29 to 0.64, and decreased percent bias from 19.1 to 11.9%. The model including population growth and stress response, but not price, further improves model performance on the three metrics. Building and landscape code changes had the largest influence on model quality, due to the rapid population growth in Las Vegas during the study period. This is consistent with recent case based analyses that have found that building level technologies and landscape changes such as xeriscaping and efficient irrigation systems contributed to the observed decrease in per capita water use (Suero, Mayer, and Rosenberg Citation2012; Sovocool, Morgan, and Bennett Citation2006). Additionally, Coomes et al. find that low-flow appliances was the primary contributor to the decline in per capita water use across US cities (Coomes et al. Citation2010). However, the relative importance of these factors could vary if population growth and streamflow shift in the future. The inclusion of water stress is notable because including stress response as a driver of water use change links changes in water use to supply variability. This feedback implies supply and consumption should not be considered in isolation and that simultaneously evaluating infrastructure and policy gives an improved view of system performance. The differential equation approach used facilitates extension to model two-way feedback between supply and demand.
The model including all three drivers only slightly outperformed the model including population growth and stress response. This may be because price and salience, under some circumstances, covary. Utilities change water rates both in response to water stress and in response to financial conditions. Rate changes driven by water stress typically, as is the case in Las Vegas, coincide with periods of increased salience. Rate changes, driven by changes in financial resources, such as revenue declines or new expenses, may not coincide with salience peaks. Although these rate changes are not aimed at decreasing demands, they do trigger a demand response. This covariance would explain why incorporating all three variables results in the narrowly best performing model, and that different values of α (a measure of water stress response) are found for the third and fourth models (1.91, 0.71).
The model developed in this study is a simple stylized representation of the Las Vegas case. In comparison to more detailed models built on disaggregated water usage data, this simplicity has both advantages and disadvantages. High resolution water use models have significant advantages in linking specific interventions (i.e. installation of efficient faucets, voluntary conservation measures) (Mini, Hogue, and Pincetl Citation2015; Abdallah and Rosenberg Citation2014) to water use changes. However, simple models representing the dominant drivers for a given problem have advantages in building and testing theory (Sivapalan et al. Citation2003; Bokulich Citation2013). We agree with Stirzaker et al. (Citation2010) that these two approaches are synergistic and that iteration between models of varying levels of complexity builds scientific understanding. This work, viewed in concert with more detailed water use modeling studies (e.g. Brelsford and Abbott Citation2017), can help to bridge the gap between case specific findings and generalizable understanding of urban water supply and demand as a complex socio-hydrological system. Further work can assess and enhance the robustness and generalizability of this model. First, a thorough evaluation of model uncertainty, particularly that stemming from the estimated and calibrated parameters, could drive further data collection and model refinement. For example, the average per capita consumption generated by buildings constructed according to a given code is estimated; the variance of this estimate should be used to assess how uncertainty in this variable propagates throughout the model. Second, the other potential drivers investigated, while not dominant in this case, may prove important in other cases. Application of this methodology to other cities with observed per capita consumption changes is necessary both to test the validity of the mathematical relationships developed here and to understand how other drivers such as population density relate to per capita consumption. Third, the contributions of policy implementation and shifts in consumer perceptions and attitudes to the salience component of water use change should be investigated (Gilg and Barr Citation2006; Jones et al. Citation2011). Repeated surveys on environmental attitudes, risk perceptions, and policy preferences would enable researchers to isolate the impacts of these factors on water use. Finally, a challenge of any modeling effort is to judge when and if observed relationships should be projected into the future. This is a particularly relevant concern when future conditions are projected to be out of the bounds of past observations, as is the case for temperature and streamflow here (Christensen and Lettenmaier Citation2007). Application of the method to other cases can also address this challenge by testing the model under a broader range of conditions. We, however, hope this work provides a pathway for future developments, by developing a socio-hydrological modeling framework to incorporate interactions between policy choices, and external and emergent changes.
Appendix A: Model Development
Download PDF (324.4 KB)Appendix B: Seasonality and Model Fit
Download PDF (102.9 KB)Acknowledgements
Thank you to Elizabeth Koebele and Galen Treuer who aggregated and analyzed the media attention data with the lead author as part of a project funded by National Socio-Environmental Synthesis Center (NSF DBI-1052875). Thank you to Kent Portney, Avery Cohn, and Murugesu Sivapalan for your feedback.
Disclosure statement
No potential conflict of interest was reported by the authors.
SUPPLEMENTAL DATA
Supplemental data for this article can be accessed at here
Additional information
Funding
References
- Abdallah, A. M., and D. E. Rosenberg. 2014. “Heterogeneous Residential Water and Energy Linkages and Implications for Conservation and Management.” Journal of Water Resources Planning and Management 140 (March): 288–297. doi:10.1061/(ASCE)WR.1943-5452.0000340.
- Ahmad, S., and D. Prashar. 2010. “Evaluating Municipal Water Conservation Policies Using a Dynamic Simulation Model.” Water Resources Management 24 (13): 3371–3395. doi:10.1007/s11269-010-9611-2.
- Ashoori, N., D. A. Dzombak, and M. J. Small. 2016. “Modeling the Effects of Conservation, Demographics, Price, and Climate on Urban Water Demand in Los Angeles, California.” Water Resources Management. doi:10.1007/s11269-016-1483-7.
- Balling, R. C., P. Gober, and N. Jones. 2008. “Sensitivity of Residential Water Consumption to Variations in Climate: An Intraurban Analysis of Phoenix, Arizona.” Water Resources Research 44 (10): 1–11. doi:10.1029/2007WR006722.
- Beal, C. D., R. A. Stewart, and K. Fielding. 2013. “A Novel Mixed Method Smart Metering Approach to Reconciling Differences between Perceived and Actual Residential End Use Water Consumption.” Journal of Cleaner Production 60 Elsevier Ltd: 116–128. doi:10.1016/j.jclepro.2011.09.007.
- Bennett, D. 2014. “Conservation Loves a Crisis: Lessons Learnd in North America’s Driest City.” In Utah Water Conservation Forum. http://utahwaterconservationforum.org/presentations.htm
- Billings, R. B., and C. V. Jones. 2008. “Introduction to Water Forecasting.” In Forecasting Urban Water Demand, edited by M. Valentine (chapter 1), 2nd ed, 1–16. Denver, CO: American Water Works Association.
- Bokulich, A. 2013. “Explanatory Models versus Predictive Models: Reduced Complexity Modeling in Geomorphology.” In EPSA11 Perspectives and Foundational Problems in Philosophy of Science, edited by V. Karakostas and D. Dieks, 115–128. Cham: Springer International Publishing. doi:10.1007/978-3-319-01306_10.
- Booker, J. F., R. E. Howitt, A. M. Michelsen, and R. A. Young. 2012. “Economics and the Modeling of Water Resources and Policies.” Natural Resource Modeling 25 (1): 168–218. doi:10.1111/j.1939-7445.2011.00105.x.
- Bordalo, P., N. Gennaioli, and A. Shleifer. 2012. “Salience Theory of Chocie under Risk.” Quarterly Journal of Economics 127 (3): 1243–1285. doi:10.1093/qje/qjs018.Advance.
- Brelsford, C., and J. K. Abbott. 2017. “Growing into Water Conservation? Decomposing the Drivers of Reduced Water Consumption in Las Vegas, NV.” Ecological Economics 133 The Authors: 99–110. doi:10.1016/j.ecolecon.2016.10.012.
- Bureau of Reclaimation. 2012. Colorado River Accounting and Water Use Report: Arizona, California and Nevada. Boulder City, NV: U.S. Bureau of Reclamation. https://www.usbr.gov/lc/region/g4000/wtracct.html
- Cahill, R., and J. Lund. 2013. “Residential Water Conservation in Australia and California.” Journal of Water Resources Planning and Management 139: 117–121. doi:10.1061/(ASCE)WR.1943-5452.0000225.
- Cavanaugh, J. E., and A. A. Neath. 2011. “Akaike’s Information Criterion: Background, Derivation, Properties, and Refinements.” In International Encyclopedia of Statistical Science, edited by M. Lovric, 26–29. Berlin: Springer.
- Christensen, N. S., and D. P. Lettenmaier. 2007. “A Multimodel Ensemble Approach to Assessment of Climate Change Impacts on the Hydrology and Water Resources of the Colorado River Basin.” Hydrology and Earth System Sciences 11 (4): 1417–1434. doi:10.5194/hess-11-1417-2007.
- Clark County. 2014. “Historical Population and Housing Data.” http://www.clarkcountynv.gov/comprehensive-planning/demographics/Pages/default.aspx
- Cooley, H., T. Hutchins-Cabibi, M. Cohen, P. Gleick, and M. Heberger. 2007. Water Conservation and Efficiency In Hidden Oasis : Water Conservation and Efficiency in Las Vegas (Chapter 4), edited by I. Hart, 16–35. Pacific Institute. http://pacinst.org/publication/hidden-oasis-water-conservation-and-efficiency-in-las-vegas/
- Coomes, P. A., T. D. Rockaway, J. Rivard, and B. Kornstein. 2010. North America Residential Water Usage Trends Since 1992. US EPA. Washington, DC: Water Research Foundation. http://www.waterrf.org/PublicReportLibrary/4031.pdf
- Dalhuisen, J. M., R. J. G. M. Florax, H. L. F. de Groot, and P. Nijkamp. 2003. “Price and Income Elasticities of Residential Water Demand: A Meta-Analysis.” Land Economics 79 (2): 292–308. doi:10.2307/3146872.
- Dessaint, O., and A. Matray. 2015. “Do Managers Overreact to Salient Risks? Evidence from Hurricane Strikes.” SSRN Electronic Journal 1–63. doi:10.2139/ssrn.2358186.
- Dunne, T., and L. Leopold. 1978. Water in Environmental Planning. New York, NY: W.H. Freeman and Co.
- Evans, J. M., J. Calabria, T. Borisova, D. E. Boellstorf, N. Sochacka, M. D. Smolen, R. L. Mahler, and L. M. Risse. 2015. “Effects of Local Drought Condition on Public Opinions about Water Supply and Future Climate Change.” Climatic Change 132 (2): 193–207. doi:10.1007/s10584-015-1425-z.
- Fielding, K. S., S. Russell, A. Spinks, and A. Mankad. 2012. “Determinants of Household Water Conservation: The Role of Demographic, Infrastructure, Behavior, and Psychosocial Variables.” Water Resources Research 48 (10). doi:10.1029/2012WR012398.
- Franczyk, J., and H. Chang. 2009. “Spatial Analysis of Water Use in Oregon, USA, 1985-2005.” Water Resources Management 23 (4): 755–774. doi:10.1007/s11269-008-9298-9.
- Garcia, M., K. Portney, and S. Islam. 2016. “A Question Driven Socio-Hydrological Modeling Process.” Hydrology and Earth System Sciences 73–92. doi:10.5194/hess-20-73-2016.
- Gilg, A., and S. Barr. 2006. “Behavioural Attitudes Towards Water Saving? Evidence from a Study of Environmental Actions.” Ecological Economics 57 (3): 400–414. doi:10.1016/j.ecolecon.2005.04.010.
- Gonzales, P., and N. Ajami. 2017. “Social and Structural Patterns of Drought-Related Water Conservation and Rebound.” Water Resources Research 1–38. doi:10.1002/2017WR021852.
- Gowda, P. H., D. J. Mulla, E. D. Desmond, A. D. Ward, and D. N. Moriasi, Pesticide Transport. 2012. “ADAPT: Model Use, Calibration and Validation.” Transactions of the ASABE 55 (4): 1345–1352.doi:10.13031/2013.42246.
- Griffin, R. C. 2006. Water Resource Economics: The Analysis of Scarcity, Policies, and Projects. Cambridge, MA: MIT Press.
- Hand, M. S., M. J. Wibbenmeyer, D. E. Calkin, and M. P. Thompson. 2015. “Risk Preferences, Probability Weighting, and Strategy Tradeoffs in Wildfire Management.” Risk Analysis 35 (10): 1876–1891. doi:10.1111/risa.12457.
- Harou, J. J., M. Pulido-Velazquez, D. E. Rosenberg, J. Medellín-Azuara, J. R. Lund, and R. E. Howitt. 2009. “Hydro-Economic Models: Concepts, Design, Applications, and Future Prospects.” Journal of Hydrology 375 (3–4) Elsevier B.V.: 627–643. doi:10.1016/j.jhydrol.2009.06.037.
- Hester, C. M., and K. L. Larson. 2016. “Time-Series Analysis of Water Demands in Three North Carolina Cities.” Journal of Water Resources Planning and Management, April. 05016005. doi:10.1061/(ASCE)WR.1943-5452.0000659.
- Hughes, S., S. Pincetl, and C. Boone. 2013. “Triple Exposure: Regulatory, Climatic, and Political Drivers of Water Management Changes in the City of Los Angeles.” Cities 32 Elsevier Ltd: 51–59. doi:10.1016/j.cities.2013.02.007.
- Inman, D., and P. Jeffrey. 2006. “A Review of Residential Water Conservation Tool Performance and Influences on Implementation Effectiveness.” Urban Water Journal 3 (3): 127–143. doi:10.1080/15730620600961288.
- InterNACHI. 2016. “InterNACHI’s Standard Estimated Life Expectancy Chart for Homes.” https://www.nachi.org/life-expectancy.htm
- Jones, N., K. Evangelinos, P. Gaganis, and E. Polyzou. 2011. “Citizens’ Perceptions on Water Conservation Policies and the Role of Social Capital.” Water Resources Management 25 (2): 509–522. doi:10.1007/s11269-010-9711-z.
- Jorgensen, B., M. Graymore, and K. O’Toole. 2009. “Household Water Use Behavior: An Integrated Model.” Journal of Environmental Management 91 (1) Elsevier Ltd: 227–236. doi:10.1016/j.jenvman.2009.08.009.
- Kenney, D. S., C. Goemans, R. Klein, J. Lowrey, and K. Reidy. 2008. “Residential Water Demand Management: Lessons from Aurora, Colorado.” Journal of the American Water Resources Association 44 (1): 192–207. doi:10.1111/j.1752-1688.2007.00147.x.
- King, J. 2014. Las Vegas Valley (Hydrographic Basin 13-212) Groundwater Pumping Inventory.Carson City, NV: State of Nevada Department of Conservation and Natural Resources, Division of Water Resources.
- Koutiva, I., P. Gerakopoulou, C. Makropoulos, and C. Vernardakis. 2017. “Exploration of Domestic Water Demand Attitudes Using Qualitative and Quantitative Social Research Methods.” Urban Water Journal 14 (3) Taylor & Francis: 307–314. doi:10.1080/1573062X.2015.1135968.
- Mini, C., T. S. Hogue, and S. Pincetl. 2015. “The Effectiveness of Water Conservation Measures on Summer Residential Water Use in Los Angeles, California.” Resources, Conservation and Recycling 94 Elsevier B.V.: 136–145. doi:10.1016/j.resconrec.2014.10.005.
- Mini, C., T. S. Hogue, and S. Pincetl. 2014. “Patterns and Controlling Factors of Residential Water Use in Los Angeles, California.” Water Policy 1–16. doi:10.2166/wp.2014.029.
- Moriasi, D. N., J. G. Arnold, M. W. Van Liew, R. L. Bingner, R. D. Harmel, and T. L. Veith. 2007. “Model Evaluation Guidelines for Systematic Quantification of Accuracy in Watershed Simulations.” Transactions of the ASABE 50 (3): 885–900. doi:10.13031/2013.23153.
- Nash, J. E., and B. I. Barsi. 1983. “A Hybrid Model for Flow Forecasting on Large Catchments.” Journal of Hydrology 65 (1–3): 125–137. doi:10.1016/0022-1694(83)90213-5.
- Nevada State Legislature. 2015. “Minimal Standards for Plumbing Fixtures in Certain Structures.” Nevada Revised Statues. http://www.leg.state.nv.us/NRS/NRS-278.html#NRS278Sec582
- Noel, J. E., and R. E. Howitt. 1982. “Conjunctive Multibasin Management: An Optimal Control Approach.” Water Resources Research 18 (4): 753–763. doi:10.1029/WR018i004p00753.
- Olmstead, S. M., W. M. Hanemann, and R. N. Stavins. 2007. “Water Demand under Alternative Price Structures.” Journal of Environmental Economics and Management 54 (2): 181–198. doi:10.1016/j.jeem.2007.03.002.
- Perrone, D., G. Hornberger, O. van Vliet, and V. D. V. Marijn. 2015. “A Review of the United States’ past and Projected Water Use.” Journal of the American Water Resources Association 51 (5): 1183–1191. doi:10.1111/1752-1688.12301.
- Quesnel, K. J., and N. K. Ajami. 2017. “Changes in Water Consumption Linked to Heavy News Media Coverage of Extreme Climatic Events.” Science Advances, 3 (October): 1–10.
- Rahmandad, H., and J. Sterman. 2004. “Heterogeneity and Network Structure in the Dynamics of Diffusion: Comparing Agent-Based and Differential Equation Models.” SSRN Electronic Journal 1–32. doi:10.2139/ssrn.607302.
- Randall, A. 1981. “Property Entitlements and Pricing Policies for a Maturing Water Economy.” The Australian Journal of Agricultural Economics 25 (3): 195–220. doi:10.1111/j.1467-8489.1981.tb00398.x.
- Russell, S., and K. Fielding. 2010. “Water Demand Management Research: A Psychological Perspective.” Water Resources Research 46 (5): 1–12. doi:10.1029/2009WR008408.
- Salvaggio, M., R. Futrell, C. D. Batson, and B. G. Brents. 2014. “Water Scarcity in the Desert Metropolis: How Environmental Values, Knowledge and Concern Affect Las Vegas Residents’ Support for Water Conservation Policy.” Journal of Environmental Planning and Management 57 (November 2013): 588–611. doi:10.1080/09640568.2012.756806.
- Schleich, J., and T. Hillenbrand. 2009. “Determinants of Residential Water Demand in Germany.” Ecological Economics 68 (6) Elsevier B.V.: 1756–1769. doi:10.1016/j.ecolecon.2008.11.012.
- Scott, G. E., J. B. Erickson, and B. A. Buttram. 1983. “Attempts to Promote Residential Water Conservation with Educational, Behavioral and Engineering Strategies.” Population and Environment 6 (2): 96–112. doi:10.1007/BF01362290.
- Sivapalan, M., G. Bloschl, L. Zhang, and R. Vertessy. 2003. “Downward Approach to Hydrological Prediction.” Hydrological Processes 17 (11): 2101–2111. doi:10.1002/hyp.1425.
- SNWA. 2005a. Drought Plan. Las Vegas, NV: Southern Nevada Water Authority (SNWA).
- SNWA. 2005b. Integrated Water Planning Advisory Committee Recommendations Report. Las Vegas, NV: Southern Nevada Water Authority (SNWA).
- SNWA. 2009a. Water Resources Management Plan. Las Vegas, NV: Southern Nevada Water Authority (SNWA).
- SNWA. 2009b. Conservation Plan: 2009-2013. Las Vegas, NV: Southern Nevada Water Authority (SNWA).
- SNWA. 2013. Integrated Resource Planning Advisory Committee Recommendations Report, Phase I: Funding Sources. Las Vegas, NV: Southern Nevada Water Authority (SNWA). https://www.snwa.com/assets/pdf/ws_irpac_recommendations_final.pdf
- Sovocool, K. A., M. Morgan, and D. Bennett. 2006. “An In-Depth Investigation of Xeriscape as a Water Conservation Measure.” Journal American Water Works Association 98 (2): 82–93. doi:10.1002/j.1551-8833.2006.tb07590.x.
- Srinivasan, V., M. Sanderson, M. Garcia, M. Konar, G. Blöschl, M. Sivapalan, M. Sanderson, et al. 2016. “Prediction in a Socio-Hydrological World.” Hydrological Sciences Journal – Journal Des Sciences Hydrologiques Taylor & Francis: 1–8.doi:10.1080/02626667.2016.1253844.
- Stirzaker, R., H. Biggs, D. Roux, and P. Cilliers. 2010. “Requisite Simplicities to Help Negotiate Complex Problems.” Ambio 39 (8): 600–607. doi:10.1007/s13280-010-0075-7.
- Stoutenborough, J. W., and A. Vedlitz. 2014. “Public Attitudes toward Water Management and Drought in the United States.” Water Resources Management 1–18. doi:10.1007/s11269-013-0509-7.
- Suero, F. J., P. W. Mayer, and D. E. Rosenberg. 2012. “Estimating and Verifying United States Households’ Potential to Conserve Water.” Journal of Water Resources Planning and Management 138 (3): 299–306. doi:10.1061/(ASCE)WR.1943-5452.0000182.
- Treuer, G., E. Koebele, A. Deslatte, K. Ernst, M. Garcia, and K. Manago. 2017. “A Narrative Method for Analyzing Transitions in Urban Water Management: The Case of the Miami-Dade Water and Sewer Department.” Water Resources Research 53 (1): 891–908. doi:10.1002/2016WR019658.
- U.S. General Accounting Office. 2000. Water Infrastructure: Water-Efficient Plumbing Fixtures Reduce Water Consumption and Wastewater Flows. Washington D.C, U.S: General Accounting Office, Resources, Community, and Economic Development Division. http://www.gao.gov/new.items/rc00232.pdf
- US Census. 2000a. “General Population and Housing Characteristics.” American Fact Finder. https://factfinder.census.gov/faces/tableservices/jsf/pages/productview.xhtml?src=CF
- US Census. 2000b. “Selected Economic Characteristics.” American Fact Finder. https://factfinder.census.gov/faces/tableservices/jsf/pages/productview.xhtml?src=CF
- US Census. 2010a. “General Demographic Characteristics.” American Fact Finder. https://factfinder.census.gov/faces/tableservices/jsf/pages/productview.xhtml?src=CF
- US Census. 2010b. “Selected Economic Characteristics.” American Fact Finder. https://factfinder.census.gov/faces/tableservices/jsf/pages/productview.xhtml?src=CF
- USGS. 2016. “National Land Cover Database.” http://www.mrlc.gov/finddata.php
- Wentz, E. A., and P. Gober. 2007. “Determinants of Small-Area Water Consumption for the City of Phoenix, Arizona.” Water Resources Management 21 (11): 1849–1863. doi:10.1007/s11269-006-9133-0.
- Willis, R. M., R. A. Stewart, D. P. Giurco, M. R. Talebpour, and A. Mousavinejad. 2013. “End Use Water Consumption in Households: Impact of Socio-Demographic Factors and Efficient Devices.” Journal of Cleaner Production 60 Elsevier Ltd: 107–115. doi:10.1016/j.jclepro.2011.08.006.
- Zhang, Q., Y. Diao, and J. Dong. 2013. “Regional Water Demand Prediction and Analysis Based on Cobb-Douglas Model.” Water Resources Management 27 (8): 3103–3113. doi:10.1007/s11269-013-0335-y.