Abstract
The launch of the Japanese Advanced Himawari Imager (AHI) on 7 October 2014 represents a new era of geostationary operational environmental satellite (GOES) imagers, providing many more channels than any previously launched GOES imagers for the first time. In this study, we compare the impacts of assimilating all AHI versus GOES-like infrared channels radiances on regional forecasts over Eastern China. The National Centers for Environmental Prediction (NCEP) Gridpoint Statistical Interpolation (GSI) analysis system and Advanced Research Weather Research and Forecast model are employed. Positive impacts are obtained on quantitative precipitation forecasts (QPFs) associated with a typical summer precipitation case over eastern China in both set-ups, i.e. one assimilating all 10 AHI infrared channels (AHIA) and the other assimilating only four GOES-like AHI channels (AHIG). It is found that a southwest to northeast oriented band of the atmosphere with high water vapor content that was formed and moved inland with time under the influence of a subtropical high and an eastward-propagating middle-latitude trough was responsible for the persistent precipitation in the eastern China of the selected case. The AHIA experiment generated the largest improvement on QPFs due to it generating a wetter atmosphere in the middle and low troposphere over the ocean off the southeast coast of China than the AHIG experiment and a control experiment without assimilating any AHI channel.
1. Introduction
In the past two decades, the United States geostationary operational environmental satellite program (GOES) observations were not only applied to nowcasting (Velden, Citation1996; Velden et al., Citation1997, Citation1998; Mecikalski and Bedka, Citation2006; Mecikalski et al., Citation2015; Gravelle et al., Citation2016), but also extended to regional numerical weather prediction. For example, positive impacts of the GOES radiance assimilation on regional weather forecasts were demonstrated by Köpken et al. (Citation2004) and Okamoto (Citation2013) for the Meteosat Visible and Infrared Imager (MVIRI); Szyndel et al. (Citation2005), Guedj et al. (Citation2011) and Stengel et al. (Citation2009) for the Spinning Enhanced Visible and Infrared Imager (SEVIRI) on board Meteosat-8, Zou et al. (Citation2011, Citation2015) and Qin et al. (Citation2013) for the GOES imagers on board the United States GOES-11, -12, -13 and -15.
On 7 October 2014, the Japan Meteorological Agency (JMA) successfully launched a Geostationary Meteorological Satellite (GMS) – Himawari-8. It carries the newest generation of the geostationary visible and infrared imager – the Advanced Himawari Imager (AHI). The AHI observes the earth atmosphere with more visible, near-infrared, water vapor infrared and surface-sensitive infrared channels than JMA’s Multi-functional Transport SATellites (MTSAT) and the US GOES imagers. The same AHI was also on board on Himawari-9, which was launched on 2 November 2016. The AHIs on board Himawari-8 and -9 are expected to provide the imagery observations with higher temporal and horizontal resolutions to continually support the weather forecasts, natural-disaster counter-measures in the Western Pacific and Asia-oceanic regions. The AHI onboard Himawari-8 and -9 inherit those channels similar to the previously launched GOES imagers in the United States, which has only four infrared channels. It is of interest to examine the benefits for AHI to have six more infrared channels than GOES imagers.
Since Himawari-8 is positioned nominally at 140.7°E and perched at 35,800 km above the equator, a typical summer precipitation case over eastern China is selected for such a study. Specifically, we compare the added benefits of directly assimilating all 10 AHI infrared channels than only four GOES-like infrared channels radiance data for the quantitative precipitation forecasts (QPFs) over eastern China. The data assimilation and forecast model used are the National Centers for Environmental Prediction (NCEP) Gridpoint Statistical Interpolation (GSI) analysis system (Wu et al., Citation2002; Purser et al., Citation2003a, Citation2003b) and the Advanced Research Weather Research and Forecasting (WRF) (ARW) model, respectively. These systems were made publically accessible for numerical weather prediction (NWP) user community (Shao et al., Citation2016). Section 2 provides a brief description of AHI data characteristics, case selection and experiment design. Details on cloud detection, bias correction, data thinning, quality control, convergence and analysis increments are presented in Section 3. Section 4 shows the forecast results demonstrating the benefits of assimilating all 10 AHI infrared channels radiance data over only four GOES-like infrared channels on the QPFs from 12 to 48 h. Summary and conclusions are provided in Section 6.
2. Case selection and experiment design
2.1. AHI infrared channel characteristics
The AHI is a geostationary satellite imager that has 16 channels for the first time. The 10 infrared channels (7–16) are particularly important for NWP. Channel 7 is located at the shortwave region centred at 3.85 µm. Channels 8, 9 and 10 are centred at 6.25, 6.95 and 7.35 µm, respectively, for sensing the atmospheric water vapor. Channels 11, 13, 14 and 15 are at 8.6, 10.45, 11.20 and 12.35 µm. Channel 16 has a central wavelength at 13.3 µm that is located near the atmospheric CO2 absorption line at 15 µm. Channel 12 is located at 9.63 µm that is sensitive to tropospheric ozone but is not considered in this study, because its weight function has a second peak at about 30 hPa that is higher than the model top (50 hPa). Figure shows the weighting function profiles for the nine infrared channels 7–11 and 13–16, overlapped onto the 43 model pressure levels from the surface to 50 hPa (see Section 2.2). It is seen that the three AHI water vapor channels are strongly affected by the water vapor in the middle and upper troposphere. The remaining six infrared channels are located near the earth surface. Out of these six surface sensitive infrared channels, channel 16 is affected by the temperature in the low and middle troposphere the mostly.
Fig. 1. Weighting functions for the nine infrared channels 7–16. The 43 WRF–ARW model levels from surface to 50 hPa are shown in grey horizontal lines and the layer thicknesses are shown by black dashed curve.
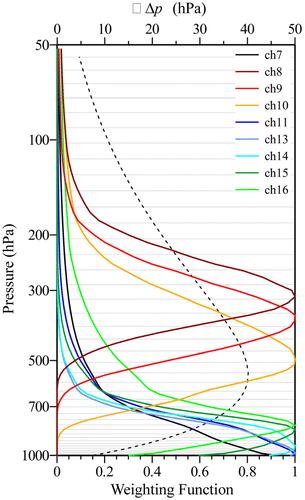
The spatial resolution, i.e. an instantaneous geometric field of view, of all AHI infrared channels is 2 km at the sub-satellite point. It was pointed out by Heidinger (Citation2011) that a consistent 2-km spatial resolution for all AHI infrared channels is beneficially important for deriving AHI multi-channels retrieval products based on spectral differences such as the cloud mask (CM) test algorithms. This consistency in resolution among AHI infrared channels is also good for effective cloud detection, quality control and data assimilation.
2.2. Experiment design
The case selected for this study is characterized by a typical summer precipitation case that occurred downstream of an eastward propagating middle latitude trough. The observed amounts of 12-h accumulative rainfall over land are provided in Fig. for the time period from 1200 UTC 2 July to 0000 UTC 4 July 2016. The rainfall observations were obtained from merging the hourly rain gauge data at more than 30,000 automatic weather stations in China with the Climate Precipitation Center Morphing (CMORPH) precipitation product using a probability density function based optimal interpolation method (Shen et al., Citation2014). To associate the rainfall distributions with large-scale flow features, the 500-hPa geopotential heights from the NCEP FNL at 0000 UTC 3 July (Fig. a), 1200 UTC 3 July (Fig. b) and 0000 UTC 4 July (Fig. c) 2016 are added to Fig. . A southwest to northeasterly oriented heavy precipitation band persisted for over 36 h. A continuous supply of water vapor to this area by advection is thus important for an NWP model to perform well on QPFs.
Fig. 2. The observations of 12-h accumulative rainfall amounts over land (shaded) ending at and the 500 hPa geopotential height from NCEP FNL at (a) 0000, (b) 1200 and (c) 2400 UTC 3 July 2016 (contour interval: 10 m). The dashed rectangle shows the validation area.
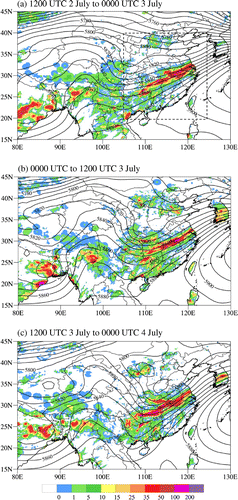
Three data assimilation and forecast experiments were carried out. The control experiment (CTRL) assimilates all the conventional radiosonde and surface observations. The conventional observations consist of the surface and upper-air reports operationally collected by NCEP, including land surface, marine surface, radiosonde and aircraft reports from the Global Telecommunications System, US radar-derived and profiler winds, Special Sensor Microwave Imager oceanic winds and atmospheric total column precipitable water retrievals, as well as satellite wind data from the National Environmental Satellite, Data, and Information Service (NESDIS) of National Oceanic and Atmospheric Administration (NOAA). In the second experiment (AHIA), the AHI infrared channels 7–16 are assimilated along with those data assimilated in CTRL. The third experiment (AHIG) is the same as AHIA except for taken away the two AHI water vapor channels 8 and 10 and four AHI surface sensitive channels 11, 12, 13 and 15. The model domain is shown in Fig. a in which the brightness temperature observations of AHI channel 16 at 0000 UTC 1 July 2016 is plotted. Areas of clouds and precipitation are characterized by low brightness temperatures of AHI channel 16 whose central frequency is located at 13.3 μm.
Fig. 3. (a) Spatial distribution of brightness temperature observations of AHI channel 16 at 0000 UTC 1 July 2016 within the model domain. (b) Schematic illustration of the data assimilation cycling from 0000 UTC 1 July to 0000 UTC 2 July 2017 and the forecast experiments initialized at 0000 UTC 2 July 2017.
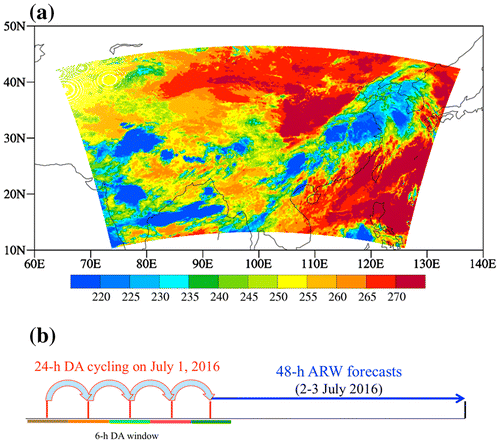
The AHI data assimilation experiments are carried out using the NCEP GSI system version 3.3 (Shao et al., Citation2016). The Community Radiative Transfer Model (CRTM) (Han et al., Citation2007; Weng, Citation2007) is employed in the GSI system as the observation operator for satellite data assimilation. A one-day data assimilation length with a 6-h cycling interval is chosen (Fig. b). The data assimilation cycling is executed as follows: The first data assimilation minimization is carried out at 0000 UTC 1 July 2016 using the NCEP Global Forecast System (GFS) output as the background field (). Once the analysis (
) at 0000 UTC 1 July 2016 is obtained by running the GSI data assimilation system, the Advanced Research WRF (ARW) is used to obtain a 6-h forecast (i.e. the first data assimilation cycle). This 6-h ARW model forecast is used as the background field for the data assimilation carried out at 0600 UTC 1 July 2016, and the remaining procedures are the same as the first cycle. A total of five such data assimilation cycles are carried out continuously until the analysis at 0000 UTC 2 July 2016 is obtained.
The Advanced Research Weather Forecasting (WRF) model (ARW) version 3.2 is used for the 48-h QPFs starting at 0000 UTC 2 July 2016. The horizontal model resolution is 15 km. There are 43 vertical levels from the Earth surface to a model top located around 50 hPa. The total model grid points in the three-dimensional (3D) space are 400 × 250 × 43. The WRF single-moment three-class microphysics scheme (Hong and Lim, Citation2006), the Kain–Fritsch cumulus parameterization scheme (Kain and Fritsch, Citation1990, Citation1993; Kain, Citation2004), and the Yonsei planetary boundary layer scheme (Hong and Dudhia, Citation2003) are used in the ARW.
3. Data assimilation
3.1. Cloud detection
The AHI observations in clear-sky conditions are assimilated. For this purpose, an infrared only CM algorithm that was developed and tested by Zhuge and Zou (Citation2016) is used to identify cloud-contaminated AHI pixels. The infrared-only CM algorithm consists of the following 10 CM tests: a new CM test (i.e. the New Optically Thin Cloud test – NOTC) for optically thin clouds, two modified Advanced Baseline Imager (ABI) CM tests (4 μm Emissivity test – EMISS4, Modified Uniform Low Stratus test – ULST) and seven other ABI CM tests (Relative Thermal Contrast Test – RTCT, Emissivity at Tropopause test – ETROP, Positive Fourteen Minus Fifteen Test – PFMFT, Negative Fourteen Minus Fifteen Test – NFMFT, Relative Fourteen Minus Fifteen Test – RFMFT, Cirrus Water Vapor test – CIRH2O, Temporal Infrared test – TEMPIR). A brief description of physical considerations and mathematical formulations for each of these 11 CM tests was provided in Zhuge and Zou (Citation2016). Validated by the Moderate Resolution Imaging Spectroradiometer (MODIS) CMs, this infrared only AHI cloud detection algorithm achieved a high Probability of Correct Typing (PCT) and a lower false alarm rate.
Figure shows a spatial distribution of cloudy pixels detected by the 11 channel infrared-only CM algorithm (Fig. a) and the CM obtained by the clouds from the AVHRR Extended System (CLAVR-x) (Fig. b) at 2300 UTC 1 July 2016. The CLAVR-x uses not only the infrared channels but also visible and near-infrared channels (Heidinger et al., Citation2012). It is seen that the CMs generated by the 11 CM tests in the infrared only CM algorithm compares favourably with the CLAVR-x CM, as was demonstrated for other cases in Zhuge and Zou (Citation2016).
3.2. Quality control
The AHI data assimilation was not yet included in the GSI version 3.3. We added the capability for AHI data assimilation to GSI. Specifically, the AHI data in clear-sky conditions are selected at the 2-km observation resolution by applying the cloud detection algorithm of Zhuge and Zou (Citation2016). They are then put into the Binary Universal Form for the Representation of meteorological data (BUFR) that was maintained by the World Meteorological Organization (WMO) (Dragosavac, Citation2007), and finally inputted into the GSI analysis system. For a 15-km model resolution to be used in our numerical experiments, the AHI data are thinned to a spatial resolution of 75 km to reduce the representative errors. The data biases (μi) and observation error statistics () required by the AHI data assimilation, where the subscript ‘i’ indicates the channel number, are calculated following the same method as Zou et al. (Citation2016). The observation weights (wi, i = 7, …, 11, 13, …, 16) is firstly assigned as the inverse of the observation error (
). For channels 8–10, the observation weight is multiplied by a terrain factor fH = 2000/H if the terrain height H is higher than 2000 m.
The scaled observation weights () are then modified according to surface conditions defined by surface emissivity (ɛi) and surface air temperature (Ts). Specifically,
is divided by the following factor
where fɛ is set to 0.01 over sea and land and 0.02 over other surface types, and fT is equal to 0.5 over sea, 2.0 over land, 3.0 over ice or snow and 5.0 over other types of surface. The observations weights over land vary greatly with the surface type and the surface temperature. Currently, only the AHI channels 7, 11, 13–16 over sea, and channels 8–10 over both sea and land radiance observations are assimilated in the GSI system. Observations over ice, snow and other surfaces are excluded from assimilation. Finally, a quality control is applied to the AHI channels 7, 11, 13–16 over sea, and channels 8–10 over both sea and land. An AHI observation is rejected from assimilation if the difference of brightness temperature between observation (O) and model simulation (B) is larger than 2 K or 3σobs.
3.3. Mode fit to observations and analysis increments
The mean and standard deviation of the differences between AHI observations and model simulations before and after data assimilation in the AHIA experiment are presented in Fig. . All data assimilated during the one-day cycling period are included. The data counts assimilated for different channels are provided in Fig. b. Since water vapor channels 8–10 are assimilated over both land and ocean, the data counts for these three channels are in general larger than the other surface sensitive channels which are assimilated only over ocean. Also, the weighting function leak of channel 8 is the highest among channels 8–10, the data count for channel 10 is the largest since fewer pixels are affected by the residual cloud effects. Before data assimilation, channels 7, 10–11 and 13–16 have negative biases with their magnitude varying from −0.2 to −0.8 K, and channels 8 and 9 have a positive bias around 0.3 and 0.1 K, respectively (Fig. a). The standard deviations are greater than 0.7 K but smaller than 1.1 K before data assimilation (Fig. b). After data assimilation, the mean and standard deviation reduced to below 0.1 and 0.6 K, respectively, for all assimilated AHI infrared channels.
Fig. 5. (a) Mean and (b) standard deviation of the differences between AHI observations and model simulations before (red bars) and after (blue bars) data assimilation in the AHIA experiment. All data assimilated during the one-day cycling period (black circles) are included.
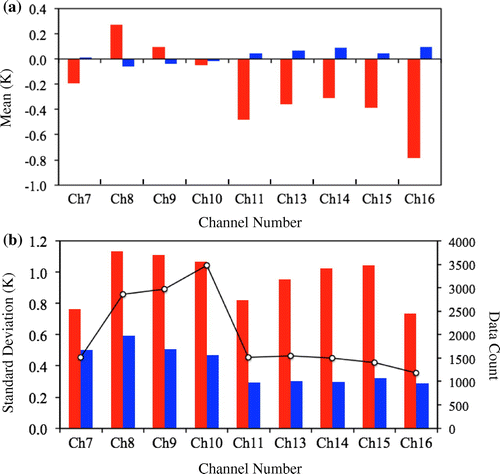
The differences between observations and the background fields (O–B) and those between observations and analysis fields (O–A) at those AHI data points that pass the GSI quality control and are assimilated at 0000 UTC 1 July 2016 in the AHIA experiment are presented for AHI channels 9 and 10 as examples (Fig. ). The O–B differences can be as large as ±1.5 K (Fig. a and b). The O–A differences of channel 9 (Fig. c and d), which is assimilated in both AHIA and AHIG experiments, are significantly smaller than the O–B differences (Fig. a and b). But for channel 10, which is not assimilated in AHIG, the O–A differences from the AHIG experiment (Fig. f) remain as large as ±1.5 K at many data points where channel 9 is assimilated (Fig. b).
Fig. 6. Spatial distribution of (a, b) O–B and (c, f) O–A of channel 9 (left panels) and channel 10 (right panels) from (c, d) AHIA and (e, f) AHIG experiments at 0000 UTC 1 July 2016, which is the beginning time of the AHIA data assimilation cycling. Black dots represent data rejected by QC.
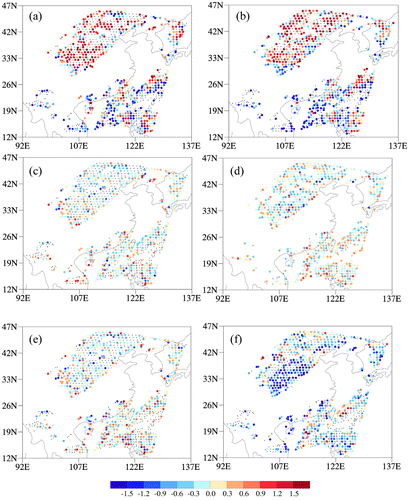
Figure a and b provides the spatial distributions of the analysis and analysis increments of water vapor mixing ratio at the model level σ = 0.743 (~750 hPa) at 0000 UTC 1 July 2016 from the CTRL experiment (Fig. a) and the AHIA experiment (Fig. b). It is seen that the water vapor mixing ratio is significantly increased (~4 g kg−1) off the east coast of China where AHI observations are assimilated. Figure c shows the cross section of the water vapor analysis differences between the AHIA and CTRL experiments (shaded) as well as the AHIA analysis increments (contours) along the 115E longitude at 0000 UTC 1 July 2016. It is seen that the water vapor increments are the largest around 720 hPa and extend below and above this pressure level south of 21 N. The latitudinal and vertical distributions of the largest analysis differences of the water vapor mixing ratio between the AHIG and CTRL experiments as well as the AHIG analysis increments of water vapor mixing ratio (Fig. d) are smaller in both magnitude and the vertical extent than those for AHIA in Fig. c. Due to the missing of two water vapor channels and four surface channels, the maximum of AHIG’s increments decreased from 2.8 to 1.8 in the south of 21 N, the max value centre is located at about 850 hPa, which is lower than AHIA’s. The negative increments in the north of 35 N are also enhanced at about 600 hPa.
Fig. 7. (a, b) Spatial distributions of the analysis of water vapor mixing ratio (black contour), the analysis of wind (vector), and the analysis increments (colour shading) of water vapor mixing ratio (contour interval: 1.0 g kg−1) at σ = 0.743 (~750 hPa) at 0000 UTC 1 July 2016 (i.e. the beginning of the one day DA cycle) from (a) CTRL and (b) AHIA experiments. (c) Cross sections of the water vapor analysis differences between the AHIA and CTRL experiments (colour shading) and the AHIA analysis increments (curve, contour interval: 0.4 g kg−1) along the 115E longitude at 0000 UTC 1 July 2016. (d) Same as (c) except for AHIG.
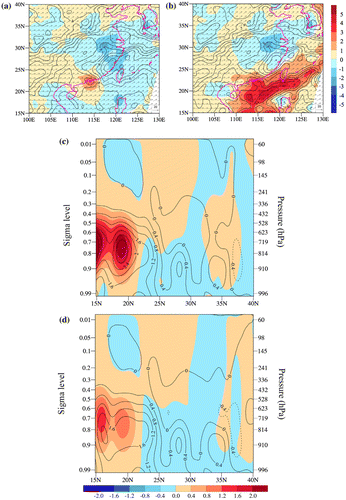
The spatial distributions of the analysis increments of temperature at the model level σ = 0.743 (~750 hPa) at 0000 UTC 1 July 2016 from the CTRL experiment and the AHIA experiment are shown in Figure a and b. It is seen that the temperature is decreased (~0.8 K) off the east coast where water vapor mixing ratio is significantly increased (see Fig. a). Positive analysis increments of temperature around 0.45 K are found in the middle latitudes east of 100E and along the east coast in both experiments, suggesting that they are generated mostly due to radiosonde observations. The cross section of the analysis differences (shaded) between the AHIA and CTRL experiments of atmospheric temperature along the 115E longitude at 0000 UTC 1 July 2016 is presented in Fig. c. Temperature increments in the low latitudes over ocean are negative with the largest magnitude located around 720 hPa and extending below and above this pressure level, another negative increment centre is in the north of 30 N, AHI data assimilation strengthened these negative increments in the north of 35 N at about 620 hPa. Increments higher than 300 hPa are mainly caused by conventional data. Same as the water vapor mixing ratio, the latitudinal and vertical distributions of the largest analysis differences (shaded) of water vapor mixing ratio between the AHIG and CTRL experiments as well as the AHIG analysis increments (contours) of water vapor mixing ratio (Fig. d) are also smaller in both magnitude and the vertical extent than those for AHIA in Fig. c. The most obvious increment differences between AHIA and AHIG is in the south of 21 N, the max value decreases from 0.4 to 0.2 K, but the height of the maximum centre for both of the two experiments is located at about 720 hPa.
4. Forecast impacts
Impacts of the AHI observed information over the ocean off the east coast of China in the low latitudes on the forecasts of the precipitation event in the middle latitudes over land is shown in Fig. . Contours show the temporal evolution of the forecasts of water vapor mixing ratio at σ = 0.743 (~750 hPa) from the AHIA and AHIG experiments, shaded represents the forecast differences between the AHIA and CTRL experiments and between AHIG and CTRL at 0000 UTC and 1200 UTC 2 July and 0000 UTC 3 July 2016. Winds are also shown in vectors. Vertical and latitudinal cross sections for the water vapor mixing ratio forecast of the AHIA experiment and the forecast difference of water vapor mixing ratio between the AHIA and CTRL experiments from 0000 UTC 2 July to 0000 UTC 4 July 2016 are shown in Fig. . It is clearly seen that as the forecast advances in time, the band of the atmosphere that is wetter in the AHIA and AHIG experiments than the CTRL experiment, which is oriented from the southwest to northeast, along the direction of winds, moves northward to supply more water vapor to areas of precipitation (Fig. ). Not only the atmosphere around 750 hPa in the low latitudinal band 15–22 N are wetter in the AHIA and AHIG experiments than those of the CTRL experiment at the initial time, the atmosphere in the middle latitudes around 35 N is also more moist for the AHIA than the CTRL initial conditions (Fig. ). As the forecast time advances, the positive forecast differences of water vapor mixing ratio located initially in the low (middle) latitudes propagate northward (southward), which is favourable for the AHI data assimilation to have an impact on the forecast of a persistent precipitation around 30 N. Again, differences between AHIA and AHIG are mainly reflected in the magnitudes of water vapor mixing ratio.
Fig. 9. Forecasts of water vapor mixing ratio at σ = 0.743 (~750 hPa) from the AHIA (left panels) and AHIG (right panels) experiments (contour interval: 1 g kg−1), as well as the forecast differences between the AHIA and CTRL experiments (colour shading, left panels) and the forecast differences between the AHIG and CTRL experiments (colour shading, right panels) from 0000 UTC 2 July to 0000 UTC 2 July 2016 at 12-h interval.
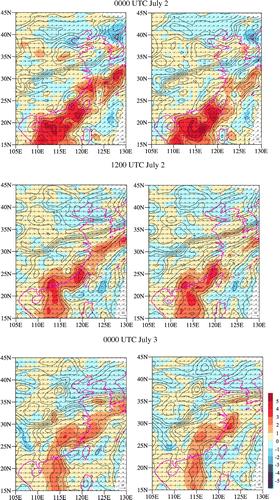
Fig. 10. Vertical and latitudinal cross sections along the 115E longitude for the analysis of water vapor mixing ratio of the AHIA experiment (contour interval: 1 g kg−1) and the forecast differences of water vapor mixing ratio between the AHIA and CONV experiments (AHIA-CTRL, shaded) at (a) 0000 UTC, (b) 1200 UTC and 1800 UTC 2 July 2016. The 3-h accumulative rainfall observations (purple curve) ending at (a) 0000 UTC, (b) 1200 UTC and (c) 1800UTC 2 July 2016 are indicated by the y-axis on the right by a logarithmic scale.
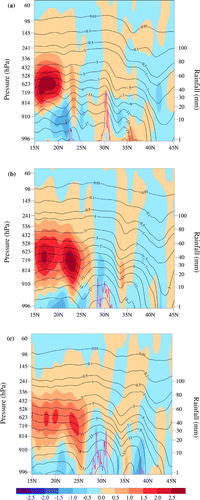
A comparison among the CTRL, AHIG and AHIA experiments can then be made for the QPFs over land. Figure provides the spatial distributions of the 12-h accumulative rainfall amounts over land from the AHIA and AHIG experiments from 1200 UTC 2 July to 0000 UTC 3 July, 0000 UTC 3 July to 1200 UTC 3 July, and 1200 UTC 3 July to 0000 UTC 4 July 2016. The geopotential height at 500 hPa at the ending time of each of the three 12-h intervals is also shown in Fig. . Both of the two experiments can forecast the southwest to northeasterly oriented heavy rain band, but the spatial distributions of the 12-h accumulative rainfall amounts from the AHIA experiment compare more favourably to the observed precipitation distributions (see Fig. ) than those from the AHIG experiment. The rain band of AHIG is a bit of north of the observation, especially for the time period from 0000 to 1200 UTC July 3, observed rain belt lies between 115 and 119E, but the maximum rainfall of AHIG is located in 119–120E, reaches about 200 mm. AHIA reduces the rainfall amounts between 119–120E and 50–100 mm, and more rainfall can be found in the position of observed rain belt for AHIA. At the last 12-h time interval, the middle-latitude trough of AHIA is stronger than that of AHIG, therefore, the rain band of AHIA is slimmer than that of AHIG, and more consist with the observations.
Fig. 11. The 12-h accumulative rainfall amounts over land (colour shading) from the AHIA (left panels) and AHIG (right panels) experiments from 1200 UTC 2 July to 0000 UTC 3 July (top panels), 0000 UTC 3 July to 1200 UTC 3 July (middle panels), and 1200 UTC 3 July to 0000 UTC 4 July (bottom panels) 2016, as well as the geopotential height (black curve) at 500 hPa at the ending time of each of the three 12-h intervals.

The equitable threat scores at the thresholds of 1, 5, 10 and 15 mm during the 12–48 h forecast times, which corresponds to a time period from 1200 UTC 2 July to 0000 UTC 4 July 2016, are shown in Fig. . It is seen that the equitable threat scores of both the AHIG and AHIA experiments are consistently higher than those from the CTRL experiment throughout the forecast time period from 12 to 48 h. The AHIA experiment gives the highest overall scores, especially for the QPFs at higher thresholds.
Fig. 12. Equitable threat scores (ETS) for the 3-h accumulative rainfall amounts over land at the thresholds of 1, 5, 10 and 15 mm during the 12–48 h forecasts, which are initialized at 0000 UTC 2 July 2016, from the CONV (black bars), AHIG (grey bars) and AHIA (blue bars) experiments from 1200 UTC 2 July to 0000 UTC 4 July 2016.
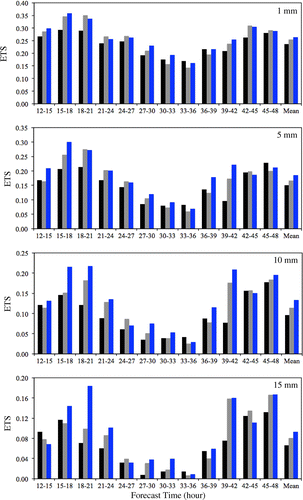
5. Summary and conclusions
Through a case study, we demonstrate added benefits for assimilation of more infrared channels from AHI to improve QPFs over eastern China. Three experiments (CTRL, AHIA and AHIG) are conducted to evaluate the impact of AHI data assimilation, especially for those newly added two water vapor channels and four surface channels. The AHIA experiment generated the largest improvement on QPFs due to it generating a wetter atmosphere in the middle and low troposphere over the ocean off the southeast coast of China. The preliminary results highlight the potential benefits of those newly added channels. The GOES-R ABI was launched on 19 November 2016, and the ABI data will soon be made available to NWP communities. ABI has higher resolutions and five times faster scans of the Earth than the current operational GOES satellites, GOES-13 and -15. The only major difference between ABI and AHI is that ABI has one less visible channel than AHI. This has no impact on data assimilation of ABI infrared channels. Therefore, the work completed in this study for AHI data assimilation will allow an accelerated implementation of GOES-R ABI data assimilation in the GSI system be accelerated once the ABI data become available.
Disclosure statement
No potential conflict of interest was reported by the authors.
Funding
This research was jointly supported by the National Oceanic and Atmospheric Administration (NOAA) GOES-R Program [project number NA14NES4320003], the National Natural Science Foundation of China [grant number 91337218], [grant number 41475103], and the Special Fund for Meteorological Research in the Public Interest of China [project number GYHY201406008].
Acknowledgements
The views and opinions contained in this paper reflect those of us and should not be construed as an official National Oceanic and Atmospheric Administration or U. S. Government position, policy, or decision.
References
- Dragosavac, M. 2007. BUFR User’s Guide. ECMWF Technical note. Online at: http://www.wmo.int/pages/prog/gcos/documents/gruanmanuals/ECMWF/bufr_user_guide.pdf (last accessed 8 August 2014).
- Gravelle, C. M., Mecikalski, J. R., Line, W. E., Bedka, K. M., Petersen, R. A. and co-authors. 2016. Demonstration of a GOES-R satellite convective toolkit to ‘bridge the gap’ between severe weather watches and warnings: an example from the 20 May 2013 Moore, Oklahoma, tornado outbreak. Bull. Amer. Meteor. Soc. 97, 69–84.10.1175/BAMS-D-14-00054.1
- Guedj, S., Karbou, F. and Rabier, F. 2011. Land surface temperature estimation to improve the assimilation of SEVIRI radiances over land. J. Geophys. Res. 116, D14107.10.1029/2011JD015776
- Han, Y., Weng, F., Liu, Q. and van Delst, P. 2007. A fast radiative transfer model for SSMIS upper atmosphere sounding channels. J. Geophys. Res. 112, D11121. DOI:10.1029/2006JD008208.
- Heidinger, A. 2011. ABI cloud mask. NOAA NESDIS STAR Algorithm Theoretical Basis Doc., 93 pp. Online at: http://www.goes-r.gov/products/ATBDs/baseline/Cloud_CldMask_v2.0_no_color.pdf
- Heidinger, A. K., Evan, A. T., Foster, M. J. and Walther, A. 2012. A Naive Bayesian cloud-detection scheme derived from CALIPSO and applied within PATMOS-x. J. Appl. Meteor. Climatol. 51, 1129–1144.10.1175/JAMC-D-11-02.1
- Hong, S.-Y. and Dudhia, J. 2003. Testing of a new non-local boundary layer vertical diffusion scheme in numerical weather prediction applications. In: 20th Conference on Weather Analysis and Forecasting/16th Conference on Numerical Weather Prediction, Seattle, WA.
- Hong, S.-Y. and Lim, J.-O. J. 2006. The WRF singlemoment 6-class microphysics scheme (WSM6). J. Korean Meteor. Soc. 42, 129–151.
- Kain, J. S. 2004. The Kain–Fritsch convective parameterization: an update. J. Appl. Meteor. 43, 170–181.10.1175/1520-0450(2004)043<0170:TKCPAU>2.0.CO;2
- Kain, J. S. and Fritsch, J. M. 1990. A one-dimensional entraining/detraining plume model and its application in convective parameterization. J. Atmos. Sci. 47, 2784–2802.10.1175/1520-0469(1990)047<2784:AODEPM>2.0.CO;2
- Kain, J. S. and Fritsch, J. M. 1993. Convective parameterization for mesoscale models: the Kain-Fritsch scheme. In The Representation of Cumulus Convection in Numerical Models. Meteorological Monographs, No. 24. Boston: American Meteorological Society, pp. 165–170.
- Köpken, C., Kelly, G. and Thépaut, J.-N. 2004. Assimilation of Meteosat radiance data within the 4D-Var system at ECMWF: assimilation experiments and forecast impact. Q. J. R. Meteorolo. Soc. 130, 2277–2292.10.1256/qj.02.230
- Mecikalski, J. R. and Bedka, K. M. 2006. Forecasting convective initiation by monitoring the evolution of moving convection in daytime GOES imagery. Mon. Wea. Rev. 134, 49–78.10.1175/MWR3062.1
- Mecikalski, J. R., Williams, J. K., Jewett, C. P., Ahijevych, D., LeRoy, A. and co-authors. 2015. Probabilistic 0–1-h convective initiation nowcasts that combine geostationary satellite observations and numerical weather prediction model data. J. Appl. Meteor. Climatol. 54, 1039–1059.10.1175/JAMC-D-14-0129.1
- Okamoto, K. 2013. Assimilation of overcast cloudy infrared radiances of the geostationary MTSAT-1R imager. Q. J. R. Meteorol. Soc. 139, 715–730.10.1002/qj.v139.672
- Purser, R. J., Wu, W.-S., Parrish, D. F. and Roberts, N. M. 2003a. Numerical aspects of the application of recursive filters to variational statistical analysis. Part I: spatially homogeneous and isotropic Gaussian covariances. Mon. Wea. Rev. 131, 1524–1535.10.1175//1520-0493(2003)131<1524:NAOTAO>2.0.CO;2
- Purser, R. James, Wu, W.-S., Parrish, D. F. and Roberts, N. M. 2003b. Numerical aspects of the application of recursive filters to variational statistical analysis. Part II: spatially inhomogeneous and anisotropic general covariances. Mon. Wea. Rev. 131, 1536–1548.10.1175//2543.1
- Qin, Z., Zou, X. and Weng, F. 2013. Evaluating added benefits of assimilating GOES Imager radiance data in GSI for coastal QPFs. Mon. Wea. Rev. 141, 75–92.10.1175/MWR-D-12-00079.1
- Shao, H., Derber, J., Huang, X.-Y., Hu, M., Newman, K. and co-authors. 2016. Bridging research to operations transitions: status and plans of community GSI. Bull. Amer. Meteor. Soc. 97, 1427–1440. DOI:10.1175/BAMS-D-13-00245.1.
- Shen, Y., Zhao, P., Pan, Y. and Yu, J. 2014. A high spatiotemporal gauge-satellite merged precipitation analysis over China. J. Geophys. Res. Atmos. 119, 3063–3075. DOI:10.1002/2013JD020686.
- Stengel, M., Undén, P., Lindskog, M., Dahlgren, P., Gustafsson, N. and co-authors. 2009. Assimilation of SEVIRI infrared radiances with HIRLAM 4D-Var. Q. J. R. Meteorol. Soc. 135, 2100–2109.10.1002/(ISSN)1477-870X
- Szyndel, M. D. E., Thépaut, J.-N. and Kelly, G. 2005. Evaluation of potential benefit of SEVIRI water vapour radiance data from Meteosat-8 into global numerical weather prediction analyses. Atmos. Sci. Lett. 6, 105–111.10.1002/(ISSN)1530-261X
- Velden, C. S. 1996. Winds derived from geostationary satellite moisture channel observations: applications and impact on numerical weather prediction. Meteor. Atmos. Phys. 60, 37–46.10.1007/BF01029784
- Velden, C. S., Hayden, C. M., Nieman, S. J., Menzel, W. P., Wanzong, S. and Goerss, J. S. 1997. Upper-tropospheric winds derived from geostationary satellite water vapor observations. Bull. Amer. Meteor. Soc. 78, 173–195.10.1175/1520-0477(1997)078<0173:UTWDFG>2.0.CO;2
- Velden, C. S., Olander, T. L. and Wanzong, S. 1998. The impact of multispectral GOES-8 wind information on Atlantic tropical cyclone track forecasts in 1995. Part I: dataset methodology, description, and case analysis. Mon. Wea. Rev. 126, 1202–1218.10.1175/1520-0493(1998)126<1202:TIOMGW>2.0.CO;2
- Weng, F. 2007. Advances in radiative transfer modeling in support of satellite data assimilation. J. Atmos. Sci. 64, 3799–3807.10.1175/2007JAS2112.1
- Wu, Wan-Shu, James Purser, R. and Parrish, David F. 2002. Three-dimensional variational analysis with spatially inhomogeneous covariances. Mon. Wea. Rev. 130, 2905–2916.10.1175/1520-0493(2002)130<2905:TDVAWS>2.0.CO;2
- Zhuge, X. and Zou, X. 2016. Test of a modified infrared only ABI cloud mask algorithm for AHI radiance observations. J. App. Meteor. Climatol. 55, 2529–2546. DOI:10.1175/JAMC-D-16-0254.1.
- Zou, X., Qin, Z. and Weng, F. 2011. Improved coastal precipitation forecasts with direct assimilation of GOES-11/12 imager radiances. Mon. Wea. Rev. 139, 3711–3729.10.1175/MWR-D-10-05040.1
- Zou, X., Qin, Z. and Zheng, Y. 2015. Improved tropical storm forecasts with GOES-13/15 imager radiance assimilation and asymmetric vortex initialization in HWRF. Mon. Wea. Rev. 143, 2485–2505. DOI:10.1175/MWR-D-14-00223.1.
- Zou, X., Zhuge, X. and Weng, F. 2016. Characterization of bias of Advanced Himawari Imager infrared observations from NWP background simulations using CRTM and RTTOV. J. Ocean Atmos. Tech. 33, 2553–2567. DOI:10.1175/JTECH-D-16-0105.1.