Abstract
The relationship between aerosol index (AI) and cloud effective radius (CER) was examined over five sea regions in the northern Pacific using daily Moderate Resolution Imaging Spectroradiometer data from 2002–2014. The results show that there tends to be a negative relationship between AI and CER at lower AI, becoming positive at higher AI, suggesting the Twomey effect held in low aerosol environment. Over the entire AI range, the correlation between AI and CER was significantly positive over the two marginal seas (the Bohai–Yellow Sea and the Sea of Japan), and it was significantly negative over the three open oceans (the North Pacific subtropical gyre, the western and eastern subarctic North Pacific) for all seasons, except that the correlations in summer were significantly positive over the two subarctic North Pacific regions. A series of statistical analyses showed that the AI–CER relationship (the regression slope) is significantly correlated with relative humidity (RH) and precipitable water vapour (PWV); on average, PWV accounts for 60% of the variance of the slope for the two marginal seas and higher for the three open oceans (79%). The slope can change from negative to positive at high PWV levels, suggesting that water vapour plays an important role in the variability of the slope. Aerosol hygroscopic growth and the growth of CER in humid condition may counteract the Twomey effect at high AI, particularly over the two marginal seas. The error high contribution of the low liquid cloud fraction (LCF) to AI–CER relationship may partially account for the positive slope over the two marginal seas, while the low LCF has a negligible impact on the three open oceans. Additionally, over the three open oceans, stable thermodynamic state may prevent the effect of aerosol on cloud at high AI.
1. Introduction
Clouds are one of the most important and uncertain components in weather forecasting and climate prediction. Activated aerosol particles can serve as cloud condensation nuclei (CCN) and ice nuclei (IN), and then influence cloud droplets, ice crystals, and even precipitation (e.g. Tao et al., Citation2012; Wang, Citation2013; Xiao et al., Citation2014). Clouds and aerosols are also very important in Earth’s global energy (radiative) budget (Trenberth et al., Citation2009). Assessment of aerosol–cloud interactions (ACI) is challenging and important for understanding climate change because aerosols (e.g. their concentration, chemical composition, and size) and clouds are highly variable in space and time.
On the one hand, enhanced aerosol concentrations can increase the concentration of cloud droplets (Ramanathan et al., Citation2001; Bréon et al., Citation2002; Feingold et al., Citation2003; Penner et al., Citation2012) and reduce droplet size (Nakajima et al., Citation2001; Twohy et al., Citation2005), and hence lead to an increase in cloud albedo under the assumption of fixed liquid water content, this is called the Twomey aerosol indirect effect (Twomey, Citation1977; Twomey et al., Citation1984) or the first indirect effect (Ramaswamy et al., Citation2001). Based on this effect, a linear relationship is expected between a change in aerosol number concentration (usually the equivalent of aerosol optical depth [AOD] or the aerosol index [AI], which is defined as AOD by Ångström exponent) and a change in cloud droplet effective radius (CER) in log scale under a constant liquid water path (LWP) (Kaufman and Fraser, Citation1997; Nakajima et al., Citation2001; Bréon et al., Citation2002; McComiskey and Feingold, Citation2012; Costantino and Bréon, Citation2013). The negative slope of AOD or AI and CER on a log–log scale (–dlog[CER]/dlog[AI]) is referred to as the quantification of the aerosol indirect effect (Bréon et al., Citation2002; Feingold et al., Citation2003), and the terminology was later changed to ACI (McComiskey and Feingold, Citation2012). The slope is considerably variable and usually negative in many places in the world (Jin and Shepherd, Citation2008; Yuan et al., Citation2008). Nevertheless, positive correlations are also found in some places, such as near coasts of the Gulf of Mexico and southeastern China (Yuan et al., Citation2008), the Mediterranean Sea (Myhre et al., Citation2007), the Eastern China mainland and Yellow Sea (Tang et al., Citation2014), and the Southeast Atlantic in unstable and high aerosol loading environment (Andersen and Cermak, Citation2015). A case study over Northern China even indicated that precipitation amount under polluted conditions (AOD > 0.9) can increase up to 17% during the lifetime of a cloud, indicating an increase in CER at high AOD (Guo et al., Citation2014).
Satellites can consistently observe large-scale aerosol and cloud properties and hence provide a series of valid data-sets with which to investigate the relationships between them. Statistical analyses have been used to investigate the first indirect effect of aerosols using remote sensing aerosol and cloud parameters, such as AOD, AI, CER, and/or cloud droplet number concentration (Nakajima et al., Citation2001; Bréon et al., Citation2002; Feingold et al., Citation2003; Kaufman et al., Citation2005; Huang et al., Citation2006; Quaas et al., Citation2009; Penner et al., Citation2012; Costantino and Bréon, Citation2013; Tang et al., Citation2014). However, those studies are focused on the global scale or on various continental or ocean regions.
To date, few studies have compared aerosol indirect effects over marginal seas, including the China Seas, the Sea of Japan, and the open oceans in the northern Pacific Ocean. The China Seas and the Sea of Japan is close to the Chinese continent, where air pollution is heavy (Wang, Shi, et al., Citation2015; Wang, Xue, et al., Citation2015), and they are also frequently affected by Asian dust storms (Zhao et al., Citation2006; Tan et al., Citation2013). The downwind regions with high aerosol loading are notably influenced by continental aerosols, and CER over areas downwind of land surfaces are clearly affected by continental influences owing to atmospheric transport (Bréon et al., Citation2002; Jin and Shepherd, Citation2008). The North Pacific subtropical gyre and the subarctic North Pacific are farther from the continent. In this study, the similarities and differences between the correlations of AI and CER over the marginal seas and open oceans were examined using aerosol and cloud properties derived from Moderate Resolution Imaging Spectroradiometer (MODIS) observations onboard the Aqua satellite and meteorological parameters from reanalysis data.
2. Data and methods
The MODIS Level 3 Collection 6 daily product MYD08_D3 was used (King et al., Citation2003; Levy et al., Citation2013). Comparisons with surface-based sun photometer data revealed that Collection 6 should improve upon Collection 5, and overall, 69.4% of MODIS Collection 6 AOD fell within an expected uncertainty of ±(0.05 + 15%) (Levy et al., Citation2013). Data included AOD at 0.55 and 0.86 μm, cloud top pressure (CTP, hPa), cloud top temperature (CTT, K), CER (μm), LWP (g m−2), and PWV (cm). The spatial resolution was 1°°× 1°.
The Ångström exponent (α; 0.55 μm vs. 0.86 μm) is a qualitative indicator of aerosol particle size. An α < 1 indicates the dominance of coarse mode aerosols with radii > 0.5 μm, which are usually associated with dust and sea salt (Guo et al., Citation2014). Ångström exponent was calculated from AOD at 0.55 and 0.86 μm. An AI, defined as AOD × α, was used to represent aerosol properties among the researched sea regions as it is better to estimate column aerosol number using AI than AOD alone and AI is representative of the column CCN concentration under some assumptions (Nakajima et al., Citation2001; Lebsock et al., Citation2008; McComiskey and Feingold, Citation2012; Stier, Citation2016).
To exclude pixels that contain large amount of high cloud or low clouds topped with cirrus (Yuan et al., Citation2008; Costantino and Bréon, Citation2013), the pixel with CTP ≤ 680 hPa and a ratio of CTP less than 680 hPa ≤ 0.5 were excluded. The ratio is determined from CTP histogram counts in MYD08_D3 product.
One of the advantages of MODIS cloud products is the thermodynamic phase of the cloud, which is used in subsequent processing of cloud optical and microphysical properties. The MODIS CER algorithm utilizes three water-absorbing spectral bands (1.6, 2.1, and 3.7 μm) containing strong particle size information in conjunction with three non-absorbing bands to minimize the underlying surface reflectance: 0.65, 0.86 and 1.24 μm for land, ocean and ice/snow surfaces, respectively. The standard MODIS CER product is retrieved from the 2.1 μm wavelength channel and was used in this study. The CER from the 3.7 μm channel was also used for comparison.
Based on the meteorological background and geographical location, the northern Pacific Ocean was partitioned into five sea regions (Fig. ) with varying degrees of influence from Asian monsoons: the Bohai–Yellow Sea (32–41°N, 117°–127°E), the Sea of Japan (34–50°N, 127°–143°E), the western subarctic North Pacific (45–65°N, 145°E–180°E), the eastern subarctic North Pacific (45–65°N, 180°W–135°W), and the North Pacific subtropical gyre (15–35°N, 150°E–135°W). The two marginal seas of the North Pacific, the Bohai–Yellow Sea and the Sea of Japan, have high AOD and AI values relative to the three open oceans, the western and eastern subarctic North Pacific and the North Pacific subtropical gyre (Fig. a and b). The western and eastern subarctic North Pacific have similar PWV values (Fig. c), but AOD and AI values are higher and CER (Fig. d) are lower over the western subarctic North Pacific. Asian monsoons are one of the most significant components of the global circulation system (Yang and Lu, Citation2014). Northwesterlies over East Asia and westerlies over the subtropical western North Pacific generated by the East Asian winter monsoon (Yang and Lu, Citation2014) and the westerlies in the middle latitudes (Fig. e and f) could transport Asian dust particles and anthropogenic aerosols from the eastern Asian continent to the Pacific Ocean from late autumn to spring (Zhao et al., Citation2006; Wang, Shi, et al., Citation2015; Wang, Xue, et al., Citation2015). The impacts of dust storms and dust deposition flux differ in the five seas (Tan et al., Citation2013). During the period from May to September, the prevailing Asian–Pacific summer monsoon will induce southwesterly winds (Fig. g) over the South to southern East Asian, and plays an important role in rainfall in Eastern China, the Chinese coastal seas, the Sea of Japan and the subtropical western North Pacific (Wang and Lin, Citation2002; Ding and Chan, Citation2005). The Asian monsoon circulation must affect aerosol characteristics, such as size, chemical composition and mixing state, in the five examined sea regions.
Fig. 1. (a–d) The MODIS-derived climatological daily mean AOD, AI, precipitable water vapor (cm), and CER (μm) from 1 December 2002 to 31 December 2014. (e–h) the shaded images show the NCEP monthly long-term mean wind speeds (m s−1) from 1981–2010 at the surface (e, g) and 500 hPa (f, h). Contour lines in (e, g) indicate sea level pressure (hPa) and contour lines in (f, h) indicate geopotential height (m). Rectangles A–E in (a–d) show the maps of the studied sea regions in the northern Pacific, A: the Bohai–Yellow Sea (32–41°N, 117°–127°E), B: the Sea of Japan (34–50°N, 127°–143°E), C: the western subarctic North Pacific (45–65°N, 145°E–180°E), D: the eastern subarctic North Pacific (45–65°N, 180°W–135°W), and E: the North Pacific subtropical gyre (15–35°N, 150°E–135°W).
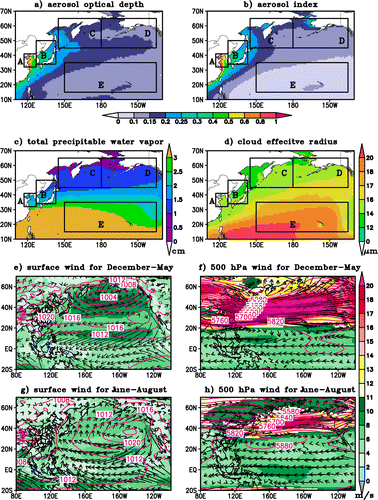
The correlations between AI and CER were examined during the period from 1 December 2002 to 31 December 2014, thus the seasonal relationship can also be examined. To examine the possible factors affecting the observed correlations, the National Centers for Environmental Prediction/National Center for Atmospheric Research (NCEP/NCAR) global reanalysis RH data were also used to represent the water vapour conditions in the same manner as MODIS PWV. The NCEP monthly long-term mean wind speed (m s−1), sea level pressure (hPa), and geopotential height (m) from 1981–2010 were used to investigate the meteorological background over the examined sea regions. The data were available on a latitude–longitude grid with a resolution of 2.5° × 2.5° and were interpolated into the same resolution as MODIS. To investigated the effect of thermodynamic state, lower tropospheric stability (LTS), defined as the difference in potential temperature between 700 hPa and the surface (Klein and Hartmann, Citation1993; Andersen and Cermak, Citation2015), was derived from ERA Interim reanalysis pressure and temperature data provided by the European Centre for Medium-Range Weather Forecasts (ECMWF). The spatial resolution of 1° × 1°, the same as MODIS, was used here.
3. Results
3.1. Relationship between AI and CER
The correlations between AI and CER for the five sea regions in the North Pacific were determined in log scale according to previous definitions about the first indirect effect of aerosol (Bréon et al., Citation2002; Feingold et al., Citation2003). To demonstrate the variation in CER in response to AI, after filtering data, the CERs were sorted as a function of AI and averaged for every 300 samples, as done by Tang et al. (Citation2014), producing one AI value and one CER value on the scatter plots. Figure shows the seasonal and annual relationships between AI and CER for the five sea regions; the corresponding slope (hereafter ‘ACICER’) and correlation coefficient (R) values of the regression curves between AI and CER are shown in Table . Noted that, the results are noisier due to smaller data-sets (1 or 2 at the most) over the low AI range (≤0.1, 0.15, or 0.2) for the Bohai–Yellow Sea (see Fig. a). Thus, the ACICER and R values over the low AI range for each point in Fig. a representing AI and CER values averaged for every 50 samples or with no average, as done by Koren et al. (Citation2008), are also shown in Table . The relationships between AI and CER in all other situations exhibited the same positive or negative correlation, thus they are not shown in Table .
Fig. 2. Scatter plots of CER against AI in log scale over the five sea regions. (a–e) seasonal, (f) annual, (g) annual for CER at 3.7 μm. In (f) and (g), the letters a–e represent the five sea regions, respectively. The colours of points correspond to the colours of the letters. Data are from the MODIS Aqua Level 3 Collection 6 daily product MYD08_D3. The points represent CER sorted by AI, and both AI and CER are averaged over every 300 data points.
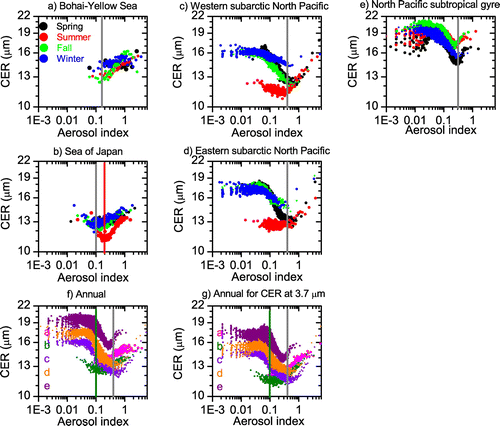
Table 1. Seasonal and annual correlation coefficients (R) and slopes (ACICER) of linear regression curves between aerosol index (AI) and cloud effective radius (CER) in log scale from 1 December 2002 to 31 December 2014.
The correlations varied among the seas and seasons. For the two marginal seas (the Bohai–Yellow Sea and the Sea of Japan), negative correlations existed between AI and CER over the low AI range (≤0.1, 0.15, 0.2, or 0.3) with ACICER = –0.01 to –0.11 (R = –0.08 to –0.85). The negative correlations were statistically significant (significance level < 0.05) during summer, autumn, winter and annually but not (significance level > 0.05) in spring. Over the high AI range (>0.1, 0.15, 0.2, or 0.3), there were statistically significant positive correlations with ACICER = 0.02 to 0.12 (R = 0.56 to 0.95) except for spring over the Bohai–Yellow Sea. The CER was about 11–14 μm at an AI of ~0.1, but was as high as 13–16 μm at high AI over these seas.
Over the three open oceans, the two AI sub-ranges for the two reversed correlations between AI and CER was shifted to ≤or> 0.3 or 0.4, respectively. For the western and eastern subarctic North Pacific, over the entire AI range, there were significant negative correlations during spring, autumn, winter, and annually (ACICER = –0.01 to –0.14) and a significant positive correlation during summer (ACICER = 0.02 or 0.01). Over the North Pacific subtropical gyre, the positive correlation over the high AI range was not statistically significant in autumn but significant in the other seasons. Over the entire AI range, there was a significant negative correlation between AI and CER with ACICER = –0.03 to –0.08. When AI ≤ 0.3, the maximum CER was as high as 21 μm; when AI increased to 0.3, the CER decreased to 15 μm.
The relationship between AI and CER supports Twomey’s hypothesis over all five research areas when AI is relatively small. At higher AIs, the correlation between AI and CER became positive, particularly over the Bohai–Yellow Sea, the Sea of Japan, and the subarctic North Pacific. It seems that ACI was robust under low aerosol loadings (Andersen and Cermak, Citation2015), while it may be also strong but counteracted under high aerosol loadings. Over the entire AI range, the relationship between AI and CER supports Twomey’s hypothesis over the three open oceans except the subarctic North Pacific in summer.
The spatial distribution of the correlation coefficients between AI and CER (Fig. ) reflects the same results as the scatter plots. Over the entire AI range, the correlation between AI and CER was negative over the three open oceans and positive over the two marginal seas (Fig. a). Over the low AI range (Fig. b), the correlation between AI and CER was negative over part of the Bohai–Yellow Sea and the Sea of Japan. Over the high AI range, the positive correlation coefficient was >0.1 over most of the two marginal seas (Fig. c). The correlation was negative and R < –0.1 over most of the three open oceans over the low AI range (Fig. d), while R became positive over most of the sea regions when AI > 0.4 (Fig. e).
3.2. Seasonal and monthly variation in the correlation between AI and CER
The statistical significance of the negative correlation between AI and CER over the low AI range for the two marginal seas and the positive correlation over the high AI range for the three open oceans varied with season. Hence, we investigated the seasonal and monthly correlations between AI and CER in 2002–2014. Seasonal and monthly correlation coefficients are shown in Fig. , S1, and S2, respectively.
Fig. 4. Seasonal variation in the correlation coefficient (R) between AI and CER in log scale over the five sea regions. For each year, the sequence of the bars runs from spring (March, April, and May) to winter (December, January and February of the following year). The solid bars are statistically significant (p < 0.05) while the empty bars are not statistically significant (p > 0.05). The method is the same as in Fig. , but each point was averaged over every 50 data points. Each pair in one season in (c) and (d) represents R over the low (≤0.2, 0.3 or 0.4) and high (>0.2, 0.3 or 0.4) AI range, respectively.
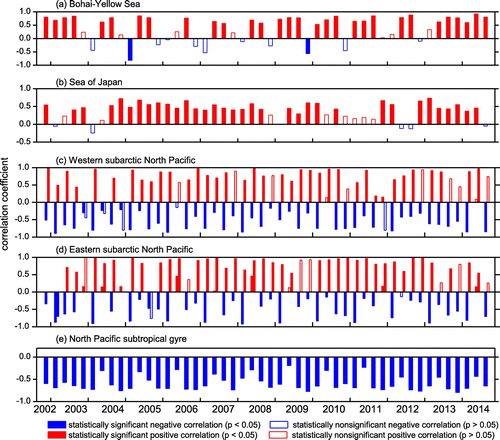
The correlation between AI and CER was significantly positive over the entire AI range for the Bohai–Yellow Sea and the Sea of Japan and significantly negative over the North Pacific subtropical gyre in most seasons (Fig. a, b, and e). Over the two subarctic North Pacific regions, in most seasons, there was a significantly negative correlation between AI and CER over the low AI range and a significantly positive correlation over the high AI range (Fig. c and d). However, over the entire AI range, AI was significantly positively correlated with CER in most summers and significantly negatively correlated with CER in most other seasons (Fig. S1). Similar results were observed for monthly variation in the correlation between AI and CER (Fig. S2). The correlation was positive in most months over the two marginal seas and negative in most months over the North Pacific subtropical gyre. Over the two subarctic North Pacific regions, there was a positive correlation between AI and CER in the summertime, mainly from May to August.
4. Possible impacts and reasons for the relationship between AI and CER
4.1. Uncertainty due to CER retrieval wavelength
Comparisons with aircraft data have shown that the standard MODIS CER product, CER at the 2.1 μm wavelength channel, is overestimated at the cloud top by ~13–20% (Painemal and Zuidema, Citation2011; King et al., Citation2013). Recent studies have indicated that CER retrieved from the 3.7 μm channel was less affected by cloud inhomogeneity (Zhang and Platnick, Citation2011), in the best agreement with in situ values (King et al., Citation2013), or have less zonal mean biases (Liang et al., Citation2015). Thus we also calculated the AI–CER correlation using CER retrieved from the 3.7 μm channel, which is shown in Fig. g. The annual CER pattern on the 3.7 μm channel scatter plots against AI was the same as for CER retrieved from the 2.1 μm wavelength channel. The patterns still showed apparent positive correlations between AI and CER over the high AI range over the two marginal seas and the three open oceans with ACICER = 0.07 (R = 0.93) for the Bohai–Yellow Sea, ACICER = 0.06 (R = 0.83) for the Sea of Japan, ACICER = 0.10 (R = 0.86) for the western subarctic, ACICER = 0.12 (R = 0.91) for the eastern subarctic, and ACICER = 0.11 (R = 0.75) for the North Pacific subtropical gyre.
4.2. Effects of cloud top pressure and cloud top temperature
In the Mediterranean Sea, the positive correlation between CER and AOD was attributed to CTP decreasing with increasing AOD, as CER increases with decreasing CTP (Myhre et al., Citation2007). Studies have suggested that CTT or CTP are not the only reasons for the positive correlation between AOD and CER near coasts of the Gulf of Mexico (Yuan et al., Citation2008) and over the North China Plain (Tang et al., Citation2014). CER is predicted to increase with height in warm water clouds by parcel theory (King et al., Citation2013). This is consistent with observations by aircraft (Stephens and Platt, Citation1987; King et al., Citation2013), Doppler radar (Kato et al., Citation2001), and satellite (Rosenfeld and Lensky, Citation1998). On the other hand, CER may increase towards the cloud base due to larger drizzle or precipitation-sized droplets forming lower in the cloud, as has been observed in satellite (Nakajima et al., Citation2010) and aircraft (King et al., Citation2013) data. Either of the two cases might cause an artificial positive correlation between AI and CER. To determine the influence of CTP or CTT, the correlation between AI and CER was calculated within narrow intervals of CTP (10 hPa) and CTT (1 degree).
The relationship between CTT or CTP and the ACICER (Fig. ) showed that when CTP < ~920 hPa or CTT < ~275 K, the ACICER either increased slightly or did not changed with decreasing CTT or CTP. That may be because CER increases from cloud base to cloud top in non-precipitating liquid water clouds. When CTP > ~920 hPa or CTT > ~275 K, the ACICER increased with increasing CTT or CTP. Nakajima et al. (Citation2010) showed that CER could increase lower in the cloud due to large drizzle and precipitation-sized droplets forming there. Aircraft-observed profiles have also indicated that the presence of larger drizzle or precipitation-sized droplets may cause CER to increase towards the base of clouds (King et al., Citation2013). That may cause the ACICER to increase or even become positive.
Fig. 5. Slopes of linear regression curves between AI and CER plots (ACICER) for 10 hPa cloud top pressure (CTP) intervals and 1 K cloud top temperature (CTT) intervals. Each ACICER in the figure is significant at the 0.05 level.
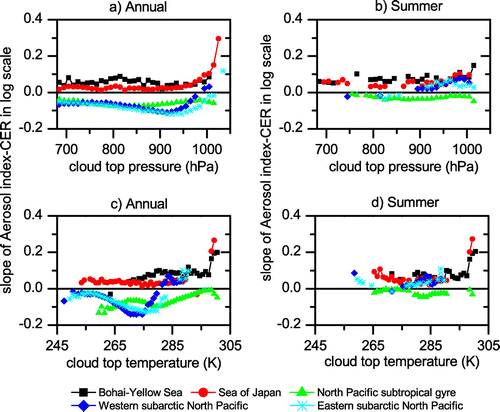
In the case that CTT or CTP is the only cause of the positive relation between AI and CER, no positive correlation could occur under the conditions of very small changes in CTT (1 K) or CTP (10 hPa). However, the ACICER is always positive over the Bohai–Yellow Sea and the Sea of Japan, as well as over the subarctic North Pacific in summer, suggesting that changes in CTT and CTP may not be the sole causes of the positive correlation between AI and CER over those sea regions.
4.3. Possible impacts of water vapour
There was a robust positive correlation between AI and CER over the two marginal seas and the two subarctic North Pacific regions in summer over the high AI range regardless of artefacts of satellite measurements, which means that cloud droplet size increases with increasing aerosol loading. Potential explanations are discussed below.
Previous studies have demonstrated the effects of environmental and meteorological parameters on associations between aerosols and cloud properties. Kaufman and Fraser (Citation1997) reported that the variability of the effects of smoke aerosols on cloud droplet size with latitude was correlated with the total PWV. Yuan et al. (Citation2008) found that PWV was the most influential factor driving the variation in the efficiency of the aerosol indirect effect; the effect is positive for moist regions and negative for dry regions. Koren et al. (Citation2010) showed that cloud top height and cloud fraction correlate best with model pressure updraft velocity and RH. Tang et al. (Citation2014) determined that southerly or southwesterly winds prevailed over the North China Plain and the East Sea on polluted days; pollutants and abundant water vapour were thereby transported from Southern China or South-East Asia to those regions, which induced increases in CER and AOD. Andersen and Cermak (Citation2015) found that AI and CER are mostly positively associated in the stable environment over the Southeast Atlantic, and meteorological factors, e.g. RH, may be the main factor determining cloud microphysics in situations with high aerosol loading. The above studies imply the important role of water vapour. A series of statistical analyses among reanalysis data and satellite-observed cloud and aerosol properties showed that RH and PWV play a role in the variability of the correlation between AI and CER, suggesting the consistency with previous studies.
4.3.1. Correlation between water vapour and the relationship between AI and CER
Table shows the correlation coefficients (R) between monthly RH or PWV and the ACICER or R between AI and CER (R[AI–CER]) (see Fig. S2). Correlation analyses indicated that RH is significantly positively correlated with the relationship between AI and CER over the four sea regions except for the Sea of Japan, with the correlation coefficient ranging from 0.15 to 0.65. The same is true for PWV except for the Sea of Japan; the significant R between PWV and ACICER or R[AI–CER] were in the range of 0.28–0.60.
Table 2. Correlation coefficients (R) between monthly relative humidity (RH) or precipitable water vapour (PWV) and the regression slope (ACICER) and correlation coefficient (R[AI–CER]) of AI–CER in log scale, and p-values of the Granger causality test between them. The p-value of the Granger causality test is the significance level using the F distribution.
In addition to the correlation test, we also applied Granger causality theory. It has been used previously to test anthropogenic influences (such as trace gas concentrations and sulphate aerosols) on global temperature (Kaufmann and Stern, Citation1997). Granger causality definition could test whether lagged information on a variable X provides statistically significant information about a variable Y in the presence of lagged values of Y. If not, then X does not ‘Granger cause’ Y; otherwise, it can be said variable X ‘Granger causes’ variable Y or variable X is the ‘Granger cause’ of variable Y (Granger, Citation1969; Eichler, Citation2012). The Granger causality does not necessarily imply the presence of a physical causal mechanism between the two variables. The coefficient estimates may be biased by the omission of relevant variables that are in fact the causal variables (Kaufmann and Stern, Citation1997). The Granger causality test does not detect non-linear causal relationships and can lead to spurious causalities when confounding variables are not included in the analysis (Eichler, Citation2012). However, it remains a popular method for causality analysis in time series due to its computational simplicity (Eichler, Citation2012).
A detailed method for the test can be found in Kaufmann and Stern (Citation1997) and Eichler (Citation2012). In this study, Granger causality tests were conducted using the Free Statistics Software (Wessa, Citation2015), and the significance levels (p-values) of the tests are shown in Table . The results indicated that RH and PWV do Granger cause the correlation between AI and CER except for RH over the eastern subarctic North Pacific.
4.3.2. Dependence of the relationship between AI and CER on total PWV
For the five studied sea regions, the LWP increased or decreased with AI and 66–92% of LWP is less than 100 g m−2; and the percentage was >82% for the two marginal seas. Twomey’s hypothesis assumes a constant LWP. To constrain the variability caused by changes in LWP, the effects of PWV on the ACICER were analysed within small intervals of LWP (10–30 g m−2). In addition, the situation for total LWP was also analysed because 25–34% of LWP was >100 g m−2 for the three open oceans. The variation in the ACICER with PWV (cm) for different LWP intervals and total LWP is shown in Fig. , and the corresponding correlation coefficients of linear regression between the ACICER and PWV are shown in Table .
Fig. 6. (a -h) Dependence of the slopes of linear regression curves between AI and CER (ACICER) on precipitable water vapor (PWV, cm) for 10 or 30 g m−2 LWP intervals; (i) Dependence of ACICER on PWV for total LWP; (j) Dependence of ACICER on lower tropospheric stability. Each ACICER in the figure is significant at the 0.05 level.
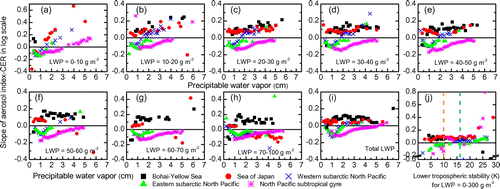
Table 3. Correlation coefficients of linear regression between the slope of the AI–CER curve in log scale (ACICER) and precipitable water vapour (PWV) for different liquid water path (LWP) intervals.
In every LWP interval, the ACICER values increased with PWV for all five seas. The ACICER was negative at low PWV and may be shifted to positive at high PWV. The mean regression correlation coefficient between the ACICER and PWV over LWP ≤ 100 g m−2 or over total LWP was ~0.8 for the five sea regions, or in other words, precipitable water accounts for 64% (R2) of the variance of the correlation between AI and CER in the five research sea regions. The ratio was lower for the two marginal seas (60%) and higher for the three open oceans (79%), and it was the lowest for the Sea of Japan (27%) and the highest for the North Pacific subtropical gyre (90% for total LWP). Over the two marginal seas, the PWV value at which the relationship between AI and CER changes from negative to positive is ~2 to 6 times smaller than that over the three open oceans. It seems that over the two marginal seas, the positive relationship between AI and CER could develop if PWV increases slightly, while that wouldn’t happen unless PWV increases to a very high value over the open oceans.
It is noted that the minor variation in LWP may be not appropriate here because MODIS LWP is not an independent measure with CER and Level 3 LWP were used, which is average at 1° × 1° spatial resolution from high spatial resolution Level 2 product (McComiskey and Feingold, Citation2012). In addition, except for PWV, other meteorological parameters, not addressed here, may influence the sensitivity of CER response to AI. Particularly, over the Sea of Japan, PWV has the lowest contribution to the ACICER, it only accounted for 27% (R = 0.52) of the variance of the ACICER.
5. Discussions
5.1. Effects of aerosol hygroscopic growth on the relationship between AI and CER
According to the above analyses, increased water vapour may be the reason for the positive correlation between AI and CER. The question is why a positive correlation exists at high AI (>0.1–0.4). It may be related to the hygroscopic growth properties of aerosol particles.
In Eastern China, dust type air pollution and non-dust air pollution dominate in spring and in autumn and winter, respectively (Zhang et al., Citation1998; Gong and Zhang, Citation2008; Wang et al., Citation2014; Wang, Shi, et al., Citation2015; Wang, Xue, et al., Citation2015). High aerosol loading conditions in East Asia resulted from increasing anthropogenic aerosol emissions along with the rapid increase in gross domestic product and economic growth. This must affect the downwind coastal seas, such as the Bohai–Yellow Sea and the Sea of Japan, due to atmospheric transport. Aerosol hygroscopic growth in East Asia has been studied with many observations and models in recent years (e.g. Jung and Kim, Citation2011; Kim et al., Citation2011; Liu et al., Citation2013; Li et al., Citation2014). The hygroscopic growth factor of the aerosol scattering coefficient for four different parameterization schemes was compared by Li et al. (Citation2014). Their results showed that the hygroscopic growth factor is small and almost unchanged at lower RH (<60–80% for different schemes), while it increases rapidly with humidity at higher RH. The relationships between AI or CER and PWV or RH for the two marginal seas are shown in Fig. and the climatological RH and PWV values over the low and high AI range are shown in supplementary material Table S1.
Fig. 7. Scatter plots of precipitable water vapour (cm) and relative humidity (%) against AI and CER over the two marginal seas. Each point was obtained in the same manner as in Fig. .
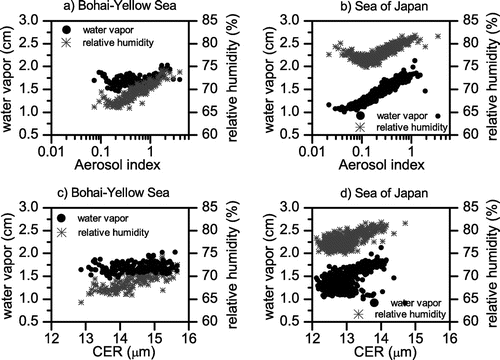
Figure shows that the AI–PWV curve and AI–RH curve are very similar to the AI–CER curve for the two marginal seas. PWV and RH decrease with increasing AI over the low AI range, and then increase with increasing AI over the high AI range. Over the high AI range, the climatological mean values of PWV and RH were 6–38 and 2–4% higher, respectively, than over the low AI range for the Bohai–Yellow Sea, the Sea of Japan, and the western and eastern subarctic North Pacific regions (Table S1). The same is true for the climatological mean minimum and maximum PWV and RH (Table S1). Over the North Pacific subtropical gyre, the mean PWV and RH over the high AI range was slightly higher than or comparable to that over the low AI range.
Over the high AI range, RH and PWV increased with increasing AI (Fig. ), the hygroscopic effect of water vapour on aerosol becomes more obvious at higher humidity or water vapour conditions (Li et al., Citation2014). On the other hand, CER also increased with increasing RH or PWV over the two marginal seas (Fig. ). The correlation coefficients between AI or CER and PWV or RH were 0.21–0.81 for the Bohai–Yellow Sea and 0.51–0.71 for the Sea of Japan. This may be the reason for the positive correlation between AI and CER at high AI and high water vapour. Similarly, the aerosol hygroscopic properties also partially counteracted the Twomey effect at high AI over the two subarctic North Pacific because strong positive correlation featured between RH or PWV and CER there (R = 0.44–0.63). In summer, over the two subarctic North Pacific regions, PWV and RH were 53–191% and 2–7%, respectively, higher than that in the other three seasons, which produced more significant aerosol hygroscopic growth, thus inducing the positive correlation between AI and CER. Over the North Pacific subtropical gyre, CER was heavily associated with PWV (R = 0.63) or RH (R = 0.23), the growth of CER in high PWV and RH condition may cause the positive AI–CER relationship at high AI there.
5.2. Sensitivity of AI–CER relationship to LTS
Matsui et al. (Citation2004) revealed that the aerosol indirect effect is sensitive to lower-tropospheric static stability over oceans between 37°S–37°N; greater fraction of aerosols are converted into cloud droplets in the lower LTS thereby decreasing the droplet size (i.e. stronger aerosol indirect effect). Andersen and Cermak (Citation2015) found that AI and CER is heavily dependent on LTS over the Southeast Atlantic. In an unstable environment (low LTS), more turbulent mixing leads to ACI (i.e. negative correlation between AI and CER), while in the stable environment (high LTS), situations with high aerosol loading tend to feature larger cloud droplets (most positive correlation between AI and CER).
The dependence of the ACICER on LTS over the five sea regions in this study was also investigated (see Fig. j). The similar result with was found at high AI for four sea regions except for the Bohai–Yellow Sea. In an unstable environment, the AI and CER has negative associations over the three open oceans and lower positive correlation over the Sea of Japan, while weak negative or strong positive correlation occurred in the stable environment. The increase of the ACICER with increasing LTS implicated that the effect of aerosol on cloud was decreased with increasing LTS, suggesting that stable thermodynamic state may prevent the effect of aerosol in high aerosol loading environment, particularly over the three open oceans.
5.3. Effects of low liquid cloud fraction
Gryspeerdt and Stier (Citation2012) indicated that the negative sensitivity between cloud droplet number concentration (Nc) and AOD over land, defined by dlnNc/dlnAOD, is generated by the low liquid cloud fraction (LCF) regime (shallow cumulus) because the contribution of low LCF regime to the sensitivity was amplified due to its high frequency of occurrence and more valid aerosol retrieval. The negative relationship between Nc and AOD might have induced the positive relationship between CER and AI in this study. Although Gryspeerdt and Stier (Citation2012) did not include the two marginal seas and the two subarctic North Pacific regions included in this study, the possible impact of low LCF was analysed by rechecking the AI–CER relationship for ten LCF bins, namely 10% LCF intervals over the full range of LCF (0–100%) (Fig. ).
Fig. 8. The slope of linear regression curves between AI and CER (ACICER) in 10 10% liquid cloud fraction (LCF) intervals over the 5 sea regions. Each pair in one 10% LCF intervals represents the ACICER over the low, high and entire AI range, respectively. The solid bars are statistically significant (p < 0.05) while the empty bars are not statistically significant (p > 0.05).
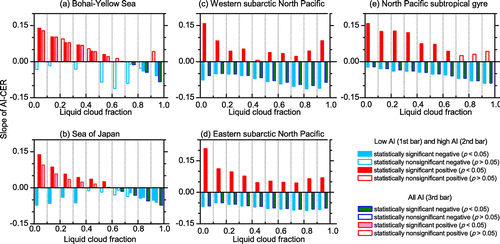
Results showed that over the two marginal seas, the Bohai–Yellow Sea and the Sea of Japan, the positive ACICER was more significant for low LCF and the ACICER became negative over the high and entire AI range for high LCF (>60%), suggesting a larger contribution from low LCF. Over the 3 open oceans, the AI–CER relationship for the 10 LCF bins is very similar. The positive relationship between AI and CER over the high AI range for high LCF was comparable to or even larger than low LCF, particularly in the western and eastern North Pacific. That suggests that LCF has a negligible impact on the positive relationship between AI and CER over the high AI range for the three open oceans.
5.4. Effects of giant CCNs in environments with high aerosol concentrations
Many studies have shown that adding giant CCNs (e.g. dust particles and sea salt) to polluted conditions would result in an early development of large raindrops and an earlier initiation of precipitation. This has been simulated by many models, including box model, 2-D cloud model (Feingold et al., Citation1999; Yin et al., Citation2000; Teller and Levin, Citation2006), an extended regional atomospheric modelling (Solomos et al., Citation2011), and a 3-D GCM model (Posselt and Lohmann, Citation2008). Combination analyses of satellite and model data also indicate that the probability of precipitation increasing in regions of high sea salt concentrations (L’Ecuyer et al., Citation2009). The enhancement of the coalescence between water droplets and the formation of precipitation associated with the presence of giant CCNs (L’Ecuyer et al., Citation2009; Sorooshian et al., Citation2009) could lead to an increase in CER. Yuan et al. (Citation2008) illustrated that CER increased with the addition of a few giant CCNs using a 2-D ensemble model. They deduced that giant CCNs could be one of the reasons for the positive relationship between AI and CER. On the other hand, sea salt is also another source of giant CCNs, and it should exist over the three open oceans. However, model simulations showed that giant CCNs could enhance the total precipitation on the ground in polluted clouds (with high CCN concentrations), but they have little effect on cleaner clouds (with low CCN concentrations) as drizzle is often active anyway (Feingold et al., Citation1999; Yin et al., Citation2000; Teller and Levin, Citation2006; Solomos et al., Citation2011). Figure shows that the two marginal seas, the Bohai–Yellow Sea and the Sea of Japan, are under conditions with high AOD and high AI, and AI is representative of the column CCN concentration under some assumptions (Nakajima et al., Citation2001; Lebsock et al., Citation2008). The mean AOD and AI values over the two marginal seas were 67–620% higher than over the three open oceans.
Dust aerosol provides an effective reactive surface for acidic gaseous species, such as SOx and NOy, and mixes internally and/or externally with anthropogenic aerosols and sea salt during long-range transport, further increasing the potential for dust to act as a giant CCN (Matsuki et al., Citation2010; Li et al., Citation2012). Aerosol measurements in the coastal cities of the Yellow Sea and the East China Sea have shown that the mass concentration of coarse particles is relatively large in these areas due to the contributions of sea salt, and that the concentration of >3 μm coarse particles in spring is larger than that in other seasons due to frequent dust events (Wang, Zhu, et al., Citation2015). Observations over the eastern Pacific Ocean have revealed that an aged aerosol layer effectively behaves as a CCN owing to its large size, this aged layer is probably associated with dust because the back trajectories indicate a source from Northern China (Roberts et al., Citation2006).
Over the two marginal seas, the occurrence frequency of α < 1 over the high AOD range was higher than that over the low AOD range, namely, 17% higher for the Bohai–Yellow Sea and 8% higher for the Sea of Japan. The higher occurrence frequency of α < 1 over the high AOD range suggests a greater possibility for presence of giant CCNs than over the low AOD range because α < 1 indicates that aerosol is dominated by coarse mode usually associated with dust and sea salt (Guo et al., Citation2014). Thus, the positive relationship between AI and CER over the high AI range might be caused by giant CCNs, mainly sea salt and the intrusion of dust particles from Asian dust events.
6. Conclusions
We examined the relationships between AI and CER over the northern Pacific using daily MODIS data during the period from 1 December 2002 to 31 December 2014. The northern Pacific was partitioned into five sea regions according to the meteorological background and geographical location. Two different correlations occurred between AI and CER depending on various AI ranges, suggesting that the CER response to increasing AI is non-monotonic.
Over the low AI range, there tends to be a negative relationship between AI and CER, becoming positive at higher AIs. Over the entire AI range, the correlation was significantly positive in summer and significantly negative in the other seasons over the western and eastern subarctic Pacific. Over the North Pacific subtropical gyre, there was a significant negative correlation between AI and CER in all seasons and annually over the entire AI range.
The variability of the ACICER is closely related to water vapour. The ACICER increased with increasing PWV. Once PWV reached a certain high value, the correlation between AI and CER could shift from negative to positive. The critical value of PWV for the two marginal seas was 2–6 times smaller than for the three open oceans. The two marginal seas have high AI and CCN concentrations; giant CCNs and the hygroscopic growth properties of aerosol particles may account for their sensitivity to water vapour for the correlation of AI and CER changing from negative to positive. In addition, for the two marginal seas, the effects of low LCF may partially account for the positive correlation between AI and CER. Over the three open oceans, LCF has a small impact on AI–CER over the high AI range for the three open oceans. Additionally, the stable environment may prevent the Twomey effect because high LTS features positive correlation between AI and CER over the three open oceans.
Mostly in western North Pacific, the error in ACICER emerges when the region size increases (Grandey and Stier, Citation2010). The accuracy of aerosol and cloud properties may induce some uncertainty due to analysis scale and limitations of satellite data such as spatial resolution. Therefore in small-scale regions, higher spatial resolution and independent data are required to be investigated in future. However, in this study the similarities and differences between the coastal and open oceans in North Pacific were compared, the results suggested that the condition of water vapour, environmental thermodynamic state and different cloud regime may worth to be considered during investigating the effect of aerosol on cloud microphysics.
Disclosure statement
No potential conflict of interest was reported by the authors.
Funding
This work was supported by the National Natural Science Foundation of China [grant number 41590874]; the Ministry of Science and Technology of China [grant number 2014CB953703]; the Jiangsu Collaborative Innovation Center for Climate Change.
Supplemental data
Supplemental data for this article can be accessed https://doi.org/10.1080/16000889.2017.1391656.
Supplementary Material
Download MS Word (149.6 KB)Acknowledgements
The MODIS AOD and cloud properties were provided by the Level 1 and Atmosphere Archive and Distribution System (LAADS) of the NASA Goddard Space Flight Center.
References
- Andersen, H. and Cermak, J. 2015. How thermodynamic environments control stratocumulus microphysics and interactions with aerosols. Environ. Res. Lett. 10, 024004.10.1088/1748-9326/10/2/024004
- Breon, F.-M., Tanré, D. and Generoso, S. 2002. Aerosol effect on cloud droplet size monitored from satellite. Science 295, 834–838.10.1126/science.1066434
- Costantino, L. and Bréon, F. M. 2013. Aerosol indirect effect on warm clouds over South-East Atlantic, from co-located MODIS and CALIPSO observations. Atmos. Chem. Phys. 13, 69–88.10.5194/acp-13-69-2013
- Ding, Y. and Chan, J. C. L. 2005. The East Asian summer monsoon: an overview. Meteorol. Atmos. Phys. 89, 117–142.
- Eichler, M. 2012. Causal inference in time series analysis. In: Causality: Statistical Perspectives and Applications (eds. Berzuini, C., P. Dawid and L. Bernardinelli). John Wiley & Sons, Ltd, Chichester, pp. 327–354.10.1002/9781119945710
- Feingold, G., Cotton, W. R., Kreidenweis, S. M. and Davis, J. T. 1999. The impact of giant cloud condensation nuclei on drizzle formation in stratocumulus: implications for cloud radiative properties. J. Atmos. Sci. 56, 4100–4117.10.1175/1520-0469(1999)056<4100:TIOGCC>2.0.CO;2
- Feingold, G., Eberhard, W. L., Veron, D. E. and Previdi, M. 2003. First measurements of the Twomey indirect effect using ground-based remote sensors. Geophys. Res. Lett. 30, 1287.
- Gong, S. L. and Zhang, X. Y. 2008. CUACE/Dust – an integrated system of observation and modeling systems for operational dust forecasting in Asia. Atmos. Chem. Phys. 8, 2333–2340.10.5194/acp-8-2333-2008
- Grandey, B. S. and Stier, P. 2010. A critical look at spatial scale choices in satellite-based aerosol indirect effect studies. Atmos. Chem. Phys. 10, 11459–11470.10.5194/acp-10-11459-2010
- Granger, C. W. J. 1969. Investigating causal relations by econometric models and cross spectral models. Econometrica 37, 424–438.10.2307/1912791
- Gryspeerdt, E. and Stier, P. 2012. Regime-based analysis of aerosol-cloud interactions. Geophys. Res. Lett. 39, L21802.
- Guo, X., Fu, D., Guo, X. and Zhang, C. 2014. A case study of aerosol impacts on summer convective clouds and precipitation over northern China. Atmos. Res. 142, 142–157.10.1016/j.atmosres.2013.10.006
- Huang, J., Lin, B., Minnis, P., Wang, T., Wang, X. and co-authors. 2006. Satellite-based assessment of possible dust aerosols semi-direct effect on cloud water path over East Asia. Geophys. Res. Lett. 33, 1042.10.1029/2006GL026561
- Jin, M. and Shepherd, J. M. 2008. Aerosol relationships to warm season clouds and rainfall at monthly scales over east China: urban land versus ocean. J. Geophys. Res. Atmos. 113, D24S90.
- Jung, J. and Kim, Y. J. 2011. Tracking sources of severe haze episodes and their physicochemical and hygroscopic properties under Asian continental outflow: long-range transport pollution, postharvest biomass burning, and Asian dust. J. Geophys. Res. Atmos. 116, D02206.
- Kato, S., Mace, G. G., Clothiaux, E. E., Liljegren, J. C. and Austin, R. T. 2001. Doppler cloud radar derived drop size distributions in liquid water stratus clouds. J. Atmos. Sci. 58, 2895–2911.10.1175/1520-0469(2001)058<2895:DCRDDS>2.0.CO;2
- Kaufman, Y. J. and Fraser, R. S. 1997. The effect of smoke particles on clouds and climate forcing. Science 277, 1636–1639.10.1126/science.277.5332.1636
- Kaufman, Y. J., Koren, I., Remer, L. A., Rosenfeld, D. and Rudich, Y. 2005. The effect of smoke, dust, and pollution aerosol on shallow cloud development over the Atlantic Ocean. PNAS 102, 11207–11212.10.1073/pnas.0505191102
- Kaufmann, R. K. and Stern, D. I. 1997. Evidence for human influence on climate from hemispheric temperature relations. Nature 388, 39–44.10.1038/40332
- Klein, S. A. and Hartmann, D. L. 1993. The seasonal cycle of low stratiform clouds. J. Climate 6, 1587–1606.10.1175/1520-0442(1993)006<1587:TSCOLS>2.0.CO;2
- Kim, J. H., Yum, S. S., Shim, S., Yoon, S. C., Hudson, J. G. and co-authors. 2011. On aerosol hygroscopicity, cloud condensation nuclei (CCN) spectra and critical supersaturation measured at two remote islands of Korea between 2006 and 2009. Atmos. Chem. Phys. 11, 12627–12645.10.5194/acp-11-12627-2011
- King, M. D., Menzel, W. P., Kaufman, Y. J., Tanre, D., Bo-Cai, G. and co-authors. 2003. Cloud and aerosol properties, precipitable water, and profiles of temperature and water vapor from MODIS. IEEE Trans. Geosci. Remote Sens. 41, 442–458.10.1109/TGRS.2002.808226
- King, N. J., Bower, K. N., Crosier, J. and Crawford, I. 2013. Evaluating MODIS cloud retrievals with in situ observations from VOCALS-REx. Atmos. Chem. Phys. 13, 191–209.10.5194/acp-13-191-2013
- Koren, I., Martins, J. V., Remer, L. A. and Afargan, H. 2008. Smoke invigoration versus inhibition of clouds over the Amazon. Science 321, 946–949.10.1126/science.1159185
- Koren, I., Feingold, G. and Remer, L. A. 2010. The invigoration of deep convective clouds over the Atlantic: aerosol effect, meteorology or retrieval artifact? Atmos. Chem. Phys. 10, 8855–8872.10.5194/acp-10-8855-2010
- Lebsock, M. D., Stephens, G. L. and Kummerow, C. 2008. Multisensor satellite observations of aerosol effects on warm clouds. J. Geophys. Res. Atmos. 113, 2684.10.1029/2008JD009876
- L’Ecuyer, T. S., Berg, W., Haynes, J., Lebsock, M. and Takemura, T. 2009. Global observations of aerosol impacts on precipitation occurrence in warm maritime clouds. J. Geophys. Res. Atmos. 114, D09211.
- Levy, R. C., Mattoo, S., Munchak, L. A., Remer, L. A., Sayer, A. M. and co-authors. 2013. The collection 6 MODIS aerosol products over land and ocean. Atmos. Meas. Tech. 6, 2989–3034.10.5194/amt-6-2989-2013
- Li, J., Han, Z. and Zhang, R. 2014. Influence of aerosol hygroscopic growth parameterization on aerosol optical depth and direct radiative forcing over East Asia. Atmos. Res. 140–141, 14–27.10.1016/j.atmosres.2014.01.013
- Li, J., Wang, Z., Zhuang, G., Luo, G., Sun, Y. and co-authors. 2012. Mixing of Asian mineral dust with anthropogenic pollutants over East Asia: a model case study of a super-duststorm in March 2010. Atmos. Chem. Phys. 12, 7591–7607.
- Liang, L., Di Girolamo, L. and Sun, W. 2015. Bias in MODIS cloud drop effective radius for oceanic water clouds as deduced from optical thickness variability across scattering angles. J. Geophys. Res. Atmos. 120, 7661–7681.10.1002/2015JD023256
- Liu, X., Gu, J., Li, Y., Cheng, Y., Qu, Y. and co-authors. 2013. Increase of aerosol scattering by hygroscopic growth: observation, modeling, and implications on visibility. Atmos. Res. 132–133, 91–101.10.1016/j.atmosres.2013.04.007
- Matsui, T., Masunaga, H., Pielke, R. A. and Tao, W.-K. 2004. Impact of aerosols and atmospheric thermodynamics on cloud properties within the climate system. Geophys. Res. Lett. 31, L06109.
- Matsuki, A., Schwarzenboeck, A., Venzac, H., Laj, P., Crumeyrolle, S. and co-authors. 2010. Cloud processing of mineral dust: direct comparison of cloud residual and clear sky particles during AMMA aircraft campaign in summer 2006. Atmos. Chem. Phys. 10, 1057–1069.
- McComiskey, A. and Feingold, G. 2012. The scale problem in quantifying aerosol indirect effects. Atmos. Chem. Phys. 12, 1031–1049.10.5194/acp-12-1031-2012
- Myhre, G., Stordal, F., Johnsrud, M., Kaufman, Y. J., Rosenfeld, D. and co-authors. 2007. Aerosol-cloud interaction inferred from MODIS satellite data and global aerosol models. Atmos. Chem. Phys. 7, 3081–3101.10.5194/acp-7-3081-2007
- Nakajima, T., Higurashi, A., Kawamoto, K. and Penner, J. E. 2001. A possible correlation between satellite-derived cloud and aerosol microphysical parameters. Geophys. Res. Lett. 28, 1171–1174.10.1029/2000GL012186
- Nakajima, T. Y., Suzuki, K. and Stephens, G. L. 2010. Droplet growth in warm water clouds observed by the a-train. Part II: a multisensor view. J. Atmos. Sci. 67, 1897–1907.10.1175/2010JAS3276.1
- Painemal, D. and Zuidema, P. 2011. Assessment of MODIS cloud effective radius and optical thickness retrievals over the Southeast Pacific with VOCALS-REx in situ measurements. J. Geophys. Res. Atmos. 116, D24206.
- Penner, J. E., Zhou, C. and Xu, L. 2012. Consistent estimates from satellites and models for the first aerosol indirect forcing. Geophys. Res. Lett. 39, L13810.
- Posselt, R. and Lohmann, U. 2008. Influence of giant CCN on warm rain processes in the ECHAM5 GCM. Atmos. Chem. Phys. 8, 3769–3788.10.5194/acp-8-3769-2008
- Quaas, J., Ming, Y., Menon, S., Takemura, T., Wang, M. and co-authors. 2009. Aerosol indirect effects – general circulation model intercomparison and evaluation with satellite data. Atmos. Chem. Phys. 9, 8697–8717.10.5194/acp-9-8697-2009
- Ramanathan, V., Crutzen, P. J., Kiehl, J. T. and Rosenfeld, D. 2001. Aerosols, climate, and the hydrological cycle. Science 294, 2119–2124.10.1126/science.1064034
- Ramaswamy, V., Boucher, O., Haigh, J., Hauglustaine, D., Haywood, J. and co-authors. 2001. Radiative forcing of climate change. In: Climate Change 2001: The Scientific Basis. Contribution of Working Group I to the Third Assessment Report of the Intergovernmental Panel on Climate Change (ed. Houghton, J. T., Y. Ding, D. J. Griggs, M. Noguer, P. J.v. d. Linden and co-authors). Cambridge University Press, Cambridge, pp. 349–416.
- Roberts, G., Mauger, G., Hadley, O. and Ramanathan, V. 2006. North American and Asian aerosols over the eastern Pacific Ocean and their role in regulating cloud condensation nuclei. J. Geophys. Res. Atmos. 111, 81, D13205.10.1029/2005JD006661
- Rosenfeld, D. and Lensky, I. M. 1998. Satellite-based insights into precipitation formation processes in continental and maritime convective clouds. Bull. Amer. Meteor. Soc. 79, 2457–2476.10.1175/1520-0477(1998)079<2457:SBIIPF>2.0.CO;2
- Solomos, S., Kallos, G., Kushta, J., Astitha, M., Tremback, C. and co-authors. 2011. An integrated modeling study on the effects of mineral dust and sea salt particles on clouds and precipitation. Atmos. Chem. Phys. 11, 873–892.10.5194/acp-11-873-2011
- Sorooshian, A., Feingold, G., Lebsock, M. D., Jiang, H. and Stephens, G. L. 2009. On the precipitation susceptibility of clouds to aerosol perturbations. Geophys. Res. Lett. 36, L13803.10.1029/2009GL038993
- Stephens, G. L. and Platt, C. M. R. 1987. Aircraft observations of the radiative and microphysical properties of stratocumulus and cumulus cloud fields. J. Climate Appl. Meteorol. 26, 1243–1269.10.1175/1520-0450(1987)026<1243:AOOTRA>2.0.CO;2
- Stier, P. 2016. Limitations of passive remote sensing to constrain global cloud condensation nuclei. Atmos. Chem. Phys. 16, 6595–6607.10.5194/acp-16-6595-2016
- Tan, S.-C., Yao, X., Gao, H.-W., Shi, G.-Y. and Yue, X. 2013. Variability in the correlation between asian dust storms and chlorophyll a concentration from the North to Equatorial Pacific. PLoS ONE 8, e57656.10.1371/journal.pone.0057656
- Tang, J., Wang, P., Mickley, L. J., Xia, X., Liao, H. and co-authors. 2014. Positive relationship between liquid cloud droplet effective radius and aerosol optical depth over Eastern China from satellite data. Atmos. Env. 84, 244–253.10.1016/j.atmosenv.2013.08.024
- Tao, W.-K., Chen, J.-P., Li, Z., Wang, C. and Zhang, C. 2012. Impact of aerosols on convective clouds and precipitation. Rev. Geophys. 50, RG2001.
- Teller, A. and Levin, Z. 2006. The effects of aerosols on precipitation and dimensions of subtropical clouds: a sensitivity study using a numerical cloud model. Atmos. Chem. Phys. 6, 67–80.10.5194/acp-6-67-2006
- Trenberth, K. E., Fasullo, J. T. and Kiehl, J. 2009. Earth’s global energy budget. Bull. Amer. Meteor. Soc. 90, 311–323.10.1175/2008BAMS2634.1
- Twohy, C. H., Petters, M. D., Snider, J. R., Stevens, B., Tahnk, W. and co-authors. 2005. Evaluation of the aerosol indirect effect in marine stratocumulus clouds: droplet number, size, liquid water path, and radiative impact. J. Geophys. Res. Atmos. 110, D08203.
- Twomey, S. 1977. The influence of pollution on the shortwave albedo of clouds. J. Atmos. Sci. 34, 1149–1152.10.1175/1520-0469(1977)034<1149:TIOPOT>2.0.CO;2
- Twomey, S. A., Piepgrass, M. and Wolfe, T. L. 1984. An assessment of the impact of pollution on global cloud albedo. Tellus 36B, 356–366.10.3402/tellusb.v36i5.14916
- Wang, B. and Lin, H. 2002. Rainy season of the Asian–Pacific Summer Monsoon. J. Climate 15, 386–398.10.1175/1520-0442(2002)015<0386:RSOTAP>2.0.CO;2
- Wang, C. 2013. Impact of anthropogenic absorbing aerosols on clouds and precipitation: A review of recent progresses. Atmos. Res. 122, 237–249.10.1016/j.atmosres.2012.11.005
- Wang, H., Tan, S.-C., Wang, Y., Jiang, C., Shi, G.-Y. and co-authors. 2014. A multisource observation study of the severe prolonged regional haze episode over eastern China in January 2013. Atmos. Env. 89, 807–815.10.1016/j.atmosenv.2014.03.004
- Wang, H., Shi, G. Y., Zhang, X. Y., Gong, S. L., Tan, S. C. and co-authors. 2015. Mesoscale modelling study of the interactions between aerosols and PBL meteorology during a haze episode in China Jing–Jin–Ji and its near surrounding region – Part 2: aerosols’ radiative feedback effects. Atmos. Chem. Phys. 15, 3277–3287.10.5194/acp-15-3277-2015
- Wang, H., Xue, M., Zhang, X. Y., Liu, H. L., Zhou, C. H. and co-authors. 2015. Mesoscale modeling study of the interactions between aerosols and PBL meteorology during a haze episode in Jing–Jin–Ji (China) and its nearby surrounding region – Part 1: aerosol distributions and meteorological features. Atmos. Chem. Phys. 15, 3257–3275.10.5194/acp-15-3257-2015
- Wang, H., Zhu, B., Shen, L., Xu, H., An, J. and co-authors. 2015. Water-soluble ions in atmospheric aerosols measured in five sites in the Yangtze River Delta, China: Size-fractionated, seasonal variations and sources. Atmospheric Environment. Part B, 123, 370–379.
- Wessa, P. 2015. Free statistics software, office for research development and education, version 1.1.23-r7, Online at: http://www.wessa.net/
- Xiao, H., Yin, Y., Jin, L., Chen, Q. and Chen, J. 2014. Simulation of aerosol effects on orographic clouds and precipitation using WRF model with a detailed bin microphysics scheme. Atmos. Sci. Lett. 15, 134–139.10.1002/asl2.2014.15.issue-2
- Yang, S.-H. and Lu, R. 2014. Predictability of the East Asian winter monsoon indices by the coupled models of ENSEMBLES. Adv. Atmos. Sci. 31, 1279–1292.10.1007/s00376-014-4020-8
- Yin, Y., Levin, Z., Reisin, T. G. and Tzivion, S. 2000. The effects of giant cloud condensation nuclei on the development of precipitation in convective clouds – a numerical study. Atmos. Res. 53, 91–116.10.1016/S0169-8095(99)00046-0
- Yuan, T., Li, Z., Zhang, R. and Fan, J. 2008. Increase of cloud droplet size with aerosol optical depth: An observation and modeling study. J. Geophys. Res. Atmos. 113, D04201.
- Zhang, X. Y., Arimoto, R., Zhu, G. H., Chen, T. and Zhang, G. Y. 1998. Concentration, size-distribution and deposition of mineral aerosol over Chinese desert regions. Tellus 50B, 4317–4331.
- Zhang, Z. and Platnick, S. 2011. An assessment of differences between cloud effective particle radius retrievals for marine water clouds from three MODIS spectral bands. Journal of Geophysical Research: Atmospheres. 116, D20215.
- Zhao, T. L., Gong, S. L., Zhang, X. Y., Blanchet, J.-P., McKendry, I. G. and co-authors. 2006. A simulated climatology of Asian dust aerosol and its trans-pacific transport. Part I: mean climate and validation. J. Climate 19, 88–103. DOI:10.110.1175/JCLI3605.1171.