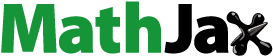
Abstract
The first study of tree-ring stable carbon isotopes in Thailand has demonstrated that stable carbon isotope in northwestern Thailand represents a promising proxy for the temperature reconstruction of core-monsoon periods. A tree-ring δ13C chronology was constructed based on four cores covering the period of 1788–2013. After removing the long-term decreasing trend reflecting atmospheric CO2 concentrations, the ∆13C chronology was able to capture both temperature and hydro-climate signals. ∆13C chronology showed particularly strong and significant negative correlation (r = –0.62, p < 0.0001) with June–September maximum temperature (CRU TS 3.24). The maximum temperature was reconstructed, which explained 37.8% of the variance in the instrumental maximum temperatures over the period of 1901–2013. The maximum temperature reconstruction revealed that four cooler and three warmer periods, as well as a slightly increasing temperature trend, occurred during the late seventeenth to mid-eighteenth centuries, which were followed by severe temperature fluctuations during the twentieth century century. While the sea surface temperature anomaly in the Indian Ocean might not affect the maximum temperature, its unstable relationship with the El Niño-Southern Oscillation (ENSO) was detected. In addition, a close relationship was observed between the maximum temperature and ENSO during the negative phase of the Pacific Decadal Oscillation (PDO), but this relationship was lost during the positive phase of the PDO.
1. Introduction
The spread of dense population, civilization and biodiversity throughout South-East Asia has been shaped and characterized by the influence of the Asian monsoon. Since the beginning of modern civilization, anthropogenic activity has been a potential factor in driving global climate; it tends to increase the global temperature, which causes variable changes in monsoon systems (IPCC, Citation2013) In Thailand, 80% of annual rainfall occurs during the summer monsoon season (May–October), when the feast or famine of a population depends on monsoonal rainfall. Contradictory changes in the Asian monsoon over Thailand under the background of global warming have been investigated due to the interaction between the climate of the Pacific and Indian Oceans and driving forces such as the El Niño-Southern Oscillation (ENSO) and the warm pool in the Indian Ocean (the Indian Ocean Dipole). Climate records obtained using instruments and natural palaeoclimate archives are the keys to understanding the spatial and temporal changes in monsoon mechanisms and their magnitudes. Studies have used instrumental climate data to reveal the relationship between ENSO and the onset of monsoons, which suggested that weak monsoons occurred in this region during El Niño events, and vice versa (Rupakumar and Pant, Citation1997; Hamada et al., Citation2002). In addition, an unsteady relationship has existed between ENSO and the Indian summer monsoon since 1980 (Kumar et al., Citation1999). Due to the south-eastward shift in the descending limb of the Walker circulation during El Niño events, weak correlations between ENSO and Indian summer monsoon rainfall after 1980 have also appeared in summer monsoon rainfall in northern Thailand, and its correlation with rainfall increased in the centre of Thailand (Singhrattna et al., Citation2005).
There are many natural archives of palaeoclimatic information, including ice cores, lake sediment (varves), tree-rings, coral reefs, and pollen, among others (Pielou, Citation1991; Agashe, Citation1995; Bradley, Citation1999; Fagan, Citation2000). Tree-ring analyses, unlike other proxy records, have two great advantages: (1) each ring yields an exactly annual resolution and (2) multiple samples enhance the signal to noise ratio. In tropical and subtropical regions, where rainfall and temperature are not limiting factors for tree growth, proxy data, such as trees that build obvious annual rings, are limited. Three tree species (Tectona grandis, Pinus merkusii and Pinus kesiya) have been used in dendrochronology studies in Thailand and have been considered to be promising climate proxies that record precipitation, temperature and Palmer Drought Severity Index (PDSI) data (Buckley, Barbetti, et al., Citation1995; Pumijumnong et al., Citation1995; Buckley, Duangsathaporn, et al. Citation2007; Buckley, Palakit, et al., Citation2007; Pumijumnong and Eckstein, Citation2010; Pumijumnong, Citation2012). However, a limitation of traditional tree-ring width analysis in this region is that these data usually only reflect climate signals during premonsoon periods.
In recent decades, using new advances in isotopic geochemistry, including a better understanding of the physiological controls on isotopic variations (McCarroll and Loader, Citation2004; McCarroll et al., Citation2009; Leavitt, Citation2010), an increasing number of researchers have been able to reconstruct climate factors using stable isotope ratios in tree-rings in South-East Asia. Tree-ring stable isotopes highlight two advantages: (1) the low-frequency climate signals are well preserved since detrending processes are unnecessary during their reconstructions (McCarroll and Loader, Citation2004; Gagen et al., Citation2007; Szymczak et al., Citation2012); (2) far less samples are required (4–5 in general) for building a reliable chronology, comparing with other tree-ring parameters (Gagen et al., Citation2007; Leavitt, Citation2010; Liu et al., Citation2014). Tree-ring stable oxygen isotope ratios (δ18O) have been rapidly measured throughout South-East Asia. In north-west Thailand, Pinus kasiya was used to study intra-annual variations; it exhibited negative correlations with local July–November precipitation (Zhu et al., Citation2012). Subsequently, July–October precipitation was reconstructed using Pinus merkusii δ18O which showed close relationship with ENSO during the period 1871–2000 (Xu et al., Citation2015). Sano et al. (Citation2012) reconstructed an annual multivariate ENSO Index over the past 300 years using Fokienia hodginsii cellulose δ18O data in Vietnam, and their δ18O chronology exhibited significant correlations with precipitation and PDSI. Furthermore, Xu et al. (Citation2011) indicated that the δ18O chronology in Vietnam showed good agreement with that in Northern Laos. The latter showed a significantly negative correlation with the May–October PDSI and revealed the significant impact of ENSO on tree-ring cellulose in Laos. Although tree ring δ18O data in South-East Asia have been proven to represent a reliable hydroclimatic archive, in order to further understand climate characteristics, stable carbon isotope ratios in tree-rings (δ13C) must be studied. The δ13C values in tree-rings are a result of the fractionation of the stable carbon isotope composition of the atmosphere due to the balance between stomata conduction, the photosynthesis rate and carboxylation (McCarroll and Loader, Citation2004). Therefore in many previous studies, the climatic signals that were captured by δ13C in tree rings include relative humidity, soil moisture, light level and; particularly, temperature (Leavitt et al., Citation2002; Nakatsuka et al., Citation2004 Gagen et al., Citation2007; Liu et al., Citation2012, Citation2014; Zhao et al., Citation2014; Bégin et al., Citation2015) (which are related to stomata conduction and photosynthesis).
In this article, we present a maximum temperature reconstruction spanning 228 years based on δ13C values in a pine tree ring in northwestern Thailand. This reconstruction captured the core-monsoon temperature and revealed increases in temperature fluctuations during the twentieth century. In addition, this 228-year-long temperature reconstruction was used to investigate the influence of the teleconnection of the Pacific and Indian Oceans on northwestern Thailand.
2. Materials and methods
2.1. Study area and climatology
Merkus pines (Pinus merkusii) were collected from the Mae Surin arboretum (18°52′34′′N, 97°56′36′′E) which is located in Mae Hong Son province (MHS), northwestern Thailand (Fig. ). The geography of this site is characterized as a sandstone plateau (612 m a.s.l). The dominant species of the sampling site are two-needle leaf pine (Pinus merkusii) and dipterocarp (Shorea obtuse, Shorea siamensis) (Bumrungsak, Citation2003; Santisuk, Citation2013). In general, the climate of the sampling site is influenced by the south-west monsoon (summer monsoon) and the north-east monsoon (winter monsoon). The south-west monsoon appears from May to October (described as a rainy or wet monsoon season) and brings a warm, moist air mass from the Indian Ocean. The north-east monsoon appears from November to February (described as a cool season) and brings a cold dry air mass from China. According to the climate diagram obtained from the MHS meteorological station during 1951–2013 AD (Fig. ), the dry period (in which there is less precipitation than evaporation) occurs from November to March, while the wet period (in which there is more precipitation than evaporation) occurs from May to October. The mean annual rainfall is 1228 mm; observations indicate that the highest rainfall occurs in August and the lowest rainfall occurs in February. The 63-year mean temperature is 25 °C; the highest mean temperature occurs in April (30 °C) and the lowest occurs in January (21 °C) (Thai Meteorological Department, Citation2015).
Fig. 2. Monthly mean temperature, precipitation and relative humidity data since 1951 in Mae Hong Son.
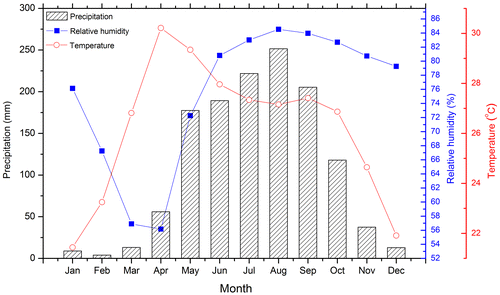
The samples were collected in May 2014, during the transition period between dry and wet seasons. An increment borer with a diameter of 10 mm was used to collect the samples at breast height. The cores were dried at room temperature and sanded until their cross-sections revealed boundaries of rings that were clear enough to produce tree ring measurements. The cores were measured using a LinTab measuring system (with a precision of 0.001 mm) and plotted using a TSAP-Win program (Rinn, Citation2011). To cross-date the tree-ring width series, each individual series was visually compared with the plots graphed using the TSAP program, which were statistically checked by the COFECHA program (Holmes, Citation1983; Grissino-Mayer, Citation2001).
2.2. Cellulose extraction and isotopic measurements
Four cores from four trees with clear ring boundaries and no missing ring were selected for isotopic analysis. During the most recent 100 years, the rings are very narrow, and they contain a few layers of late wood; although the rings during the younger period are much wider, the boundary between early wood and late wood is not clear due to the high amounts of resin in the wood. Therefore, we used the whole annual ring to measure stable carbon isotopes. Each core was cut into very small pieces (with a thickness of <0.5 mm for potential chemical reactions), year by year, using a razor blade under a stereoscope. The Jayme-Wise method was employed to extract α-cellulose (Green, Citation1963). The homogenized cellulose samples were wrapped in tin capsules, and their stable carbon isotope ratios were measured using a continuous flow isotope ratio mass spectrometer (Flash 2000 and Delta V Advantage). The ratios of 13C to 12C are presented using δ notation, which represents the deviation (‰) from a reference standard (IAEA CH3) based on the following equation:(1)
(1)
where Rsample and Rstandard are the 13C/12C ratios of the sample and the standard, respectively.
After each individual δ13C time series was obtained, the first several decades were removed to avoid the juvenile effect (McCarroll and Loader, Citation2004; Leavitt, Citation2010). Then, the remaining individual δ13C time series were defined as PM1b (1788–2013 AD), PM2a (1810–2013 AD), PM4b (1788–2013 AD) and PM5a (1788–2013 AD). Because the atmospheric CO2 concentration has continuously increased since the Industrial Revolution, 13C has become increasingly depleted in the atmosphere, which has caused the long-term declining trend of tree-ring δ13C (McCarroll and Loader, Citation2004; McCarroll et al., Citation2009). To reveal the common climatic signal in the δ13C time series, the effects of atmospheric CO2 should be removed. Here, we used the discrimination method (∆13C, McCarroll and Loader, Citation2004) to calibrate each individual δ13C time series based on the δ13C atmospheric CO2 measurements derived from ice core measurements and direct atmospheric monitoring, using the following equation:(2)
(2)
where δ13Ca is the δ13C of the atmospheric CO2, and δ13Ctree is the δ13C value in the tree-ring.
To diminish the differentiation of the physiological response of each tree to ∆13C values, each ∆13C series was compressed by the average value of each series to yield one single isotopic discrimination value ranging from 1788 to 2013 AD. The running expressed population signal (EPS), which is the chronology confidence estimation commonly used in dendrochronology studies and is based on the number of trees and the mean correlations between trees, was performed using a window of 30 years and a sliding step of 15 years.
2.3. Climate data and statistical analysis
To evaluate the climatic response of tree-ring δ13C, two sources of climatological data were used in this study. First, monthly climatological data (precipitation, temperature, relative humidity and number of rainy days) from 1951–2013 AD were obtained from the MHS meteorological station (269 m a.s.l.), which is located approximately 40 km from the sampling site. Second, six climatic parameters, namely, cloud cover, Diurnal Temperature Range (DTR), precipitation, mean temperature (Tmean), minimum temperature (Tmin), and maximum temperature (Tmax), were obtained from the climatic Research Unit time-series (CRU TS) 3.24 (University of East Anglia Climatic Research Unit et al., Citation2008) in 0.5° × 0.5° gridded data-sets (97°45′–98°15′E, 18°45′–19°15′N) from 1901–2013.
Statistical tests used to investigate the relationship between mean ∆13C time series and monthly climatic data were performed using the DENDROCLIM 2002 software (Biondi and Waikul, Citation2004). DENDROCLIM estimates significant correlation coefficient values using a bootstrapped correlation function for a single interval (i.e. an entire time series correlation) and multiple intervals (i.e. a moving interval and an evolutionary interval). In addition, monthly climatic data were combined between two different periods to determine the influence of seasonal climatic factors on mean ∆13C time series. Then, the climate parameter that showed the best correlation with the ∆13C series was reconstructed using a simple linear regression. Split-period calibration-verification was used to test the stability and reliability of the climate reconstruction model (Fritts, Citation1976; Fritts, Citation1991; Cook and Kairiukstis, Citation1990; Cook et al., Citation1999). The correlation coefficient (r), sign test, reduction of error test (or RE) (Fritts, Citation1976), and coefficient of efficiency (or CE) (Cook et al., Citation1999) were used to verify this reconstruction, along with the split-period calibration-verification.
3. Results
3.1. δ13C and ∆13C
The 4 δ13C series were significantly inter-correlated (p < 0.01), as they yielded r values ranging from 0.66 to 0.84 (Table ). The mean values of the δ13C series fell between –23.58 and –23.48‰, and the differences between the maximum and minimum values of the time series from PM1b, PM2a, PM4b and PM5a were 3.85, 3.58, 4.23 and 3.65‰, respectively. All 4 δ13C series showed slightly deceasing trends throughout almost all of their series, and the linear regression of the average 4 δ13C series over time (1788–2013 AD) yielded a decreasing rate of 0.0046‰ yr−1. However, after 1850 AD (which represents the beginning of the Industrial Revolution), the rate of decrease accelerated to 0.01‰ yr−1, and it dramatically decreased to a rate of 0.028‰ yr−1 after 1960. The Pearson’s correlation between the 4 δ13C series and the δ13C values of atmospheric CO2 ranged from 0.53 to 0.76 (p < 0.01) (Fig. a).
Table 1. The correlation between the original series (δ13C) and detrended series (∆13C) from Mae Hong Son (1788–2013 AD).
Fig. 3. (a) Average δ13C (black) values from 4 trees (grey) and δ13C values in the atmosphere (blue); (b) ∆13C series after removing the long-term decreasing trend of δ13C in the atmosphere; (c) the EPS and Rbar statistics of ∆13C.
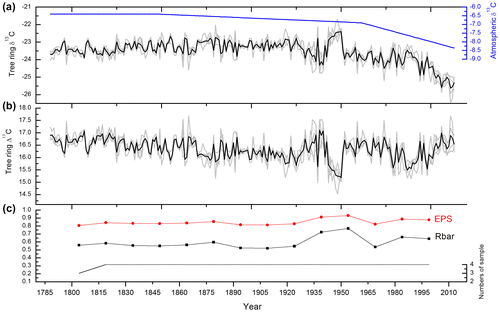
After being corrected to remove the long-term atmospheric decline in δ13C (Fig. b) using the discrimination equation (Equation (Equation2(2)
(2) )), the inter-correlation between each ∆13C series decreased to be lower than the correlation between the original δ13C series (i.e. from r = 0.66–0.84 and p < 0.01 to r = 0.53–0.66 and p < 0.01) (Table ). Considering the high correlation between the original δ13C series and the δ13C of atmospheric CO2, as well as the decrease in the inter-correlation values after removing the atmospheric decline in δ13C, these data indicated that the long-term declining trends in all four original δ13C series from the 4 Pinus merkusii trees were strongly affected by the decreasing trend of atmospheric δ13C due to the increasing concentrations of CO2 in the atmosphere.
Although the inter-correlation values of the ∆13C series were lower than those of the original δ13C series, the mean inter-series correlation (Rbar) among the individual ∆13C series ranged from 0.52 to 0.77 over the period of 1788–2013 AD, and the running expressed population signal (EPS) values, based on a 30-year window and a sliding step of 15 years over the period of 1788–2013 AD, ranged from 0.81 to 0.93 (Fig. c). The analysis of Pinus merkusii and Pinus kasiya tree-ring widths from 16 sites (with approximately 26 trees per site) along the northwestern region to Upper Peninsula of Thailand showed tree ring-width correlations ranging from 0.31 to 0.50 (Pumijumnong and Eckstein, Citation2010). Thus, the ∆13C series yielded a potentially stronger correlation than the tree ring-width time series while also using fewer samples.
3.2. ∆13C climatic response
Four individual ∆13C series were combined into a master series covering a time period of 226 years (Fig. b). Pearson’s correlation was used to identify climatic responses (Fig. ).
Fig. 4. Correlations between tree-ring ∆13C chronology and climatic factors obtained from the MHS station (mean temperature, rainy days and relative humidity) and the CRU TS3.24 (diurnal temperature and maximum temperature) at the 95% significance confidence level. The grey area is the 95% confidence limit after the Bonferroni adjustment.
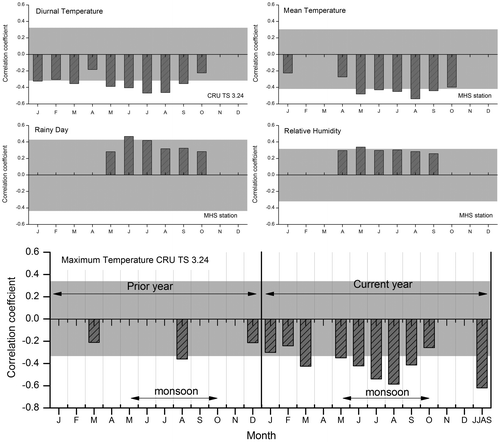
During the common record period of two climatological data-sets (1951–2013 AD), the ∆13C chronology showed a weak positive correlation with precipitation in July (a month into the first half of monsoon season) on both a local (MHS meteorological station; r = 0.28, p < 0.05, n = 63) and regional scale (CRU TS 3.24; r = 0.28, p < 0.05, n = 63), but it exhibited higher correlations with relative humidity and rainy days (MHS meteorological station; r = 0.27–0.34, p < 0.05, n = 63 and r = 0.27–0.47, p < 0.05, n = 52, respectively) from the premonsoon period to the end of monsoon season (April–October).
More significant negative correlations were observed with almost all of the temperature indices, except for the monthly average minimum temperature, which showed no significant correlation with ∆13C chronology throughout the entire year. On a regional scale (CRU TS 3.24), monthly mean temperature exhibited negative correlations in March and May–October (r = –0.46 to –0.19, p < 0.01, n = 113); it exhibited the highest correlation in August, which was the month with the highest amount of rainfall. DTR and Tmax exhibited similar correlations with ∆13C chronology from January to September, except in April (i.e. the hottest month of the year), in which Tmax exhibited no significant correlation and DTR had the lowest correlation among 10 correlated months. The correlation coefficients between DTR and ∆13C chronology ranged from –0.19 to –0.47 (p < 0.01, n = 113), and those between Tmax and ∆13C chronology ranged from –0.26 to –0.58 (p < 0.01, n = 113). On a local scale (MHS meteorological station), tree-ring ∆13C values were significantly negatively correlated (p < 0.01) with monthly mean temperatures from April to October (i.e. r ranged from –0.27 to –0.54). Tree-ring ∆13C values were significantly negatively correlated (p < 0.01) with Tmax values from April to June and August, as their correlation coefficients ranged from –0.30 to –0.47; they were also significantly negatively correlated (p < 0.01) with DTR values from January to June and August, as their correlation coefficients ranged from –0.24 to –0.45. Although ∆13C chronology was still significantly negatively correlated with the MHS meteorological DTR and Tmax data in August (the month with the highest precipitation in the year) in the same way that it was on a regional scale, ∆13C chronology exhibited a better negative correlation with DTR and Tmax during the premonsoon season (i.e. April to June, with the highest correlation on May) than it exhibited with DTR and Tmax on a regional scale, which performed better throughout the whole monsoon season.
After combining data from each climatic parameter over several months to determine the local climate response (MHS meteorological station), ∆13C chronology was negatively correlated with the average mean temperature from the premonsoon period to the end of the monsoon period, i.e. from May to October (r = –0.60, p < 0.001, n = 63) (Fig. ). For the regional climate response (CRU 3.24), ∆13C chronology was negatively correlated with the average maximum temperature during the peak of the monsoon period, i.e. from June to September (r = –0.62, p < 0.001, n = 113) (Fig. ). Previous studies of cambium activity in two species of Thai pines (Pinus kesiya and Pinus merkusii) in the northern region of Thailand (Pumijumnong and Wanyaphet, Citation2006) revealed that the growth of pines started in May and ended in the early dry season (December to January). However, depending on the soil moisture level in the area, the cambium in some trees may still be active through the late dry season (March to April). Consequently, the δ13C values in tree rings should reflect the temperature during their growth period.
Although the Pinus merkusii tree-ring δ13C data in this study captured the climatic signal of the regional Tmax and local Tmean data from May to October, which are related to the growth period, it is still unclear if the cambium activity of the trees in this study ceases in October, which means that the whole ring may potentially reflect the temperature during the time of tree growth, or if the cambium remains dormant throughout the dry season, which means that the whole ring reflects the temperature during the time that the early wood was formed. In the latter case, the δ13C value that is preserved in the whole ring did not exhibit significant correlation with the climate signal from November to April, which may be explained by the different proportions of wood in the two different periods (i.e. the monsoon period and dry period). Because the number of cells in the cambium zone is higher from May to October (i.e. in the monsoon period of the current year) than it is from November to April (i.e. during the following dry period) (Pumijumnong and Wanyaphet, Citation2006), it seems likely that the δ13C values preserved in the higher proportion of wood dominated the δ13C values preserved in the lower proportion of wood.
In general, the maximum temperature, especially during the monsoon season, is more likely to increase with decreasing rainfall because in cloudless conditions or clear weather, daytime temperatures tend to be higher as they receive more direct radiation from the sun. Therefore, the decadal warm period in the Tmax series might reflect drought conditions. To determine whether this hypothesis is consistent with the MHS climatic data, we performed the Pearson’s correlation between the actual June–September maximum temperature (CRU TS 3.24), actual June–September average precipitation (CRU TS 3.24) and June–September average relative humidity (MHS meteorological station). All of the climatological data were calculated as a smoothed 11-year moving average. The fact that Tmax exhibited negative significance with both precipitation (r = –0.5, p < 0.01) and relative humidity (r = –0.75, p < 0.01) confirmed this hypothesis; in particular, its high correlation with relative humidity directly reflected drought conditions. Therefore, we decided to reconstruct June–September maximum temperature data based on the relationship between ∆13C chronology and the CRU TS 3.24 June–September Tmax, which had the strongest relationship among other climate signals and an indirect relationship between maximum temperature and other climate signals.
3.3. Transfer function and reconstruction of June–September mean maximum temperature data
Based on the relationship between ∆13C chronology and the CRU TS 3.24 June–September Tmax, the following simple linear regression model was developed:(3)
(3)
(N = 113, r = –0.62, R2 = 0.384, R2adj = 0.378, F = 69.178, p < 0.0001)
where r is the correlation coefficient between Tmax Jun–Sep and ∆13C chronology. The explained variance of the reconstruction was 38.4% (37.8% after adjusting for the degree of freedom). A comparison of the reconstructed and actual regional June–September Tmax is shown in Fig. . The split-cross calibration and verification were performed to test the stability and reliability of the regression equation (Table ). The values of the reduction error (RE) and the coefficient of efficiency (CE) were positive, which indicated that Tmax Jun–Sep model potentially produced a legitimate reconstruction. Based on the linear regression model (Equation (Equation3(3)
(3) )), the 226-year mean maximum temperature from June–September was reconstructed to cover the period from 1788 to 2013 AD. The mean Tmax Jun–Sep value was 30.09 °C, although it ranged from 29.52 to 30.94 °C, with a standard deviation of ±0.29 °C (Fig. a).
Fig. 5. Comparison of the reconstructed and actual June–September maximum temperature from 1901–2013.
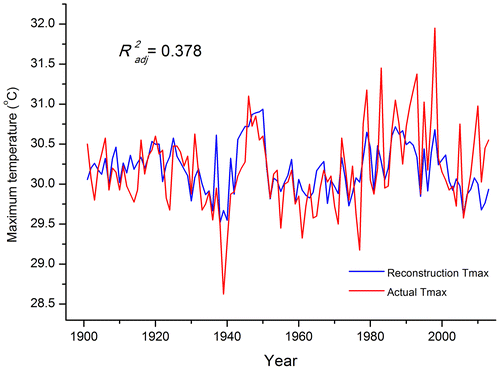
Table 2. The split-cross calibration of the June–September maximum temperature reconstruction.
4. Discussion
4.1. δ13C variability and its climatic implication
In the δ18O-based precipitation reconstruction in northwestern Thailand (Xu et al., Citation2015), the δ18O time series from the 4 Pinus merkusii trees exhibited less inter-tree variability than the ∆13C series in our study, which was expressed in both its greater values of Rbar (0.66–0.83) and EPS (0.88–0.94) obtained from the same amount of samples. This greater inter-tree variability in δ13C values may be related to the different mechanisms that control the δ18O and δ13C values in tree-rings (McCarroll and Loader, Citation2004; Leavitt, Citation2010). Tree-ring cellulose δ18O values obviously reflect the δ18O values of ambient precipitation, which are generally dominated by precipitation and temperature; meanwhile, tree-ring cellulose δ13C values are controlled by the balance between the stomata conductance rate and the photosynthesis rate. Stomatal conductance is influenced by the factors that control ambient water (i.e. precipitation, relative humidity, and soil moisture status, which are generally negative correlated with δ13C), and the photosynthesis rate, which is influenced by the factors that control the ambient temperature and light level (i.e. mean, minimum and maximum temperature and solar irradiation, which are generally positive correlated with δ13C).
In our results, the ∆13C values of Pinus merkusii showed weak correlations with hydroclimatic factors (which modify the stomata conductance rate) but exhibited strong correlations with temperature (which modifies the photosynthetic rate). Many previous studies have reported weak correlations between precipitation and δ13C, thus suggesting that tree-ring δ13C values are less sensitive to precipitation in non-arid areas where ambient water is not a main limiting factor (Warren et al., Citation2001; Porter et al., Citation2009; Bégin et al., Citation2015). The annual precipitation of 1228 mm in our study area supports this hypothesis; although 90% of the annual rainfall occurs from May to October, the average monthly relative humidity during the dry season (November to April) is 68%, which keeps this area far from experiencing moisture stress during the dry season.
4.2. Characteristics of the June–September maximum temperature reconstruction
To investigate the physiology of the non-stationary TmaxJun–Sep reconstruction under shifting climatic conditions, the Bernaola-Galvan Segmentation Algorithm (Bernaola-Galván et al., Citation2001) was run on the Tmax Jun–Sep reconstruction (Fig. b). The following four lengths of segments with different local mean Tmax Jun–Sep values were revealed to be significant at the 0.01 level: 1788–1829 (mean = 29.83 °C), 1830–1869 (mean = 30 °C), 1870–2001 (mean = 30.22 °C) and 2002–2013 (mean = 29.91 °C). During the first two segments, from the late 1700s to the mid-1800s (i.e. 1788 to 1869 AD, which are assumed to be the pre-Industrial Revolution period), the maximum temperature increased slightly at a rate of 0.03 °C/decade, but the mean maximum temperature was obviously lower than that of the third segment (1870–2001), which represents the post-Industrial Revolution period (29.91 °C vs. 30.17 °C). During the third segment (1870–2001), the maximum temperature pattern seemed to be constant compared to the changing rate (+0.004 °C/decade). However, the temperature fluctuations were rather high compared with that of the previous time period (σ ± 0.28 vs. σ ± 0.20). The short fourth segment, which occurred from 2002 to 2013, showed a deceasing trend at a rate of 0.12 °C/decade.
We defined a warmer year as >mean +σ (30.38 °C) and a cooler year as <mean – σ (29.8). We defined 34 warmer years and 40 cooler years, which accounted for 14.91 and 17.70% of the entire reconstruction period, respectively. Interestingly, all of the ten warmest years appeared after 1900 (from highest to lowest temperatures, these years include 1950, 1949, 1948, 1947, 1945, 1946, 1987, 1998, 1989 and 1979), in contrast to the ten coolest years, which were scattered throughout the entire series (from lowest to highest temperatures, these years include 1938, 1807, 1940, 2006, 1821, 1817, 1789, 1936, 1797 and 1939). Consequently, the Mae Hong Son tree-ring δ13C–base maximum temperature data potentially captured the effects of twentieth century global warming, which may not exhibit obvious increases in maximum temperature trends but may instead reflect the increased severity of temperature fluctuations.
To observe decadal maximum temperature patterns, 11-year moving averages were obtained in order to emphasize low-frequency climate signals. Four cool and three warm periods were defined by average temperatures that were lower and higher than the 228-year mean temperature, respectively. The cool periods were 1788–1871, 1931–1939, 1956–1974 and 2004–2013. The three warm periods were 1872–1930, 1940–1955 and 1975–2003. It should be noted that all three of the warm periods appeared after 1850 AD.
The mean temperature from the first cool decades (1788–1829) in the Tmax reconstruction is the lowest among all four of the cool periods, with a mean maximum temperature of 29.82 °C. This condition may result from a negative climate forcing phase. Negative solar forcing and volcanic forcing (which is also known as volcanic-solar downturn) during 1791–1820 has been reported in volcanic forcing reconstructions based on ice core index analyses (Gao et al., Citation2008; Crowley and Unterman, Citation2013) and solar irradiance reconstruction (Shapiro et al., Citation2011; Vieira et al., Citation2011). Two negative climate forcing events coincidentally occurred during the same period, namely, the Dalton minimum (which featured low solar activity due to a low sunspot count) from 1800–1820 AD (Shapiro et al., Citation2011) and the eruptions of two volcanoes in 1809 and 1815 (Gao et al., Citation2008); these events caused a significant decrease in global temperature.
The three warm periods in our monsoon maximum temperature reconstruction, especially those that occurred during the late nineteenth century, coincided with drought periods that have been observed in many tree-ring-based studies. Pumijumnong and Eckstein (Citation2010) reconstructed premonsoon temperatures based on Pinus merkusii and Pinus kesiya tree-ring chronology along northwestern Thailand that reflected drought periods during 1880–1910, 1950–1965 and 1980–1990. Buckley, Palakit, et al. (Citation2007) used teak chronology from Mae Hong Son as a proxy for May–December drought conditions. Mid-eighteenth- and late-nineteenth-century droughts were reflected in this chronology. Additionally, the March–May PDSI reconstruction over northern Vietnam based on Forkienia hodginsii tree-ring widths revealed two prominent droughts that occurred in the mid-eighteenth and late nineteenth century (Sano et al., Citation2008).
4.3. Teleconnection with climate driving force
The coupled ocean–atmosphere system controls the characteristics of the monsoon climate over Thailand. This region is compressed on two opposite sides by the Pacific Ocean and the Indian Ocean, which produce mass driving mechanisms that have broad-scale influence. Generally, monsoon rainfall in Thailand is a result of the interplay between the south-west monsoon, the sub-region of the Indian summer monsoon and the movement of the Inter-Tropical Convergence Zone (ITZC), which brings a warm-moisture air mass from the Indian Ocean towards Thailand from May to October. The Indian Ocean Dipole (IDO), which is an irregular warm–cool oscillation sea surface temperature in the western Indian Ocean, represents a Dipole mode index (DMI) (Saji et al., Citation1999), which is a sea surface temperature index that can be used to calculate the difference between the Western Tropical Indian Ocean index (WTIO) and the southeastern Tropical Indian Ocean index (SEITIO); this was used to investigate the influence of the Indian monsoon on the temperature over northwestern Thailand. Although the precipitation over northwestern Thailand is mainly affected by the Indian summer monsoon, the values of the DMI, WTIO and SEITO indexes showed no significant correlation with the Tmax reconstruction.
The effects of ENSO on the inter-annual and inter-decadal variability of temperature over Thailand have been reported, as El Niño tends to exhibit higher temperatures than normal (with the opposite occurring during La Niña events) (Limsakul and Goes, Citation2008). Similar to the results of our Tmax reconstruction, high peak temperature values were observed in 1919, 1941, 1958, 1972, 1982, 1987, 1995, 1998 and 2009 and they coincide with previous winter and/or current El Niño years. Conversely, no obvious Tmax reconstruction showed low temperature peaks during La Niña years. Thus, the centre Pacific El Niño and the eastern Pacific El Niño exhibit discrete influences on monsoon precipitation (Weng et al., Citation2009). Therefore, the HadlSST Nino 3 index (1870–2013) was selected to represent the eastern Pacific El Niño, and the HadlSST Nino 4 (1870–2013) was selected to represent the centre Pacific El Niño (Weng et al., Citation2009; Yeh et al., Citation2009; Xu et al., Citation2015). The Pearson’s correlation between our maximum temperature reconstruction and the two Nino indexes exhibited weak correlation, but it was significant during the beginning of the monsoon period (May–June), in which rNino 3 vs. Tmax was 0.21 and p < 0.05 and rNino 4 vs. Tmax was 0.19 and p < 0.05. To investigate changes in the long-term relationship between Tmax reconstruction and the Nino index, a 21-year running correlation was performed. In Fig. , the 21-year running correlation between Tmax reconstruction and the 2 Nino indexes exhibited the same positive unstable correlation trends. Low correlations appeared during 1899–1958 and 1984–2013, while higher correlations appeared during 1788–1898 and 1959–1983. The fact that the relationship between Nino 3 SST and Indian summer monsoon rainfall has weakened since 1980 (Kumar et al., Citation1999) may be a reason for the decreasing correlation trend between Tmax reconstruction and the two Nino indexes observed after 1984 in our study. The south-eastward shift of the descending limb of Walker Circulation, which broke down the relationship between ENSO and Indian monsoon, had significantly different responses to precipitation between the northwestern and central regions of Thailand. Decreasing correlations after 1980 were also observed in the relationship between the tree ring δ18O-based July–October precipitation reconstruction in northwestern Thailand and ENSO (Xu et al., Citation2015), but ENSO has since shifted its influence to overcome the precipitation over central Thailand (Singhrattna et al., Citation2005).
Fig. 7. (a) 21-year running correlation between June–September Tmax reconstruction and May–June Nino 3 and Nino 4; (b) May–June sea surface temperature of the PDO index.
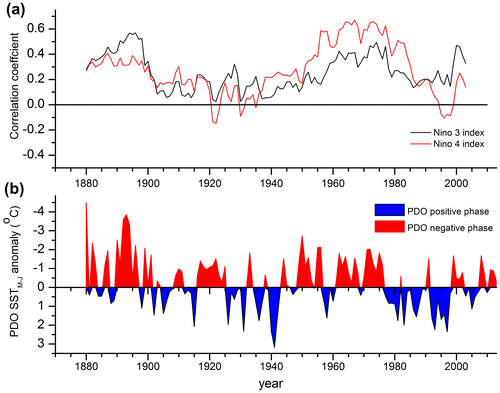
The low–high correlation between Tmax reconstruction and the two Nino indexes appears to reflect an oscillation pattern, which raises more questions about whether there are any possible phenomena in the Pacific Ocean that could have produced this pattern. This was investigated using the warm–cool phase (positive–negative phase) of the Pacific Decadal Oscillation (PDO) index. Although the May–June PDOersst index (https://www.ncdc.noaa.gov/teleconnections/pdo/) from 1880–2013 did not show significant correlations with the Tmax reconstruction, a 21-year running correlation between Tmax reconstruction and the PDO index tended to exhibit a positive correlation during periods in which the Tmax reconstruction and the Nino index exhibited high correlations, and they exhibited a negative correlation during periods in which the Tmax reconstruction and Nino index exhibited low correlations (Fig. a). Interestingly, the positive phase of PDO seems to decrease the relationship between Tmax over northern Thailand and ENSO (and vice versa) (Fig. b). The effects of PDO and ENSO on each other have been explained in terms of their magnitudes. Gershunov and Barnett (Citation1998) concluded that highly positive PDO values may lead to stronger El Niño events and, conversely, that stronger La Niña events tended to occur during periods of highly negative PDO values. However, it still is too early to conclude that PDO exerts an influence on Tmax in northwestern Thailand or even physically controls ENSO; thus, further investigation is needed.
5. Conclusions
The stable carbon isotopes of the Merkus pines in northwestern Thailand significantly captured temperature signals over the core-monsoonal season. Thus, a 226-year June–September maximum temperature record extending back to 1788 AD was reconstructed based on ∆13C chronology. The analysis of climatic shifting conditions revealed a slightly increasing trend in maximum temperature before 1869 AD, followed by increasing temperature fluctuations. In addition, warm period from 1872–1930 observed in this reconstruction were consistent with the drought periods that have been observed during the late nineteenth century in tree-ring-based studies in Thailand and Vietnam. Unstable relationships were detected between the May–June Nino 3 and Nino 4 indexes and our reconstruction; in particular, a weaker relationship after 1980 may be related to a shift in the descending limb of the Walker Circulation. Furthermore, we discovered that this unstable relationship coincided with PDO; specifically, negative phases of PDO tend to increase this relationship and vice versa.
Disclosure statement
No potential conflict of interest was reported by the authors.
Funding
This study was jointly supported by Grants from the CAS Key Research Program of Frontier Sciences [grant number QYZDJ–SSW–DQC021], [grant number NSFC41630531], [grant number XDPB05], [grant number GJHZ1777]; the Key Project of IEECAS and the SKLLQG.
References
- Agashe, S. N. 1995. Paleobotany: Plants of the Past, their Evolution, Paleoenvironment and Application in Exploration of Fossil Fuels Science Publishers Inc., New Hampshire, p. 359.
- Bégin, C., Gingras, M., Savard, M. M., Marion, J., Nicault, A. and co-authors. 2015. Assessing tree-ring carbon and oxygen stable isotopes for climate reconstruction in the Canadian northeastern boreal forest. Palaeogogr. Palaeocl. 423, 91–101.10.1016/j.palaeo.2015.01.021
- Bernaola-Galván, P., Ivanov, P. C., Nunes Amaral, L. A. and Stanley, H. E. 2001. Scale invariance in the nonstationarity of human heart rate. Phys. Rev. Lett. 87(16), 843.10.1103/PhysRevLett.87.168105
- Biondi, F. and Waikul, K. 2004. DENDROCLIM2002: a C++ program for statistical calibration of climate signals in tree-ring chronologies. Comput. Geosci. 30, 303–311.10.1016/j.cageo.2003.11.004
- Bradley, R. S. 1999. Paleoclimatology: reconstructing climates of the quaternary, 2nd ed. Academic Press, San Diego, CA, p. 613.
- Buckley, B. M., Barbetti, M., Watanasak, M., D’Arrigo, R. D., Boonchirdchoo, S. and co-authors. 1995. Dendrochronological investigations in Thailand. IAWA J. 16(4), 393–409.10.1163/22941932-90001429
- Buckley, B. M., Duangsathaporn, K., Palakit, K., Butler, S., Syhapanya, V. and co-authors. 2007. Analyses of growth rings of Pinus merkusii from Lao P.D.R. Forest Ecol. Manag. 253(1–3), 120–127.10.1016/j.foreco.2007.07.018
- Buckley, B. M., Palakit, K., Duangsathaporn, K., Sanguantham, P. and Prasomsin, P. 2007. Decadal scale droughts over northwestern Thailand over the past 448 years: links to the tropical Pacific and Indian Ocean sectors. Clim. Dynam. 29(1), 63–71.10.1007/s00382-007-0225-1
- Bumrungsak, J. 2003. Response of the tree-ring of Pinus merkusii to climate: case study in Mae Hong Sorn province, Suphanburi province and Phetchaburi Province. Thesis. Mahidol University, Nakorn Pathom, 127 pp.
- Cook, E. R. and Kairiukstis, L. A. 1990. Methods of Dendrochronology: applications in the environmental Kluwer, Dordrecht, 394 pp.10.1007/978-94-015-7879-0
- Cook, E. R., Meko, D. M., Stahle, D. W. and Cleaveland, M. K. 1999. Drought reconstructions for the continental United States. J. Climate 12, 1145–1162.10.1175/1520-0442(1999)012<1145:DRFTCU>2.0.CO;2
- Crowley, T. and Unterman, M. 2013. Technical details concerning development of a 1200-year proxy index for global volcanism. Earth Syst. Sci. Data 5, 187–197.10.5194/essd-5-187-2013
- Fagan, B. 2000. The Little Ice Age: How climate made history Basic Books, New York, 276 pp.
- Fritts, H. C. 1976. Tree rings and climate Academic Press, New York, 567 pp.
- Fritts, H. C. 1991. Reconstructing Large-scale Climatic Patterns from Tree-ring Data: a Diagnostic Analysis The University of Arizona Press, Tucson, AZ, 286 pp.
- Gagen, M., McCarroll, D., Loader, N. J., Robertson, L., Jalkanen, R. and co-authors. 2007. Exorcising the ‘segment length curse’: summer temperature reconstruction since AD 1640 using non-detrended stable carbon isotope ratios from pine trees in northern Finland. Holocene 17, 435–446.10.1177/0959683607077012
- Gao, C., Robock, A. and Ammann, C. 2008. Volcanic forcing of climate over the past 1500 years: An improved ice core-based index for climate models. J. Geophy. Res. 113, 1657. DOI: 10.1029/2008JD010239.
- Gershunov, A. and Barnett, T. P. 1998. Interdecadal modulation of ENSO teleconnections. Bull. Am. Meteorol. Soc. 79(12), 2715–2725.10.1175/1520-0477(1998)079<2715:IMOET>2.0.CO;2
- Green, J. W. 1963. Cellulose nitrate. In: Methods in Carbohydrate Chemistry (ed. R. L. Whistler, J. W. Green and J. N. BeMiller) Vol. 3, Academic Press, New York, 213 pp.
- Grissino-Mayer, H. D. 2001. Evaluating crossdating accuracy: a manual and tutorial for all computer program COFECHA. Tree-Ring Res. 57(2), 205–221.
- Hamada, J., Yamanaka, M. D., Matsumoto, J., Fukao, S., Winarso, P. A. and Sribimawati, T. 2002. Spatial and temporal variations of the rainy season over Indonesia and their link to ENSO. J. Meteorol. Soc. Jpn. 80, 285–310.10.2151/jmsj.80.285
- Holmes, R. L. 1983. Computer-assisted quality control in tree-ring dating and measurement. Tree-Ring Bull. 43, 69–78.
- IPCC. 2013. Climate Change 2013: The Physical Science Basis. Contribution of Working Group I to the Fourth Assessment Report of the Intergovernmental Panel on Climate Change. Cambridge University Press, Cambridge, United Kingdom and New York, NY.
- Kumar, K. K., Rajagopalan, B. and Cane, M. 1999. On the weakening relationship between the Indian Monsoon and ENSO. Science 284(5423), 2156–2159. DOI: 10.1126/science.284.5423.2156.
- Leavitt, S. W. 2010. Tree-ring C–H–O isotope variability and sampling. Sci. Total Environ. 408(22), 5244–5253.10.1016/j.scitotenv.2010.07.057
- Leavitt, S. W., Wright, W. E. and Long A. 2002. Spatial expression of ENSO, drought, and summer monsoon in seasonal d13C of ponderosa pine tree rings in southern Arizona and New Mexico. J. Geophys. Res.-Atmos. 107(D18), 4319.
- Limsakul, A. and Goes, J. I. 2008. Empirical evidence for interannual and longer period variability in Thailand surface air temperatures. Atmos. Res. 87(2), 89–102.10.1016/j.atmosres.2007.07.007
- Liu, Y., Wang, R., Leavitt, S. W., Song, H., Linderholm, H. W. and co-authors. 2012. Individual and pooled tree-ring stable-carbon isotope series in Chinese pine from the Nan Wutai region, China: Common signal and climate relationships. Chem. Geol. 330, 17–26.10.1016/j.chemgeo.2012.08.008
- Liu, Y., Wang, Y., Li, Q., Song, H., Linderholm, H. W. and co-authors. 2014. Tree-ring stable carbon isotope-based May–July temperature reconstruction over Nanwutai, China, for the past century and its record of 20th century warming. Quat. Sci. Rev. 93, 67–76.10.1016/j.quascirev.2014.03.023
- McCarroll, D., Gagen, M. H., Loader, N. J., Robertson, I., Anchukaitis, K. J. and co-authors. 2009. Correction of tree ring stable carbon isotope chronologies for changes in the carbon dioxide content of the atmosphere. Geochim. Cosmochim. Acta 73(6), 1539–1547.10.1016/j.gca.2008.11.041
- McCarroll, D. and Loader, N. J. 2004. Stable isotopes in tree rings. Quat. Sci. Rev. 23(7–8), 771–801.10.1016/j.quascirev.2003.06.017
- Nakatsuka, T., Ohnishi, K., Hara, T., Sumida, A., Mitsuishi, D. and co-authors. 2004. Oxygen and carbon isotopic ratios of tree-ring cellulose in a conifer-hardwood mixed forest in northern Japan. Geochem. J. 38, 77–88.10.2343/geochemj.38.77
- Pielou, E. C. 1991. After the Ice Age: The Return of Life to Glaciated North America. The University of Chicago Press, Chicago, IL, 366 pp.10.7208/chicago/9780226668093.001.0001
- Porter, T. J., Pisaric, M. F. J., Kokelj, S. V. and Edwards, T. W. D. 2009. Climatic Signals in δ13C and δ18O of Tree-rings from White Spruce in the Mackenzie Delta Region. Arctic Antarctic Alpine Res. 41(4), 497–505.10.1657/1938-4246-41.4.497
- Pumijumnong, N. 2012. Dendrochronology in Southeast Asia. Trees 27(2), 343–358.
- Pumijumnong, N. and Eckstein, D. 2010. Reconstruction of pre-monsoon weather conditions in northwest Thailand from the tree-ring widths of Pinus merkusii and Pinus kesiya. Trees 25(1), 125–132.
- Pumijumnong, N., Eckstein, D. and Sass, U. 1995. Tree-ring research on Tectona Grandis in Northern Thailand. IAWA J. 16(4), 385–392.10.1163/22941932-90001428
- Pumijumnong, N. and Wanyaphet, T. 2006. Seasonal cambial activity and tree-ring formation of Pinus merkusii and Pinus kesiya in Northern Thailand in dependence on climate. Forest Ecol. Manag. 226(1–3), 279–289.10.1016/j.foreco.2006.01.040
- Rinn, F. 2011. TSAP-WinTM: Time series Analysis and Presentation for Dendrochronology and Relate Applications. Heidelberg, 92 pp.
- Rupakumar, K. and Pant, G. B. 1997. Climate of South Asia John Wiley and Sons, Chichester, 344 pp.
- Saji, N. H., Goswami, B. N., Vinayachandran, P. N. and Yamagata, T. 1999. A dipole mode in the tropical Indian Ocean. Nature 401, 360–363.
- Sano, M., Buckley, B. M. and Sweda, T. 2008. Tree-ring based hydroclimate reconstruction over northern Vietnam from Fokienia hodginsii: eighteenth century mega-drought and tropical Pacific influence. Clim. Dynam. 33(2), 331–340.
- Sano, M., Xu, C. and Nakatsuka, T. 2012. A 300-year Vietnam hydroclimate and ENSO variability record reconstructed from tree ring δ18O. J. Geophys. Res. 117(D12), D12115.
- Santisuk, T. 2013. Forest of Thailand. Forest Herbarium Department of National Park, Wildlife and Plant Conservation Religious printing house, Bangkok, 124 pp.
- Shapiro, A. I., Schmutz, W., Rozanov, E., Schoell, M., Haberreiter, M. and co-authors. 2011. A new approach to the long-term reconstruction of the solar irradiance leads to large historical solar forcing. Astron. Astrophys. 529, A67.10.1051/0004-6361/201016173
- Singhrattna, N., Rajagopalan, B., Kumar, K. K. and Clark, M. 2005. Interannual and interdecadal variability of Thailand summer monsoon season. J. Clim. 18(11), 1697–1708.10.1175/JCLI3364.1
- Szymczak, S., Joachimski, M. M., Bräuning, A., Hetzer, T. and Kuhlemann, J. 2012. Are pooled tree ring δ13C and δ18O series reliable climate archives? – A case study of Pinus nigra spp. laricio (Corsica/France). Chem. Geol. 308–309, 40–49.10.1016/j.chemgeo.2012.03.013
- Thailand Meteorological Department. 2015. Average Rainfall and Temperature Data from Mae Hong Son Station. Online at: http://www.tmd.go.th
- University of East Anglia Climatic Research Unit, Jones, P. D. and Harris, I. C. 2008. Climatic Research Unit (CRU) time-series datasets of variations in climate with variations in other phenomena. NCAS British Atmospheric Data Centre. Online at: http://catalogue.ceda.ac.uk/uuid/3f8944800cc48e1cbc29a5ee12d8542d
- Vieira, L. E. A., Solanki, S. K., Krivova, N. A. and Usoskin, I. 2011. Evolution of the solar irradiance during the Holocene. Astron. Astrophys. 531, A6.10.1051/0004-6361/201015843
- Warren, C. R., McGrath, J. F. and Adams, M. A. 2001. Water availability and carbon isotope discrimination in conifers. Oecologia 127(4), 476–486.10.1007/s004420000609
- Weng, H., Behera, S. K. and Yamagata, T. 2009. Anomalous winter climate conditions in the Pacific rim during recent El Niño Modoki and El Niño events. Clim. Dynam. 32(5), 663–674.10.1007/s00382-008-0394-6
- Xu, C., Pumijumnong, N., Nakatsuka, T., Sano, M. and Li, Z. 2015. A tree-ring cellulose δ18O-based July–October precipitation reconstruction since AD 1828, northwest Thailand. J. Hydrol. 529, 433–441.10.1016/j.jhydrol.2015.02.037
- Xu, C., Sano, M. and Nakatsuka, T. 2011. Tree ring cellulose δ18O of Fokienia hodginsii in northern Laos: A promising proxy to reconstruct ENSO? J. Geophys. Res. 116(D24), D24109.
- Yeh, S.-W., Kug, J.-S., Dewitte, B., Kwon, M.-H., Kirtman, B. P. and co-authors. 2009. El Niño in a changing climate. Nature 461, 511–514.10.1038/nature08316
- Zhao, X., Zheng, Z., Shang, Z., Wang, J., Cheng, R. and co-authors. 2014. Climatic information recorded in stable carbon isotopes in tree rings of Cryptomeria fortunei, Tianmu Mountain, China. Dendrochronologia 32(3), 256–265.10.1016/j.dendro.2014.06.002
- Zhu, M., Stott, L., Buckley, B. and Yoshimura, K. 2012. 29th century seasonal moisture balance in Southeast Asian montane forests from tree cellulose δ18O. Clim. Change, 1–13. DOI: 10.1007/s10584-012-0439-z.