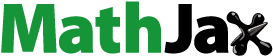
ABSTRACT
The paper situates personalization by comparing widely used numbering practices on social media and other digital platforms. It draws on A.N. Whitehead's analysis of approximation to identify how probabilities and hashes, two key approximating practices, combine to configure platforms and individual users. It shows how personalization in a typical social media setting, the Instagram Explore Page, both distributes individual differences in a statistical manifold and indexes a state of affairs of persons, things, transactions, times and places in numbers such as hashes. Approaching personalization as a practice of entangled approximations, I suggest, shows how relational mappings developed by social media platform overflow the cultural-economic logic of targetted advertising. I argue that the combination of probabilities and hashes, or statistical manifolds and distributed coordination practices, articulate new versions of the some-any relationships embedded in many facets of social life. As approximations, these numberings point to the possibility of new critical framings of technical ensembles and their capacity to condition the formation of groups.
Ganaele Langlois and Greg Elmer suggest that
social media are attempting to establish themselves as the unavoidable mediators for all aspects of life, with a capacity to act simultaneously at the molar level of large-scale social shaping of attitudes and habits and the molecular level of personalized targeting of users (Langlois and Elmer Citation2019, 245).
It might be possible for social theory, with the support of social studies of technology, to make sense of this transition from personal to impersonal by following commonly computed numbers. This paper tracks two treatments of number – numberings – found in social media and platforms more generally: probabilities and hashes. Although it is tempting to picture what is happening on social media platforms in terms of macro/micro-social aspects of life, or large-scale attitudes/habits versus individual users, or network effects and contagions, the movement of numbers does not fit that partitioning of scales. There are transformation of numbering practices running through platform developments of the last few decades, some of them associated with architectures of information retrieval and coordination of resources, some of them taking root in the processes of economization of digital media.
If we follow the numberings, it might be possible to not only track the expansive dynamics of impersonal-personalization, but sense how they overflow the platforms. The hash and the probability estimate, the two numbering practices discussed here, subtend dynamics in contemporary ensembles that drive hyper-convergent platforms and hyper-divergent collectives. The premise of the paper is that the presence of platforms as a major coordinating form in contemporary life depends heavily on numberings running through technical ensembles. Nearly all contemporary platform personalization is an explicitly calculated approximation. Conversely, it is hard to make sense of personalization, its limits and alternatives, without making understanding how technical ensembles provisionally stabilize as platforms. The two numberings discussed here are approximations that translate the relation potentials of ensembles into orderings and enumerations that can filter, rank and recommend but also distribute, share, coordinate and stabilize ensembles as platforms.
Approximating a
At core, at the on-chip level of computation, a major part of platform processing is engaged in approximation. For instance, on the image-sharing platform Instagram's ‘Explore Page’, user a queries for the hashtag ‘#platform’. The servers respond with an indefinite scrolling grid of photographic images. Most days, the page shows many shoes, and particularly shoes with thick soles or high heels. Images of platform shoes, boots and sandals are followed by train station platforms, places where groups of people go to get on trains, as in bleak snow-covered platforms in Russia or crowded bullet-train platforms in Japan, with an occasional Baker St-style London tube image, or Platform at Kings Cross. Viewing and diving platforms pop up occasionally too. Shoes and public transport don't have any strong resonance with each other but perhaps the mixture of fashion and infrastructure is worth contemplating. It seems to literally figure the simultaneous personal and large-scale processes Langlois and Elmer describe. Notably, none of the images show platforms such as Instagram or its ilk (SnapChat, Weibo, Tiktok, Twitter, etc.). The platform themselves remains un-imaged.
Images of personal effects and impersonal urban architectures roll across Instagram and similar social media platforms (Manovich Citation2020). They enact complicated processes of subject formation, group identity and social ordering. The intensely-felt attachment-affects such as depression, loneliness and sadness revealed in Facebook's internal research into the depressive dynamics of Instagram (Milmo and editor Citation2021) and already noted by media theorists (Lovink Citation2019), the sometimes fragile constructions of platform-mediated social realities (Couldry and Hepp Citation2018) and the now well-documented corrosively stratifed subjectification driven by reputational and recommendation systems (Bucher Citation2017) are perhaps only part of what is at stake in personalization.
The platform images that user a flicks through on the Instagram Explore Page are a personalized selection of some images from a large collection. Such selections from collections have become common in the last decade, 2010–2020, of platform ensembles. Selection is shaped by a numerical approximation. In his non-technical but profound An Introduction to Mathematics, A.N. Whitehead frames approximation as a limited relation between some and any:
A set of numbers approximates to a number a within a standard k, when the numerical difference between a and every number of the set is less than k. Here k is the “standard of approximation.” Thus the set of numbers 3, 4, 6, 8, approximates to the number 5 within the standard 4. (Whitehead Citation2017, 94)
Reviews, postings by Instagram users and reports written by Instagram software engineers help sketch the trajectory of approximation out from various sides. A Wired review of the Explore Page accents variety in the set of images:
Now Explore is more like a personal inside joke, a collection of things only you could possibly understand. You can actually see it chase your affections. Watch a bunch of wrestling videos and the WWE comes crashing into your feed. Tap through a few #transformationtuesday posts and Instagram rushes to show you more. In a way, Explore makes delivers the great promise of the internet as a place to find people just like you (Pierce Citation2017).
From their side as developers working on the platform, Instagram engineers highlight, against sometimes monolithic critical understandings of platforms, the specific standards and accompanying measures of difference active on the Explore Page. Like the Amazon website landing page, Instagram's Explore Page is a valuable facet of the platform. The set of 24 or so images first shown there have the potential to send users on meandering trails of distracted attention. Instagram places 90 million or so personalizing predictions per second on this page (Medvedev Citation2019). The image sets are drawn from the billions of photographs and videos stored on the platform by a retrieval pipeline whose focus does not concern the ostensible content of images. It instead approximates the account of some Instagrammer, user a, using the set of proximate users in the platform ‘community’.
The Instagram engineers write:
we created a retrieval pipeline that focuses on account-level information rather than media-level. By building account embeddings, we’re able to more efficiently identify which accounts are topically similar to each other. We infer account embeddings using ig2vec, a word2vec-like embedding framework. Typically, the word2vec embedding framework learns a representation of a word based on its context across sentences in the training corpus. Ig2vec treats account IDs that a user interacts with — e.g., a person likes media from an account — as a sequence of words in a sentence (Medvedev Citation2019)
As is often the case in machine learning pursued by major Web 2.0 platforms, the widely-used and emulated Word2vec model depends on very large training datasets (∼6 billion words). Word2vec predicts contextual relations between words: if Paris is to France, Athens is what? ‘Greece’ Word2vec responds. Word2vec represents each word in the data as a vector or a set of coordinates in a high-dimensional space. Words with neighbouring coordinates share contexts. For Ig2vec, the analogy would run: user a is to platform shoes as account b is to what? Platform sandals? In both Word2vec and Ig2Vec, the model approximates by measuring proximity between vectors. Given a word or an account, word2vec or Ig2Vec locates nearby or proximate vectors. Context has a parallel sense in the two models. Word2vec wrangles the fluxing sensitivities of word occurrence to the words around them as a set of probabilities. It predicts sets of preceding or following words as a set of joint probabilities for a given word. Similarly, when Instagram accounts become ‘embeddings’ in ig2vec, all the recorded account actions and interactions such as scrolling, liking, following, sharing, clicking ‘show fewer like this’, or saving, etc, form a context or an ambient set approximating a given user. The computation of embeddings – vectors in a limited-dimensional space (100 or so dimensions) that approximate the inaccessibly fluxing relations of the higher dimensional vectors (‘65 billion features’ according to the Instagram engineers) – defines the standard of approximation that guides the set of numbers or the selection of images put on the Explore Page.
Personal embeddings: statistical platform manifolds
Several observations flow from this brief description of Ig2Vec. First, the embedding approach is an approximation, a mapping of ‘any’ to ‘some’ within a ‘standard of approximation’ in Whitehead's sense. Although first developed as a reduced or compressed representation of the slender probabilities of co-occurrences of words, it lends itself to many variations and imitations. This mode of numbering anything through other things is not specific to Instagram and its pipelines. Accounts on Instagram, accommodation on Airbnb, dishes on UberEats, videos to watch on Youtube, and many other platform personalizations have taken an embeddings approach. Association with images, words, people, places, things and events have been widely subject to embedding treatments.
Second, and importantly for present purposes, here, as in many other machine learning settings, rather than moving from the personal to the impersonal, or vice versa, or from micro to macro, the embeddings re-map the relation between scales. At a general level, this is not a new social-theoretical point. Scaleability or shifting in scales is, for instance, a staple of actor-network theory (Latour et al. Citation2012). But the embedding approximation of account/user similarities propagates a transformation specific to large ensembles. It recapitulates a solution to a problem first posed in terms of the relation between macroscopic attributes and molecular movements in physical systems.
The approximation driving the selection of images to show to user a in fact uses the ensemble abstraction formulated in mid-nineteenth-century physics when Ludwig Boltzmann developed statistical mechanics as a way of re-conceptualizing macroscopic properties such as temperature, pressure and volume as system microstates defined by the locations and movements of individual particles. In statistical mechanics, moving particles are not studied in isolation but in collections of slightly varying copies known as ensembles. The concept of an ensemble, formalized by Josiah Gibbs at the start of the twentieth century (Gibbs Citation1902), replaces the single closed system of particles with many copies of the system. ‘Instead of studying the system at a single microscopic state’, write the authors of a recent review, ‘ensemble theory considers the system's microscopic state as unknown and employs a probability density function to describe each state's probability’ (Gao Citation2022). The probability density function, known as the Boltzmann distribution, spans all the microstates in the ensemble and assigns them various probabilities, depending on the macroscopic properties of the system.Footnote1
An ensemble in this sense, a sense that might be useful to think with, is the uneven, always fluxing, manifold of many versions of a system. Just as statistical mechanics used probability distributions to approximate the movements of particles using the now statistical properties of pressure, heat, or temperature, we might say that the contemporary platform ensembles reconfigure social orderings ranging from personal to impersonal as a flickering set of states ranging across probability distributions. In the millions, perhaps billions of approximations streaming each second across platforms such as Instagram, Youtube, Spotify, Uber or Tiktok, probability distributions – embeddings – approximated from microstates configure new microstates. The separation between the molecule/individual and the molar/large-scale social shaping does not capture the ensemble embeddings. The ensemble construct that first changed how physics thought about matter and energy renders the macrostate as an effect of many systems, probable and improbable. The ensemble substitutes many copies of the system in different microstates for the state of the system. Note that ensembles are inherently multiple in the sense that any a can be approximated by a set. Rather than the biopolitical averaging of many by a single norm, the embedding numbering presents any particular state of affairs in terms of its closest matches or approximations. Recommender systems such as the Instagram Explore Page are just one point of the surface of the ensemble, a surface populated by different, even disparate copies and embeddings of the same data and data sources.
Versioning through hashing
The versioning of technical ensembles as statistical platforms manifolds is remarkably widespread, and perhaps, as Langlois and Elmer put it, ‘unavoidable’. But the ensemble dynamics that support platforms cannot rely on embeddings, no matter how they multiply or approximate more closely. Perhaps the ensemble in its dynamic plurality can be glimpsed in a second stream of numbers, hashes, produced by hashings even more numerous than the embeddings. The hash, again following Whitehead's formulation, is a relation between any and some, an approximation bounded by a standard k. The standards of approximation in hashing differ greatly from those in the embeddings we have just discussed.
Before they appear in user a’s Instagram Explore Page, the shoes and trains have traversed Facebook/Meta’s ‘perceptual hashing’ algorithm, PDQ:
Known as PDQ and TMK+PDQF, these technologies are part of a suite of tools we use at Facebook to detect harmful content, and there are other algorithms and implementations available to industry such as pHash, Microsoft's PhotoDNA, aHash, and dHash. … These technologies create an efficient way to store files as short digital hashes that can determine whether two files are the same or similar, even without the original image or video (Kerl [Citation2015] Citation2022).
The many hashes moving through code such as PDQ and IGDiskCache drift in the numeric haze pervading contemporary technical ensembles. Listing them is relatively easy: heavily used cryptographic hash function such as SHA-1/2/3 (Secure Hash Algorithm 1, 2 and 3) can be found in the ubiquitous Transport Layer Security (TLS) on which most web, email, instant messaging and voice-over-IP communications depend; hash checksums and hash message digests are used to check file and message integrity when files are copied or moved, hash message digests validate digital signatures; SHAs form the basis of the important platform-technical development practices known as code-versioning used in Github and the like; peer-to-peer file-sharing systems such as BitTorrent, and centrally-used distributed datastores such as Redis, Cassandra, MongoDB and Couchbase are based on hashtables; proof-of-work hash trees in cryptocurrencies and blockchains such as Ethereum or Bitcoin, and proof-of-stake hashing in ‘Web3.0 applications’ such as NFTs (None Fungible Tokens), dApps (de-centralized Applications), DAOs (Distributed Autonomous Organizations) and Defi (De-centralized finance) more generally are key to the emerging post-platform configurations of ensembles. Hashes cross-hatch social media platforms. When Mazdak Hashemi, VP of Infrastructure and Operations at Twitter, writes that 21.2% of Twitter's hardware infrastructure is working on key-value storage engines such as Redis and Memcached (Hashemi Citation2017), and these modes of storage are critical to the observable liveliness of Twitter traffic, he implicitly affirms the vast hashing substrate of that platform.
If hashes pervade platforms in their many configurations, what is it about this numbering that makes them so numerous? What kind of approximation, or mapping of any to some, are they? A hash function – the term is said to date from the 1950s – is a computation that maps any particular state of affairs, some data or content, a configuration, a transaction, a version or elements in a set to an integer or a whole number, a hash (or hashcode). In a terminal emulator, the screen console that many developers work in, the commonly used SHA256 hash function maps the word ‘platforms’ to a 64-digit-long hexadecimal (base-16) number shown in Figure 1, itself a shorthand encoding of a 256-bit binary number (not printed here). The hash of the very similar word ‘platform’ appears directly beneath. Originally introduced as a US National Institute of Standards information processing standard for digital signing of documents (NIST Citation1995), the SHA algorithms operate on data in 512-bit blocks, so short messages like ‘platforms’ will be padded with extra characters to make them up to minimum block side needed to produce a SHA256-length hash. The SHA256 hash of the whole of this article would still be the same length as the hash of ‘platform’, as would the SHA256 hash of the images showing on user a's Explore Page.
The fact that the first two hashes are much longer than the data they approximate (‘platform(s)’) points to an important formal property of hashes. Hashes are usually approximations of fixed size to fields of activity on many scales. Their fixed size means they can be built into concatenated chains of reference as they are in the blockchains of Bitcoin or Ethereum, where hashes are the hashing of previous hashes.
Mobility of numberings
Unlike probabilities or other measuring, counting and ranking approximations, hashes have no indirect connection to quantity, geometry, measure or similarity. As approximations, their proximities and distance are concerned with identity and difference rather than with degrees of similarity. Hashes support, more than other numberings, divergence rather than convergence, as seen in the difference between the hashes for ‘platform’ and ‘platforms’. Closely related words generate divergent SHA-hashes. Thus, in many settings, hashes work as relatively unique indexes. That is, they are numberings that ideally correspond to one unique location. They are difficult to model or predict.
As Whitehead's account of approximation suggests, mappings of any number by some set of numbers, for instance, all input data by a 256-digit SHA256 hash, only work within some standard of approximation. The desirable cryptographic hash properties of constant size and unique mapping of any input data are only reliable within Whitehead's ‘standard of approximation k’, but this standard depends on the state of numbering in the ensemble. The hashing algorithm SHA-1 was shown to sometimes generate non-unique hashes (‘a hash collision’), a problem that in 2017 occasioned minor shock waves in the worlds of platform security and administration. Researchers in Amsterdam and Google, in a crypto-attack they called ‘Shattered’, developed a way to generate different PDF files with identical SHA-1 hashes (Stevens Citation2017 [Citation2022]). If different files can have the same hash, then the mapping of any by some is no longer unique. Cryptographic verification of identities, commodities, assets and transactions and software updates breaks down. The fact that the researchers relied on the compute capacities of the Google platform, including heavy use of its many GPU (Graphics Processing Unit) processors, suggests the intense interest of large platforms in the capacity of hashes to uniquely differentiate data. It also points to the ongoing pertinence of the ‘standard of approximation’ in any numbering. The rapid replacement of SHA-1 by SHA256 and other hashing algorithms confirmed the sensitivity of platforms to their hash-based operation.
Compared to the numberings of finance, statistics, calculus, geometry, or algebra that have shaped the engineering and commerce associated with platforms, hashes have a curiously flat, inert presence, directly linked to legal and financial institutions and to the technical operations of ensembles. There is no obstacle to observing these numberings and the functions that produce them in for instance every online payment. The terse terminal commands indicate their availability. Like the embedding numberings, hashing is a form of compression. As shown above, SHA256 shapes any amount of input data as a 256-digit hash. Unlike many other numberings that approximate, cryptographic hashes diverge from each other. They do not cluster or group around norms such as average. Their distribution is uniformly random. In cryptographic and blockchain uses of hashing, this distribution or dispersed set of hashes is crucial since it protects the interactions of verification, validation and authentication on which communication, security, file systems, version control and de-centralized currencies depend. They are readily available and constantly generated as part of the mundane operation of fields of devices present in an ensemble.
Platforms are hashed ensembles?
In themselves, hashes are hardly the locus of direct affective investment or identification. Unlike images or utterances, their effects are distributive. Like the statistical fluxes of Higgs bosons giving rise to mass, hashing. When they function as indexes, for instance in IGDiskCache or PDQ, or in database tables and commonly used data structures such as Python dictionaries or Java HashMaps, to support storing and retrieving data, they reinforce habits and routinized actions. The storage uses of hash are rampant in code operations, perhaps ever more so today, since design of hash functions yields measurable reductions in the time it will take to find content in increasingly distributed data stores.
The diversification of hashing of other data forms and operations signals a mode of coordination of technical ensembles that has both made personalization possible and significantly overflows it. It might be that the platforms that attract so much interest for the expansive, predatory, colonizing or extractive operations, are an outgrowth of hashing. Numberings that localize through indexes or that verify through unique keys configure many facets of platforms in the shifting manifolds of ensembles. If personalizations based on embeddings proliferate versions of ensembles bounded by measures of similarity exemplified in Ig2Vec, the versioning of ensembles based on hashing creates interstitial relations characterized by irreversibility, uniqueness, localization and verification.
To better understand how hashing support the versioning of ensembles as platforms, we might consider the hash-based platform GitHub. GitHub presents itself as a tool for making software products – ‘millions of developers and companies build, ship and maintain their software on GitHub, the largest and most advanced development platform in the world’ (GitHub Citation2022). What actually happens there is considerably more complicated than the productivist ‘build-ship-maintain’ mantra suggests. Since 2007, GitHub as a platform has been rocked by controversies of sexism, racism, exploitation and political suppression, yet it is awash with software developers, researchers, scientists, engineers, writers and others, coordinating their devices and their workflows according to plural libertarian, anarchist, communitarian, corporate, civil and incivil sociotechnical imaginaries.
For platforms driven by constant mutation (Chun Citation2016), it is as if Github makes possible the osmotic exchanges and cross-hybridizations, the inter-process communications and connections that the delimit platforms against the fluxing relationality of ensembles. Itself a platform, Github is a place where ensembles are rendered as platforms through a constant process of exchange, imitation, combination and divergence. Github coordinates, for instance, the coding development of crucial technical ensembles such as the operating systems Android, Windows and Linux, all major programming languages, a panoply of experiments in prediction and the protocols for Web 3.0 de-centralized coordination. The millions of repositories on Github encrust, infiltrate and support the seemingly smooth, hardened laminations of platforms. The tens of millions of code repositories on GitHub present the edges of platform as mutable, provisional and mobile rather than fixed.
The hashing of code indexes this individuation of technical ensembles. It is rare to find a platform named after a hashing function, but Github relies on the file system hashes generated by the underlying git software, a distributed code version control system based on the idea that every change in a set of files can be indexed by hashing the files using the SHA-1 hash function (Rodrigo Citation2021; Tomakyo Citation2008; Hamano Citation2022). Since its launch in 2005, around the same time as internet platforms were enclosing significant amount of online activity, git rapidly propagated throughout software development. git makes it possible to relate to manifolds of technical elements on scales ranging from individual to collectives, and to maintain many versions of the same thing without much coordinating effort. Hash functions have become the basic mode of address problems of change, integration and coordination in increasingly distributed fields of devices and developers. SHA hash functions digest every change in code or any other files stored in a git repository as ‘commit hashes’. The git hash of the state of a repository, which may contain thousands of files worked on hundreds of developers, supports the creation of chains of revisions, branches, mergers, clones, and other forms of change and variation that constitute a functional temporality of ensembles.
The hashing of code is the core of the platform-critical practices of version control. It also weaves the connective continuum of contemporary platform ensembles in their mutability. The propensity of contemporary platforms to rearrange elements in a kaleidoscopic array of variations and modifications, or enclose sub-ensembles within their oscillating individuation relies on code versions and hence on hashing. Even on a single platform, experiment, development and maintenance takes place constantly. It depends on the addressability of changes to the code. The nascent logic of devops, or development-operations, and ‘continuous integration’, a mode of continuous making endemic to platform economies, is an outgrowth of the hashing of code. Software repository platforms such as GitHub, BitBucket and GitLab enfold ever-extending development ‘pipelines’ running from coding into platform/infrastructure-as-a-service products such as Amazon's EC2 or Google Compute.
Distributed coordinated variation through hashing also plays out in the proliferation of SHA-based blockchains or in the generalized ‘going crypto’ of finance, art markets, darknets, institutions and some nation-states such as El Salvador. These alternative realizations of hashing have complex connections with the platform ensembles and discourse, sometimes, as in Web3.0, presenting a de-personalized post-platform vision of technical ensembles. Hashing thus has an ambivalent relation to personalization.
Conclusion
Approximating is a way of moving closer to something: ‘a set of numbers approximates to a number a within a standard k, when the numerical difference between a and every number of the set is less than k’. Whitehead's account of approximation was intended to open a way of linking a series of seemingly unrelated developments in modern mathematics, including sets, topology, series, functions and calculus. The idea of approximation of any by some within a shifting set of limits can also address the seemingly unrelated developments of personalized content on social media platforms and the design of file systems or encrypted communications. Both, as numerate practices, are forms of approximation. From the perspective of approximation, personalization and its flipside, platform coordination, are numberings of any by some within some framing of difference by limits.
Hashes and probabilities sometimes entwine with each other, mingling in the stream of operations unfolding in platforms. The #platform shoe and train images reaching user a's Instagram app percolate through technical ensembles we call platforms along densely woven channels. They surface on the Explore Page as a configured microstate of the ensemble, a microstate configured by calculations of the most likely state of the ensemble given the probability distribution of microstates similar to that of user a. At the same time, their movement on the way to user a's Explore Page passes through hashing functions that test whether they are similar to images already in the data centre image collections, whether have they been downloaded by the app before, and whether the connection between an individual user app and the content-provider platform is secure, etc. The streaming of personalized experience-approximations is also the diffuse numbering epitomized by the hashes tumbling out of the SHA-hardware accelerated Intel chips, the Nvidia GPUs of the Bitcoin miners churning with hashing functions, the hashes generated for every blockchain transaction, artists minting an NFT, each coin on the Ethereum blockchain, each state of play of a git repository, or the hashed location of any of the trillions of file in key-value datastores.
Approximations of any by some play out within different limits in embedding and hashing. The recommendation systems and hash-based coordination vary widely in their configuration of experience of grouping. Social media platforms centred on personalization focus on generating probability distributions for microstates of the ensemble. The embeddings renders ensembles as platforms by gathering, calculating and ranking of microstates under a spanning set of probabilities. Probability distributions determine the limits. By contrast, in hashing, limits defined by the capacity of hashes to uniquely index a given state of affairs, are the basis of the coordination, traceability and verification on which many aspects of platform experience, including individual use, depend.
From this dual numbering perspective on contemporary technical ensembles, what can we say about the significance of the personalization typical of social media platforms such as the Instagram Explore Page? Much social research and public controversy shows that personalization has enclosed and internally colonized tracts of experience that once lay on the edges of technical ensembles. But understood as approximation of any by some, personalization presents the problem of how the shifting phases of ensembles stabilize as platforms. The probability approximation gathers so many hitherto protected microstates of individual habit into its embeddings. Most platforms show little interest in the impersonal potentials of these numberings or how these forms of statistical approximation might activate new forms of some-any grouping. The effectiveness of platforms as a stabilization, as measured by billions of user accounts, is tinged with a structure of felt-ambivalence associated with other potentials of the ensembles.
It is ironic that platform-personalization has intensified just as much social theory has turned towards relationality, knowing-in-place, more-than-human kinship and human-nonhuman co-becomings, towards in short accounts of more distributed groupings, constitutively open fields of experience and indeed accounts of experience and sociality in which personhood diversifies, sometimes to the point of disintegration. The challenge of tracing these impersonalizing trajectories in the context of platforms derives from the persistence of the predicates of individualization in platform discourse.
Is it necessary to approach contemporary technical ensembles via numberings? What can be learned from approximating numberings that could not be elicited from what people say or do on social media platforms? It is not only that probability embeddings and hash numberings have become endemic operators, the ‘primitives’ to use a computer science term for smallest units of processing, in contemporary technical ensembles. It is also that it is difficult, analytically and experientially, to go against the tide of subjectifying personalization associated with platforms. The analysis of approximations perhaps helps suspend the subjectifying processes at work there and leaves some space for what overflows them to appear.
The limits that define relations between any and some can proliferate in different directions. It is no accident that hashing becomes an ethico-economic imperative as ensembles oscillate between tightly-delimited, hyper-converged platforms intent on individual user-engagement and the somewhat untethered commutative groups, experimental organizing and value-hybridizing seen in emergent forms of coordination across cultural production, new institutional forms and technical invention. What platforms cannot fully contain of the coordinating potentials of their own technical substrates comes to fore as the localizing and validating relations of hashing ensembles. The ensemble or collection of microstates mapped by a probability distribution could spawn versions of ensembles configured around quite different coordinations of elements and experiences.
Disclosure statement
No potential conflict of interest was reported by the author(s).
Additional information
Notes on contributors
Adrian Mackenzie
Adrian Mackenzie (Professor in the School of Sociology, ANU) researches how people work and live with media, devices, knowledges and infrastructures. He often focuses on software and platforms. He has a keen interest in the methodological challenges of media and science infrastructures for sociology. He is currently researching models, apps and sensors for extreme events.
Notes
1 In the context of machine learning, the Boltzmann probability distribution is known as ‘softmax.’ It is frequently used as the ‘activation’ or output layer in neural networks such as Ig2Vec, the final set of calculations that shape preceding sets of numbers into probabilities summing to one.
References
- Bucher, Taina. 2017. The algorithmic imaginary: Exploring the ordinary affects of Facebook algorithms. Information, Communication & Society 20, no. 1: 30–44. doi:10.1080/1369118X.2016.1154086.
- Chun, Wendy Hui Kyong. 2016. Updating to remain the same: Habitual new media. Cambridge, MA: The MIT Press. https://library.anu.edu.au/record=b7409443
- Couldry, Nick, and Andreas Hepp. 2018. The mediated construction of reality. London: John Wiley & Sons. https://ebookcentral-proquest-com.virtual.anu.edu.au/lib/anu/reader.action?docID=4769058&ppg=4.
- Couldry, Nick, and Ulises A. Mejias. 2019. The costs of connection: How data is colonizing human life and appropriating it for capitalism, 1st edition. Stanford: Stanford University Press. https://ebookcentral-proquest-com.virtual.anu.edu.au/lib/anu/detail.action?docID=5789180
- Engineering, Instagram. 2016. Building an open source, carefree android disk cache. Medium. June 24. https://instagram-engineering.com/building-an-open-source-carefree-android-disk-cache-af57aa9b7c7.
- Facebook. [2016] 2021. IgDiskCache. Meta Archive. https://github.com/facebookarchive/ig-disk-cache.
- Gao, Xiang. 2022. The mathematics of the ensemble theory. Results in Physics 34, no. March: 1–6. https://www.sciencedirect.com/science/article/pii/S2211379722000390.
- Gibbs. 1902. Elementary principles in statistical mechanics: Developed with especial reference to the … C. Scribner’s sons; [etc ., etc.]. http://archive.org/details/elementaryprinc00gibbgoog.
- GitHub. 2022. GitHub: Where the world builds software. GitHub. https://github.com/.
- Hamano, John. 2022. Git(1). https://mirrors.edge.kernel.org/pub/software/scm/git/docs/.
- Hashemi, Mazdak. 2017. The infrastructure behind Twitter: Scale. January 19. https://blog.twitter.com/engineering/en_us/topics/infrastructure/2017/the-infrastructure-behind-twitter-scale.html.
- Kerl, John. [2015] 2022. Facebook ThreatExchange. Meta. https://github.com/facebook/ThreatExchange/blob/31914a51820c73c8a0daffe62ccca29a6e3d359e/hashing/hashing.pdf.
- Langlois, Ganaele, and Greg Elmer. 2019. Impersonal subjectivation from platforms to infrastructures. Media, Culture & Society 41, no. 2: 236–51. doi:10.1177/0163443718818374.
- Latour, Bruno, Pablo Jensen, Tommaso Venturini, Sébastian Grauwin, and Dominique Boullier. 2012. ‘The whole is always smaller than its parts’–A digital test of G Abriel T Ardes’ Monads. The British Journal of Sociology 63, no. 4: 590–615.
- Lovink, Geert. 2019. Sad by design: On platform nihilism. London: Pluto Press. https://www.jstor.org/stable/j.ctvg8p6dv.
- Mackenzie, Adrian. 2021. Experience distributed in the biodiversity science-base. Science & Technology Studies. 35, no. 3. https://sciencetechnologystudies.journal.fi/article/view/100417
- Manovich, Lev. 2020. The aesthetic society: Or how I edit my Instagram 1. In Data publics: Public plurality in an era of data determinacy, eds. P. Mörtenböck, and H. Mooshammer. London: Routledge.
- Medvedev, Ivan. 2019. Powered by AI: Instagram’s explore recommender system. Medium. November 26. https://instagram-engineering.com/powered-by-ai-instagrams-explore-recommender-system-7ca901d2a882.
- Mikolov, Tomas, Kai Chen, Greg Corrado, and Jeffrey Dean. 2013. Efficient estimation of word representations in vector space. http://arxiv.org/abs/1301.3781.
- Milmo, Dan, and Dan Milmo Global technology editor. 2021. Facebook revelations: What is in cache of internal documents? The Guardian: Technology, October 25. https://www.theguardian.com/technology/2021/oct/25/facebook-revelations-from-misinformation-to-mental-health.
- NIST, Secure Hash. 1995. FIPS Pub 180-1. National Institute of Standards and Technology 17: 15.
- Pierce, David. 2017. How Instagram explore became the realest place on the web. Wired. May 23. https://www.wired.com/2017/05/instagram-explore-became-realest-place-web/.
- Rodrigo, M. S. 2021. The 1st commit of git/git no longer belongs to Linus Torvalds. Quine. November 12. https://medium.com/quine/the-1st-commit-of-git-git-no-longer-belongs-to-linus-torvalds-ea1df6f8f025.
- Stevens, Marc. 2017. SHAttered. https://shattered.io/.
- Stevens, Marc. [2017] 2022. Sha1collisiondetection. https://github.com/cr-marcstevens/sha1collisiondetection.
- Tomakyo, Ryan. 2008. The thing about git. Ryan Tomayko. April 8. https://tomayko.com/blog/2008/the-thing-about-git.
- Whitehead, Alfred North. 2017. An introduction to mathematics. New York: Dover Publications. https://books.google.com?id=_F_KDgAAQBAJ.