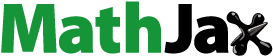
Abstract
We define weighted geometric mean method of convergence for sequences in by using multiplicative calculus and obtain necessary and sufficient conditions under which convergence of sequences in
follows from convergence of their weighted geometric means. We also obtain multiplicative analogues of Schmidt type slow oscillation condition and Landau type two-sided condition for the convergence in particular. Besides, we introduce the concepts of
convergence,
convergence,
convergence,
convergence for sequences of intuitionistic fuzzy numbers (IFNs) and apply the aforementioned conditions to achieve convergence in intuitionistic fuzzy number space. Examples of sequences are also given to illustrate the proposed methods of convergence.
1. Introduction
Multiplicative calculus [Citation1,Citation2] is alternative to classical calculus and uses ratios instead of differences in order to measure deviations and compare numbers. The operations multiplication and division are crucial in multiplicative calculus and many concepts such as differentiation and integration are based on these operations. Being the main concept of this paper, the convergence of sequences of positive real numbers is also defined via these operations in multiplicative calculus. In this paper, we use multiplicative calculus to deal with the convergence of sequences of real numbers through weighted geometric means. By the way, weighted geometric means are encountered in many topics of mathematics one of which is sequences of IFNs. In particular, see intuitionistic fuzzy aggregation operators [Citation3,Citation4].
There are many examples of sequences in real number space and in intuitionistic fuzzy number space where the convergence can not be achieved via existing types of convergence. Besides, in some cases, the limit may not be unique or may not be the intended value even if the convergence is achieved via those types of convergence. To recover the convergence of such sequences, we need new types-methods of convergence. The main aim of this paper is to introduce the weighted geometric mean method of convergence for sequences in by using multiplicative calculus and prove related convergence theorems in
with application to intuitionistic fuzzy number space. Recently, Çanak et al. [Citation5] used multiplicative calculus and defined a geometric mean method to assign a limit value to sequences which fail to converge in
. Besides, they obtained conditions in the multiplicative sense under which convergence in
follows from the convergence of geometric means and gave multiplicative analogues of Schmidt type slow oscillation condition and Landau type two-sided condition as corollaries. In Example 3.2, the geometric mean method fails to assign a limit. Furthermore, in Example 3.3, the geometric mean method may not assign the intended limit value even if it achieves a limit value in
. In such cases, we may use weighted geometric means instead of geometric means. Hence, by using multiplicative calculus, in Section 3 we define the weighted geometric mean method for sequences in
and obtain necessary and sufficient conditions under which convergence in
follows from convergence of weighted geometric means. In Section 4, we define two types of convergence and weighted mean limitation methods for sequences of IFNs to handle sequences such in Examples 4.3, 4.13 and 4.21 and apply the conditions of Section 3 to sequences of IFNs in order to recover the convergence.
2. Definitions and notations
Let . Then, the absolute value of u in the multiplicative sense is [Citation6]
Let
. Then, the properties below are valid. Abbas et al. [Citation7]
if and only if
.
Multiplicative distance is defined by Bashirov et al. [Citation6]
and satisfies the following properties:
for all
,
if and only if u = v,
for all
,
for all
.
A sequence in
is said to be *convergent to
if for all
there exists
such that
whenever
, and denoted by
. Sequence
is said to be *bounded if there exists B>1 such that
for all
. For further concepts such as multiplicative derivative, multiplicative differential equations and the Newtonian counterparts, see [Citation1,Citation2,Citation6–11].
Remark 2.1
We note that a sequence in
*converges to
if and only if
converges to a with respect to the usual absolute value metric in
. That is, *convergence and convergence are equivalent in
. On the other hand, the same is not valid for *boundedness and boundedness in
which can be seen by the sequence
that is bounded in
but is not *bounded in
. See also [Citation6, Section 4.3].
Let be a sequence of nonnegative numbers such that
(1)
(1) and
for a positive number λ.
is the set of all sequences
satisfying [Citation12]
Definition 2.2
[Citation5]
A sequence of positive real numbers is said to be
slowly oscillating if
or equivalently
Now, we give some definitions concerning intuitionistic fuzzy sets which are necessary for Section 4.
Let X be a non-empty set. Then, an Atanassov's intuitionistic fuzzy set(A-IFS) [Citation13] has the following form: where
is called membership function and
is called non-membership function. For any
,
. In special case
, A-IFS degenerates to fuzzy set [Citation14]. For convenience, Xu and Yager [Citation3] called
an IFN which satisfies
,
, and
.
Definition 2.3
[Citation3,Citation4]
Let and
be two IFNs,
and
the scores of
and
, respectively,
and
the accuracy degrees of the
and
, respectively. Then,
If
, then
is smaller than
, denoted by
.
If
, then
If
, then
equal to
, i.e.
,
denoted by
;
If
, then
is smaller than
, denoted by
;
If
, then
is larger than
, denoted by
.
Definition 2.4
[Citation15]
Let and
be two IFNs. Then
If
and
, then
If
and
, then
If
and
, then
Definition 2.5
[Citation3,Citation4,Citation16]
Let ,
be two IFNs and
. Then,
where
and
The addition region of IFN ξ is defined by . Let
be a sequence of IFNs where
. We call
an addition sequence of ξ if there exists
such that
for all
[Citation16].
3. Main results
In this section, we first define the weighted geometric mean method of convergence in by using multiplicative calculus in order to achieve the convergence of sequences such as Examples 3.2 and 3.3. Then, we give various conditions under which convergence of sequences in
follows from the convergence of their weighted geometric means.
We note that denotes a sequence in
throughout this section. Let
be a sequence of nonnegative real numbers satisfying (Equation1
(1)
(1) ). The weighted geometric means
of sequence
is defined by
(2)
(2) We say that
*converges to
by weighted geometric mean method, briefly:
*convergent to a if
. When
for all
,
*convergence reduces to the geometric mean method defined in [Citation5]. For weighted arithmetic means, see [Citation12,Citation17,Citation18].
Theorem 3.1
If sequence is *convergent to
, then
is
*convergent to a.
Proof.
Let be *convergent to
. Then, for given
there exist
and B>1 such that
for
and
for
. Besides, there is
such that
in view of the fact
. Hence, we have
for
which completes the proof.
The converse of Theorem 3.1 is not true in general. That is, *convergence does not imply *convergence in
which can be seen by the following examples.
Example 3.2
Sequence defined by
is not *convergent and not
*convergent introduced by Çanak et al. [Citation5], but it is
*convergent to 1 for
.
Example 3.3
Sequence defined by
is not *convergent, but it is
*convergent to 1 for
and
*convergent to
for
With an appropriate choice of the weights
, the other intended values in
can also be assigned as a limit value to sequence
by the help of weighted geometric mean method.
In this section, we give some conditions for *convergence to imply *convergence in
. We need the following lemma to prove the main results of this section.
Lemma 3.4
(i) | Let | ||||
(ii) | Let |
Proof.
(i) By the fact that
we have
which implies (Equation3
(3)
(3) ).
(ii) The proof of (Equation4(4)
(4) ) can be done similarly, hence it is omitted.
We now give the necessary and sufficient conditions under which *convergence implies *convergence in
.
Theorem 3.5
Let . If
is
*convergent to
, then
is *convergent to a if and only if one of the following two conditions hold:
(5)
(5) or
(6)
(6)
Proof.
Necessity. Suppose . Let
. Then, from Theorem 3.1 we have
which implies
(7)
(7) and
(8)
(8) in view of
. Also, from Equation (Equation3
(3)
(3) ) we have
(9)
(9) Then, (Equation5
(5)
(5) ) is satisfied by virtue of (Equation7
(7)
(7) ), (Equation8
(8)
(8) ) and (Equation9
(9)
(9) ).
Let . Since
, we have
(10)
(10) in view of
. Also, from Equation (Equation4
(4)
(4) ) we have
(11)
(11) Then, (Equation6
(6)
(6) ) is satisfied by virtue of (Equation7
(7)
(7) ), (Equation10
(10)
(10) ) and (Equation11
(11)
(11) ).
Sufficiency. Suppose is
*convergent to a and (Equation5
(5)
(5) ) is satisfied. Then from (Equation5
(5)
(5) ) there exists
such that
(12)
(12) where
. Also by equality (Equation3
(3)
(3) ) we have
(13)
(13) Hence, from (Equation8
(8)
(8) ), (Equation12
(12)
(12) ) and (Equation13
(13)
(13) ) we get
which implies
in view of the fact that
.
Suppose is
*convergent to a and (Equation6
(6)
(6) ) is satisfied. Then, from (Equation6
(6)
(6) ) there exists
such that
(14)
(14) Also by equality (Equation4
(4)
(4) ) we have
(15)
(15) Hence, from (Equation10
(10)
(10) ), (Equation14
(14)
(14) ) and (Equation15
(15)
(15) ) we get
which implies
in view of the fact that
.
In view of Theorem 3.5, we give the following corollary as a result of the fact that slowly oscillation implies (Equation5
(5)
(5) ) and (Equation6
(6)
(6) ).
Corollary 3.6
If is
*convergent to
and
slowly oscillating, then it is *convergent to a.
Lemma 3.7
If is *bounded then
is
slowly oscillating, where
Proof.
Let be *bounded. Then, there is H>1 such that
for every
. Let
be given. Then, for
and
we get
which implies that
is
slowly oscillating.
In view of Corollary 3.6 and Lemma 3.7 we give the following results.
Corollary 3.8
Let . If
is
*convergent to
and
is *bounded, then
is *convergent to a.
Corollary 3.9
Let . If
is
*convergent to
and
, then
is *convergent to a.
Remark 3.10
In view of Remark 2.1, “*convergent” can be replaced by “convergent” in the theorems and corollaries of this section.
4. Convergence of sequences of IFNs
Authors have done many studies concerning intuitionistic fuzzy sets [Citation16,Citation19–30]. Among them, Lei and Xu [Citation16] are the first to define convergence of sequences of IFNs by using subtraction operation.
Definition 4.1
[Citation16]
Let be an addition sequence of IFN ξ. If
there is a positive integer
such that
for
, then ξ is the addition limit of
.
In this definition, Lei and Xu [Citation16] implemented the assumption that is an addition sequence of ξ in order to guarantee
to be an IFN, by which we mean
is an IFN for
. Besides, they proved that this convergence, under the same assumption, satisfies Theorems 4.5 and 4.7 which are very useful in the calculation of limits of sequences of IFNs. However, there are many sequences of IFNs which are not addition sequences of the limit points as in Example 4.3 and hence Definition 4.1 is not applicable to such sequences. Zhang and Xu [Citation26] removed the assumption on the sequence and defined following convergence by using the addition operation and the order relation given in Definition 2.3.
Definition 4.2
[Citation26]
Let be a sequence of IFNs and ξ be an IFN. Sequence
is said to be convergent to ξ if for any IFN
, there exists
such that
hold for
.
In Definition 4.2, there is no assumption on the sequence but the limit is not unique. Besides, this type of convergence does not satisfy Theorems 4.5 and 4.7 which are useful theorems. These can be seen in the following example.
Example 4.3
Consider the sequence of IFNs defined by .
Case 1 (convergence by Definition 4.1). The only candidate for the limit value is , but
is not addition sequence of
since
is not an IFN for any
. Hence, Definition 4.1 is not applicable.
Case 2 (convergence by Definition 4.2).
converges to IFNs
and
in view of the facts that
for any IFN
and
. In fact,
has infinite number of limits since
converges to any IFN ξ such that
Hence, the limit is not unique. On the other hand, Theorems 4.5 and 4.7 are not satisfied since
but
and
.
In this section, following [Citation16,Citation26], we first define the concepts of convergence and
convergence for sequences of IFNs by means of the partial order given in Definition 2.4. Then, we apply the results of Section 3 in order to achieve convergence in intuitionistic fuzzy number space.
Definition 4.4
Let be a sequence of IFNs and ξ be an IFN. Sequence
is said to be
convergent to ξ if for any IFN
, there exists
such that
hold for
.
Theorem 4.5
A sequence of IFNs
converges to an IFN
if and only if
and
.
Proof.
Necessity. Suppose
converges to ξ. Let
be given. Then, for
we have
and
Since
is arbitrary, this implies
and
.
Sufficiency. Let and
. For given
followings hold:
There exists
such that
and
for
and these imply
and
, respectively. Hence, we have
for
.
By the assumption
we have
and
and so there exists
such that
and
for
. On the other hand, there exists
such that
and
for
. These imply
and
for
. Hence, for
we have
and
which implies
.
From (i) and (ii), we conclude that
for
which completes the proof.
Now we give convergence for sequences of IFNs.
Definition 4.6
Let be a sequence of IFNs and ξ be an IFN. Sequence
is said to be
convergent to ξ if for any IFN
, there exists
such that
hold for
.
Theorem 4.7
A sequence of IFNs
converges to an IFN
if and only if
and
.
Proof.
Necessity. Suppose
converges to ξ. Let
be given. Then, for
we have
and
Since
is arbitrary, this implies
and
.
Sufficiency. The proof can be done similar to the sufficiency part of the proof of Theorem 16 by changing the roles of μ and ν, and replacing the operation ⊕ by the operation ⊗.
Remark 4.8
If the limit exists by convergence, then it is unique by Theorem 4.5. Similar case is also valid for
convergence by Theorem 4.7. As an example,
convergence and
convergence work in Example 4.3 with unique limit
.
Remark 4.9
We note that if , then
convergence and
convergence are equivalent in intuitionistic fuzzy number space.
4.1. Convergence via weighted arithmetic means
In some cases of sequences of IFNs as in Example 4.13, convergence may fail in intuitionistic fuzzy number space. In such cases we may use weighted arithmetic means to grasp a limit. In this subsection, we assume
for any IFN β.
Definition 4.10
Let be a sequence of IFNs and sequence
of nonnegative real numbers satisfying (Equation1
(1)
(1) ). Then, sequence of weighted arithmetic means of
is defined by
Sequence
is said to be
convergent by the weighted arithmetic mean method, or
convergent, to IFN ξ if
converges to ξ.
We note that is, in fact, an infinite sequence of intuitionistic fuzzy weighted averaging operators (IFWA) defined by Xu [Citation4].
Theorem 4.11
A sequence of IFNs is
convergent to an IFN ξ if and only if
and
, where
is the weighted geometric mean operator in (Equation2
(2)
(2) ).
Proof.
Let be a sequence of IFNs. We have
By Theorem 4.5, sequence
converges to ξ if and only if
and
. Hence, the proof is completed.
Theorem 4.12
If sequence of IFNs is
convergent to an IFN ξ, then it is
convergent to ξ.
Proof.
Let
converge to ξ. From Theorem 4.5 we have
and
. Then, from Theorem 3.1 we have
and
which implies
is
convergent to ξ in view of Theorem 4.11.
convergence of a sequence of IFNs does not imply
convergence by the following example.
Example 4.13
Consider sequence of IFNs defined by
is not
convergent by Theorem 4.5. But, it is
convergent with
to IFN
by the facts that
and
in view of Theorem 4.11.
In view of Theorems 3.5, 4.5, 4.11 and Corollaries 3.6, 3.8, 3.9; we give the conditions under which convergence implies
convergence in intuitionistic fuzzy number space.
Theorem 4.14
Let . If sequence
of IFNs is
convergent to an IFN ξ, then
is
convergent to ξ if and only if one of the following two conditions hold:
or
Theorem 4.15
Let . If sequence
of IFNs is
convergent to an IFN ξ and sequences
,
of real numbers are
slowly oscillating, then
is
convergent to ξ.
Theorem 4.16
Let . If sequence
of IFNs is
convergent to an IFN ξ and sequences
,
of real numbers are *bounded, then
is
convergent to ξ.
Theorem 4.17
Let . If sequence
of IFNs is
convergent to an IFN ξ and
,
, then
is
convergent to ξ.
4.2. Convergence via weighted geometric means
In some cases of sequences of IFNs as in Example 4.21, convergence may fail in intuitionistic fuzzy number space. In such cases, we may use weighted geometric means to grasp a limit. In this subsection, we assume
for any IFN β.
Definition 4.18
Let be a sequence of IFNs and sequence
of nonnegative real numbers satisfying (Equation1
(1)
(1) ). Then, sequence of weighted geometric means of
is defined by
Sequence
is said to be
convergent by the weighted geometric mean method, shortly
convergent, to IFN ξ if
converges to ξ.
We note that is, in fact, an infinite sequence of intuitionistic fuzzy weighted geometric operators (IFWG) defined by Xu and Yager [Citation3].
Theorem 4.19
A sequence of IFNs is
convergent to an IFN ξ if and only if
and
, where
is the weighted geometric mean operator in (Equation2
(2)
(2) ).
Proof.
Let be a sequence of IFNs. We have
By Theorem 4.7, sequence
converges to ξ if and only if
and
. Hence, the proof is completed.
Theorem 4.20
If sequence of IFNs is
convergent to an IFN ξ, then it is
convergent to ξ.
Proof.
Let
converge to ξ. From Theorem 4.7 we have
and
. Then, from Theorem 3.1 we have
and
which implies
is
convergent to ξ in view of Theorem 4.19.
convergence of a sequence of IFNs does not imply
convergence by the following example.
Example 4.21
Consider sequence of IFNs defined by
is not
convergent by Theorem 4.7. But, it is
convergent to IFN
for
in view of the facts that
and
by virtue of Theorem 4.19.
In view of Theorems 3.5, 4.7, 4.19 and Corollaries 3.6, 3.8, 3.9; we give the conditions under which convergence implies
convergence in intuitionistic fuzzy number space.
Theorem 4.22
Let . If sequence
of IFNs is
convergent to an IFN ξ, then
is
convergent to ξ if and only if one of the following two conditions hold:
or
Theorem 4.23
Let . If sequence
of IFNs is
convergent to an IFN ξ and sequences
,
of real numbers are
slowly oscillating, then
is
convergent to ξ.
Theorem 4.24
Let . If sequence
of IFNs is
convergent to an IFN ξ and sequences
,
of real numbers are *bounded, then
is
convergent to ξ.
Theorem 4.25
Let . If sequence
of IFNs is
convergent to an IFN ξ and
,
, then
is
convergent to ξ.
Remark 4.26
The results of Subsections 4.1–4.2 can be extended to other convergence types of sequences of IFNs, provided that chosen type of convergence satisfies Theorem 4.5 or Theorem 4.7.
5. Conclusion
In this paper, we have used multiplicative calculus and introduced the weighted mean method of convergence in . We have obtained various conditions under which convergence of sequences of positive real numbers follows from the convergence of their weighted geometric means. Besides, we have defined some new types of convergence for sequences of IFNs and applied the obtained conditions to occurring weighted geometric averages of membership and non-membership functions in order to grasp convergence in intuitionistic fuzzy number space. Examples of sequences such that our methods of convergence work but the methods in the literature do not work have been also given to illustrate the advantage of proposed methods. In particular,
sequence of real numbers in Example 3.2: ordinary convergence and the geometric mean method [Citation5] do not work but
*convergence works,
sequence of real numbers in Example 3.3:
*convergence is able to assign an intended limit value with appropriate choice of the weights
,
sequence of IFNs in Example 4.3: Definition 4.1 does not work, Definition 4.2 works with an infinite number of limits but
convergence and
convergence work with a unique limit,
sequences of IFNs in Examples 4.13 and 4.21: the methods in the literature do not work but
convergence and
convergence work.
The results of this paper may help researchers to handle sequences of positive real numbers and sequences of IFNs. The results may also help when dealing with sequences of weighted geometric averages occurring in many fields of science as in sequences of IFNs mentioned in Section 4. For future work, the results may be extended to different types of fuzzy sets such as Linear Diophantine fuzzy sets [Citation31], Pythagorean fuzzy sets [Citation32–34], etc.
Acknowledgments
The author would like to thank Assoc. Prof. Özer Talo for his valuable comments and suggestions which helped to improve the quality of the manuscript.
Disclosure statement
No potential conflict of interest was reported by the author(s).
Correction Statement
This article has been republished with minor changes. These changes do not impact the academic content of the article.
References
- Grossman M, Katz R. Non-Newtonian calculus. Pigeon Cove (MA): Lee Press; 1972.
- Stanley D. A multiplicative calculus. Primus. 1999;9(4):310–326.
- Xu ZS, Yager RR. Some geometric aggregation operators based on intuitionistic fuzzy sets. Int J Gen Syst. 2006;35:417–433.
- Xu ZS. Intuitionistic fuzzy aggregation operations. IEEE Trans Fuzzy Syst. 2007;15:1179–1187.
- Çanak İ. Necessary and sufficient conditions for geometric means of sequences in multiplicative calculus. Miskolc Math Notes. 2016;17(2):791–800.
- Bashirov AE, Kurpınar EM, Özyapıcı A. Multiplicative calculus and its applications. J Math Anal Appl. 2008;337(1):36–48.
- Abbas M, Ali B, Suleiman YI. Common fixed points of locally contractive mappings in multiplicative metric spaces with application. Int J Math Sci. 2015;2015:Article ID 218683.
- Tor AH. An introduction to non-smooth convex analysis via multiplicative derivative. J Taibah Univ Sci. 2019;13(1):351–359.
- Seadawy AR, Lu D, Yue C. Travelling wave solutions of the generalized nonlinear fifth-order KdV water wave equations and its stability. J Taibah Univ Sci. 2017;11(4):623–633.
- Seadawy AR, Iqbal M, Lu D. Nonlinear wave solutions of the Kudryashov–Sinelshchikov dynamical equation in mixtures liquid-gas bubbles under the consideration of heat transfer and viscosity. J Taibah Univ Sci. 2019;13(1):1060–1072.
- Özkan YS, Seadawy AR, Yaşar E. Multi-wave, breather and interaction solutions to (3+1) dimensional Vakhnenko–Parkes equation arising at propagation of high-frequency waves in a relaxing medium. J Taibah Univ Sci. 2021;15(1):666–678.
- Chen CP, Hsu JM. Tauberian theorems for weighted means of double sequences. Anal Math. 2000;26:243–262.
- Atanassov K. Intuitionistic fuzzy sets. Fuzzy Sets Syst. 1986;20:87–96.
- Zadeh LA. Fuzzy sets. Inform Control. 1965;8:338–353.
- Deschrijver G, Kerre EE. On the relationship between some extensions of fuzzy set theory. Fuzzy Sets Syst. 2003;133:227–235.
- Lei Q, Xu ZS. Derivative and differential operations of intuitionistic fuzzy numbers. Int J Intell Syst. 2015;30:468–498.
- Móricz F, Rhoades BE. Necessary and sufficient Tauberian conditions for certain weighted mean methods of summability. Acta Math Hungar. 1995;66(1–2):105–111.
- Sezer SA, Çanak İ. On the core of weighted means of sequences. Afrika Mat. 2021;32:363–367.
- Mursaleen M, Mohiuddine SA. Statistical convergence of double sequences in intuitionistic fuzzy normed spaces. Chaos Solitons Fractals. 2009;41:2414–2421.
- Mursaleen M, Mohiuddine SA, Edely OHH. On the ideal convergence of double sequences in intuitionistic fuzzy normed spaces. Comput Math Appl. 2010;59(2):603–611.
- Alghamdi MA, Alotaibi A, Lohani QMD, et al. Statistical limit superior and limit inferior in intuitionistic fuzzy normed spaces. J Inequal Appl. 2012;2012:96.
- Lei Q, Xu ZS. Fundamental properties of intuitionistic fuzzy calculus. Knowl Based Syst. 2015;76:1–16.
- Lei Q, Xu ZS. Intuitionistic fuzzy calculus. Cham (Switzerland): Springer; 2017.
- Ai ZH, Xu ZS, Lei Q. Limit properties and derivative operations in the metric space of intuitionistic fuzzy numbers. Fuzzy Optim Decis Mak. 2017;16:71–87.
- Ai ZH, Xu ZS, Lei Q. Fundamental properties with respect to the completeness of intuitionistic fuzzy partially ordered set. IEEE Trans Fuzzy Syst. 2017;25(6):1741–1751.
- Zhang S, Xu ZS. Infinite intuitionistic fuzzy series and product. Int J Intell Syst. 2017;32(6):645–662.
- Akram M, Ali G, Alcantud JCR. New decision-making hybrid model: intuitionistic fuzzy N-soft rough sets. Soft Comput. 2019;23:9853–9868.
- Zhang L, Zhan J, Xu Z, et al. Covering-based general multigranulation intuitionistic fuzzy rough sets and corresponding applications to multi-attribute group decision-making. Inf Sci. 2019;494:114–140.
- Alcantud JCR, Khameneh AZ, Kilicman A. Aggregation of infinite chains of intuitionistic fuzzy sets and their application to choices with temporal intuitionistic fuzzy information. Inf Sci. 2020;514:106–117.
- Ma R, Liu S, Xu ZS, et al. Series based on the new order in intuitionistic fuzzy environment. J Intell Fuzzy Syst. 2021;40(1):319–330.
- Riaz M, Hashmi MR. Linear diophantine fuzzy set and its applications towards multi-attribute decision-making problems. J Intell Fuzzy Syst. 2019;37:5417–5439.
- Yager RR. Pythagorean membership grades in multi-criteria decision making. IEEE Trans Fuzzy Syst. 2014;22:958–965.
- Zhang X, Xu Z. Extension of TOPSIS to multiple criteria decision making with pythagorean fuzzy sets. Int J Intell Syst. 2014;29:1061–1078.
- Peng X, Yang Y. Some results for pythagorean fuzzy sets. Int J Intell Syst. 2015;30:1133–1160.