Abstract
To investigate the impacts of uncertain parameters on simulated Pacific Walker circulation (PWC), a large number of perturbed parameter simulations are conducted using GAMIL2 (the Grid-point Atmospheric Model of IAP/LASG, version 2), and three different PWC indices are selected. The results show that the influences of some parameters on PWC are dependent on the selected index – a finding supported by the inconsistent responses of different indexes to these parameters. Among the nine parameters, the RH threshold for deep convection (RHCRIT) is the most sensitive in simulating PWC. Increased RHCRIT weakens deep convective heating and stratiform cooling, and strengthens shallow convective heating. Further analysis reveals that uncertain parameters affect the simulated PWC through changing the diabatic heating and vertical motion.
摘要
沃克环流是全球气候系统中的重要分量之一。目前为止,针对气候模式本身,特别是湿物理过程中的云相关不确定性参数对于沃克环流模拟的作用如何研究较少。本研究对利用均匀抽样方法定量化地分析了IAP/LASG的大气环流模式GAMIL2中不确定参数对沃克环流模拟的影响。结果显示,基于不同物理量定义的强度指数对某些参数的响应不一致,存在明显的不确定性。9个所选参数中,深对流触发的相对湿度阈值对于模拟沃克环流强弱的作用最敏感。不确定性参数通过改变非绝热加热和垂直速度进一步影响沃克环流的模拟强度。
1. Introduction
The Pacific Walker circulation (PWC) is characterized by rising motion over the western Pacific and sinking motion over the eastern Pacific (Bjerknes Citation1969). This pattern is directly driven by the active convection in the western Pacific warm pool, with the winds blowing from east to west at the surface and reversed in the upper troposphere. The change in PWC under global warming has been a hot topic. Vecchi et al. (Citation2006), Power and Kociuba (Citation2011), Tokinaga et al. (Citation2012), and Yu and Zwiers (Citation2010) found that PWC weakened during the twentieth century, based on changes in the gradient of sea level pressure (SLP), , the gradient of sea surface temperature (SST), and the atmospheric zonal mass streamfunction, ψ, along the equatorial Pacific. The weakening of PWC under global warming is theoretically expected to be caused by a decrease in convective mass fluxes, which balances the slower increase in global-mean precipitation at a rate of around 1%–3% K−1, compared to the increase in atmospheric water vapor content at a rate of around 7% K−1, with constant relative humidity (Held and Soden Citation2006). However, some studies have argued that the twentieth century PWC was strengthened, based on
(L’Heureux, Lee, and Lyon Citation2013; Sandeep, Stordal, and Sardeshmukh Citation2014), ψ (Ma and Zhou Citation2016), and boundary layer water vapor transport (Sohn and Park Citation2010). Although these conflicting results regarding PWC trends are closely associated with the different time periods and datasets employed, an explanation has not been sought in terms of the different PWC indices (PWCIs).
On the other hand, most of the GCMs that have participated in CMIP produce a descending trend of PWC in historical runs and scenario projections, which mainly results from greenhouse gas warming (Dinezio, Vecchi, and Clement Citation2013; Sandeep, Stordal, and Sardeshmukh Citation2014; Vecchi and Soden Citation2007). Aerosol forcing appears to drive a strengthening PWC (Dinezio, Vecchi, and Clement Citation2013). Ma and Zhou (Citation2016) reported that AGCM simulations exhibit PWC enhancement for the past three decades, dominantly forced by La Niña–like SST. Most of these studies on the changes in PWC focused on the external and boundary forcings. However, whether the model itself – especially uncertain parameters in model physical schemes – could influence the simulated PWC, has not been fully investigated. It is known that model parameters are a major source of uncertainty in climate simulation and projection (e.g. Collins et al. Citation2011; Jackson et al. Citation2008; Murphy et al. Citation2004; Stainforth et al. Citation2005). Uncertain parameters have significant influences on the simulation of ENSO (Watanabe et al. Citation2011), the Madden–Julian Oscillation (Boyle et al. Citation2015), and the Asian summer monsoon (Yang et al. Citation2015). Moist parameters are closely associated with large-scale convection and precipitation, of which the strength and location have a profound influence on the intensity of PWC (Philander Citation1990; Sohn et al. Citation2016). Hence, the motivation behind the present study is to examine the impacts of different parameters on PWC simulation using different indices.
The remainder of the paper is organized as follows: The model used and the experimental design are briefly described in Section 2. In Section 3, a detailed analysis of the results is presented. Section 4 summarizes the study’s key findings and offers further discussion.
2. Model and methodology
2.1. Model description
Version 2 of the Grid-point Atmospheric Model of IAP/LASG (GAMIL2) is used in this study (Li, Lin, Yu, et al. Citation2013; Li, Wang, Dong, et al. Citation2013). GAMIL2 is also the atmospheric component of FGOALS-g2, which participated in CMIP5. It uses a finite difference dynamical core that conserves mass and effective energy while solving the primitive hydrostatic equations of a baroclinic atmosphere (Wang et al. Citation2004), with the horizontal resolution of 128 × 60 and the 26-sigma vertical resolution.
Moist physical processes in GAMIL2 are parameterized as three components: deep convection, shallow convection, and stratiform processes. The Zhang and McFarlane (Citation1995) and Zhang and Mu (Citation2005) parameterization is used for deep convection. GAMIL2 employs the shallow convective parameterization scheme proposed by Hack (Citation1994). The cloud macrophysics (Zhang et al. Citation2003) provides a subgrid environment for microphysical processes. Cloud microphysics is represented by the two-moment bulk microphysics parameterization of Morrison and Gettelman (Citation2008), in which both the number concentration and mass mixing ratio of cloud particles are predicted.
2.2. Selected parameters and experimental design
We focus on nine cloud-related parameters. Descriptions and perturbations for these parameters are summarized in Table . The lower and upper bounds of the parameters, based on previous studies and the experience of model developers, can represent the uncertainty of the parameters. Among the nine parameters, C0_deep, KE, ALFA, CAPELMT, and RHCRIT are from the deep convection scheme. The ranges of these deep convective parameters are mainly based on Jackson et al. (Citation2008), Li, Wang, Dong, et al. Citation(2013), Covey et al. (Citation2013), and Zhang et al. (Citation2015). The ranges of two shallow convective parameters, C0_shc and CMFTAU, are mainly based on Zhang et al. (Citation2015). RHMINL and RHMINH are cloud fraction parameters that are closely related to stratiform processes. Their ranges are given by Yang et al. (Citation2015).
Table 1. Default values, ranges, sampling intervals, number of samples, and descriptions of the nine parameters.
To explore the parameter sensitivities, the uniform sampling (US) method is used to sample points within the range of one parameter. The US method divides the range of one parameter into n equal bins, and then the lower and upper limits of the parameter range and the boundaries of the n bins are sampled in the parameter space. The US method can sample a small quantity of points to represent the entire parameter space. In this study, the US sampling for the parameters is conducted as follows: When one of the nine parameters is sampled in its space, the other eight parameters remain unchanged as their default values. All the sampled parameter sets are applied to conduct the experiments from July 2002 to December 2003, and the model outputs for 2003 are used for the analyses and comparisons.
The ERA-Interim (Simmons et al. Citation2006) and NCEP-2 (Kanamitsu et al. Citation2010) datasets are used to evaluate the model results.
2.3. Analysis method
To measure the strength of PWC, we use three PWCIs. The first is based on the zonal mass streamfunction and is expressed as:(1)
where ψ denotes the zonal streamfunction, a is the radius of the Earth, is the width of the band 5°S–5°N along the equator in radians, g is the gravitational acceleration, uD is the divergent component of the zonal wind, and p is the pressure (Yu and Zwiers Citation2010; Yu et al. Citation2012). To estimate PWC strength, the ψ is averaged between 150°E and 120°W.
The second index is defined by the effective boundary layer wind, VE. Comprehensive descriptions of VE were provided by Sohn and Park (Citation2010), and Sohn et al. (Citation2013, Citation2016). Here, we present only a brief description and derivation. The total horizontal water vapor transport can be defined by:
(2)
where is the horizontal wind, q is the specific humidity,
is the surface pressure, and p0 is the pressure at the top of the atmosphere. Then, the effective wind is given by:
(3)
where is the divergent component of
, and
is the vertically integrated specific humidity.
is shown as:
(4)
The easterly component of is proportional to the strength of the lower branch of the PWC. We calculate the PWCI by averaging the easterly component of
over the Pacific domain (10°S–10°N, 120°E–120°W), referred as
.
The third index is defined using the zonal SLP gradient (Dinezio, Vecchi, and Clement Citation2013; Power and Kociuba Citation2011; Vecchi et al. Citation2006), and is expressed as:(5)
3. Results
The observed and simulated ψ along the equator is displayed in Figure (a)–(c). Both reanalyses show that PWC is characterized by a broad region of rising motion over the western-central Pacific and sinking motion in the eastern equatorial Pacific. GAMIL2 captures the main features of the observed ψ. Nevertheless, a smaller center of negative ψ over the western Pacific (120°–150°E), a larger center of positive ψ over the central-eastern Pacific (180°–150°W), and stronger upward branch of PWC are shown in GAMIL2 compared to both reanalyses, indicating GAMIL2 simulates a relatively stronger PWC.
Figure 1. Vertical cross section of ψ in the tropical Pacific from (a) ERA-Interim, (b) NCEP-2, and (c) GAMIL2, and (d–f) the responses of ψ to different parameters (normalized to [0,1]). Model responses are calculated as the regression coefficients between output variables and input parameters. The dotted areas indicate that the responses are statistically significant at the 95% confidence level.
![Figure 1. Vertical cross section of ψ in the tropical Pacific from (a) ERA-Interim, (b) NCEP-2, and (c) GAMIL2, and (d–f) the responses of ψ to different parameters (normalized to [0,1]). Model responses are calculated as the regression coefficients between output variables and input parameters. The dotted areas indicate that the responses are statistically significant at the 95% confidence level.](/cms/asset/6c890f06-2412-4d59-a585-bfc9ce03f64f/taos_a_1392228_f0001_oc.gif)
Model responses are calculated as the regression coefficients between output variables and parameters. The responses of the three PWCIs to parameters are given in Table . It is clear that the responses of the different indexes to some parameters (C0_deep, KE, CAPELMT, C0_shc, and RHMINH) are inconsistent, implying that the influences of these parameters on PWC are dependent on the selected index. For example, larger precipitation efficiency for deep convection (C0_deep) can result in smaller ψ (−0.0785) and larger VE (0.0391), and a larger threshold of RH for high cloud (RHMINH) causes larger ψ (0.2297) and smaller (−0.4600). The responses of the PWCIs to the other four parameters (ALFA, CMFTAU, RHCRIT, and RHMINL) are the same in terms of their sign. Larger initial cloud downdraft mass flux (ALFA) and timescale for consumption rate of shallow CAPE (CMFTAU) cause smaller PWCIs, while a larger threshold value of RH for deep convection (RHCRIT) and threshold of RH for low clouds (RHMINL) induce larger PWCIs. ψ is most sensitive to RHMINL, with a regression coefficient reaching −0.8283; and VE and
are most sensitive to RHCRIT, with regression coefficients of 2.2469 and 1.4246, respectively. It is also apparent from Table that the responses of the three PWCIs to parameters are the same in terms of their sign when the responses are very strong. However, when the responses are weak, their signs are not fully the same. This means that the three PWCIs are not fully equivalent. To comprehensively investigate the influence of parameters on the different indexes, three parameters (CMFTAU, RHCRIT, and RHMINL) are selected for further analysis, based on the following criteria: (1) that at least two out of the three regression coefficients between the PWCIs and the selected parameter pass the significance test; and (2) that each of the three main moist physical parameterization schemes (shallow convection, deep convection, and stratiform processes) provides one parameter.
Table 2. Reponses of the Pacific Walker circulation indices’ variability percentages to different parameters’ variability percentages (based on the mean value of all sampled values for any parameter).
With larger CMFTAU, ψ is increased over the region to the west of 160°E and to the east of 120°W, but is significantly reduced over the 160°E–120°W region, with the largest responses at the 400-hPa level (Figure (d)). Thus, the rising branch of PWC over the western-central Pacific and sinking branch over the eastern Pacific become weaker. The effect of RHCRIT is found to be almost opposite to that of CMFTAU. Large RHCRIT contributes to increasing ψ over the 160°E–130°W region, with a maximum center at 500–600 hPa, and decreasing ψ (especially in the upper level to the west of 170°E and in the mid–lower level to the east of 130°W), which leads to a stronger rising branch and sinking branch (Figure (e)). Although RHMINL is used to diagnose the low-level layered cloud amount, RHMINL plays a more major role in influencing ψ in the mid-upper level. Increasing RHMINL could enhance ψ over the 150°E–120°W region (Figure (f)).
The easterly component of can be reasonably interpreted as the strength of PWC because the effective boundary layer wind is directly linked to water vapor transport from the eastern Pacific subsidence region to the western Pacific ascending region of the Walker circulation (Sohn et al. Citation2016). The responses of
, its potential function (PF), and SLP, to parameters are shown in Figure . Similar to the impact on
, CMFTAU and RHCRIT also exhibit opposite influences on the response of
and PF. With larger CMFTAU, PF is increased to the west of 170°W, with a maximum over the western Pacific convection center (150°E–180°) accompanied by anomalous divergence, and reduced to the east of 170°W (Figure (a)). This leads to anomalous westerlies over the equatorial Pacific. The SLP response is highly correlated with the response of
. The anomalous divergence over the western Pacific could induce stronger downward motion, such that the SLP increases (Figure (d)). Conversely, larger RHCRIT causes anomalous convergence (divergence) of
and smaller (larger) SLP over the western (eastern) Pacific, which induces stronger easterlies of the lower PWC branch (Figure (b) and (e)). Larger RHMINL causes greater PF to the east of the dateline and smaller PF in the Maritime Continent, as well as weaker easterlies to a certain extent (Figure (c)).
Figure 2. Responses of (a–c) VE (vectors) and its potential function (coloring) to different parameters. (d–f) As in (a–c) but for SLP. Model responses are calculated as the regression coefficients between output variables and input parameters. The dotted areas indicate that the responses are statistically significant at the 95% confidence level.
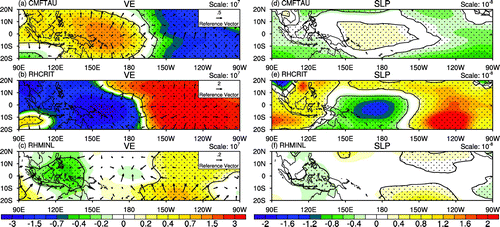
It is known that surface divergence is closely related to vertical motion. Diabatic heating (cooling) and adiabatic upward cooling (downward heating) are balanced in the tropics (Holton Citation1992). To further understand the impact of parameters on vertical motion, we examine the responses of diabatic heating (DH) from moist processes in Figure . By prolonging the adjustment time, larger CMFTAU directly weakens the shallow convective heating, which dominates the weakened total latent heating (LH) over the western Pacific convection region (150°E–180°) (Figure (a) and (j)). Through the interactions with shallow convection, the deep convective heating and stratiform cooling in the mid-lower troposphere are both strengthened (Figure (d) and (g)). The increased deep convective heating dominates the stronger LH over the eastern Pacific in the mid–high troposphere (Figure (j)). Such anomalous vertical motion with longitude corresponds to the anomalously weaker rising branch of PWC over the western-central Pacific and weaker sinking branch over the eastern Pacific (Figure (d)). For RHCRIT, a larger value increases the threshold for triggering deep convection, so that it directly weakens the deep convective heating and indirectly leads to less stratiform cooling and more shallow convective heating (Figure (b), (e), and (h)). The increased LH over 150°E–180° may be attributable to the decreased stratiform evaporation and increased shallow convective heating, while the decreased LH over the eastern Pacific between 850 hPa and 600 hPa is mainly caused by the decreased deep convective heating (Figure (k)). Similarly, the total LH response to RHCRIT coincides with the vertical velocity response (Figure (e)). Despite a strong direct effect on the stratiform process in the lower troposphere (Figure (f)), larger RHMINL strengthens (weakens) the LH in the central-eastern Pacific in the mid–high troposphere, mainly caused by the deep convection changes (Figure (c) and (l)). In short, changes the total moist processes below 850 hPa are dominated by changes in stratiform cooling and shallow convection. Above 850 hPa, changes the total moist processes are attributable to all three of their components. The PWC changes are not dependent on any single moist process. Its change is affected by changes in deep convection, shallow convection, and stratiform cloud processes combined. All these results indicate that parameters affect the vertical motion by changing the DH from physical processes, and further contribute to the PWC changes.
Figure 3. Vertical cross section of the responses of (a–c) the heating rate (units: K d−1) in deep convective, stratiform, shallow convective, and total moist processes over the equatorial (5°S–5°N) Pacific to parameters. Model responses are calculated as the regression coefficients between output variables and input parameters. The dotted areas indicate that the responses are statistically significant at the 95% confidence level.
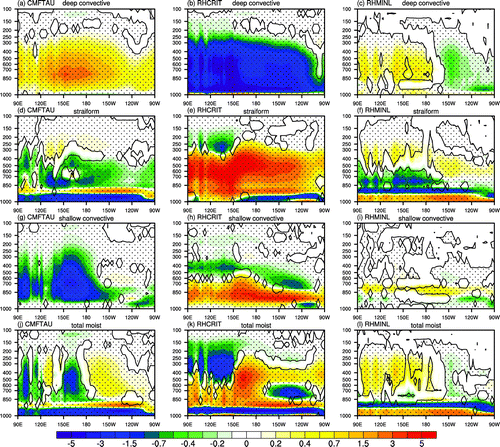
4. Summary and discussion
The impacts of uncertain parameters in moist physical schemes on PWC simulation are studied by applying the US method and conducting a large number of parameter-perturbed ensemble simulations. Three PWCIs, based on atmospheric mass flux (ψ), zonal gradient of SLP (), and effective wind related to water vapor transport (
), are used to quantify the strength of PWC, separately. The results show that GAMIL2 reproduces the main features of the observed PWC reasonably well, albeit with a relatively stronger ψ than in ERA-Interim and NCEP-2. Discrepancies in the response of the three PWCIs to several parameters (C0_deep, KE, CAPELMT, C0_shc, and RHMINH) imply that the influences of some parameters on PWC depend on the selected index. The measure of PWC using a single PWCI is not comprehensive.
The responses of the PWCIs to CMFTAU, RHCRIT, and RHMINL are selected for detailed investigation. Larger CMFTAU (RHCRIT, RHMINL) causes decreased (increased) PWCI values. Larger CMFTAU generally reduces the ψ over the 150°E–120°W region. Over the western Pacific convection center, the weakened rising motion coincides with increased SLP, which induces anomalous divergence of . Larger CMFTAU also weakens the sinking motion over the eastern Pacific. The changes in vertical velocity with longitude could lead to anomalous surface westerlies. The effect of RHCRIT on PWC is almost opposite to that of CMFTAU. A larger value of RHCRIT causes stronger upward (downward) motion over the western (eastern) Pacific, corresponding to anomalous convergence (divergence) of
and decreased (increased) SLP. These could lead to stronger surface easterlies over the equatorial Pacific. As for the increased RHMINL, the ψ over the 150°E–120°W region is increased, especially in the mid–high troposphere. Anomalous convergence (divergence) of
in the Maritime Continent (eastern Pacific) also coincides with the decreased (increased) SLP.
The simulated DH is closely associated with these cloud and precipitation parameters. The decreased shallow convective heating directly induced by larger CMFTAU dominates the weakened LH over the western Pacific. Through interaction among moist processes, larger CMFTAU indirectly increases the deep convective heating and stratiform cooling. Such increased deep convective heating contributes to the increased LH over the eastern Pacific in the mid–high troposphere. These interactions among moist processes are also found in the experiments of RHCRIT and RHMINL. Larger RHCRIT directly decreases deep convective heating, and indirectly decreases the shallow convective heating and stratiform cooling, which dominates the decreased LH over the western Pacific. In the mid–high troposphere, the total LH response dominates that of the total DH. RHMINL has a strong direct effect on the stratiform processes in the lower troposphere, but causes LH changes in the mid–high troposphere through its indirect effect on deep convection. The response of vertical velocity shows good agreement with that of total LH, indicating that parameters affect the vertical motion through changing the DH from physical processes, and further influence the simulated PWC.
Several limitations should be considered and deserve future investigation. Firstly, parameter responses are represented by the regression coefficients between model variables and parameters. The measure of responses could further be explained by the linear, high-order terms of parameters. Secondly, in this study, when one parameter is perturbed, the other parameters are kept unchanged. In future, several efficient sampling approaches, such as the Latin hypercube sampling method, could be used to evaluate the interaction among parameters and its role in affecting simulated PWC. Surrogate models are also useful for examining the interaction among parameters (Yan et al. Citation2015). Moreover, due to length limitations, deep analysis of the interaction among moist processes has not been given in detail in this paper.
Disclosure statement
No potential conflict of interest was reported by the authors.
Funding
This work was jointly funded by the National Key Research Project [grant number 2016YFB0200805], the National Natural Science Foundation of China [grant number 41622503], [grant number 41475043], [grant number 41405073], and [grant number 91530323], Open Fund of Key Laboratory of Data Analysis and Applications, SOA [grant number LDAA-2014-03], and the National Key Basic Research Program of China [grant number 2015CB954101].
Acknowledgments
The authors acknowledge ZHANG Tao and XU Haoyu of Tsinghua University for their help with the model configuration.
References
- Bjerknes, J. 1969. “Atmospheric Teleconnections from the Equatorial Pacific.” Monthly Weather Review 97: 163–172. doi:10.1175/1520-0493(1969)097, 0163:ATFTEP.2.3.CO;2.
- Boyle, J. S., S. A. Klein, D. D. Lucas, H.-Y. Ma, J. Tannahill, and S. Xie. 2015. “The Parametric Sensitivity of CAM5’s MJO.” Journal of Geophysical Research Atmospheres 120 (4): 1424–1444. doi:10.1002/2014JD022507.
- Collins, M., B. B. B. Booth, B. Bhaskaran, G. R. Harris, J. M. Murphy, D. M. H. Sexton, and M. J. Webb. 2011. “Climate Model Errors, Feedbacks, and Forcings: A Comparison of Perturbed Physics and Multi-model Ensembles.” Climate Dynamics 36 (9): 1737–1766. doi:10.1007/s00382-010-0808-0.
- Covey, C., D. D. Lucas, J. Tannahill, X. Garaizar, and R. Klein. 2013. “Efficient Screening of Climate Model Sensitivity to a Large Number of Perturbed Input Parameters.” Journal of Advances in Modeling Earth Systems 5 (3): 598–610. doi:10.1002/jame.20040.
- Dinezio, P. N., G. A. Vecchi, and A. C. Clement. 2013. “Detectability of Changes in the Walker Circulation in Response to Global Warming*.” Journal of Climate 26 (12): 4038–4048. doi:10.1175/JCLI-D-12-00531.1.
- Hack, J. J. 1994. “Parameterization of the Moist Convection in the NCAR Community Climate Model CCM2.” Journal of Geophysical Research Atmospheres 99 (D3): 5541–5568. doi:10.1002/2014MS000315.
- Held, I. M., and B. J. Soden. 2006. “Robust Responses of the Hydrological Cycle to Global Warming.” Journal of Climate 19: 5868–5699. doi:10.1175/JCLI3990.1.
- Holton, J. R. 1992. An Introduction to Dynamic Meteorology, 511. 3rd ed. Waltham, MA: Academic Press.
- Jackson, C. S., M. K. Sen, G. Huerta, Y. Deng, and K. P. Bowman. 2008. “Error Reduction and Convergence in Climate Prediction.” Journal of Climate 21 (24): 6698–6709. doi:10.1175/2008JCLI2112.1.
- Kanamitsu, M., W. Ebisuzaki, J. Woollen, S. K. Yang, J. J. Hnilo, M. Fiorino, and G. L. Potter. 2010. “NCEP-DOE AMIP-II Reanalysis (R-2).” Bulletin of the American Meteorological Society 83 (11): 1631–1643. doi:10.1175/BAMS-83-11-1631.
- L’Heureux, M. L., S. Lee, and B. Lyon. 2013. “Recent Multidecadal Strengthening of the Walker Circulation Across the Tropical Pacific.” Nature Climate Change 3 (3): 571–576. doi:10.1038/nclimate1840.
- Li, L. J., P. F. Lin, Y. Q. Yu, B. Wang, T. J. Zhou, L. Liu, J. Liu, et al. 2013. “The Flexible Global Ocean-atmosphere-land System Model Version g2.” Advances in Atmospheric Sciences 30 (3): 543–560. doi:10.1007/s00376-012-2140-6.
- Li, L. J., B. Wang, L. Dong, L. Liu, S. Shen, N. Hu, W. Q. Sun, et al. 2013. “Evaluation of Grid-point Atmospheric Model of IAP LASG, Version 2.0 (GAMIL 2.0).” Advances in Atmospheric Sciences 30 (3): 855–867. doi:10.1007/s00376-013-2157-5.
- Ma, S. M., and T. J. Zhou. 2016. “Robust Strengthening and Westward Shift of the Tropical Pacific Walker Circulation During 1979–2012: A Comparison of 7 Sets of Reanalysis Data and 26 CMIP5 Models.” Journal of Climate 29 (9): 3097–3118. doi:10.1175/JCLI-D-15-0398.1.
- Morrison, H., and A. Gettelman. 2008. “A New Two-moment Bulk Stratiform Cloud Microphysics Scheme in the Community Atmosphere Model, Version 3 (CAM3). Part I: Description and Numerical Tests.” Journal of Climate 21 (15): 3642–3659. doi:10.1175/2008JCLI2105.1.
- Murphy, J. M., D. M. H. Sexton, D. N. Barnett, G. S. Jones, M. J. Webb, M. Collins, and D. A. Stainforth. 2004. “Quantification of Modelling Uncertainties in a Large Ensemble of Climate Change Simulations.” Nature 430 (7001): 768–772. doi:10.1038/nature02771.
- Philander, S. G. 1990. El Nino, La Nina, and the Southern Oscillation, 293. London: Academic Press.
- Power, S. B., and G. Kociuba. 2011. “What caused the Observed Twentieth-century Weakening of the Walker Circulation?” Journal of Climate 24 (24): 6501–6514. doi:10.1175/2011JCLI4101.1.
- Sandeep, S., F. Stordal, and P. D. Sardeshmukh. 2014. “Pacific Walker Circulation Variability in Coupled and Uncoupled Climate Models.” Climate Dynamics 43 (1–2): 103–117. doi:10.1007/s00382-014-2135-3.
- Simmons, A., S. Uppala, D. Dee, and S. Kobayashi. 2006. “ERA-interim: New ECMWF Reanalysis Products from 1989 Onwards.” ECMWF Newsletter 110: 26–35. doi:ECMWF Newsletter n.110.
- Sohn, B. J., and S. C. Park. 2010. “Strengthened Tropical Circulations in Past Three Decades Inferred from Water Vapor Transport.” Journal of Geophysical Research Atmospheres 115 (D15): 4447–4458. doi:10.1029/2009JD013713.
- Sohn, B. J., S. W. Yeh, J. Schmetz, and H. J. Song. 2013. “Observational Evidences of Walker Circulation Change Over the last 30 years Contrasting with GCM Results.” Climate Dynamics 40 (7–8): 1721–1732. doi:10.1007/s00382-012-1484-z.
- Sohn, B. J., S. Lee, E. S. Chung, and H. J. Song. 2016. “The Role of the Dry Static Stability for the Recent Change in the Pacific Walker Circulation.” Journal of Climate 29 (8): 160210125433000. doi:10.1175/JCLI-D-15-0374.1.
- Stainforth, D. A., T. Aina, C. Christensen, M. Collins, N. Faull, D. J. Frame, J. A. Kettleborough, et al. 2005. “Uncertainty in Predictions of the Climate Response to Rising Levels of Greenhouse Gases.” Nature 433 (7024): 403–406. doi:10.1038/nature03301.
- Tokinaga, H., S. P. Xie, C. Deser, Y. Kosaka, and Y. M. Okumura. 2012. “Slowdown of the Walker Circulation Driven by Tropical Indo-Pacific Warming.” Nature 491 (7424): 439–443. doi:10.1038/nature11576.
- Vecchi, G. A., and B. J. Soden. 2007. “Global Warming and the Weakening of the Tropical Circulation.” Journal of Climate 20: 4316–4340. doi:10.1175/JCLI4258.1.
- Vecchi, G. A., B. J. Soden, A. T. Wittenberg, I. M. Held, A. Leetmaa, and M. J. Harrison. 2006. “Weakening of Tropical Pacific Atmospheric Circulation due to Anthropogenic Forcing.” Nature 441 (7089): 73–76. doi:10.1038/nature04744.
- Wang, B., H. Wan, Z. Z. Ji, X. Zhang, R. C. Yu, Y. Q. Yu, and H. T. Liu. 2004. “Design of a New Dynamical Core for Global Atmospheric Models Based on Some Efficient Numerical Methods.” Science in China Series A: Mathematics 47 (s1): 4–21.
- Watanabe, M., M. Kimoto, Y. Imada, and M. Chikira. 2011. “Convective Control of ENSO Simulated in MIROC5.” Journal of Climate 24 (2): 543–562. doi:10.1175/2010JCLI3878.1.
- Yan, H., Y. Qian, C. Zhao, H. L. Wang, M. H. Wang, B. Yang, X. H. Liu, and Q. Fu. 2015. “A New Approach to Modeling Aerosol Effects on East Asian Climate: Parametric Uncertainties Associated with Emissions, Cloud Microphysics, and Their Interactions.” Journal of Geophysical Research Atmospheres 120: 8905–8924. doi:10.1002/2015JD02344.
- Yang, B., Y. Zhang, Y. Qian, T. W. Wu, A. N. Huang, and Y. J. Fang. 2015. “Parametric Sensitivity Analysis for the Asian Summer Monsoon Precipitation Simulation in the Beijing Climate Center AGCM Version 2.1.” Journal of Climate 28 (14): 150423122028005. doi:10.1175/JCLI-D-14-00655.1.
- Yu, B., and F. W. Zwiers. 2010. “Changes in Equatorial Atmospheric Zonal Circulations in Recent Decades.” Geophysical Research Letters 37 (5): 137–147. doi:10.1029/2009GL042071.
- Yu, B., F. W. Zwiers, G. J. Boer, and M. F. Ting. 2012. “Structure and Variances of Equatorial Zonal Circulation in a Multimodel Ensemble.” Climate Dynamics 39 (9–10): 2403–2419. doi:10.1007/s00382-012-1372-6.
- Zhang, G. J., and N. A. McFarlane. 1995. “Sensitivity of Climate Simulations to the Parameterization of Cumulus Convection in the Canadian Climate Centre General Circulation Model.” Atmosphere-Ocean 33 (3): 407–446. doi:10.1080/07055900.1995.9649539.
- Zhang, G. J., and M. Mu. 2005. “Effects of Modifications to the Zhang-McFarlane Convection Parameterization on the Simulation of the Tropical Precipitation in the National Center for Atmospheric Research Community Climate Model, Version 3.” Journal of Geophysical Research Atmospheres 110: D09109. doi:10.1029/2004JD005617.
- Zhang, M. H., W. Lin, C. S. Bretherton, J. J. Hack, and P. J. Rasch. 2003. “A Modified Formulation of fraCtional Stratiform Condensation Rate in the NCAR Community Atmospheric Model (CAM2).” Journal of Geophysical Research Atmospheres 108 (D1): 4035. doi:10.1029/2002JD002523.
- Zhang, T., L. J. Li, Y. L. Lin, W. Xue, F. Xie, H. Y. Xu, and X. Huang. 2015. “An Automatic and Effective Parameter Optimization Method for Model Tuning.” Geoscientific Model Development Discussions 8 (11): 3791–3822. doi:10.5194/gmd-8-3579-2015.