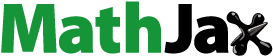
ABSTRACT
Introduction
Tacrolimus is a potent immunosuppressive drug with many side effects including nephrotoxicity and post-transplant diabetes mellitus. To limit its toxicity, therapeutic drug monitoring (TDM) is performed. However, tacrolimus’ pharmacokinetics are highly variable within and between individuals, which complicates their clinical management. Despite TDM, many kidney transplant recipients will experience under- or overexposure to tacrolimus. Therefore, dosing algorithms have been developed to limit the time a patient is exposed to off-target concentrations.
Areas Covered
Tacrolimus starting dose algorithms and models for follow-up doses developed and/or tested since 2015, encompassing both adult and pediatric populations. Literature was searched in different databases, i.e. Embase, PubMed, Web of Science, Cochrane Register, and Google Scholar, from inception to February 2023
Expert Opinion
Many algorithms have been developed, but few have been prospectively evaluated. These performed better than bodyweight-based starting doses, regarding the time a patient is exposed to off-target tacrolimus concentrations. No benefit in reduced tacrolimus toxicity has yet been observed. Most algorithms were developed from small datasets, contained only a few tacrolimus concentrations per person, and were not externally validated. Moreover, other matrices should be considered which might better correlate with tacrolimus toxicity than the whole-blood concentration, e.g. unbound plasma or intra-lymphocytic tacrolimus concentrations.
1. Introduction
Tacrolimus is the most frequently used immunosuppressant for the prevention of acute rejection after kidney transplantation [Citation1]. The target of tacrolimus (formerly known by its investigational name FK-506) is the immunophilin FKBP-12 FK506-binding protein (FKBP-12). The tacrolimus-FKBP-12 complex inhibits the phosphatase activity of the enzyme calcineurin, which is present in T lymphocytes [Citation2]. This results in suppression of T lymphocyte growth factors such as interleukin-2, and the inhibition of T lymphocyte activation and proliferation [Citation3]. Through inhibition of the formation of T helper lymphocytes, the B lymphocyte response can be prevented [Citation4]. Although tacrolimus is highly effective, it is also toxic. Side effects include nephrotoxicity (both acute and chronic), neurotoxicity, hypertension, opportunistic infections and post-transplant diabetes mellitus (PTDM). Acute rejection and tacrolimus-induced toxicity seem to have a drug-concentration dependent relationship, where low pre-dose concentrations are associated with rejection and overexposure to toxicity [Citation5], although this has not been a universal finding [Citation6]. To balance the efficacy and toxicity of tacrolimus, which is complicated by the highly variable intra- and inter-patient pharmacokinetics (PK), therapeutic drug monitoring (TDM) is routinely performed for tacrolimus targeting its so-called ‘therapeutic range’ [Citation7]. The parameter most widely used for TDM is the tacrolimus whole-blood pre-dose concentration (C0) [Citation8].
1.1. Tacrolimus’ pharmacokinetics
Following oral administration, tacrolimus is absorbed in the small intestine and colon and subsequently undergoes first-pass metabolism in the intestinal wall. In the circulation, tacrolimus binds excessively, around 95%, to erythrocytes as they have a high concentration of the tacrolimus receptor FKBP-12. In plasma, the majority of tacrolimus, around 90%, is bound to plasma proteins, such as albumin and α1-acid glycoprotein [Citation5]. Intracellular concentrations of tacrolimus in enterocytes are lowered by efflux back into the intestinal lumen, mediated by the drug transporter P-glycoprotein (encoded by the ABCB1 gene). P-glycoprotein is present in enterocytes, which limits the bioavailability of tacrolimus, and in lymphocyte membranes, where it limits lymphocyte inhibition [Citation9]. Tacrolimus is mostly metabolized in the liver by the cytochrome P450 (CYP) enzyme system (CYP3A4 and CYP3A5) [Citation10] and has a very low hepatic extraction ratio (3%) with a clearance (CL) of approximately 2 L/h [Citation11]. The main route of tacrolimus excretion is biliary (95%), whereas renal excretion accounts for only ~3% of unchanged tacrolimus [Citation5].
Erythrocyte-bound tacrolimus is thought not to be therapeutically active since only free drug molecules are able to cross cellular membranes and bind to its receptors at the target site [Citation12,Citation13]. The unbound tacrolimus fraction in plasma (normally <0.1% compared to whole-blood) is considered therapeutically active [Citation14]. Hypoalbuminemia may influence the unbound fraction of tacrolimus (and is frequent in kidney transplant recipients) [Citation15], whereas hematocrit is believed not to influence the unbound fraction [Citation16]. The intracellular tacrolimus concentration in T lymphocytes might better reflect the truly pharmacologically active fraction [Citation17,Citation18], since tacrolimus is used to inhibit T lymphocytes [Citation19].
1.2. Intra- and inter-individual variability
In most kidney transplant recipients, absorption of tacrolimus occurs fast with reported peak whole-blood concentrations within 0.5–2 hours after ingestion [Citation20]. However, there exists a large inter-patient variability in the tacrolimus absorption rate, resulting in a flat absorption profile, an extended lag time (e.g. the time it takes for absorption to start after drug ingestion) or in secondary peaks of absorption, with bioavailability (F) ranging from ~5% to 95% (reviewed in [Citation5]). Drug efflux transporters [Citation9], tacrolimus formulation type, fasting state [Citation21] and enteric metabolism (i.e. diarrhea [Citation22,Citation23]) affect absorption. The effect of diabetes mellitus on tacrolimus’ PKs is less-clearly defined and may relate to altered adipose tissue blood flow, muscle blood flow and delayed gastric emptying [Citation21,Citation24]. Concerning the volume of distribution (Vd), a wide range has been observed (from 0.97 to 104.8 L/kg) which is explained by the high lipophilicity of tacrolimus (reviewed in [Citation5]).
Single-nucleotide polymorphisms (SNPs) in genes encoding for drug transporting proteins and drug metabolizing enzymes (reviewed in [Citation25]), have their effect on the metabolism of tacrolimus and its inter-individual variability. Drug transporting proteins for tacrolimus (reviewed in [Citation26]) can be classified into two super families; SLCs (solute carrier transporters) and ABCs (ATP-binding cassette transporters). The most extensively studied intestinal drug efflux pump P-glycoprotein might partly cause tacrolimus’ low oral bioavailability, in addition to its importance for the distribution of tacrolimus [Citation27]. However, the contribution of all the ABCB1 gene SNPs on the protein’s function remains unclear [Citation25]. Regarding drug metabolizing enzymes, about 40–50% of the inter-patient variability in tacrolimus dose requirement can be explained by CYP3A5 gene polymorphisms [Citation28,Citation29]. Individuals carrying the CYP3A5*1 allele, CYP3A5 expressers, require a 50% higher tacrolimus dose compared to individuals homozygous for the CYP3A5*3 allele, CYP3A5 non-expressers [Citation30]. Other, less frequent, variant alleles include CYP3A5*6 and CYP3A5*7, which are both similar in function compared to the CYP3A5*3 allele, e.g. loss of protein function [Citation31]. Regarding the CYP3A4 SNP, individuals carrying the CYP3A4*22 allele, which is associated with reduced CYP3A4 enzymatic activity, require a 20% lower tacrolimus dose compared to individuals carrying the CYP3A4*1 allele [Citation32–34]. Besides inter-individual differences in ABCB1 and CYP3A activity, the P450 oxidoreductase (POR) enzyme is thought to explain some of the residual variability in tacrolimus CL. It was suggested that the POR*28 SNP contributes to increased tacrolimus metabolism, and thus a higher dose requirement in CYP3A5 expressers [Citation35–38].
Numerous other factors have been associated with the apparent whole-blood clearance (CL/F) of tacrolimus. Increasing age of the recipient was demonstrated to have an effect on tacrolimus’ PKs, resulting in a decreased CL/F [Citation39,Citation40]. Hematocrit could predict variability in tacrolimus whole-blood concentrations but is also highly variable and increases substantially after kidney transplantation [Citation16]. The effects of ethnicity are often tied to the different prevalence of CYP3A gene SNPs across different ethnicities [Citation25]. These differences can lead to lower tacrolimus pre-dose concentrations and reduced graft survival in African American recipients compared to their Caucasian counterparts [Citation25,Citation31]. The circadian rhythm was also demonstrated to influence tacrolimus CL/F, with a higher C0 and area-under the concentration versus time-curve (AUC) after the morning dose compared to the night dose [Citation41–43]. Other factors include drug–drug interactions [Citation44], time after transplantation, liver function, and bodyweight (BW). However, BW alone is known to correlate poorly with the required tacrolimus dose and overweight patients are at risk of overexposure [Citation39,Citation45–47].
1.3. Dosing algorithms
According to the Summary of Product Characteristics, the tacrolimus starting dose should commence at a dose of 0.20–0.30 mg/kg BW/day [Citation48,Citation49], despite the known poor correlation between BW and CL/F. First-steady state following BW-based dosing is 2–4 days (five times its half-life) after initiation of tacrolimus treatment [Citation50]. Several studies demonstrated that only 18.5–37.4% of the recipients were within the target range at first steady-state following BW-based dosing [Citation51–53]. Furthermore, despite the use of TDM, it can take as much as 3 weeks for a patient to reach the target concentration range [Citation52,Citation53]. In order to limit the time of exposure to tacrolimus concentrations outside the target range, dosing algorithms have been developed over the past decade. A dosing algorithm is able to provide calculations based on medical data to define the need for a reduced or increased dose of tacrolimus rather than a standard BW-based dose. Starting dose algorithms could aid physicians in individualizing a patient’s dose requirement based on numerous variables instead of BW alone, whereas maintenance dose algorithms could also take numerous variables into account in comparison to TDM.
2. Methods
Our research group previously published an overview of dosing algorithms for initiation of the immunosuppressive drug ciclosporin, tacrolimus and mycophenolic acid in solid organ transplant recipients [Citation54]. The present paper aims to provide a comprehensive overview of the newly developed and prospectively tested population PK (popPK) models for tacrolimus since then (2015). A literature search was performed using Embase, Medline (Ovid), Web of Science Core Collection (Web of Knowledge), Cochrane (Wiley) and Google Scholar, from inception to February 2023 for this review. Search terms included ‘kidney transplantation,’ ‘tacrolimus,’ ‘dose-response relationship,’ ‘pharmacokinetics’ and ‘models.’ The full literature search is described in the supplementary information. This search retrieved n = 515 articles, of which duplicates were removed (n = 4), and articles were excluded based on title-abstract screening (n = 391). Of the remaining 120 articles, 95 were excluded for the following reasons; only the abstract was available (n = 31), it was a review (n = 20), no popPK modeling was performed (n = 31), other transplant recipients than kidney (n = 3), or the article was discussed in the previously published overview (n = 10). Thus, n = 25 articles were included (adults n = 18, pediatrics n = 4, trials prospectively testing dosing algorithms n = 3) ().
This review is divided in sections considering adult and pediatric kidney transplant recipients, and these sections are further subdivided to discuss covariates that are incorporated in the identified popPK models and to review trials that tested these popPK models prospectively. Specifically for adults, we tested the popPK models on our database of kidney transplant recipients transplanted in the Erasmus MC consisting of n = 59 kidney transplant recipients [Citation55].
3. Kidney transplantation dosing algorithms
3.1. Adult transplant recipients
New developed dosing algorithms for the starting dose of tacrolimus in adult transplant recipients are presented in , along with the main findings of these popPK studies (n = 12) [Citation56–67]. If no dosing algorithm was available, this was calculated based on the popPK model. All starting dose algorithms were rewritten to achieve a tacrolimus target C0 of 10 ng/mL, which corresponds with an AUC of 175 ng/h/mL [Citation1,Citation8]. The formula to create the required total daily dose based on the CL/F is as follows: . The identified models for follow-up doses by use of a PK software tool (model informed precision dosing; MIPD) of tacrolimus are presented in , along with the main findings of these popPK studies (n = 6) [Citation68–73]. The trials prospectively testing dosing algorithms will be discussed in detail in a subsequent section (n = 3) [Citation55,Citation74,Citation75].
Table 1. Tacrolimus starting dose algorithms for adult kidney transplant recipients.
Table 2. Models for tacrolimus CL/F for model informed precision dosing (MIPD) in adult kidney transplant recipients.
3.1.1. Covariates incorporated in popPK models
As presented in , an overarching covariate tested and included in the popPK models is the CYP3A genotype [Citation57–60,Citation62–68,Citation71–73]. Although the relationship between the CYP3A5*3, CYP3A5*6 and CYP3A4*22 SNPs and tacrolimus dosing requirements is clear, there is no evidence that genotype-based dosing improves clinical outcomes. Two RCTs investigated the proportion of patients within the tacrolimus therapeutic range after six unaltered doses based on CYP3A5-guided dosing [Citation52,Citation53]. Only the TACTIQUE study demonstrated a small increase in this proportion [Citation53], whereas neither of these two clinical studies demonstrated an effect on the clinical outcome, i.e. less acute rejection as a result of genotype-guided dosing. In addition, it may be difficult to assess the significance of any single SNP due to ethnic variation in the prevalence of CYP3A5, CYP3A4 and ABCB1 genotypes being high and clinically relevant. When conducting a study within one population, there might not be a high enough number of patients with each SNP. Possibly, comparisons across populations increase the chance that there are other (unidentified) differences between the populations that contribute to these effects.
Hematocrit remained significant as a covariate in several models [Citation57,Citation60,Citation61,Citation63–65,Citation71–73], and all correlated inversely with tacrolimus CL. All discussed models were developed for whole-blood tacrolimus concentrations, as this is current TDM practice. However, as tacrolimus binds >95% to erythrocytes [Citation12,Citation13], changes in CL due to changes in hematocrit might alter the unbound, pharmacologically active concentration of tacrolimus [Citation14]. Perhaps, the unbound tacrolimus fraction in plasma is a variable we should investigate for the correlation with tacrolimus toxicity, or the intra-lymphocytic concentration, as this is the site of action of tacrolimus [Citation76]. The correlation between the whole-blood and intracellular concentration was recently investigated [Citation61], but no dosing algorithm can yet be developed from this, since the target concentration range for intracellular tacrolimus is unknown.
Post-operative days (POD) and/or the days a patient was on tacrolimus therapy remained significant in half of the models [Citation59,Citation62–64,Citation66,Citation70–73]. All came to the same conclusion, namely an increase in days (i.e. the longer a patient is after transplantation) will require a lower tacrolimus dose, independent of the lower target range in that period. Some demonstrated this for the immediate phase post-transplantation [Citation62–64,Citation66,Citation72], i.e. up to 12 days post-transplantation, whereas others demonstrated this for 125–180 days post-transplantation [Citation59,Citation71,Citation73]. This is in line with previous findings where tacrolimus dose-requirement (corrected for BW) decreased during the first post-operative year [Citation77]. The incorporation of days in a model might be biased if not adjusted for the corticosteroid dose, which is tapered in the post-operative phase [Citation78]. Likewise, the possibility of a simultaneous change (recovery) of kidney and/or liver function should be adjusted for.
Age of the kidney transplant recipient was tested as a covariate in nearly all popPK models, but only five studies demonstrated a correlation with tacrolimus CL and these were all starting dose algorithms [Citation57–60,Citation66]. All found a negative correlation (indicating a decreasing CL with aging), except for the popPK model by Ben-Fredj et al. [Citation59]. Perhaps, this could be attributed to the fact that Ben-Fredj et al. included relatively young kidney transplant recipients with a median age of 33.6 years ranging from 20 to 58 years [Citation59].
All studies investigated the effect of body composition parameters on tacrolimus CL, e.g. BW, body mass index (BMI), body surface area (BSA), fat mass, and lean bodyweight (LBW). No associations were found between PK parameters and BMI and fat mass, respectively. Interestingly, BW remained significant in four models [Citation59,Citation62,Citation72,Citation73], despite the known poor correlation between BW and tacrolimus CL as discussed in the introduction. Andrews et al. demonstrated a positive correlation between BSA and the required tacrolimus starting dose [Citation58]. However, when other studies applied this popPK base model [Citation60,Citation61], the correlation of BSA with tacrolimus CL disappeared and other body composition parameters were identified. LBW was found to correlate positively with the ratio between whole-blood and intracellular tacrolimus concentration [Citation61], although the implication of this for clinical care remains yet unclear as no target intracellular tacrolimus concentrations have been identified. Francke et al. conducted a study specifically investigating the effect of multiple body composition parameters measured by use of bio-impedance spectroscopy, e.g. adipose tissue mass, fat tissue index, lean tissue index, lean tissue mass, over-hydration, bio-impedance spectroscopy-derived phase angle, and estimated the patient’s BMI, BSA, ideal bodyweight and lean tissue mass [Citation60]. The phase angle, calculated as the arc tangent of reactance over resistance and thus relates to body cell mass, membrane integrity and hydration status [Citation79], resulted in the best correlation of all body composition parameters, in which the phase angle was positively correlated with a higher required tacrolimus starting dose [Citation60]. The use of body composition parameters other than BW may be interesting as tacrolimus is a lipophilic drug, and people in general tend to have a different body composition (muscle versus fat mass).
Concomitant drugs were often tested [Citation58,Citation59,Citation63–66,Citation68–70,Citation72,Citation73] but remained significant in only five models. Wuzhi capsule usage (a traditional Chinese medicine) was significant when tested [Citation63,Citation72]. Wuzhi capsules contain the extract of Schisandra sphenanthera, which is a medicinal herb and known to inhibit tacrolimus metabolism, leading to a lower dose requirement [Citation80]. Induction therapy with antithymocyte globulin [Citation59], use of an anti-cytomegalovirus drug [Citation66] and use of steroids [Citation66] were only of significant influence in one of the popPK models.
Numerous other covariates were tested in several popPK models (aspartate aminotransferase (AST), sex, hemoglobin, albumin, estimated glomerular filtration rate, serum creatinine), but each of these covariates remained only of significant influence in one of the popPK models [Citation56,Citation59,Citation60,Citation69,Citation70]. Therefore, these covariates might represent chance findings. Especially, the explanation for the association of tacrolimus CL with renal function (either estimated glomerular filtration rate or serum creatinine) remains unclear as tacrolimus undergoes hepatic elimination and almost no renal elimination. Sex was only identified as a significant covariate in the study in Tunisian patients by Ben-Fredj et al. [Citation59] but this might be attributed to the relatively high number of men included compared to women (70 men versus 32 women).
Apart from the selected covariates, more than half of the papers had a retrospective cohort design in common. Meaning that they were only able to re-use information from standard care sampling or previous studies, which mostly included only pre-dose concentrations (as opposed to AUC measurements).
3.1.2. Trials prospectively testing starting dose algorithms
Prospectively tested models are rare. Three models were identified for adults [Citation55,Citation74,Citation75]. In 2015, Størset et al. [Citation74] were the first to prospectively test a previously developed dosing algorithm by Åsberg et al. [Citation81]. In a single-center RCT, n = 78 de novo kidney transplant recipients were randomized to receive either MIPD (n = 39) or conventional, TDM-based follow-up dosing (n = 39; control group) during the first 8 weeks post-transplantation. For both groups, the tacrolimus starting dose was based on conventional BW dosing. For algorithm-based dosing, the model without CYP3A5 genotype was used as most of the recipients had not been genotyped before transplantation. For each following dose prediction, updated patient characteristics were needed: fat-free-mass (FFM), hematocrit, time after transplantation, tacrolimus dosing history and previously measured tacrolimus concentrations (up to 5 measurements). Standard-risk (tacrolimus target range 3–7 ng/mL) and high-risk (target range 8–12 ng/mL) recipients were analyzed separately. Overall, the proportion of concentrations per standard-risk patient within the target range was significantly higher with algorithm-based dosing (median 90%, 95% confidence interval (95% CI) 85–95%) than in the control group (78%, 95% CI 76–82%) (p-value <0.001). The same was observed for high-risk patients (77%, 95% CI 71–80%; algorithm-based dosing versus 59%, 95% CI 40–74%; control group; p-value = 0.04). However, there was only a significant difference in the median time to achieve the target concentration in high-risk patients (three days in algorithm-based dosing versus five days in control group; p-value = 0.04). There was no difference between the groups in frequency of biopsy-proven acute rejection, and considering tacrolimus toxicity, no difference in recorded infections and fasting glucose concentrations. However, a significant difference between the groups was observed for 2-hour plasma glucose concentrations at 8 weeks post-transplantation in favor for the algorithm based dosing group (5.9 mmol/L, 95% CI 5.6–6.6 mmol/L versus 6.8 mmol/L, 95% CI 6.1–8.1 mmol/L) (p-value = 0.008).
A single-arm prospective trial was performed by Francke et al. [Citation55], testing a starting dose algorithm previously developed by Andrews et al. [Citation58]. Fifty-nine de novo kidney transplant recipients were initiated on a starting dose based on the algorithm including CYP3A4 and CYP3A5 genotype, BSA and age, aiming for a target range 7.5–12.5 ng/mL at day 3 post-transplantation. Hereafter, TDM was performed as part of routine clinical care aiming for the same target range in weeks 1 to 2. The proportion of recipients within the target range at day 3 was 58% (95%-CI 47–68%), and the median tacrolimus C0 was 8.4 ng/mL (IQR 6.9–10.4). In most patients, the algorithm recommended a lower starting dose than a BW-based approach. This was most pronounced in obese patients and in CYP3A5 non-expressers [Citation55]. The participants were compared to a historic control group of patients who received a starting dose based on BW [Citation52]. In this group, the proportion of recipients within the target range at day 3 was 37.4% (95%-CI 28.5–47%), with a median tacrolimus C0 of 13.3 ng/mL (range 2.6–30.0) [Citation52]. In conclusion, it was demonstrated that algorithm-based tacrolimus dosing leads to the achievement of the desired target range in more patients than BW dosing.
A simulation trial was subsequently performed by these same authors [Citation75] including the previously discussed patients that received an algorithm-based tacrolimus starting dose followed by TDM (control group) [Citation55]. For every measured tacrolimus C0, a model-based dosing advice was simulated based on previous tacrolimus doses and measured C0, age, BSA, CYP3A4 and CYP3A5 genotype, hematocrit, albumin and creatinine (simulation group). A total of 190 C0 values were simulated and included. The proportion of observed tacrolimus C0 within the target range (7.5–12.5 ng/mL) following TDM was not significantly different from the simulated tacrolimus C0, 121 out of 190 C0 in observation group (63.7%, 95% CI 56.8–70.5) versus 126 out of 190 in the simulation group (66.3%, 95% CI 59.6–73.0) (p-value = 0.89) [Citation75]. This implicates that the additional effect of model-based follow-up dosing on the initial algorithm-based starting dose seems small.
3.1.3. Simulations of the starting dose algorithms
To demonstrate the potential effect of the starting dose algorithms for adults on the actual total daily starting dose of tacrolimus, we tested these algorithms aiming for an AUC of 350 ng/h/mL according to the methods described in section 3.1. This was done in a database of kidney transplant recipients transplanted in the Erasmus MC consisting of n = 59 kidney transplant recipients who were treated according to the starting-dose algorithm of Andrews et al. [Citation58], in which the median age at time of transplantation was 59 years (range 19–83 years), 63% was male (n = 37), 90% was Caucasian (n = 53), and the median BW at time of transplantation was 80.0 kg (range 49.3–119.5 kg). All baseline characteristics of these patients are described elsewhere [Citation55]. This algorithm-based starting dose was compared to the BW-based starting dose in these patients (0.20 mg/kg/day). In order to evaluate the performance of the models, we estimated the C0 that these patients would have had on day 3 post-transplantation, both for the BW-based and algorithm-based starting dose. This was calculated by use of the actual administered daily dose in these patients, the measured C0 in these patients at day 3 and the hypothetical algorithm-based or the BW-based daily starting dose of these patients: Estimated tacrolimus C0 = (algorithm-based daily starting dose OR BW-based daily starting dose) × measured tacrolimus C0 / administered daily dose [Citation55].
In our database, not all necessary values were available at pre-transplantation. Therefore, we chose to estimate the starting dose and C0 at day 3 based on the covariates measured at day 1 post-transplantation. The median BW-based starting dose was 16 mg/day (range 10–24 mg/day). The median algorithm-based starting dose per algorithm is presented in , along with their ranges. All proposed a lower starting dose compared to the BW-based starting dose of 16 mg/day.
The median estimated C0 at day 3 for the BW-based starting dose was 11.2 ng/mL (range 4.3–49.5 ng/mL). The median estimated C0 at day 3 for the algorithm-based starting dose per algorithm is plotted in . Only the algorithm of Ben-Fredj et al. [Citation59] had the median C0 in the therapeutic target range of 8–12 ng/mL (median 8.0 ng/mL, range 3.3–33.1 ng/mL). Most estimated C0 were under the lower limit of the target concentration range, whereas the algorithm by Sanghavi et al. [Citation66] was above. The algorithm-based starting dose and estimated C0 for the algorithm by Woillard et al. could not be calculated since the algorithm is not provided in the paper [Citation67].
Figure 2. Estimated tacrolimus pre-dose concentration at day 3 post-transplantation grouped per algorithm.
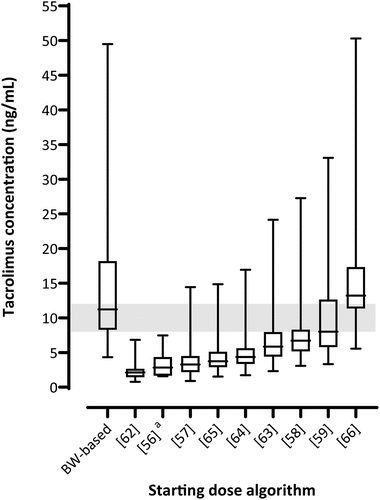
Most algorithms underperformed compared to BW-based dosing, although the variability of the estimated C0 was smaller. However, we estimated the doses and C0 based on the covariates measured at day 1 post-transplantation. In clinical care, these values of the first post-transplant day are of course not known at pre-transplantation when calculating the initial starting dose. On the other hand, incorporating laboratory values in a starting dose algorithm might be less reliable since most of these values differ greatly between pre- and post-transplantation. Furthermore, the algorithm-based starting dose for the algorithm of Alqahtani et al. [Citation56] could only be predicted for n = 6 kidney transplant recipients as only those had an AST concentration available at day 1 post-transplantation. Another limitation of implementing these algorithms on our patients is based on the ethnicity of the population, as most of these patients were Caucasian. Since Alqahtani et al. [Citation56] developed their model in a Saudi population, Ben-Fredj et al. [Citation59] and Henin et al. [Citation62] developed theirs in a Tunisian population, Jing et al. [Citation63] and Ling et al. [Citation64] in a Chinese population, Reséndiz-Galván et al. [Citation65] in a Mexican population and Sanghavi et al. [Citation66] in an African-American population, our database might not be representable for these algorithms. The model of Andrews et al. [Citation58] was developed in our population and would thus fit better compared to the other algorithms. Furthermore, the algorithm by Henin et al. was developed in a population that used a once-daily tacrolimus formulation [Citation62]. Subsequently, the correlation between the AUC and C0 might be different for this population compared to the target AUC of 350 ng/h/mL we used, and this has to be taken into consideration when interpreting the simulations. Finally, the target AUC of 350 ng/h/mL was based on the consensus report of the Immunosuppressive Drugs Scientific Committee of the International Association of Therapeutic Drug Monitoring and Clinical Toxicity (IATDMCT) [Citation8]. However, some models incorporate a calculated AUC based on their population, as, for example, the AUC of 444 ng/h/mL in the ‘Caucasian’ algorithm of Andrews et al. [Citation58].
3.2. Paediatric transplant recipients
presents an overview of the new developed dosing algorithms for the starting dose of tacrolimus in pediatric kidney transplant recipients, along with the main findings of these popPK studies (n = 3) [Citation82–84]. All starting dose algorithms were rewritten to achieve a tacrolimus C0 of 10 ng/mL, according to the methods described in section 3.1. If no dosing algorithm was available, this was calculated based on the popPK model. In the model identified for MIPD of tacrolimus in pediatric kidney transplant recipients is presented, along with the main findings of this popPK study (n = 1) [Citation85]. One study prospectively tested their dosing algorithm and this will be discussed in detail in a subsequent section [Citation83].
Table 3. Tacrolimus starting dose algorithms for pediatric kidney transplant recipients.
Table 4. Models for tacrolimus CL/F for model informed precision dosing (MIPD) in pediatric kidney transplant recipients.
3.2.1. Covariates incorporated in popPK models
All four identified popPK models include CYP3A5 genotype as a covariate, with the need for higher tacrolimus doses in case of expressers (range of 1.45–1.93 fold higher compared to non-expressers) [Citation82–85]. Indeed, in 2018, one RCT investigated the efficacy of dosing based on age and CYP3A5 genotype (intervention group) compared to standard care, BW dosing (control group) in n = 53 pediatric solid organ transplant recipients [Citation86]. Patients were randomized (2:1) to the intervention (n = 35) or control group (n = 18) and were further stratified by their genotype (expresser versus non-expressers, e.g. CYP3A5*1/*1 or CYP3A5*1/*3, and CYP3A5*3/*3, respectively) and by organ type (liver versus non-liver). Genotype dosing was also stratified by age (≤ or >6 years). The starting dose was unaltered for the first 36–48 hours and patients were followed for 30 days. Age and genotype-based dosing led to faster achievement of the desired tacrolimus target range compared to standard care BW dosing (median 3.4 days (IQR 2.6–6.6) versus 4.7 days (IQR 3.5–8.6); p-value = 0.049). However, a difference in (adverse) clinical outcomes was not observed between the different dosing strategies, which may be the result of under powering.
BW was incorporated in three popPK models [Citation82,Citation83,Citation85], all proposing a higher weight-normalized dose for children with a lower BW compared to children with a higher BW. This is in line with the previously developed starting dose algorithms [Citation87–89], discussed in our previous review [Citation54]. Age was tested in all four identified models but never demonstrated to influence tacrolimus CL significantly.
In contrast to the adult algorithms, hematocrit only remained significant in the study by Prytula et al. [Citation85]. Andrews et al. did identify hematocrit as a significant covariate, but retained it from their final starting dose algorithm because the last measured hematocrit before kidney transplantation did not significantly influence the CL and hematocrit itself often changes greatly after transplantation [Citation82]. On the other hand, many adult algorithms incorporated hematocrit as a covariate and this might be reasonable.
Prytula et al. also incorporated γ-glutamyl-transferase (γGT) in their model [Citation85]. Although it has been demonstrated previously that a decline in hepatic function could lead to a lower tacrolimus CL [Citation90], the other markers of liver function (e.g. AST or alanine aminotransferase (ALT) were not deemed to be of significant influence on tacrolimus CL in the model of Prytula et al [Citation85]. As the γGT is not entirely specific for hepato-biliary-dysfunction, the incorporation of this covariate in a popPK model for tacrolimus CL is open for discussion.
Donor type (e.g. living or deceased) only remained significant in the first model of Andrews et al. [Citation82]. However, it remains unclear why a kidney allograft from a deceased donor would induce a higher tacrolimus CL, since tacrolimus is mostly metabolized by the liver and undergoes almost no renal clearance. In their updated model [Citation83], this covariate was no longer significant.
3.2.2. Trial prospectively testing starting dose algorithm
Andrews et al. were the first to prospectively test their developed starting dose algorithm aiming for a tacrolimus C0 range of 10–15 ng/mL at day 3 [Citation83]. However, the interim analysis after the inclusion of n = 15 recipients demonstrated that the algorithm predicted really high doses (i.e. 0.80 mg/kg/day) in CYP3A5 expressers who received a kidney from a deceased donor (n = 3). Considering these high doses, the C0 was measured at day 1 or 2 post-transplantation and because these were too high, the tacrolimus dose was altered before day 3. Although the a priori criteria for success were met (31% of the recipients was on target at day 3 post-transplantation, whereas the minimum for success was set at 25%), the study was discontinued prematurely because of serious concern among the treating physicians regarding the possibility of overdosing in combination with the fact that 25% of the recipients were CYP3A5 expressers and received a kidney from a deceased donor. Thus, a new dosing algorithm was developed in an extended cohort, consisting of total n = 95 pediatric recipients. The final algorithm included BW and the CYP3A5 genotype and this was successfully internally validated [Citation83]. This model has not yet been prospectively tested.
4. Conclusions
Multiple tacrolimus dosing algorithms have been developed for the starting dose and follow-up doses for both adult and pediatric kidney transplant recipients. As shown in , hematocrit and CYP3A genotype are incorporated in most of these algorithms. Although many algorithms are available, the majority has not been validated externally by use of an external database, and only a few have been tested prospectively. These prospectively tested algorithms performed better than conventional BW-dosing for adults in terms of the time a patient needs to reach the target C0 [Citation55,Citation74]. Considering clinical outcomes of tacrolimus and toxicity, e.g. allograft rejection, onset of PTDM and the incidence of infections, no difference was observed between algorithm-based dosing and conventional BW-dosing with TDM. If this can be attributed to a lack of superiority of dosing algorithms themselves, an underpowered study, or to current questions regarding the precision of whole-blood tacrolimus C0, has yet to be answered. From a theoretical point of view, clinical outcomes of tacrolimus toxicity might better correlate with unbound plasma or intracellular tacrolimus concentrations instead of whole-blood concentrations. In order to test and implement dosing algorithms for these concentrations in the clinic, target ranges first have to be determined.
5. Expert opinion
The starting dose of tacrolimus is based exclusively on BW in the majority of settings [Citation48,Citation49]. TDM is accepted as the solution to any issues with the initial dose, although this practice was recently discussed [Citation7,Citation91]. Many popPK models have been developed over the years trying to change the current standard practice, but only a few were prospectively tested. Some demonstrated to be significantly better than BW-based dosing [Citation55,Citation74]. However, a few did bring to light the difficulties with modeling. Namely, misprediction errors in the model itself causing harm via over-exposure to tacrolimus [Citation83], or physicians changing the recommended dose due to it being too high/low in their eyes [Citation74], both highlighting the importance of properly validating algorithms before using them. While changing the dose may sound like a purely negative event, it can sometimes be beneficial, and prevent possible negative outcomes. Despite the difficulties, a few points were found that should be noted for the future. In adults, there may be a need for lower weight-based doses for obese patients and CYP3A non-expressers [Citation55], and for pediatric patients, higher weight-doses for lower BW and CYP3A expressers [Citation83]. As is evident, the latter two studies came to the same conclusion regarding dosing, mainly the role of CYP3A combined with BW.
The developed and prospectively tested dosing algorithms are not yet beneficial in limiting tacrolimus-related toxicity. A possible question is the correlation between the measured C0 and toxicity, since patients that have concentrations in the therapeutic window still develop adverse events. As tacrolimus CL differs greatly between individuals and patients with a higher CL requires a higher dose for a specific target range (fast metabolizers), these patients are possibly exposed to high tacrolimus peak concentrations (Cmax) in the first hours after oral administration [Citation92]. The Cmax is not routinely measured when performing TDM of tacrolimus. AUC could also be an important target, since the AUC in a fast metabolizer would be relatively high compared to a slower metabolizer with the same C0. This would also result in a higher Cmax. Moreover, other matrices should be considered which might better correlate with tacrolimus toxicity than the whole-blood concentration, e.g. unbound plasma (the therapeutically active tacrolimus [Citation14]) or intra-lymphocytic tacrolimus concentrations (pharmacologically active fraction at the site of action [Citation17,Citation18]). The first popPK model for the intra-lymphocytic concentration of tacrolimus has been developed [Citation61], however no target concentration for intracellular tacrolimus is (yet) known. An obstacle to obtain such target concentrations is the need for specific and sensitive biomarkers to rely on, such as the promising minimally invasive biomarker donor-derived cell-free DNA [Citation93].
In this review, popPK models have been divided into pediatric and adult models. Often, in both groups, the same covariates were tested and similar conclusions were drawn. However, we deemed it important to separate adult from pediatric models due to the many known physiological differences between children and adults, such as the age-induced decrease in tacrolimus CL [Citation82]. Half of the identified pediatric algorithms found BW and/or age to be significant on tacrolimus CL [Citation83,Citation85], which was not often the case for the identified adult algorithms. However, other studies demonstrated that elderly recipients (>65 years) have higher tacrolimus pre-dose concentrations when given the same dose as their younger adult counterparts [Citation40]. Furthermore, pediatric studies are much fewer in number [Citation82–85], which adds to the importance of separating the models so that the small quantities of data are fully covered.
While prospective testing would be ideal, validation is often the first step. The issue that many models share is the lack of external validation in a truly independent dataset, except for five studies [Citation57,Citation58,Citation72,Citation82,Citation85]. This lack of external validation may impact the performance of popPK models in RCTs. Some studies solved this by separating their patients into a model-building group and a validation group [Citation59,Citation64–66,Citation73]. External validation should be the norm, to allow the model to show generalizability, even if this is only in the same ethnic population. Although the starting dose algorithms in adult kidney transplant recipients developed in the same ethnic population demonstrated different simulated C0 (; Caucasian population [Citation57,Citation58], Chinese population [Citation63,Citation64], Tunisian population [Citation59,Citation62]). In addition, studies often use data from past trials, making them unable to obtain additional samples and/or information or rely on pre-dose concentrations only.
Along with validation, the availability of information on intra-patient variability is drastically different among studies. Most algorithms contain many tacrolimus concentrations per person [Citation57,Citation58,Citation62,Citation63,Citation65,Citation66,Citation69–71,Citation82–84]. However, the issue comes up with studies where there is barely more than one value per person [Citation56]. This might not be of importance for a starting dose algorithm, as this will leave the intra-patient variability out of the equation since you do not know on beforehand if a patient will have relatively high or low concentrations. However, the variability will determine the wideness of the range of concentrations, and the range will thus be smaller if most of the variability is explained by incorporated covariates. The inter-patient variability will be of issue in MIPD, when previous C0 will be taken into account. While inter-individual variability can be addressed (e.g. by determining individual clearance or performing post-hoc Bayesian analysis), the intra-individual variability cannot be addressed; hence, it could cause issues with clinical application of the model, as it will do for TDM.
Moreover, this review focused on popPK models. However, modeling is expanding quickly and other types of models should be considered. A clearer choice could be to include pharmacodynamics in the model, to obtain a full picture of the drug in the body [Citation94]. Apart from this, there are also artificial intelligence (AI) and machine learning (ML) based models being developed [Citation95–97], possibly able to identify new unknown (clusters) of impactful variables to improve models. Furthermore, with the rise in diversity and migration, a popPK model built for the current population may quickly become outdated in a dynamic patient population. In addition to being focused on popPK modeling, our review used dosing algorithms or converted CL algorithms into dosing. A drawback of this is that it ignores the effect of covariates on Vd, where Vd mostly has it influence on the Cmax, which can be of importance for tacrolimus-induced toxicity.
Lastly, the need for models or other methods for effective drug dosing will only increase in the coming years. Recent trends toward lower tacrolimus exposure or very low tacrolimus targets, when combined with everolimus and drug individualization, the need for clinical tools to avoid underexposure will probably increase. Whether this will be popPK models, AI, ML, or a combination of all three, only time will tell. However, creating a model is only half the challenge. The second half being the implementation of bedside design computer programs. The issue that arises here is that usage of such programs may not be suited for individuals without popPK modeling and/or mathematical experience. An attractive app/interface may thus be a good way to let physicians get involved in the action of predicting doses. This problem has been solved by the Limoges modeling group with their online expert system, where AUCs are determined by PK modeling and Bayesian estimation [Citation98]. As we have mentioned above, some physicians are not trusting of the model (the black box) making the decisions [Citation74], so a change in mind-set also remains part of the challenge.
The future of dosing algorithms for tacrolimus almost certainly includes some of the covariates we have seen here, even if they are not in their current models. With this in mind, we implore physicians not to discount a model from the start. However, creators of models should work with the physicians to address any concerns and keep the patient’s best interest as the top priority, along with conducting prospective validation of their models.
Article highlights
Since the first developed tacrolimus starting dose algorithm, many have been developed for the starting dose of tacrolimus. For the follow-up doses of tacrolimus by use of model informed precision dosing, many models have been developed. Hematocrit and CYP3A genotype are incorporated in most of these models. However, less than half of the models are externally validated and/or prospectively tested.
The few prospectively tested starting dose algorithms performed better than conventional bodyweight-dosing regarding the time for a patient to reach the tacrolimus target concentration.
In order to achieve physician acceptance of calculating the starting- and/or follow-up dose with a model, the developed models need to be externally validated (if not done yet), tested in a (randomized) clinical trial and have a good user interface.
Considering clinical outcomes of tacrolimus, allograft rejection and toxicity (e.g. the incidence of infections and onset of post-transplantation diabetes mellitus), no difference was observed between algorithm-based dosing and conventional BW-dosing with TDM.
Other covariates, like unbound plasma or intra-lymphocytic tacrolimus concentrations, should be considered, which might better correlate with tacrolimus toxicity than the whole-blood concentration. In order to test and implement dosing algorithms for these concentrations in the clinic, target ranges first have to be determined.
Declaration of interest
DA Hesselink has received lecture fees and consulting fees from Astellas Pharma, AstraZeneca, Chiesi Pharma, Medincell, Novartis Pharma, Sangamo Therapeutics and Vifor Pharma. He has received grant support from Astellas Pharma, Bristol-Myers Squibb, and Chiesi Pharma (paid to his institution). The authors have no other relevant affiliations or financial involvement with any organization or entity with a financial interest in or financial conflict with the subject matter or materials discussed in the manuscript apart from those disclosed.
Reviewer disclosures
Peer reviewers on this manuscript have no relevant financial or other relationships to disclose.
Supplements - Assessing Tacrolimus releated toxicity using dosing algorithms.docx
Download MS Word (21.4 KB)Supplementary material
Supplemental data for this article can be accessed online at https://doi.org/10.1080/17425255.2023.2250251.
Additional information
Funding
References
- Eckardt K-U, Kasiske BL, Zeier MG. Kidney disease: improving global outcomes transplant work G. KDIGO clinical practice guideline for the care of kidney transplant recipients. Am J Transplant. 2009 Nov;9(3):S1–155. doi: 10.1111/j.1600-6143.2009.02834.x
- Tamura K, Fujimura T, Iwasaki K, et al. Interaction of tacrolimus(FK506) and its metabolites with FKBP and calcineurin. Biochem Biophys Res Commun. 1994 Jul 15;202(1):437–443. doi: 10.1006/bbrc.1994.1947
- Taylor AL, Watson CJ, Bradley JA. Immunosuppressive agents in solid organ transplantation: mechanisms of action and therapeutic efficacy. Crit Rev Oncol Hematol. 2005 Oct;56(1):23–46. doi: 10.1016/j.critrevonc.2005.03.012
- Heidt S, Roelen DL, Eijsink C, et al. Calcineurin inhibitors affect B cell antibody responses indirectly by interfering with T cell help. Clin Exp Immunol. 2010 Feb;159(2):199–207. doi: 10.1111/j.1365-2249.2009.04051.x
- Staatz CE, Tett SE. Clinical pharmacokinetics and pharmacodynamics of tacrolimus in solid organ transplantation [review]. Clin Pharmacokinet. 2004;43(10):623–653. doi: 10.2165/00003088-200443100-00001.
- Bouamar R, Shuker N, Hesselink DA, et al. Tacrolimus predose concentrations do not predict the risk of acute rejection after renal transplantation: a pooled analysis from three randomized-controlled clinical trials(†). Am J Transplant. 2013 May;13(5):1253–1261. doi: 10.1111/ajt.12191
- Lemaitre F, Hesselink DA. TDM is Alive and Kicking! Ther Drug Monit. 2023 Feb 1;45(1):3–5. doi: 10.1097/FTD.0000000000001034
- Brunet M, van Gelder T, Åsberg A, et al. Therapeutic drug monitoring of tacrolimus-personalized therapy: second consensus report. Ther Drug Monit. 2019 Jun;41(3):261–307. doi: 10.1097/FTD.0000000000000640
- Kanado Y, Tsurudome Y, Omata Y, et al. Estradiol regulation of P-glycoprotein expression in mouse kidney and human tubular epithelial cells, implication for renal clearance of drugs. Biochem Biophys Res Commun. 2019 Nov 12;519(3):613–619. doi: 10.1016/j.bbrc.2019.09.021
- Hesselink DA, van Gelder T, van Schaik RH. The pharmacogenetics of calcineurin inhibitors: one step closer toward individualized immunosuppression? Pharmacogenomics. 2005 Jun;6(4):323–337. doi: 10.1517/14622416.6.4.323
- Möller A, Iwasaki K, Kawamura A, et al. The disposition of 14C-labeled tacrolimus after intravenous and oral administration in healthy human subjects. Drug Metab Dispos. 1999 Jun;27(6):633–636.
- Iwasaki K, Miyazaki Y, Teramura Y, et al. Binding of tacrolimus (FK506) with human plasma proteins re-evaluation and effect of mycophenolic acid. Res Commun Mol Pathol Pharmacol. 1996 Dec;94(3):251–257.
- Nagase K, Iwasaki K, Nozaki K, et al. Distribution and protein binding of FK506, a potent immunosuppressive macrolide lactone, in human blood and its uptake by erythrocytes. J Pharm Pharmacol. 1994 Feb;46(2):113–117. doi: 10.1111/j.2042-7158.1994.tb03752.x
- Undre NA, Stevenson P, Schäfer A. Pharmacokinetics of tacrolimus: clinically relevant aspects. Transplant Proc. 1999 Nov;31(7A):21S–24S. doi: 10.1016/S0041-1345(99)00788-5
- Hebert MF, Zheng S, Hays K, et al. Interpreting tacrolimus concentrations during pregnancy and postpartum. Transplantation. 2013 Apr 15;95(7):908–915. doi: 10.1097/TP.0b013e318278d367
- Størset E, Holford N, Midtvedt K, et al. Importance of hematocrit for a tacrolimus target concentration strategy. Eur J Clin Pharmacol. 2014 Jan;70(1):65–77. doi: 10.1007/s00228-013-1584-7
- Lemaitre F, Vethe NT, D’Avolio A, et al. Measuring intracellular concentrations of calcineurin inhibitors: expert consensus from the international association of therapeutic drug monitoring and clinical toxicology expert panel. Ther Drug Monit. 2020 Oct;42(5):665–670. doi: 10.1097/FTD.0000000000000780
- Sallustio BC. Monitoring intra-cellular tacrolimus concentrations in solid organ transplantation: Use of peripheral blood mononuclear cells and graft Biopsy tissue. Front Pharmacol. 2021;12:733285. doi: 10.3389/fphar.2021.733285
- Andrews LM, Li Y, De Winter BCM, et al. Pharmacokinetic considerations related to therapeutic drug monitoring of tacrolimus in kidney transplant patients. Expert Opin Drug Metab Toxicol. 2017 Dec;13(12):1225–1236. doi: 10.1080/17425255.2017.1395413
- Venkataramanan R, Swaminathan A, Prasad T, et al. Clinical pharmacokinetics of tacrolimus. Clin Pharmacokinet. 1995 Dec;29(6):404–430. doi: 10.2165/00003088-199529060-00003
- Bekersky I, Dressler D, Mekki Q. Effect of time of meal consumption on bioavailability of a single oral 5 mg tacrolimus dose. J Clin Pharmacol. 2001 Mar;41(3):289–297. doi: 10.1177/00912700122010104
- Maes BD, Lemahieu W, Kuypers D, et al. Differential effect of diarrhea on FK506 versus cyclosporine a trough levels and resultant prevention of allograft rejection in renal transplant recipients. Am J Transplant. 2002 Nov;2(10):989–992. doi: 10.1034/j.1600-6143.2002.21018.x
- van Boekel GA, Aarnoutse RE, van der Heijden JJ, et al. Effect of mild diarrhea on tacrolimus exposure. Transplantation. 2012 Oct 15;94(7):763–767. doi: 10.1097/TP.0b013e3182629e13
- Dostalek M, Akhlaghi F, Puzanovova M. Effect of diabetes mellitus on pharmacokinetic and pharmacodynamic properties of drugs. Clin Pharmacokinet. 2012 Aug 1;51(8):481–499. doi: 10.1007/BF03261926
- Tang JT, Andrews LM, van Gelder T, et al. Pharmacogenetic aspects of the use of tacrolimus in renal transplantation: recent developments and ethnic considerations. Expert Opin Drug Metab Toxicol. 2016 May;12(5):555–565. doi: 10.1517/17425255.2016.1170808
- Tron C, Lemaitre F, Verstuyft C, et al. Pharmacogenetics of Membrane transporters of tacrolimus in solid organ transplantation. Clin Pharmacokinet. 2019 May;58(5):593–613. doi: 10.1007/s40262-018-0717-7
- Lown KS, Mayo RR, Leichtman AB, et al. Role of intestinal P-glycoprotein (mdr1) in interpatient variation in the oral bioavailability of cyclosporine. Clin Pharmacol Ther. 1997 Sep;62(3):248–260. doi: 10.1016/S0009-9236(97)90027-8
- Haufroid V, Mourad M, Van Kerckhove V, et al. The effect of CYP3A5 and MDR1 (ABCB1) polymorphisms on cyclosporine and tacrolimus dose requirements and trough blood levels in stable renal transplant patients. Pharmacogenetics. 2004 Mar;14(3):147–154. doi: 10.1097/00008571-200403000-00002
- Press RR, Ploeger BA, den Hartigh J, et al. Explaining variability in tacrolimus pharmacokinetics to optimize early exposure in adult kidney transplant recipients. Ther Drug Monit. 2009 Apr;31(2):187–197. doi: 10.1097/FTD.0b013e31819c3d6d
- Hesselink DA, Bouamar R, Elens L, et al. The role of pharmacogenetics in the disposition of and response to tacrolimus in solid organ transplantation. Clin Pharmacokinet. 2014 Feb;53(2):123–139. doi: 10.1007/s40262-013-0120-3
- Oetting WS, Schladt DP, Guan W, et al. Genomewide Association study of tacrolimus concentrations in African American kidney transplant recipients Identifies Multiple CYP3A5 Alleles. Am J Transplant. 2016 Feb;16(2):574–582. doi: 10.1111/ajt.13495
- Bandur S, Petrasek J, Hribova P, et al. Haplotypic structure of ABCB1/MDR1 gene modifies the risk of the acute allograft rejection in renal transplant recipients. Transplantation. 2008 Nov 15;86(9):1206–1213. doi: 10.1097/TP.0b013e318187c4d1
- Kuypers DR, de Jonge H, Naesens M, et al. CYP3A5 and CYP3A4 but not MDR1 single-nucleotide polymorphisms determine long-term tacrolimus disposition and drug-related nephrotoxicity in renal recipients. Clin Pharmacol Ther. 2007 Dec;82(6):711–725. doi: 10.1038/sj.clpt.6100216
- Hesselink DA, van Schaik RH, van der Heiden IP, et al. Genetic polymorphisms of the CYP3A4, CYP3A5, and MDR-1 genes and pharmacokinetics of the calcineurin inhibitors cyclosporine and tacrolimus. Clin Pharmacol Ther. 2003 Sep;74(3):245–254. doi: 10.1016/S0009-9236(03)00168-1
- Elens L, Hesselink DA, Bouamar R, et al. Impact of POR*28 on the pharmacokinetics of tacrolimus and cyclosporine a in renal transplant patients. Ther Drug Monit. 2014 Feb;36(1):71–79. doi: 10.1097/FTD.0b013e31829da6dd
- Lunde I, Bremer S, Midtvedt K, et al. The influence of CYP3A, PPARA, and POR genetic variants on the pharmacokinetics of tacrolimus and cyclosporine in renal transplant recipients. Eur J Clin Pharmacol. 2014 Jun;70(6):685–693. doi: 10.1007/s00228-014-1656-3
- Oneda B, Crettol S, Jaquenoud Sirot E, et al. The P450 oxidoreductase genotype is associated with CYP3A activity in vivo as measured by the midazolam phenotyping test. Pharmacogenet Genomics. 2009 Nov;19(11):877–883. doi: 10.1097/FPC.0b013e32833225e7
- Zhang JJ, Zhang H, Ding XL, et al. Effect of the P450 oxidoreductase *28 polymorphism on the pharmacokinetics of tacrolimus in Chinese healthy male volunteers. Eur J Clin Pharmacol. 2013 Apr;69(4):807–812. doi: 10.1007/s00228-012-1432-1
- Passey C, Birnbaum AK, Brundage RC, et al. Dosing equation for tacrolimus using genetic variants and clinical factors. Br J Clin Pharmacol. 2011 Dec;72(6):948–957. doi: 10.1111/j.1365-2125.2011.04039.x
- Jacobson PA, Schladt D, Oetting WS, et al. Lower calcineurin inhibitor doses in older compared to younger kidney transplant recipients yield similar troughs. Am J Transplant. 2012 Dec;12(12):3326–3336. doi: 10.1111/j.1600-6143.2012.04232.x
- Fontova P, Colom H, Rigo-Bonnin R, et al. Influence of the Circadian Timing system on tacrolimus pharmacokinetics and pharmacodynamics after kidney transplantation. Front Pharmacol. 2021;12:636048. doi: 10.3389/fphar.2021.636048
- Iwahori T, Takeuchi H, Matsuno N, et al. Pharmacokinetic differences between morning and evening administration of cyclosporine and tacrolimus therapy. Transplant Proc. 2005 May;37(4):1739–1740. doi: 10.1016/j.transproceed.2005.02.104
- Park SI, Felipe CR, Pinheiro-Machado PG, et al. Circadian and time-dependent variability in tacrolimus pharmacokinetics. Fundam Clin Pharmacol. 2007 Apr;21(2):191–197. doi: 10.1111/j.1472-8206.2007.00468.x
- van Gelder T. Drug interactions with tacrolimus. Drug Saf. 2002;25(10):707–712. doi: 10.2165/00002018-200225100-00003
- Andrews LM, de Winter BC, Tang JT, et al. Overweight kidney transplant recipients are at risk of being Overdosed following standard bodyweight-based tacrolimus starting dose. Transplant Direct. 2017 Feb;3(2):e129. doi: 10.1097/TXD.0000000000000644
- Rodrigo E, de Cos MA, Sánchez B, et al. High initial blood levels of tacrolimus in overweight renal transplant recipients. Transplant Proc. 2005 Apr;37(3):1453–1454. doi: 10.1016/j.transproceed.2005.02.055
- Sawamoto K, Huong TT, Sugimoto N, et al. Mechanisms of lower maintenance dose of tacrolimus in obese patients. Drug Metab Pharmacokinet. 2014;29(4):341–347. doi: 10.2133/dmpk.DMPK-13-RG-110
- Prograf, Summary of product characteristics (European Medicines Agency) 2006 [cited 2022 Oct 12]. Available from: https://www.ema.europa.eu/en/documents/referral/prograf-article-30-referral-annex-i-ii-iii_en.pdf
- Advagraf, Summary of product characteristics (European Medicines Agency) 2009 [cit 2022 Oct 12]. Available from: https://www.ema.europa.eu/en/documents/product-information/advagraf-epar-product-information_en.pdf
- Bauer LA. Chapter 16. Tacrolimus. In: Bauer L, editor. Applied Clinical Pharmacokinetics, 2e. New York, USA: McGraw Hill; 2008. p. 682–708.
- Budde K, Bunnapradist S, Grinyo JM, et al. Novel once-daily extended-release tacrolimus (LCPT) versus twice-daily tacrolimus in de novo kidney transplants: one-year results of phase III, double-blind, randomized trial. Am J Transplant. 2014 Dec;14(12):2796–2806. doi: 10.1111/ajt.12955
- Shuker N, Bouamar R, van Schaik RH, et al. A randomized Controlled trial Comparing the efficacy of Cyp3a5 genotype-based with body-weight-based tacrolimus dosing after living donor kidney transplantation. Am J Transplant. 2016 Jul;16(7):2085–2096. doi: 10.1111/ajt.13691
- Thervet E, Loriot MA, Barbier S, et al. Optimization of initial tacrolimus dose using pharmacogenetic testing. Clin Pharmacol Ther. 2010 Jun;87(6):721–726. doi: 10.1038/clpt.2010.17
- Andrews LM, Riva N, De Winter BC, et al. Dosing algorithms for initiation of immunosuppressive drugs in solid organ transplant recipients [review]. Expert Opin Drug Metab Toxicol. 2015;11(6):921–936. doi: 10.1517/17425255.2015.1033397
- Francke MI, Andrews LM, Le HL, et al. Avoiding tacrolimus Underexposure and overexposure with a dosing algorithm for renal transplant recipients: A single Arm Prospective intervention trial. Clin Pharmacol Ther. 2021 Jul;110(1):169–178. doi: 10.1002/cpt.2163
- Alqahtani S, Alenazi M, Alsultan A, et al. Estimation of tacrolimus clearance in Saudi adult kidney transplant recipients [article]. Saudi J Kidney Dis Transpl. 2021;32(1):101–110. doi: 10.4103/1319-2442.318511
- Andreu F, Colom H, Elens L, et al. A new CYP3A5*3 and CYP3A4*22 Cluster Influencing tacrolimus target concentrations: A population approach [article]. Clin Pharmacokinet. 2017;56(8):963–975. doi: 10.1007/s40262-016-0491-3
- Andrews LM, Hesselink DA, van Schaik RHN, et al. A population pharmacokinetic model to predict the individual starting dose of tacrolimus in adult renal transplant recipients [article]. Br J Clin Pharmacol. 2019;85(3):601–615. doi: 10.1111/bcp.13838
- Ben-Fredj N, Hannachi I, Chadli Z, et al. Dosing algorithm for tacrolimus in Tunisian kidney transplant patients: Effect of CYP 3A4*1B and CYP3A4*22 polymorphisms [article]. Toxicol Appl Pharmacol. 2020;407:407. doi: 10.1016/j.taap.2020.115245
- Francke MI, Visser WJ, Severs D, et al. Body composition is associated with tacrolimus pharmacokinetics in kidney transplant recipients. Eur J Clin Pharmacol. 2022 Aug;78(8):1273–1287. doi: 10.1007/s00228-022-03323-0
- Franken LG, Francke MI, Andrews LM, et al. A population pharmacokinetic model of whole-blood and intracellular tacrolimus in kidney transplant recipients [article in Press]. Eur J Drug Metab Pharmacokinet. 2022;47(4):523–535. doi: 10.1007/s13318-022-00767-8
- Henin E, Govoni M, Cella M, et al. Therapeutic drug monitoring Strategies for Envarsus in De novo kidney transplant patients using population Modelling and simulations [article]. Adv Ther. 2021;38(10):5317–5332. doi: 10.1007/s12325-021-01905-5
- Jing Y, Kong Y, Hou X, et al. Population pharmacokinetic analysis and dosing guidelines for tacrolimus co-administration with Wuzhi capsule in Chinese renal transplant recipients [article]. J Clin Pharm Ther. 2021;46(4):1117–1128. doi: 10.1111/jcpt.13407
- Ling J, Dong LL, Yang XP, et al. Effects of CYP3A5, ABCB1 and POR*28 polymorphisms on pharmacokinetics of tacrolimus in the early period after renal transplantation [article]. Xenobiotica. 2020;50(12):1501–1509. doi: 10.1080/00498254.2020.1774682
- Reséndiz-Galván JE, Medellín-Garibay SE, Milán-Segovia RDC, et al. Dosing recommendations based on population pharmacokinetics of tacrolimus in Mexican adult patients with kidney transplant [article]. Basic Clin Pharmacol Toxicol. 2019;124(3):303–311. doi: 10.1111/bcpt.13138
- Sanghavi K, Brundage RC, Miller MB, et al. Genotype-guided tacrolimus dosing in African-American kidney transplant recipients. Pharmacogenomics J. 2017 Jan;17(1):61–68. doi: 10.1038/tpj.2015.87
- Woillard JB, Mourad M, Neely M, et al. Tacrolimus updated guidelines through popPK modeling: How to benefit more from CYP3A pre-emptive genotyping prior to kidney transplantation [article]. Front Pharmacol. 2017;8(JUN). doi: 10.3389/fphar.2017.00358
- Campagne O, Mager DE, Brazeau D, et al. Tacrolimus population pharmacokinetics and Multiple CYP3A5 genotypes in black and white renal transplant recipients [article]. J Clin Pharmacol. 2018;58(9):1184–1195. doi: 10.1002/jcph.1118
- Rong Y, Mayo P, Ensom MHH, et al. Population pharmacokinetic analysis of immediate-release oral tacrolimus co-administered with mycophenolate mofetil in corticosteroid-free adult kidney transplant recipients [article]. Eur J Drug Metab Pharmacokinet. 2019;44(3):409–422. doi: 10.1007/s13318-018-0525-3
- Vadcharavivad S, Praisuwan S, Techawathanawanna N, et al. Population pharmacokinetics of tacrolimus in Thai kidney transplant patients: comparison with similar data from other populations [article]. J Clin Pharm Ther. 2016;41(3):310–328. doi: 10.1111/jcpt.12396
- Zhang HJ, Li DY, Zhu HJ, et al. Tacrolimus population pharmacokinetics according to CYP3A5 genotype and clinical factors in Chinese adult kidney transplant recipients [article]. J Clin Pharm Ther. 2017;42(4):425–432. doi: 10.1111/jcpt.12523
- Zhang SF, Tang BH, Wei AH, et al. Effect of drug combination on tacrolimus target dose in renal transplant patients with different CYP3A5 genotypes. Xenobiotica. 2022 Apr 20;52:1–10. doi: 10.1080/00498254.2022.2064252
- Zhu W, Xue L, Peng H, et al. Tacrolimus population pharmacokinetic models according to CYP3A5/CYP3A4/POR genotypes in Chinese han renal transplant patients [article]. Pharmacogenomics. 2018;19(13):1013–1025. doi: 10.2217/pgs-2017-0139
- Størset E, Åsberg A, Skauby M, et al. Improved tacrolimus target concentration achievement using computerized dosing in renal transplant recipients-a prospective, randomized study [article]. Transplantation. 2015;99(10):2158–2166. doi: 10.1097/TP.0000000000000708
- Francke MI, Hesselink DA, Andrews LM, et al. Model-based tacrolimus follow-up dosing in adult renal transplant recipients: a simulation trial [article in Press]. Ther Drug Monit. 2022;44(5):606–614. doi: 10.1097/FTD.0000000000000979
- Udomkarnjananun S, Francke MI, Dieterich M, et al. P-glycoprotein, FK-binding protein-12, and the intracellular tacrolimus concentration in T-lymphocytes and monocytes of kidney transplant recipients. Transplantation. 2022 Sep 2;107:382–391. doi: 10.1097/TP.0000000000004287
- Kuypers DR, Claes K, Evenepoel P, et al. Time-related clinical determinants of long-term tacrolimus pharmacokinetics in combination therapy with mycophenolic acid and corticosteroids: a prospective study in one hundred de novo renal transplant recipients. Clin Pharmacokinet. 2004;43(11):741–762. doi: 10.2165/00003088-200443110-00005
- Hesselink DA, Ngyuen H, Wabbijn M, et al. Tacrolimus dose requirement in renal transplant recipients is significantly higher when used in combination with corticosteroids. Br J Clin Pharmacol. 2003 Sep;56(3):327–330. doi: 10.1046/j.0306-5251.2003.01882.x
- Mulasi U, Kuchnia AJ, Cole AJ, et al. Bioimpedance at the bedside: current applications, limitations, and opportunities. Nutr Clin Pract. 2015 Apr;30(2):180–193. doi: 10.1177/0884533614568155
- Huang S, Zhang D, Li Y, et al. Schisandra sphenanthera: a comprehensive review of its botany, phytochemistry, pharmacology, and clinical applications. Am J Chin Med. 2021;49(7):1577–1622. doi: 10.1142/S0192415X21500749
- Åsberg A, Midtvedt K, Van Guilder M, et al. Inclusion of CYP3A5 genotyping in a nonparametric population model improves dosing of tacrolimus early after transplantation [article]. Transplant Int. 2013;26(12):1198–1207. doi: 10.1111/tri.12194
- Andrews LM, Hesselink DA, van Gelder T, et al. A population pharmacokinetic model to predict the individual starting dose of tacrolimus following pediatric renal transplantation [article]. Clin Pharmacokinet. 2018;57(4):475–489. doi: 10.1007/s40262-017-0567-8
- Andrews LM, de Winter BCM, Cornelissen EAM, et al. A population pharmacokinetic model does not predict the optimal starting dose of tacrolimus in pediatric renal transplant recipients in a Prospective study: lessons learned and model Improvement [article]. Clin Pharmacokinet. 2020;59(5):591–603. doi: 10.1007/s40262-019-00831-8
- Jacobo-Cabral CO, García-Roca P, Romero-Tejeda EM, et al. Population pharmacokinetic analysis of tacrolimus in Mexican paediatric renal transplant patients: role of CYP3A5 genotype and formulation [article]. Br J Clin Pharmacol. 2015;80(4):630–641. doi: 10.1111/bcp.12649
- Prytuła AA, Cransberg K, Bouts AHM, et al. The effect of weight and CYP3A5 genotype on the population pharmacokinetics of tacrolimus in stable paediatric renal transplant recipients [article]. Clin Pharmacokinet. 2016;55(9):1129–1143. doi: 10.1007/s40262-016-0390-7
- Min S, Papaz T, Lafreniere-Roula M, et al. A randomized clinical trial of age and genotype-guided tacrolimus dosing after pediatric solid organ transplantation. Pediatr Transplant. 2018 Nov;22(7):e13285. doi: 10.1111/petr.13285
- de Wildt SN, van Schaik RH, Soldin OP, et al. The interactions of age, genetics, and disease severity on tacrolimus dosing requirements after pediatric kidney and liver transplantation. Eur J Clin Pharmacol. 2011 Dec;67(12):1231–1241. doi: 10.1007/s00228-011-1083-7
- Kausman JY, Patel B, Marks SD. Standard dosing of tacrolimus leads to overexposure in pediatric renal transplantation recipients. Pediatr Transplant. 2008 May;12(3):329–335. doi: 10.1111/j.1399-3046.2007.00821.x
- Zhao W, Elie V, Roussey G, et al. Population pharmacokinetics and pharmacogenetics of tacrolimus in de novo pediatric kidney transplant recipients [article]. Clin Pharmacol Ther. 2009;86(6):609–618. doi: 10.1038/clpt.2009.210
- Abu-Elmagd K, Fung JJ, Alessiani M, et al. The effect of graft function on FK506 plasma levels, dosages, and renal function, with particular reference to the liver. Transplantation. 1991 Jul;52(1):71–77. doi: 10.1097/00007890-199107000-00015
- Holford N, Ma G, Metz D. TDM is dead. Brit J Clinical Pharma. 2022 Feb;88(4):1406–1413. doi: 10.1111/bcp.14434
- van Gelder T, Meziyerh S, Swen JJ, et al. The clinical Impact of the C(0)/D ratio and the CYP3A5 genotype on outcome in tacrolimus treated kidney transplant recipients. Front Pharmacol. 2020;11:1142. doi: 10.3389/fphar.2020.01142
- Verhoeven J, Boer K, Peeters AMA, et al. A novel high-throughput droplet digital PCR-based indel quantification method for the detection of circulating donor-derived cell-free DNA after kidney transplantation. Transplantation. 2022 Sep 1;106(9):1777–1786. doi: 10.1097/TP.0000000000004078
- Emoto C, Johnson TN, Hahn D, et al. A theoretical physiologically-based pharmacokinetic approach to ascertain covariates explaining the large interpatient variability in tacrolimus disposition [article]. CPT Pharmacometrics Syst Pharmacol. 2019;8(5):273–284. doi: 10.1002/psp4.12392
- Cai N, Zhang X, Zheng C, et al. A novel random forest integrative approach based on endogenous CYP3A4 phenotype for predicting tacrolimus concentrations and dosages in Chinese renal transplant patients [article]. J Clin Pharm Ther. 2020;45(2):318–323. doi: 10.1111/jcpt.13074
- Fu Q, Jing Y, Liu Mr G, et al. Machine learning-based method for tacrolimus dose predictions in Chinese kidney transplant perioperative patients [article in Press]. J Clin Pharm Ther. 2021;47:600–608. doi: 10.1111/jcpt.13579
- Tang J, Liu R, Zhang YL, et al. Application of machine-learning models to predict tacrolimus stable dose in renal transplant recipients [article]. Sci Rep. 2017;7(1):42192. doi: 10.1038/srep42192
- ImmunoSuppressants Bayesian dose Adjustment (ISBA) 2005 [cited 2022 Oct 12]. Available from: https://pharmaco.chu-limoges.fr/