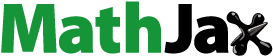
ABSTRACT
Understanding the drivers of forest transitions is relevant to inform effective forest conservation. We investigate pathways of forest transitions in the United States (1920–2010), France (1850–2010), and Austria (1830–2010). By combining evidence from forest inventories with the forest model CRAFT, we first quantify how change in forest area (ΔA), maximum biomass density (ΔBdmax), and actual biomass as fraction of maximum biomass (ΔFmax) shaped forest dynamics. Second, to investigate the connections between forest change and societal resource use, or social metabolism, we quantify the importance of selected proximate and underlying socio-metabolic drivers. We find that agricultural intensification and reduced forest grazing correlated most with positive ΔA and ΔBdmax. By contrast, change in biomass imports or harvest did not explain forest change. Our findings highlight the importance of forest growth conditions in explaining long-term forest dynamics, and demonstrate the distinct ways in which resource use drove forest change.
Introduction
Forests play crucial roles for mitigating climate change: as carbon-rich ecosystems, the conservation and recovery of forests can sequester substantial amounts of carbon that would otherwise accumulate in the atmosphere (Bastin et al., Citation2019; IPCC, Citation2019). At the same time, in many industrialized countries of the Global North, the increased use of forest products is also considered as a major pillar in sustainable resource use strategies to substitute for fossil-energy based products and services (e.g. European Commission, Citation2018), despite the possible negative side-effects of intensive forestry on biomass carbon stocks (Erb et al., Citation2018; Law et al., Citation2018; Yan, Citation2018). Both the conservation of forests and the increased use of forest products are promoted as viable climate-change mitigation strategies. Understanding how forest change intersects with resource use, both within the forest sector and outside of it, and which pathways of forest use are compatible with ecological sustainability aims, is therefore an important research frontier (Di Sacco et al., Citation2021; Scheidel & Gingrich, Citation2020). While deforestation has abrupt consequences, forest growth reacts slowly to changes in management and environmental conditions (San Roman Sanz et al., Citation2013; Thom et al., Citation2018). A comprehensive understanding about the interlinkages between long-term dynamics of forest change and resource use is however lacking to date.
The analysis of forest transitions offers a unique entry point. Forest transitions mark the long-term shift from deforestation or forest degradation to reforestation or forest recovery, and occur over decadal or centennial time periods and at national or larger landscape scales (Mather, Citation1992; Meyfroidt & Lambin, Citation2011). While global deforestation is an ongoing trend, mostly caused by tropical deforestation, forest transitions in many temperate and boreal countries of the Global North, as well as increasingly in tropical countries of the Global South are currently contributing to a decline in global deforestation rates (FAO, Citation2020; Tubiello et al., Citation2021). In addition to stable or increasing forest areas, forest ecosystems in large world regions including North America, Europe, and Eastern Asia, have also experienced significant vegetation thickening in recent decades (Köhl et al., Citation2015; Le Noë et al., Citation2021a).
Despite these tentatively encouraging trends of recent global forest change, research has also pointed towards underlying links between forest transitions and shifts towards unsustainable patterns of societal material and energy use, or social metabolism (Haberl et al., Citation2019), that may counteract the ecological benefits of forest transitions (Gingrich et al., Citation2019; Scheidel, Citation2019). Such problem shifts include the spatial displacement of deforestation through increased imports of biomass from deforesting countries, mostly in the Global South, to reforesting countries, many of which are located in the Global North (Henders et al., Citation2015; Kastner et al., Citation2011; Pendrill et al., Citation2019). Additionally, the intensification and concentration of domestic agriculture increases domestic agricultural production while freeing land for forest recovery (García et al., Citation2020; Jadin et al., Citation2016), but also comes at ecological costs including increased greenhouse gas emissions from agriculture (Garnier et al., Citation2019; Hong et al., Citation2021; Tubiello et al., Citation2013) as well as social costs such as the exclusion of rural populations from multiple forest uses (Larrère & Nougarède, Citation1990; Pichler et al., Citation2021a). Finally, in early industrialized countries of the Global North, the shift towards coal was an important factor relieving forests from providing woodfuel, allowing forest recovery while enabling higher energy use and causing additional emissions (Gingrich et al., Citation2021; Magerl et al., Citation2022; Myllyntaus & Mattila, Citation2002).
A sound understanding of how specific pathways of forest transitions have been linked to trends in social metabolism in the past is an urgent prerequisite for shaping future forest transitions that avoid undesirable problem shifts. However, analyses investigating how forest transitions are connected to changes in overall societal resource use have been conducted only for individual case studies (e.g. Gingrich et al., Citation2021; Jadin et al., Citation2016; Le Noë et al., Citation2021b), while comparative or global-scale analyses have either been restricted to forest change, without addressing changes in resource use (Kauppi et al., Citation2006; Southworth et al., Citation2012), have analysed the impact of individual drivers on forest change, such as agricultural intensification (García et al., Citation2020) or trade (Pendrill et al., Citation2019), or have focused on the economic or political dimensions of resource use (García et al., Citation2021; Liu et al., Citation2017; Mansfield et al., Citation2010; Roy Chowdhury & Moran, Citation2012; Youn et al., Citation2016), rather than their biophysical ones (Ashraf et al., Citation2017). Finally, to our knowledge, no previous work has investigated the effects of resource use on forest change beyond forest area and carbon density.
To close this research gap, we conduct a comparative analysis of long-term forest change in the course of forest transitions and its connection to social metabolism for three early-industrialized countries of the Global North, i.e. the United States, France, and Austria, covering the respective time periods since the beginning of forest expansion, i.e. 90, 160 and 180 years respectively. The long-term perspective, combining approaches from land system science (Verburg et al., Citation2015) and social ecology (Haberl et al., Citation2016), enables us to tackle long-term temporal dynamics in forest change (Aspinall et al., Citation2021; Tappeiner et al., Citation2021) and their connections to changes in social metabolism. Our analysis comprises an investigation of national-level processes of forest biomass carbon dynamics and an analysis of the links between specific forest change processes and selected changes in social metabolism. We discuss our findings in view of forest transitions research and the challenges of integrating forest conservation with sustainable resource use today.
Materials and methods
This analysis builds on a number of datasets on long-term forest change and changes in social metabolism in the United States, France and Austria and employs a decomposition analysis (Ang & Zhang, Citation2000) to quantify how forest carbon dynamics were affected by three underlying processes of forest change, as well as simple and multiple regression analyses to investigate how specific changes in social metabolism (‘socio-metabolic drivers’) were connected to these dynamics at national levels.
The three countries are all industrialized countries of the Global North that experienced forest transitions in the 19th (France and Austria) and early 20th centuries (United States) (Gingrich et al., Citation2007; Magerl et al., Citation2019; Le Noë et al., Citation2020). They make promising cases for comparison due to their important differences in terms of (1) their land-use histories and (2) their historical roles in international trade networks: (1) In contrast to France and Austria, the United States experienced strong agricultural expansion in the late 19th century in the context of White European settlement (Houghton & Hackler, Citation2000). (2) While Austria as a land-locked country and part of the Habsburg Monarchy was not a major trading partner in global markets in the 19th century (Komlos, Citation1983), France and the United States have strong historical roots in colonial trade networks (Krausmann & Langthaler, Citation2019) and are major players in global agricultural trade today (Kastner et al., Citation2021).
Quantifying forest C dynamics
We make use of long-term datasets on forest area, forest biomass density, and wood harvest to consistently quantify forest carbon dynamics in the conterminous United States (1920–2010), France (1850–2010), and Austria (1830–2010), as described in ().
Table 1. References to data sources of forest change and major rationales of estimation procedures
We use these data to calibrate the CRAFT model, which enables us to isolate the effects of specific forest change processes. The parsimonious forest growth model CRAFT, originally developed for the case of France (Le Noë et al., Citation2020), is based on an equation linking annual per-area Net Primary Production (NPP/A) to an apparent growth rate (r) which may change over time (annual change factor α), the biomass density including above- and belowground biomass (Bd), and the hypothetical per-area carrying capacity in the absence of mortality (K/A), as follows:
Biomass stocks in a succeeding year (Bt+1) are then quantified as the sum of biomass stocks in the previous year (Bt) and net primary productivity (NPPt), minus wood extraction (ht), including wood harvest as reported in statistics (see ) and felling losses of 11% (Liski et al., Citation2002), minus a country-specific mortality rate (m, i.e. 0.0565 for the United States, 0.0667 for France and 0.0519 for Austria, derived from Erb et al. (Citation2018)), adjusted for change in forest area (At,At+1):
For France, where a previous optimization is available for the entire time series (Le Noë et al., Citation2020), we modified some input data (country-specific mortality rate) and model assumptions (aggregate biomass, rather than split into deciduous and coniferous), resulting in slightly different results than the previous assessment. For the United States and Austria, where long-term model calibration had not yet been performed, we combined national information on forest area, biomass density and wood extraction with national forest yield tables (Marschall, Citation1975; Stage et al., Citation1988). For the United States, where forest mortality varied greatly due to forest fires in the late 19th and early 20th centuries (Houghton et al., Citation2000), we assumed that the generic mortality factor underestimates historical fire impacts and thus additionally considered burnt biomass in the calculation of biomass stock differences in succeeding years. To quantify burnt biomass, we multiplied annual data on burnt forest area derived from national forest fire statistics released by the United States Forest Service (“Forest Fire Statistics“ 1941–1964, “Annual Fire report for the National Forests“ 1960–1969 and “National Forest Fire Report“ 1971–1985) and from the literature (Hawbaker et al., Citation2020) by fuel loads, i.e. the fraction of total forest biomass susceptible to burning, and combustion completeness factors, i.e. the fraction of total fuel load that actually burns during a wildfire event (Urbanski et al., Citation2018; Yang et al., Citation2014).
We considered the relative contribution of deciduous and coniferous trees in each country as reported in statistics to establish plausible ranges for r and K values. Per country, we then first optimized K (one static value) and r values at the starting point of the respective time series, as well as in 1960 and 2010, assuming linear change in these two periods, to best reproduce the reported biomass data. In the United States and Austria, r could then be further optimized at decadal resolution over the whole period, resulting in dynamic change values α, while in France, due to reduced availability of observation data on biomass density, r was optimized at decadal resolution only from 1960 to 2010, resulting in a constant change value α for 1850–1960. We thus generated year- and country-specific r values, reproducing the biomass stock values of the forest inventory data with relative root mean square error values of 2%, 1% and 0.2% respectively for the United States, France and Austria.
Decomposition analysis of forest change processes
We conducted a decomposition analysis (Ang & Zhang, Citation2000) to identify the relative contribution to forest biomass dynamics of three specific forest change processes. Decomposition analyses attribute change in one variable (forest biomass stocks) to the sum of changes in several underlying variables by means of a mathematical formula called “identity“, and have been successfully applied to understand the processes underlying long-term land-use dynamics (Gingrich et al., Citation2015; Hong et al., Citation2021; Le Noë et al., Citation2020). We advanced the “forest identity“ (Kauppi et al., Citation2006; Köhl et al., Citation2015), a formula previously applied to decompose forest biomass stock change (ΔB) into the effects of changes in forest area (ΔA) and forest biomass density (ΔB/A), by distinguishing two different factors contributing to changes in forest biomass density. Based on the national long-term optimization of the CRAFT model, we quantified, for each country and each year, the variable Bmax.Bmax corresponds to the maximum biomass density in the absence of harvest at which NPP equals the mortality (m) at a given time- and country-specific value of r (the growth rate parameter). In contrast to K, the hypothetical biomass density in the absence of mortality described above, or the “potential biomass“, another commonly used ecological variable denoting the climax vegetation in the absence of land use (Erb et al., Citation2018), Bmax in a given year (Bmax,t) reflects the maximum biomass stock under the contemporary environmental and management conditions, and can be written as:
This indicator enabled us to advance the forest identity towards:
With Bdmax the maximum biomass density and Fmax the actual biomass as fraction of Bmax. An additive decomposition analysis using the Logarithmic Media Divisia Index (LMDI) proposed by Ang (Citation2005) was then conducted to quantify the contribution of ΔA, ΔBdmax, and ΔFmax to ΔB in each country and at decadal intervals:
Through our decomposition analysis, we were thus able to attribute national long-term change in forest biomass stocks (ΔB) to three forest change processes, i.e. change in forest area (ΔA), change in maximum forest biomass density, caused either by changes in management or environmental conditions (ΔBdmax), and change in the actual forest biomass as fraction of maximum biomass (ΔFmax).
Linking forest change to social metabolism
We also investigated how these dynamics of forest change were connected to changes in social metabolism, i.e. the annual socio-economic throughput of material and energetic resources (Haberl et al., Citation2019; Pauliuk & Hertwich, Citation2015). To this end, we developed a generalized analytical framework () based on conceptual considerations on proximate and underlying drivers of forest change (Geist & Lambin, Citation2002) and the specific role of social metabolism and its links to forest transitions (Gingrich et al., Citation2019). We use this framework as a tool to investigate how trends in specific consistent socio-metabolic indicators based on Material and Energy Flow Accounting (Haberl et al., Citation2004) are connected to specific forest change processes, either by physically impacting forest dynamics (i.e. as proximate drivers) or by acting upon change in the proximate drivers (i.e. as underlying drivers). Given that the annual throughput of material and energy sources increased in the three case studies investigated during the period of the forest transition (Gierlinger & Krausmann, Citation2012; Gingrich et al., Citation2016; Magalhães et al., Citation2019), we expect shifts in social metabolism relieving pressures from forests while enabling increasing resource use.
Figure 1. Analytical framework of socio-metabolic drivers of forest transitions (ΔB: change in forest biomass, ΔA: change in forest area, ΔBdmax: change in maximum forest biomass density at contemporary management and environmental conditions, ΔF: actual biomass as fraction of potential). Non-coloured boxes are not explicitly analysed in this study.
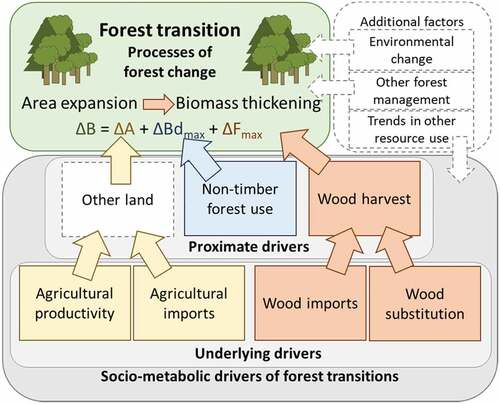
Further, biophysical factors contributing to forest change are only implicitly considered in the optimization of the α and r parameters in the CRAFT model, but are not quantitatively analysed in our study. Such processes include the selection of tree species or genotypes (e.g. Resende et al., Citation2012), the protection of seedlings (Keeton, Citation2008), and some effects of environmental change, such as increased temperature, change in precipitation or N fertilization (Hickler et al., Citation2008; Rossi et al., Citation2008). Beyond the framework condition of generally increasing resource use, specific national-level trends in resource use, e.g. as mediated by population growth, resource use efficiency or technological change (Martinico-Perez et al., Citation2017; Vilaysouk et al., Citation2017), or as consequences of singular historical events (Krausmann et al., Citation2016; Kuskova et al., Citation2008), are only considered implicitly in some of the proxy indicators for underlying drivers, which are expressed as % of domestic consumption, see ().
Table 2. Indicators used to quantify the socio-metabolic drivers of forest transitions in this study
We linked each process of forest change defined in our forest identity to specific socio-metabolic enabling conditions, describing proximate or underlying drivers of forest change (). Forest area expansion (positive ΔA) on a constant land area results in less other land. As proxy for other land, our analysis focuses on agricultural land, the dominant other land use in all our case studies. Under constant or growing demand for agricultural products, reductions in agricultural land can be enabled either by higher productivity of agricultural areas through intensification and/or spatial contraction to the most favourable areas (García et al., Citation2020), and/or by increasing agricultural imports, displacing agricultural production outside the domestic land area (Jadin et al., Citation2016). We quantify agricultural productivity as agricultural biomass extraction (applying the socio-metabolic indicator Domestic Extraction) per unit of agricultural land area. It describes the total amount of vegetal biomass entering socio-economic systems, i.e. the sum of harvest of primary and secondary products from cropland, and biomass harvested or grazed from grassland per unit of total agricultural land.
To quantify agricultural imports, we use as proxy the physical trade balance (PTB, imports minus exports) of agricultural products as a fraction of the respective Domestic Consumption of agricultural products, termed ‘agricultural import dependence’. This socio-metabolic indicator (e.g. Dorninger & Eisenmenger, Citation2016) enables us to identify to which extent a country relies on biomass imports to feed its livestock and population. As a highly aggregate indicator adding both vegetal and animal products, the physical trade balance does not inform about land displacement for agricultural production (e.g. the land required elsewhere to produce a certain amount of beef imported). To overcome this limitation, different methodological approaches would be required (Schaffartzik et al., Citation2015) which are, however, beyond the scope of this study.
We investigate changes in forest grazing as proxies for non-timber forest uses, potentially affecting changes in maximum biomass density (ΔBdmax), because forest grazing represents the major traditional agricultural side use of forests in industrialized countries of the Global North (Borman, Citation2005; Gimmi & Buergi, Citation2007). Forest grazing can, next to other forest management and environmental change, affect forest degradation or recovery either immediately or with a delay (Niedertscheider et al., Citation2017).
Finally, changes in forest biomass as a fraction of maximum biomass (ΔFmax) are the effect of dynamics in forest age (affecting the counter, B) and forest growth conditions (affecting the denominator, Bmax). The major socio-metabolic process impacting forest age is wood harvest, which we quantify as domestic extraction of wood per unit of forest area. In addition, also forest area expansion (positive ΔA) results in rejuvenation of the forest at the country-level. We investigate two additional underlying socio-metabolic drivers potentially affecting wood harvest: (1) We quantify wood imports, displacing the harvest pressure outside the domestic territory (Pendrill et al., Citation2019), as fraction of imports in domestic consumption of wood (‘wood import dependence’). (2) To assess the substitution of wood in major socioeconomic processes, we quantify the fraction of woodfuel in domestic energy consumption (‘woodfuel importance’), representing the shift from wood to coal in the context of forest transitions in the Global North (Magerl et al., Citation2022; Myllyntaus & Mattila, Citation2002).
Regression analysis
We apply multiple and simple linear regressions using the Ordinary Least Squares (OLS) model at country level, covering the respective country-level time periods, or the longest possible time periods for which the respective data were available (). Regression analyses are commonly conducted to investigate to which extent independent variables affect change in dependent variables in the context of land-use change (Meyfroidt, Citation2016). We quantify the explanatory effect of the socio-metabolic proximate and underlying drivers (independent variables) on the respective forest change processes (dependent variables): (i) agricultural intensity and agricultural imports on forest area (A); (ii) forest grazing on maximum biomass density (Bdmax); and (iii) wood harvest, wood import dependence and woodfuel importance on the actual biomass as a fraction of maximum biomass (Fmax). For (i), we apply multiple regressions, for (ii) single regressions, and for (iii) both (simple for wood harvest, multiple for wood import dependence and woodfuel importance). After testing for multicollinearity between independent variables, we examine the coefficients of determination (R2), informing about how accurately the independent variables predict the respective dependent variables, as well as the respective p-values to test for their statistical significance. We also analyse the regression coefficients of the independent variables, quantifying the direction and magnitude of change in the dependent variables effected by change in the independent variables.
Results
Three forest transitions result from different combinations of forest change processes
Due to their different histories and geographies, we expected the forest transitions in the United States, France and Austria to follow diverging trends. Across the respective time series, biomass carbon stocks increased by factors of 1.8, 2.3 and 2.3 (corresponding to average annual growth rates of 0.666%/yr, 0.471%/yr and 0.470%/yr) in the United States, France and Austria, respectively. The most pronounced biomass increase occurred in the most recent period in all countries ()), reaching values above 1%/yr in the United States and France in the early 21st century, and just below 1 in Austria in the late 20th century. While in the United States, forest areas stabilized at around 33% of the total land area since the early 20th century, in France forest areas increased from 15% in the mid-19th century to 33% of total land area in 2015, and in Austria from 40% in 1830 to almost 50% of total land area in 2010 ()). Biomass density ()) increased in all countries across the time period, was consistently highest in Austria (c.60 tCha−1 in 1830 and 110 tCha−1 in 2010), and ranged between 40 and 69 tCha−1 in the United States and France, with a more pronounced recent increase in the United States. In line with previous research on the three countries, we identify that the respective forest transitions were dominated by forest thickening in the United States (Magerl et al., Citation2019; Ramankutty et al., Citation2010), by forest area expansion in France (Le Noë et al., Citation2020), and the combined effect of both in Austria (Gingrich et al., Citation2007; Tasser et al., Citation2007).
Figure 2. Forest transitions in the United States (US), France (Fr) and Austria (At): (a) forest biomass stocks, (b) forest area (a), (c) forest biomass density (Bd), (d) maximum forest biomass density under contemporary management (Bdmax).
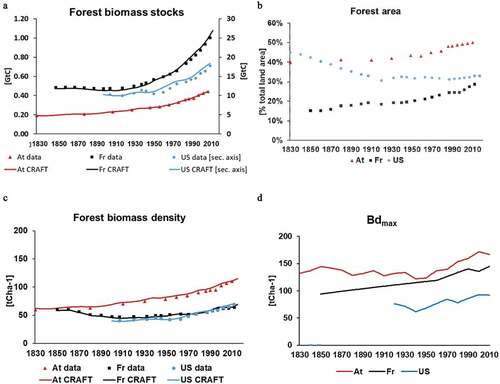
Bdmax, the newly established indicator describing the maximum density stock under contemporary environmental and management conditions ()), was highest in Austria, and consistently lowest in the United States, owing to the high share of low biomass-density shrubland (e.g. chaparral, sagebrush) in the Western United States (Magerl et al., Citation2019). In all three countries, Bmax increased in recent decades, probably as the combined effect of several factors, including improved forest management, spatial reconfiguration of forest landscapes into more productive regions and vegetation greening promoted by changing environmental conditions, mostly due to CO2 fertilization (Zhu et al., Citation2016).
The decomposition analysis, quantifying the relative contribution of these processes () reveals that forest area change (ΔA) had a positive effect on the forest transition in all countries ()), increasing biomass stocks by 9% (808 MtC) in the United States, 41% (78MtC) in Austria and 97% (458MtC) in France throughout the respective time period. This was an effect of consistent forest area expansion in France ()) and Austria ()), while area expansion in the United States was less pronounced ()) and interrupted by three decades of modest deforestation in 1960–1990 ()).
Figure 3. Processes of forest change investigated in a decomposition analysis in MtC at decadal resolution in the United States (a), France (b), Austria (c), and cumulatively as % of the biomass stock in year 1 of the respective time period (B1) (d).
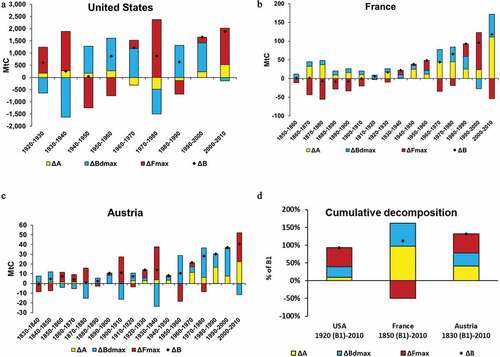
The effects of ΔBdmax and ΔFmax were more variable between countries and over time. In many cases, but not systematically, increasing ΔBdmax was partly counteracted by ΔFmax, or vice versa. These counteracting effects are particularly pronounced in the United States and Austria, where growth conditions were optimized at decadal resolution throughout the time periods of investigation. Across case studies, in the more recent decades, ΔBdmax contributed positively to the increase in biomass stocks. The cumulative effect of ΔBdmax was positive across case studies ()), contributing to increases in biomass stocks by 30% (4,660 MtC), 64% (303 MtC) and 37% (70 MtC) in the United States, France and Austria respectively, across the corresponding time periods of analysis. By contrast, change in the actual biomass as a fraction of maximum biomass (ΔFmax) was the strongest positive contributor in the United States (54% or 4,660 MtC) and Austria (54% or 103 MtC), but negative (−49% or −232 MtC) in France. This result indicates an overall ageing of forests in the United States and Austria, but a rejuvenation of forests in France, in line with the pronounced forest area expansion in this country.
Our results highlight that forest transitions in general ()), and the increase in forest biomass density in particular ()) may be the effect of variable underlying processes of forest change ()).
Socio-metabolic drivers of forest transitions
Based on our analytical framework () and the country-wide decomposition analyses (), we would expect to find specific trends in socio-metabolic drivers of forest transitions, provided that (a) the socio-metabolic drivers are dominant over drivers not considered (e.g. other forest management and environmental conditions), (b) they affect forests immediately or soon, i.e. without delay beyond our time frame of analysis, and (c) if delays between socio-metabolic drivers and forest change do occur, no processes prior to the time periods of investigation overshadow the interactions described in the period.
The regression analyses () reveal that the forest change processes could be predicted by changes in socio-metabolic drivers at varying levels of accuracy across countries. In each country, at least one socio-metabolic driver resulted in an R2 value of 0.69 or above and a p value below 0.01, indicating significant prediction of more than 70% of the change in the respective dependent variable.
Table 3. Linear regression results for the country-level processes of forest change (rows: Bdmax: maximum forest biomass density under contemporary management and environmental conditions; Fmax: actual biomass as a fraction of maximum biomass) and the socio-metabolic drivers in the respective countries (columns): regression coefficient per independent variable, and adjusted coefficient of determination (R2) per regression model. Levels of significance are indicated by asterisks: * 0.01 < p < 0.05; ** p < 0.01. a Regression was performed for 1878–2010 due to data availability; b Regression was performed for 1920–2010 due to data availability
Forest area (ΔA) expanded in all three countries, therefore agricultural productivity and/or agricultural import dependence are expected to increase particularly in Austria and France, where forest expansion was most pronounced and most persistent. The adjusted R2 values for the regressions explaining forest area change as a function of agricultural productivity and agricultural import dependence were highest in France (0.90) and Austria (0.81), where forests expanded more, but low (0.21) in the United States. We found strong collinearity of agricultural intensity and agricultural trade in the United States.
Agricultural productivity increased in all countries ()), resulting in significant, but variable regression coefficients. The lowest regression coefficient (0.05) is observed in France where high rates of agricultural intensification coincided with consistent forest expansion. Here, a combination of technological improvements most effective after World War II, political interventions such as the Common Agricultural Policy implemented as early as 1962, and favourable environmental conditions (specifically the lowland silty-loamy soils of Northern France) enabled rapid agricultural productivity increase (Duby & Wallon, Citation1976; Servolin, Citation1985). In Austria, despite explicit technological and political efforts to enhance productivity, increases in agricultural productivity were less pronounced (Gingrich et al., Citation2015; Krausmann et al., Citation2003), but agricultural productivity displays a higher regression coefficient (0.37) than in France, indicating that one unit of agricultural intensification was connected to larger forest expansion in Austria. In the United States, agricultural productivity substantially increased in the 20th century too, interrupted only during the 1930s and 1940s, due to events such as the ‘Dust Bowl’ and the ‘Great Depression’ coinciding with agricultural expansion (Cunfer et al., Citation2018; Gierlinger & Krausmann, Citation2012; Hornbeck, Citation2012).
Figure 4. Socio-metabolic drivers of forest area change (a) and change in maximum forest biomass density (Bdmax) (a) agricultural productivity: Domestic Extraction of agricultural biomass per unit of agricultural area; (b) agricultural import dependence: physical trade balance as fraction of domestic consumption of agricultural products (negative values indicate net exports); (c) forest grazing as domestic extraction of grazed biomass from forest per unit of forest land.
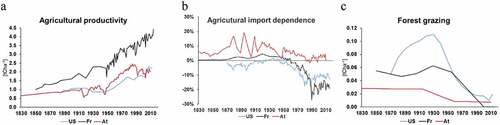
Aggregate agricultural import dependence did not increase in any of the case studies: while in the 19th century, both Austria and France were net importers of agricultural biomass, import dependence declined in Austria in the second half of the 20th century, and the United States and France became major net exporters of agricultural products in this period ()). The physical trade balances alone obscure that as part of an increasingly globalized market of agricultural products, the three countries also imported significant amounts of agricultural products, impacting remote regions of production (Kastner et al., Citation2014; Roux et al., Citation2021). In France and Austria, regression coefficients of agricultural import dependence are even negative, indicating that contrary to our expectations, across the time periods investigated, decreases in agricultural import dependence coincided with growing forest areas.
Forest grazing, the only socio-metabolic driver we linked to Bdmax, declined in all case studies, with the most pronounced dynamic in the mid-20th century, and the least total intensity in Austria ()). Despite the limited data robustness of this indicator, change in forest grazing has a high R2 in the United States (0.69) and France (0.67), and a low R2 only in Austria (0.28), where the intensity and temporal dynamic of forest grazing was least pronounced. In France and the United States, decreasing forest grazing thus emerges as an important driver of improved forest growth conditions.
Finally, Fmax, the actual forest biomass as a fraction of the maximum biomass, may, according to our analytical framework, be influenced by trends in wood harvest or forest area expansion, which resulted in rejuvenation in France ()), as well as by additional factors which we did not account for, such as other forest management or changing environmental conditions. Wood harvest emerged as a surprisingly poor predictor of Fmax, with adjusted R2 values in the simple linear regression ranging between 0.02 (France) and 0.23 (United States, ). Wood harvest declined slightly but not consistently only in France, and the increase of wood harvest in the United States ()) resulted in a negative regression coefficient, indicating that counterintuitively, higher wood harvest coincided with higher biomass stocks across the time period. In Austria, where biomass density was higher than in the other two countries ()), wood harvest was highest throughout the period. This difference may be connected to environmental conditions of forest growth allowing for high forest in most of the Austrian territory, while less stocked forests like garrigue and chaparral cover large fractions of total forest area in France and the United States, respectively. Additionally, in the United States 2% (1900) to 7% (2010) of the total forest area are ‘reserved’ forests not used for timber harvest (Oswalt et al., Citation2014). The unexpected decoupling of ΔFmax from harvest, though supported only by low adjusted R2 values, could arise from previous severe degradation due to forest over-use, e.g. through forest grazing or litter extraction before the period under investigation. This is demonstrated both by the long-term increase in maximum biomass density in all three countries in this study, and by the large distance from potential biomass stocks found in previous work (Gingrich et al., Citation2007; Magerl et al., Citation2019).
Figure 5. Socio-metabolic drivers of actual forest biomass as fraction of maximum forest biomass (Fmax) (a) wood harvest: Domestic Extraction of wood per unit forest area; (b) wood import dependence: physical trade balance of wood as fraction of domestic consumption of wood (negative values indicate net exports); (c) woodfuel importance: fraction of woodfuel in total domestic energy consumption.
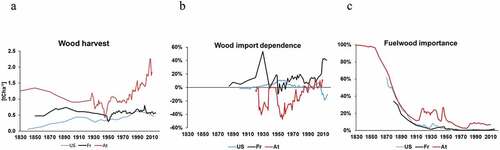
The multiple regressions conducted for the underlying drivers wood import dependence and woodfuel importance confirm that Fmax does not correlate well with socio-metabolic drivers, reaching the highest value in France, where R2 is 0.47. However, for France and Austria, limited data availability did not enable us to conduct regressions covering the entire time series, and skewed the results. Net trade in wood ()) contributed to the stable domestic wood production in France, while in Austria, net exports declined throughout the second half of the 20th century and turned into net imports in the 21st century. In the United States on the other hand, wood trade balances were fairly stable. Thus, while there is evidence that globally, countries that have experienced forest transitions tend to import more forest-risk commodities, including wood from tree plantations, contributing to deforestation abroad (Pendrill et al., Citation2019, Citation2019), we see no conclusive evidence from the three case studies investigated.
Finally, woodfuel substitution by fossil fuels played a major role in mitigating or stabilizing wood harvest on forests in all three countries, as demonstrated by the strong reduction of the woodfuel fraction in the energy mix ()). Even though wood harvest only declined in France, this driver would have been much stronger if forests had continued to provide energy (Gingrich et al., Citation2021; Le Noë et al., Citation2021b). While the regression results on this variable are not conclusive due to differences in timing between fuel shifts and the dynamics of Fmax, at least virtually, the energy substitution process reduced pressure on forests. The surprisingly low correlation between any of the socio-metabolic drivers and Fmax may arise from the fact that trends in this variable are determined equally by actual biomass dynamics as by dynamics in Bmax. Therefore, understanding the societal and environmental drivers of the Bmax increase emerges as important further research frontier.
Discussion
Our investigation of forest transitions in the United States, France and Austria highlights different pathways of long-term forest recovery. While the three forest transitions were the combined results of forest area expansion and biomass thickening with diverging relevance of these two factors, thickening resulted from two independent factors: Firstly, higher maximum biomass densities contributed to growing biomass stocks in all case studies. Secondly, the distance between observed and maximum biomass stocks was reduced in two case studies (United States and Austria), further explaining vegetation thickening, while this distance increased in France because of forest rejuvenation due to area expansion. Going beyond previous analyses investigating forest carbon dynamics as the product of forest area and biomass density (Kauppi et al., Citation2006; Köhl et al., Citation2015; Shi et al., Citation2011), our novel forest identity thus enables us to identify how biomass density change was affected by changes in maximum forest biomass density under given environmental and management conditions, and actual biomass as fraction of maximum biomass.
Across case studies and to diverging degrees, the processes of forest change were linked to changes in the proximate and underlying drivers of societal resource use, or social metabolism. Agricultural intensification was observed in all three countries, and the regression analysis revealed that together with agricultural import dependence, this factor has high explanatory power for forest expansion in France and Austria, where forest expansion was more pronounced than in the United States. This finding is in line with existing research highlighting that agricultural intensification and spatial concentration of agriculture are major enabling conditions of forest recovery in many national forest transitions (García et al., Citation2020; Jadin et al., Citation2016) and globally (Burney et al., Citation2010).
In addition, we found that the decline of forest grazing was concomitant to the increase in maximum forest biomass density across case studies, confirmed by high R2 values in the United States and France, thus suggesting that a reduction of forest secondary uses was a major driver of improved forest growth conditions enabling forest recovery. While data on forest grazing rely on rough estimates, this result is consistent with previous studies highlighting the impact of grazing on biomass stocks (Erb et al., Citation2018). Quantifying the relative contribution of reduced forest grazing to changes in maximum forest biomass, as opposed to other factors such as environmental change and other forest management, remains an important research frontier.
Beyond these clear connections between forest change and agricultural change, our analysis also points towards more complex impacts of resource use on forest change: Our results only partly confirm that a change in wood harvest affected the dynamics in forest biomass stocks as a fraction of maximum biomass: instead, we even observe periods of simultaneous increases in harvest and biomass stocks. However, such a simultaneous increase is a transitory phenomenon, apparent after the reduction of forest side uses, and is not sustained over time periods longer than a few decades in any of the case studies we analysed. Better integrating legacy effects in the analysis of harvest impacts on forest biomass stocks (Thom et al., Citation2018) is therefore a major task for future research, particularly in the context of ongoing climate change affecting forest resilience (Johnstone et al., Citation2016; Reyer et al., Citation2015).
The declining share of woodfuel in total energy consumption in all three case studies, while not concomitant with dynamics in biomass recovery, underlines the changing role of forests during forest transitions from providing a plethora of resources towards a commodification for timber production (Heilmayr et al., Citation2016; Magerl et al., Citation2022; Pichler et al., Citation2021b). This change in the provisioning function of forests is connected to resource substitution and associated problem shifts i.e. use of more efficient energy carriers instead of woodfuel, leading to increased supply and demand of these energy sources and thus surging greenhouse gas emissions from fossil energy use (Henriques & Borowiecki, Citation2017).
Finally, using the socio-metabolic indicator physical trade balance of biomass, our results did not substantiate the hypothesis that the production of agricultural biomass or the recovery of forests largely relied on biomass imports in any of the case studies. Of course, the indicators chosen only represent tons of net trade in biomass as fraction of domestic consumption, irrespective of the land demand or deforestation impacts of different traded products, which are specifically high e.g. for beef and soy in the Amazon (Zu Ermgassen et al., Citation2020; Nepstad et al., Citation2014), or oil palm in Southeast Asia (Austin et al., Citation2017; Vijay et al., Citation2016). Also, the limited sample size of only three case studies, two of which are major exporters of agricultural products, may contribute to a bias here. Despite these limitations, and in stark contrast to deforestation and forest degradation being linked to production for exports in several countries of the Global South (Henders et al., Citation2015; Pendrill et al., Citation2019; Roux et al., Citation2021), our results point to the fact that forest recovery is not necessarily enabled by increased biomass imports in industrialized countries.
Our analysis has demonstrated that forest change may be the result of a number of dynamics within the forest, enabled by diverging proximate and underlying drivers associated to resource use. Ending deforestation today, a major challenge in many tropical countries in the Global South (Harris et al., Citation2021; Le Noë et al., Citation2021a), will need to avoid falling into patterns of unsustainable resource use characteristic of today’s industrialized countries (Fanning et al., Citation2021; O’Neill et al., Citation2018). Specifically, our findings highlight two major sustainability challenges connected to present and future forest conservation.
Firstly, it will be crucial that harvest allows for conserving forest C sinks while satisfying the needs of diverse social groups, specifically under expected further increase of forest disturbances due to climate change (Seidl et al., Citation2017) when high harvest levels may no longer coincide with continuing forest growth, as in the historical cases described here. This may entail reducing the levels of annual wood extraction in intensively used forests (Law et al., Citation2018), and challenges the overly optimistic plans to increase wood use as a sustainability strategy. Other options for energy provision appear more promising, focusing on reducing land demand (Milbrandt et al., Citation2014; Turkovska et al., Citation2021) and fostering energy savings and equitable access (Millward-Hopkins et al., Citation2020; Oswald et al., Citation2020).
Secondly, minimizing agricultural land demand and mitigating emissions, while providing healthy food for all will be a major future sustainability challenge. Here, combining low-meat diets with agroecological intensification (Billen et al., Citation2021; Morais et al., Citation2021; Theurl et al., Citation2020), including the integration of trees in agricultural systems (Ramachandran Nair et al., Citation2009), appear as promising options. In addition, support for context-specific sustainable land-use practices that preserve forest ecosystems or increase forest cover while meeting local needs has been demonstrated to be not only more just, but also more effective, if collaboration with local communities (Min-Venditti et al., Citation2017) and specifically with Indigenous Peoples (Sze et al., Citation2021) is realized.
Conclusion
This study investigated long-term forest transition pathways, the forest change processes they resulted from and the proximate and underlying socio-metabolic drivers enabling them in the United States, France, and Austria. We demonstrated that the relative contribution of area, biomass maximum per area, and biomass stocks as fraction of maximum play diverging roles in explaining the different forest transitions, opening new grounds for exploring the dynamics of long-term forest change. In addition, we identified connections between forest dynamics and agricultural intensification and reduced forest grazing, demonstrating how forest change is impacted by agricultural practices in specific ways. Our findings call for further investigations of what impacted long-term dynamics in forest growth conditions, and for integrated perspectives on forest conservation that take long-term problem shifts between the forest use and other land and resource use into account.
Acknowledgments
The authors thank two anonymous reviewers for their constructive feedback on an earlier version of the manuscript. Open access funding was provided by University of Natural Resources and Life Sciences Vienna (BOKU).
Disclosure statement
No potential conflict of interest was reported by the author(s).
Data availability statement
All data underlying the figures presented in this article is availble here: https://doi.org/10.5281/zenodo.5797877
Additional information
Funding
References
- Ang, B.W., & Zhang, F.Q. (2000). A survey of index decomposition analysis in energy and environmental studies. Energy, 25(12), 1149–1176. https://doi.org/10.1016/S0360-5442(00)00039-6
- Ang, B.W. (2005). The LMDI approach to decomposition analysis: A practical guide. Energy Policy, 33(7), 867–871. https://doi.org/10.1016/j.enpol.2003.10.010
- Ashraf, J., Pandey, R., & de Jong, W. (2017). Assessment of bio-physical, social and economic drivers for forest transition in Asia-Pacific region. Forest Policy and Economics, 76, 35–44. https://doi.org/10.1016/j.forpol.2016.07.008
- Aspinall, R.J., Staiano, M., & Pearson, D.M. (2021). Data, time, change and land-system dynamics. Journal of Land Use Science, 16(2), 129–141. https://doi.org/10.1080/1747423X.2021.1879297
- Austin, K.G., Mosnier, A., Pirker, J., McCallum, I., Fritz, S., & Kasibhatla, P.S. (2017). Shifting patterns of oil palm driven deforestation in Indonesia and implications for zero-deforestation commitments. Land Use Policy, 69, 41–48. https://doi.org/10.1016/j.landusepol.2017.08.036
- Bastin, J.-F., Finegold, Y., Garcia, C., Mollicone, D., Rezende, M., Routh, D., Zohner, C.M., & Crowther, T.W. (2019). The global tree restoration potential. Science, 365(6448), 76–79. https://doi.org/10.1126/science.aax0848
- Billen, G., Aguilera, E., Einarsson, R., Garnier, J., Gingrich, S., Grizzetti, B., Lassaletta, L., Le Noë, J., & Sanz-Cobena, A. (2021). Reshaping the European agro-food system and closing its nitrogen cycle: The potential of combining dietary change, agroecology, and circularity. One Earth, 4(6), 839–850. https://doi.org/10.1016/j.oneear.2021.05.008
- Borman, M.M. (2005). Forest stand dynamics and livestock grazing in historical context. Conservation Biology, 19(5), 1658–1662. https://doi.org/10.1111/j.1523-1739.2005.00115.x
- Burney, J.A., Davis, S.J., & Lobell, D.B. (2010). Greenhouse gas mitigation by agricultural intensification. Proceedings of the National Academy of Sciences, 107(26), 12052–12057. https://doi.org/10.1073/pnas.0914216107
- Cunfer, G., Watson, A., & MacFadyen, J. (2018). Energy profiles of an agricultural frontier: The American Great Plains, 1860–2000. Regional Environmental Change, 18(4), 1021–1032. https://doi.org/10.1007/s10113-017-1157-x
- Di Sacco, A., Hardwick, K.A., Blakesley, D., Brancalion, P.H.S., Breman, E., Cecilio Rebola, L., Chomba, S., Dixon, K., Elliott, S., Ruyonga, G., Shaw, K., Smith, P., Smith, R.J., & Antonelli, A. (2021). Ten golden rules for reforestation to optimize carbon sequestration, biodiversity recovery and livelihood benefits. Global Change Biology, 27(7), 1328–1348. https://doi.org/10.1111/gcb.15498
- Dorninger, C., & Eisenmenger, N. (2016). South America’s biophysical involvement in international trade: The physical trade balances of Argentina, Bolivia, and Brazil in the light of ecologically unequal exchange. Journal of Political Ecology, 23(1), 394–409. doi:10.2458/v23i1.20240.
- Duby, G., & Wallon, A. (1976). Histoire de la France rurale. Volume 4, La Fin de la France paysanne de 1914 à nos jours. Seuil.
- Erb, K.-H., Kastner, T., Plutzar, C., Bais, A.L.S., Carvalhais, N., Fetzel, T., Gingrich, S., Haberl, H., Lauk, C., Niedertscheider, M., Pongratz, J., Thurner, M., & Luyssaert, S. (2018). Unexpectedly large impact of forest management and grazing on global vegetation biomass. Nature, 553(7686), 73–76. https://doi.org/10.1038/nature25138
- European Commission. (2018). A sustainable bioeconomy for Europe: Strengthening the connection between economy, society and the environment: Updated bioeconomy strategy. Publications Office.
- Fanning, A.L., O’Neill, D.W., Hickel, J., & Roux, N. (2021). The social shortfall and ecological overshoot of nations. Nature Sustainability. https://doi.org/10.1038/s41893-021-00799-z
- FAO. (2020). Global forest resources assessment 2020. https://doi.org/10.4060/ca8753en
- García, V.R., Caravaggio, N., Gaspart, F., & Meyfroidt, P. (2021). Long- and short-run forest dynamics: An empirical assessment of forest transition, environmental Kuznets curve and ecologically unequal exchange theories. Forests, 12(4), 431. https://doi.org/10.3390/f12040431
- García, V.R., Gaspart, F., Kastner, T., & Meyfroidt, P. (2020). Agricultural intensification and land use change: Assessing country-level induced intensification, land sparing and rebound effect. Environmental Research Letters, 15(8), 085007. https://doi.org/10.1088/1748-9326/ab8b14
- Garnier, J., Le Noë, J., Marescaux, A., Sanz-Cobena, A., Lassaletta, L., Silvestre, M., Thieu, V., & Billen, G. (2019). Long-term changes in greenhouse gas emissions from French agriculture and livestock (1852–2014): From traditional agriculture to conventional intensive systems. Science of the Total Environment, 660, 1486–1501. https://doi.org/10.1016/j.scitotenv.2019.01.048
- Geist, H.J., & Lambin, E.F. (2002). Proximate causes and underlying driving forces of tropical deforestation. BioScience, 52(2), 143. https://doi.org/10.1641/0006-3568(2002)052[0143:PCAUDF]2.0.CO;2
- Gierlinger, S., & Krausmann, F. (2012). The physical economy of the United States of America: Extraction, trade, and consumption of materials from 1870 to 2005. Journal of Industrial Ecology, 16(3), 365–377. https://doi.org/10.1111/j.1530-9290.2011.00404.x
- Gimmi, U., & Buergi, M. (2007). Using oral history and forest management plans to reconstruct traditional non-timber forest uses in the Swiss Rhone valley (Valais) since the late nineteenth century. Environment and History, 13(2), 211–246. https://doi.org/10.3197/096734007780473492
- Gingrich, S., Erb, K.-H., Krausmann, F., Gaube, V., & Haberl, H. (2007). Long-term dynamics of terrestrial carbon stocks in Austria: A comprehensive assessment of the time period from 1830 to 2000. Regional Environmental Change, 7(1), 37–47. https://doi.org/10.1007/s10113-007-0024-6
- Gingrich, S., & Krausmann, F. (2018). At the core of the socio-ecological transition: Agroecosystem energy fluxes in Austria 1830–2010. Science of the Total Environment, 645, 119–129. https://doi.org/10.1016/j.scitotenv.2018.07.074
- Gingrich, S., Lauk, C., Kastner, T., Krausmann, F., Haberl, H., & Erb, K.-H. (2016). A forest transition: Austrian carbon budgets 1830–2010. In H. Haberl, M. Fischer-Kowalski, F. Krausmann, & V. Winiwarter (Eds.), Social ecology: Society-nature relations across time and space (pp. 417–431). Springer International Publishing.
- Gingrich, S., Lauk, C., Krausmann, F., Erb, K.-H., & Le Noë, J. (2021). Changes in energy and livestock systems largely explain the forest transition in Austria (1830-1910). Land Use Policy, 109, 105624. http://dx.doi.org/10.5751/ES-05556-180238
- Gingrich, S., Lauk, C., Niedertscheider, M., Pichler, M., Schaffartzik, A., Schmid, M., Magerl, A., Le Noë, J., Bhan, M., & Erb, K. (2019). Hidden emissions of forest transitions: A socio-ecological reading of forest change. Current Opinion in Environmental Sustainability, 38, 14–21. https://doi.org/10.1016/j.cosust.2019.04.005
- Gingrich, S., Niedertscheider, M., Kastner, T., Haberl, H., Cosor, G., Krausmann, F., Kuemmerle, T., Müller, D., Reith-Musel, A., Jepsen, M.R., Vadineanu, A., & Erb, K.-H. (2015). Exploring long-term trends in land use change and aboveground human appropriation of net primary production in nine European countries. Land Use Policy, 47, 426–438. https://doi.org/10.1016/j.landusepol.2015.04.027
- Haberl, H., Fischer-Kowalski, M., Krausmann, F., Weisz, H., & Winiwarter, V. (2004). Progress towards sustainability? What the conceptual framework of material and energy flow accounting (MEFA) can offer. Land Use Policy, 21(3), 199–213. https://doi.org/10.1016/j.landusepol.2003.10.013
- Haberl, H., Fischer-Kowalski, M., Krausmann, F., & Winiwarter, V. (Eds.). (2016). Social ecology. Society-nature relations across time and space. Springer Berlin Heidelberg.
- Haberl, H., Wiedenhofer, D., Pauliuk, S., Krausmann, F., Müller, D.B., & Fischer-Kowalski, M. (2019). Contributions of sociometabolic research to sustainability science. Nature Sustainability, 2(3), 173–184. https://doi.org/10.1038/s41893-019-0225-2
- Harris, N.L., Gibbs, D.A., Baccini, A., Birdsey, R.A., de Bruin, S., Farina, M., Fatoyinbo, L., Hansen, M.C., Herold, M., Houghton, R.A., Potapov, P.V., Suarez, D.R., Roman-Cuesta, R.M., Saatchi, S.S., Slay, C.M., Turubanova, S.A., & Tyukavina, A. (2021). Global maps of twenty-first century forest carbon fluxes. Nature Climate Change, 11(3), 234–240. https://doi.org/10.1038/s41558-020-00976-6
- Hawbaker, T.J., Vanderhoof, M.K., Schmidt, G.L., Beal, Y.-J., Picotte, J.J., Takacs, J.D., Falgout, J.T., & Dwyer, J.L. (2020). The Landsat Burned Area algorithm and products for the conterminous United States. Remote Sensing of Environment, 244, 111801. https://doi.org/10.1016/j.rse.2020.111801
- Heilmayr, R., Echeverría, C., Fuentes, R., & Lambin, E.F. (2016). A plantation-dominated forest transition in Chile. Applied Geography, 75, 71–82. https://doi.org/10.1016/j.apgeog.2016.07.014
- Henders, S., Persson, U.M., & Kastner, T. (2015). Trading forests: Land-use change and carbon emissions embodied in production and exports of forest-risk commodities. Environmental Research Letters, 10(12), 125012. https://doi.org/10.1088/1748-9326/10/12/125012
- Henriques, S.T., & Borowiecki, K.J. (2017). The drivers of long-run CO2 emissions in Europe, North America and Japan since 1800. Energy Policy, 101, 537–549. https://doi.org/10.1016/j.enpol.2016.11.005
- Hickler, T., Smith, B., Prentice, I.C., Mjöfors, K., Miller, P., Arneth, A., & Sykes, M.T. (2008). CO2 fertilization in temperate FACE experiments not representative of boreal and tropical forests. Global Change Biology, 14(7), 1531–1542. https://doi.org/10.1111/j.1365-2486.2008.01598.x
- Hong, C., Burney, J.A., Pongratz, J., Nabel, J.E.M.S., Mueller, N.D., Jackson, R.B., & Davis, S.J. (2021). Global and regional drivers of land-use emissions in 1961–2017. Nature, 589(7843), 554–561. https://doi.org/10.1038/s41586-020-03138-y
- Hornbeck, R. (2012). The enduring impact of the American Dust Bowl: Short- and long-run adjustments to environmental Catastrophe. American Economic Review, 102(4), 1477–1507. https://doi.org/10.1257/aer.102.4.1477
- Houghton, R.A., Hackler, J.L., & Lawrence, K.T. (2000). Changes in terrestrial carbon storage in the United States. 2: The role of fire and fire management. Global Ecology and Biogeography, 9(2), 145–170. https://doi.org/10.1046/j.1365-2699.2000.00164.x
- Houghton, R.A., & Hackler, J.L. (2000). Changes in terrestrial carbon storage in the United States. 1: The roles of agriculture and forestry. Global Ecology and Biogeography, 9(2), 125–144. https://doi.org/10.1046/j.1365-2699.2000.00166.x
- IPCC. (2019). Climate change and land: An IPCC special report on climate change, desertification, land degradation, sustainable land management, food security, and greenhouse gas fluxes in terrestrial ecosystems. In press.
- Jadin, I., Meyfroidt, P., & Lambin, E.F. (2016). International trade, and land use intensification and spatial reorganization explain Costa Rica’s forest transition. Environmental Research Letters, 11(3), 035005. https://doi.org/10.1088/1748-9326/11/3/035005
- Johnstone, J.F., Allen, C.D., Franklin, J.F., Frelich, L.E., Harvey, B.J., Higuera, P.E., Mack, M.C., Meentemeyer, R.K., Metz, M.R., Perry, G.L., Schoennagel, T., & Turner, M.G. (2016). Changing disturbance regimes, ecological memory, and forest resilience. Frontiers in Ecology and the Environment, 14(7), 369–378. https://doi.org/10.1002/fee.1311
- Kastner, T., Chaudhary, A., Gingrich, S., Marques, A., Persson, U.M., Bidoglio, G., Le Provost, G., & Schwarzmüller, F. (2021). Global agricultural trade and land system sustainability: Implications for ecosystem carbon storage, biodiversity, and human nutrition. One Earth, 4(10), 1425–1443. https://doi.org/10.1016/j.oneear.2021.09.006
- Kastner, T., Erb, K.-H., & Haberl, H. (2014). Rapid growth in agricultural trade: Effects on global area efficiency and the role of management. Environmental Research Letters, 9(3), 034015. https://doi.org/10.1088/1748-9326/9/3/034015
- Kastner, T., Erb, K.-H., & Nonhebel, S. (2011). International wood trade and forest change: A global analysis. Global Environmental Change, 21(3), 947–956. https://doi.org/10.1016/j.gloenvcha.2011.05.003
- Kauppi, P.E., Ausubel, J.H., Fang, J., Mather, A.S., Sedjo, R.A., & Waggoner, P.E. (2006). Returning forests analyzed with the forest identity. Proceedings of the National Academy of Sciences, 103(46), 17574–17579. https://doi.org/10.1073/pnas.0608343103
- Keeton, W.S. (2008). Evaluation of tree seedling mortality and protective strategies in riparian forest restoration. Northern Journal of Applied Forestry, 25(3), 117–123. https://doi.org/10.1093/njaf/25.3.117
- Köhl, M., Lasco, R., Cifuentes, M., Jonsson, Ö., Korhonen, K.T., Mundhenk, P., de Jesus Navar, J., & Stinson, G. (2015). Changes in forest production, biomass and carbon: Results from the 2015 UN FAO Global Forest Resource Assessment. Forest Ecology and Management, 352, 21–34. https://doi.org/10.1016/j.foreco.2015.05.036
- Komlos, J. (1983). The Habsburg Monarchy as a customs union: Economic development in Austria-Hungary in the nineteenth century.
- Krausmann, F., Gaugl, B., West, J., & Schandl, H. (2016). The metabolic transition of a planned economy: Material flows in the USSR and the Russian Federation 1900 to 2010. Ecological Economics, 124, 76–85. https://doi.org/10.1016/j.ecolecon.2015.12.011
- Krausmann, F., Haberl, H., Schulz, N.B., Erb, K.-H., Darge, E., & Gaube, V. (2003). Land-use change and socio-economic metabolism in Austria—Part I: Driving forces of land-use change: 1950–1995. Land Use Policy, 20(1), 1–20. https://doi.org/10.1016/S0264-8377(02)00048-0
- Krausmann, F., & Langthaler, E. (2019). Food regimes and their trade links: A socio-ecological perspective. Ecological Economics, 160, 87–95. https://doi.org/10.1016/j.ecolecon.2019.02.011
- Kuskova, P., Gingrich, S., & Krausmann, F. (2008). Long term changes in social metabolism and land use in Czechoslovakia, 1830–2000: An energy transition under changing political regimes. Ecological Economics, 68(1–2), 394–407. https://doi.org/10.1016/j.ecolecon.2008.04.006
- Larrère, R., & Nougarède, O. (1990). La forêt dans l’histoire des systèmes agraires: De la dissociation à la réinsertion. Cahiers d’économie et sociologie rurales, 15–16, 11–38.
- Law, B.E., Hudiburg, T.W., Berner, L.T., Kent, J.J., Buotte, P.C., & Harmon, M.E. (2018). Land use strategies to mitigate climate change in carbon dense temperate forests. Proceedings of the National Academy of Sciences, 115(14), 3663–3668. https://doi.org/10.1073/pnas.1720064115
- Le Noë, J., Billen, G., Esculier, F., & Garnier, J. (2018). Long-term socioecological trajectories of agro-food systems revealed by N and P flows in French regions from 1852 to 2014. Agriculture, Ecosystems & Environment, 265, 132–143. https://doi.org/10.1016/j.agee.2018.06.006
- Le Noë, J., Erb, K.-H., Matej, S., Magerl, A., Bhan, M., & Gingrich, S. (2021a). Altered growth conditions more than reforestation counteracted forest biomass carbon emissions 1990–2020. Nature Communications, 12(1), 6075. https://doi.org/10.1038/s41467-021–26398-2
- Le Noë, J., Erb, K.-H., Matej, S., Magerl, A., Bhan, M., & Gingrich, S. (2021b). Socio-ecological drivers of long-term ecosystem carbon stock trend: An assessment with the LUCCA model of the French case. Anthropocene, 33, 100275. https://doi.org/10.1016/j.ancene.2020.100275
- Le Noë, J., Matej, S., Magerl, A., Bhan, M., Erb, K., & Gingrich, S. (2020). Modeling and empirical validation of long‐term carbon sequestration in forests (France, 1850–2015). Global Change Biology, 26(4), 2421–2434. https://doi.org/10.1111/gcb.15004
- Liski, J., Perruchoud, D., & Karjalainen, T. (2002). Increasing carbon stocks in the forest soils of Western Europe. Forest Ecology and Management, 169(1–2), 159–175. https://doi.org/10.1016/S0378-1127(02)00306-7
- Liu, J., Liang, M., Li, L., Long, H., & De Jong, W. (2017). Comparative study of the forest transition pathways of nine Asia-Pacific countries. Forest Policy and Economics, 76, 25–34. https://doi.org/10.1016/j.forpol.2016.03.007
- Magalhães, N., Fressoz, J.-B., Jarrige, F., Le Roux, T., Levillain, G., Lyautey, M., Noblet, G., & Bonneuil, C. (2019). The physical economy of France (1830–2015). The History of a Parasite?. Ecological Economics, 157, 291–300. https://doi.org/10.1016/j.ecolecon.2018.12.001
- Magerl, A., Le Noe, J., Erb, K., Bhan, M., & Gingrich, S. (2019). A comprehensive data-based assessment of forest ecosystem carbon stocks in the U.S. 1907-2012. Environmental Research Letters, 14(12), 125015. https://doi.org/10.1088/1748-9326/ab5cb6
- Magerl, A., Matej, S., Kaufmann, L., Noë, J.L., Erb, K., & Gingrich, S. (2022). Forest carbon sink in the U.S. (1870–2012) driven by substitution of forest ecosystem service flows. Resources, Conservation and Recycling, 176, 105927. https://doi.org/10.1016/j.resconrec.2021.105927
- Mansfield, B., Munroe, D.K., & McSweeney, K. (2010). Does economic growth cause environmental recovery? Geographical explanations of forest regrowth. Geography Compass, 4(5), 416–427. https://doi.org/10.1111/j.1749-8198.2010.00320.x
- Marschall, J. (1975). Hilfstafeln für die Forsteinrichtung. Österreichischer Agrarverlag. Wien.
- Martinico-Perez, M.F.G., Fishman, T., Okuoka, K., & Tanikawa, H. (2017). Material flow accounts and driving factors of economic growth in the Philippines: MFA and driving factors in the Philippines. Journal of Industrial Ecology, 21(5), 1226–1236. https://doi.org/10.1111/jiec.12496
- Mather, A.S. (1992). The forest transition. Area, 24(4), 367–379. https://www.jstor.org/stable/20003181
- Meyfroidt, P., & Lambin, E.F. (2011). Global forest transition: Prospects for an end to deforestation. Annual Review of Environment and Resources, 36(1), 343–371. https://doi.org/10.1146/annurev-environ-090710-143732
- Meyfroidt, P. (2016). Approaches and terminology for causal analysis in land systems science. Journal of Land Use Science, 11(5), 501–522. https://doi.org/10.1080/1747423X.2015.1117530
- Milbrandt, A.R., Heimiller, D.M., Perry, A.D., & Field, C.B. (2014). Renewable energy potential on marginal lands in the United States. Renewable and Sustainable Energy Reviews, 29, 473–481. https://doi.org/10.1016/j.rser.2013.08.079
- Millward-Hopkins, J., Steinberger, J.K., Rao, N.D., & Oswald, Y. (2020). Providing decent living with minimum energy: A global scenario. Global Environmental Change, 65, 102168. https://doi.org/10.1016/j.gloenvcha.2020.102168
- Min-Venditti, A.A., Moore, G.W., & Fleischman, F. (2017). What policies improve forest cover? A systematic review of research from Mesoamerica. Global Environmental Change, 47, 21–27. https://doi.org/10.1016/j.gloenvcha.2017.08.010
- Morais, T.G., Teixeira, R.F.M., Lauk, C., Theurl, M.C., Winiwarter, W., Mayer, A., Kaufmann, L., Haberl, H., Domingos, T., & Erb, K.H. (2021). Agroecological measures and circular economy strategies to ensure sufficient nitrogen for sustainable farming. Global Environmental Change, 69, 102313. https://doi.org/10.1016/j.gloenvcha.2021.102313
- Myllyntaus, T., & Mattila, T. (2002). Decline or increase? The standing timber stock in Finland, 1800–1997. Ecological Economics, 41(2), 271–288. https://doi.org/10.1016/S0921-8009(02)00034-4
- Nepstad, D., McGrath, D., Stickler, C., Alencar, A., Azevedo, A., Swette, B., Bezerra, T., DiGiano, M., Shimada, J., Seroa da Motta, R., Armijo, E., Castello, L., Brando, P., Hansen, M.C., McGrath-Horn, M., Carvalho, O., & Hess, L. (2014). Slowing Amazon deforestation through public policy and interventions in beef and soy supply chains. Science, 344(6188), 1118–1123. https://doi.org/10.1126/science.1248525
- Niedertscheider, M., Tasser, E., Patek, M., Rüdisser, J., Tappeiner, U., & Erb, K.-H. (2017). Influence of land-use intensification on vegetation C-Stocks in an Alpine Valley from 1865 to 2003. Ecosystems, 20(8), 1391–1406. https://doi.org/10.1007/s10021-017-0120-5
- O’Neill, D.W., Fanning, A.L., Lamb, W.F., & Steinberger, J.K. (2018). A good life for all within planetary boundaries. Nature Sustainability, 1(2), 88–95. https://doi.org/10.1038/s41893-018-0021-4
- Oswald, Y., Owen, A., & Steinberger, J.K. (2020). Large inequality in international and intranational energy footprints between income groups and across consumption categories. Nature Energy, 5(3), 231–239. https://doi.org/10.1038/s41560-020-0579-8
- Oswalt, S.N., Smith, W.B., Miles, P.D., & Pugh, S.A., 2014. Forest resources of the United States, 2012: A technical document supporting the Forest Service 2010 update of the RPA Assessment (No. WO-GTR-91). U.S. Department of Agriculture, Forest Service. https://doi.org/10.2737/WO-GTR-91
- Pauliuk, S., & Hertwich, E.G. (2015). Socioeconomic metabolism as paradigm for studying the biophysical basis of human societies. Ecological Economics, 119, 83–93. https://doi.org/10.1016/j.ecolecon.2015.08.012
- Pendrill, F., Persson, U.M., Godar, J., & Kastner, T. (2019). Deforestation displaced: Trade in forest-risk commodities and the prospects for a global forest transition. Environmental Research Letters, 14(5), 055003. https://doi.org/10.1088/1748-9326/ab0d41
- Pichler, M., Bhan, M., & Gingrich, S. (2021a). The social and ecological costs of reforestation. Territorialization and industrialization of land use accompany forest transitions in Southeast Asia. Land Use Policy, 101, 105180. https://doi.org/10.1016/j.landusepol.2020.105180
- Pichler, M., Schmid, M., & Gingrich, S. (2021b). Mechanisms to exclude local people from forests: Shifting power relations in forest transitions. Ambio. https://doi.org/10.1007/s13280-021-01613-y
- Ramachandran Nair, P.K., Mohan Kumar, B., & Nair, V.D. (2009). Agroforestry as a strategy for carbon sequestration. Journal of Plant Nutrition and Soil Science, 172(1), 10–23. https://doi.org/10.1002/jpln.200800030
- Ramankutty, N., Heller, E., & Rhemtulla, J. (2010). Prevailing Myths about agricultural abandonment and forest regrowth in the United States. Annals of the Association of American Geographers, 100(3), 502–512. https://doi.org/10.1080/00045601003788876
- Resende, M.D.V., Resende, M.F.R., Sansaloni, C.P., Petroli, C.D., Missiaggia, A.A., Aguiar, A.M., Abad, J.M., Takahashi, E.K., Rosado, A.M., Faria, D.A., Pappas, G.J., Kilian, A., & Grattapaglia, D. (2012). Genomic selection for growth and wood quality in Eucalyptus: Capturing the missing heritability and accelerating breeding for complex traits in forest trees. New Phytologist, 194(1), 116–128. https://doi.org/10.1111/j.1469-8137.2011.04038.x
- Reyer, C.P.O., Brouwers, N., Rammig, A., Brook, B.W., Epila, J., Grant, R.F., Holmgren, M., Langerwisch, F., Leuzinger, S., Lucht, W., Medlyn, B., Pfeifer, M., Steinkamp, J., Vanderwel, M.C., Verbeeck, H., Villela, D.M., & Coomes, D. (2015). Forest resilience and tipping points at different spatio-temporal scales: Approaches and challenges. Journal of Ecology, 103, 5–15. https://doi.org/10.1111/1365-2745.12337
- Rossi, S., Deslauriers, A., Griçar, J., Seo, J.-W., Rathgeber, C.B., Anfodillo, T., Morin, H., Levanic, T., Oven, P., & Jalkanen, R. (2008). Critical temperatures for xylogenesis in conifers of cold climates. Global Ecology and Biogeography, 17(6), 696–707. https://doi.org/10.1111/j.1466-8238.2008.00417.x
- Roux, N., Kastner, T., Erb, K.-H., & Haberl, H. (2021). Does agricultural trade reduce pressure on land ecosystems? Decomposing drivers of the embodied human appropriation of net primary production. Ecological Economics, 181, 106915. https://doi.org/10.1016/j.ecolecon.2020.106915
- Roy Chowdhury, R., & Moran, E.F. (2012). Turning the curve: A critical review of Kuznets approaches. Applied Geography, 32(1), 3–11. https://doi.org/10.1016/j.apgeog.2010.07.004
- San Roman Sanz, A., Fernandez, C., Mouillot, F., Ferrat, L., Istria, D., & Pasqualini, V. (2013). Long-term forest dynamics and land-use abandonment in the Mediterranean Mountains, Corsica, France. Ecology and Society, 18(art38). https://doi.org/10.5751/ES-05556-180238
- Schaffartzik, A., Haberl, H., Kastner, T., Wiedenhofer, D., Eisenmenger, N., & Erb, K.-H. (2015). Trading land: A review of approaches to accounting for upstream land requirements of traded products: A review of upstream land accounts. Journal of Industrial Ecology, 19(5), 703–714. https://doi.org/10.1111/jiec.12258
- Scheidel, A., & Gingrich, S. (2020). Toward sustainable and just forest recovery: Research gaps and potentials for knowledge integration. One Earth, 3(6), 680–690. https://doi.org/10.1016/j.oneear.2020.11.005
- Scheidel, A. (2019). Carbon stock indicators: Reductionist assessments and contentious policies on land use. The Journal of Peasant Studies 46(5), 913–934. https://doi.org/10.1080/03066150.2018.1428952
- Seidl, R., Thom, D., Kautz, M., Martin-Benito, D., Peltoniemi, M., Vacchiano, G., Wild, J., Ascoli, D., Petr, M., Honkaniemi, J., Lexer, M.J., Trotsiuk, V., Mairota, P., Svoboda, M., Fabrika, M., Nagel, T.A., & Reyer, C.P.O. (2017). Forest disturbances under climate change. Nature Climate Change, 7(6), 395–402. https://doi.org/10.1038/nclimate3303
- Servolin, C. (1985). Les politiques agricoles. In M. Grawitz & J. Leca (Eds.), Traité Des Sciences Politiques (pp. 155–260). Presses Universitaires de France.
- Shi, L., Zhao, S., Tang, Z., Fang, J., & Moen, J. (2011). The changes in China’s forests: An analysis using the forest identity. PLoS ONE, 6(6), e20778. https://doi.org/10.1371/journal.pone.0020778
- Southworth, J., Nagendra, H., & Cassidy, L. (2012). Forest transition pathways in Asia – Studies from Nepal, India, Thailand, and Cambodia. Journal of Land Use Science, 7(1), 51–65. https://doi.org/10.1080/1747423X.2010.520342
- Stage, A.R., Renner, D.L., & Chapman, R.C., 1988. Selected yield tables for plantations and natural stands in inland northwest forests, Research Paper INT. US Department of Agriculture, Forest Service, Intermountain Research Station.
- Sze, J.S., Carrasco, L.R., Childs, D., & Edwards, D.P. (2021). Reduced deforestation and degradation in Indigenous Lands pan-tropically. Nature Sustainability. https://doi.org/10.1038/s41893-021-00815-2
- Tappeiner, U., Leitinger, G., Zariņa, A., & Bürgi, M. (2021). How to consider history in landscape ecology: Patterns, processes, and pathways. Landscape Ecology, 36, 2317–2328. https://doi.org/10.1007/s10980-020-01163-w
- Tasser, E., Walde, J., Tappeiner, U., Teutsch, A., & Noggler, W. (2007). Land-use changes and natural reforestation in the Eastern Central Alps. Agriculture, Ecosystems & Environment, 118(1–4), 115–129. https://doi.org/10.1016/j.agee.2006.05.004
- Theurl, M.C., Lauk, C., Kalt, G., Mayer, A., Kaltenegger, K., Morais, T.G., Teixira, R.F.M., Domingos, T., Winiwarter, W., Erb, K.-H., & Haberl, H. (2020). Food systems in a zero-deforestation world: Dietary change is more important than intensification for climate targets in 2050. Science of the Total Environment, 735, 139353. https://doi.org/10.1016/j.scitotenv.2020.139353
- Thom, D., Rammer, W., Garstenauer, R., & Seidl, R. (2018). Legacies of past land use have a stronger effect on forest carbon exchange than future climate change in a temperate forest landscape. Biogeosciences, 15(18), 5699–5713. https://doi.org/10.5194/bg-15-5699-2018
- Tubiello, F.N., Conchedda, G., Wanner, N., Federici, S., Rossi, S., & Grassi, G. (2021). Carbon emissions and removals from forests: New estimates, 1990–2020. Earth System Science Data, 13(4), 1681–1691. https://doi.org/10.5194/essd-13-1681–2021
- Tubiello, F.N., Salvatore, M., Rossi, S., Ferrara, A., Fitton, N., & Smith, P. (2013). The FAOSTAT database of greenhouse gas emissions from agriculture. Environmental Research Letters, 8(1), 015009. https://doi.org/10.1088/1748-9326/8/1/015009
- Turkovska, O., Castro, G., Klingler, M., Nitsch, F., Regner, P., Soterroni, A.C., & Schmidt, J. (2021). Land-use impacts of Brazilian wind power expansion. Environmental Research Letters, 16(2), 024010. https://doi.org/10.1088/1748-9326/abd12f
- Urbanski, S.P., Reeves, M.C., Corley, R.E., Silverstein, R.P., & Hao, W.M. (2018). Contiguous United States wildland fire emission estimates during 2003–2015. Earth System Science Data, 10, 2241–2274. https://doi.org/10.5194/essd-10-2241-2018
- Verburg, P.H., Crossman, N., Ellis, E.C., Heinimann, A., Hostert, P., Mertz, O., Nagendra, H., Sikor, T., Erb, K.-H., Golubiewski, N., Grau, R., Grove, M., Konaté, S., Meyfroidt, P., Parker, D.C., Chowdhury, R.R., Shibata, H., Thomson, A., & Zhen, L. (2015). Land system science and sustainable development of the earth system: A global land project perspective. Anthropocene, 12, 29–41. https://doi.org/10.1016/j.ancene.2015.09.004
- Vijay, V., Pimm, S.L., Jenkins, C.N., Smith, S.J., & Anand, M. (2016). The impacts of oil palm on recent deforestation and biodiversity loss. PLoS ONE, 11(7), e0159668. https://doi.org/10.1371/journal.pone.0159668
- Vilaysouk, X., Schandl, H., & Murakami, S. (2017). Improving the knowledge base on material flow analysis for Asian developing countries: A case study of Lao PDR. Resources, Conservation and Recycling, 127, 179–189. https://doi.org/10.1016/j.resconrec.2017.09.006
- Yan, Y. (2018). Integrate carbon dynamic models in analyzing carbon sequestration impact of forest biomass harvest. Science of the Total Environment, 615, 581–587. https://doi.org/10.1016/j.scitotenv.2017.09.326
- Yang, Y., Zhang, S., Yang, J., Chang, L., Bu, K., & Xing, X. (2014). A review of historical reconstruction methods of land use/land cover. Journal of Geographical Sciences, 24(4), 746–766. https://doi.org/10.1007/s11442-014-1117-z
- Youn, Y.-C., Choi, J., de Jong, W., Liu, J., Park, M.S., Camacho, L.D., Tachibana, S., Huudung, N.D., Bhojvaid, P.P., Damayanti, E.K., Wanneng, P., & Othman, M.S. (2016). Conditions of forest transition in Asian countries. Forest Policy and Economics. https://doi.org/10.1016/j.forpol.2016.07.005
- Zhu, Z., Piao, S., Myneni, R.B., Huang, M., Zeng, Z., Canadell, J.G., Ciais, P., Sitch, S., Friedlingstein, P., Arneth, A., Cao, C., Cheng, L., Kato, E., Koven, C., Li, Y., Lian, X., Liu, Y., Liu, R., Mao, J., Pan, Y., … Zeng, N. (2016). Greening of the Earth and its drivers. Nature Climate Change, 6(8), 791–795. https://doi.org/10.1038/nclimate3004
- Zu Ermgassen, E.K.H.J., Godar, J., Lathuillière, M.J., Löfgren, P., Gardner, T., Vasconcelos, A., & Meyfroidt, P. (2020). The origin, supply chain, and deforestation risk of Brazil’s beef exports. Proceedings of the National Academy of Sciences, 117(50), 31770–31779. https://doi.org/10.1073/pnas.2003270117