ABSTRACT
Land cover and land use changes cause global environmental degradation yet changes in cover may have different impacts than changes in use. Here, we examine changes in land cover (rangeland conversion to vineyard) and land use (assocaited grazing loss on proximate rangelands) in Santa Barbara County, USA, between 1984 and 2019 paying attention to (1) instances where grazing was removed and (2) the effectiveness of local policies in keeping grazing active. We found that 13% of rangeland parcels converted to vineyards, and that 69% of these properties ceased grazing, even though 92% of the rangeland parcels in our analysis that converted to vineyards still had over 100 acres (40ha) that could be grazed. Statistical models showed that land use policy did not have a significant impact on these changes. Our results show the importance of distinguishing between land use and land cover to understand social and natural system changes.
Introduction
Sitting at the intersection of natural and human systems, land use provides a lens for understanding negative and positive aspects of global change (Turner et al., Citation2007). Land cover and land use changes contribute to habitat and biodiversity loss and increased carbon emissions, yet also provide new opportunities for agricultural and economic development (Foley et al., Citation2005, Citation2011). Drivers of these changes span multiple scales from increasing global wealth, which contributes to aggregate changes in demand, to individual land-owners’ decisions about how to manage their parcels, which are influenced by individual economic concerns as well as personal preferences, values, and aesthetics (Liu et al., Citation2003; Meyfroidt et al., Citation2010). Concepts such as telecoupled and indirect land use change provide nuance to our understanding of land cover and land use changes, yet even with these concepts, the drivers and impacts of combined land cover and land use change can be difficult to unpack (Liu et al., Citation2013; Meyfroidt et al., Citation2018).
In research and policy, land cover is often used as a proxy for land use (Meyfroidt, Citation2016). This is a convenient assumption because it allows outputs from land cover maps derived from remote sensing imagery to model changes in land use. Often, such assumptions are justified. For example, for maps that classify land cover as row-crop agriculture, a user can feel comfortable assuming that the land use is farming. For other land covers however, the difference between land cover and land use may be important. For example, land cover that is classified as forest may have varying uses (e.g. timberland, forests protected for recreation or biodiversity objectives, secondary forest that has regrown on abandoned agricultural land), and the difference in these uses may have important social, economic, and ecological outcomes.
Rangelands, as a land cover, make up nearly 50% of global land cover, and use of rangelands vary widely with their impacts typically hard to distinguish via remote sensing (Robinson et al., Citation2019). In many areas, rangelands are grazed with ruminants, and these animals provide economic and cultural opportunities for their owners (Plieninger & Huntsinger, Citation2018; USDA NRCS, Citation2021). At the same time, grazing impacts vegetation structure which can have important implications for biodiversity, carbon sequestration, wildfire and other ecosystem processes (Davies et al., Citation2015; Nader et al., Citation2007). However, not all rangelands are managed for domestic livestock grazing. Especially in developed economies, grazing may only make economic sense if large areas are available. Therefore, small areas suitable for livestock grazing are often fallowed, leaving rangelands without managed grazing on the landscape, which can lead to cascading changes in ecosystem structure and service provision (Maher et al., Citation2021). Questions remain however about how common these ‘fallowed’ rangelands are and the social, economic, or environmental impacts of grazing removal. Although typically used in the context of intensive agricultural crops, we use the term ‘fallowing’ here to describe the removal of agricultural activity on a unit area. While in practice this grazing cessation is frequently permanent, ‘fallowing’ emphasizes that it can be temporary and that there is nothing about the land cover change that would not allow for grazing to be resumed.
In many areas, grazing provides ecosystem service benefits that may accrue to society beyond the landowner, and land use policy may be useful in encouraging landowners to provide these services (Diamond et al., Citation2009; Wolf et al., Citation2017). However, the effectiveness of many land use policies is uncertain. This is especially true for voluntary programs where landowners opt-in (e.g. conservation reserve program in the United States or the Natura 2000 program in Europe; Cason & Gangadharan, Citation2004; Hellerstein, Citation2017). These programs pay landowners directly or reduce their property tax burden in exchange for continuing farming practices. Such policies have been criticized for adverse selection problems, where landowners who are unlikely to develop their land are likely to enroll in a policy, even though it may not affect their farming decisions (Arnold et al., Citation2013). From an analysis perspective, adverse selection in program enrollment can hinder our ability to estimate arguably causal policy effects, although quasi-experimental methods have been useful in improving estimates of land use policy impacts (Butsic et al., Citation2011).
Here, we examine the effect of land cover conversions and land use change in Santa Barbara, a rapidly developing county in California, U.S.A. The area offers an ideal place to investigate the combined effects of land cover and land use change because a predominant land use change – rangeland conversion to vineyards – often changes land cover on only a small percentage of a given property, leaving the majority of rangeland unconverted. Yet often grazing is removed from the entire parcel, leading to potential changes in ecosystem functions and services. Santa Barbara County also employs the California Land Conservation Act of 1965 (colloquially known as the ‘Williamson Act’), a policy meant to encourage agricultural activity, including grazing, on parcels. Yet, it is unclear if this land use policy is effective at achieving this goal and this limits our ability to understand future changes. Using a combination of publicly available data, a unique dataset that identifies where grazing has ceased, and quasi-experimental econometric techniques, we answer three distinct questions:
What factors are associated with rangeland conversion to vineyards from 1984 to 2016?
What factors are associated with rangeland fallowing when vineyards are developed on a parcel?
Did the Williamson Act play a role in reducing rangeland conversion or rangeland fallowing?
Methods
Study area
Our study area is Santa Barbara County in California, which straddles Central and South Coast Vegetation Mapping Zones (USDA- Forest Service, Citation2009; ). The county is 7100 km2 in area and has a Mediterranean climate with a diversity of ecosystem types, including chapparal and coastal scrub, grasslands, oak woodlands, and mixed conifer forests at higher elevations (Keeley & Syphard, Citation2018). Historically, the region had frequent fires with small spatial extents, but infrequent fires that coincided with Santa Ana wind events likely burned large areas of the landscape. The frequency of these large wildfires has increased recently with the growth of human population and anthropogenic ignitions that occur during Santa Ana wind events (Keeley & Fotheringham, Citation2001; Keeley & Zedler, Citation2009). The federal government manages a large portion of the county through Los Padres National Forest and Vandenberg Air Force Base, while ranching, agriculture, and urban areas cover large swaths of the remaining land area (Conservation Biology Institute, Citation2021a). U.S. Highway 101 is the main transportation artery through the county and runs along or near the Pacific Ocean. The cities of Santa Barbara and Santa Maria are the county’s two largest incorporated cities, by population, but there are six other incorporated cities in the county, most of which are located along US Highway 101.
Figure 1. Study Area depicting properties that (1) converted to vineyards (2) converted and fallowed grazing (3) remained in rangeland and (4) were not part of the study area.
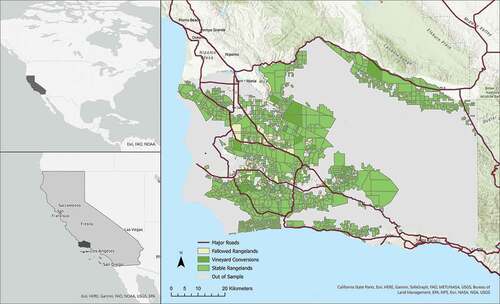
Domestic livestock, mostly cattle, have grazed on rangelands in Santa Barbara County for over two centuries (Buchman, Citation1956), and grazing has become an integral management activity to maintain important ecological values on agricultural lands. For some examples, 1) it reduces the amount of biomass produced by non-native Eurasian grass species, which in turn promotes lower-statured native forbs and reduces the threat of catastrophic wildfire (Davies et al., Citation2015; Diamond et al., Citation2009) and 2) it maintains open grassland areas necessary for the support of wildlife and, in particular, threatened and endangered species, e.g. the California Tiger Salamander (Bartolome et al., Citation2014; Didonato, Citation2006). Despite the abundant rangeland in the county, however, ranching activity has declined since the 1960s, with a decrease in both cattle and the number of ranching operations from 2002 to 2017 (Andersen et al., Citation2002; USDA-NASS, Citation2017). Still, grazing occurs on over 234,000 ha today (California Department of Conservation, Citation2021).
Data
Our primary data sources came from the following: land cover change as documented by the California Department of Conservation’s Farmland Mapping and Monitoring Program (California Department of Conservation, Citation2021); parcel boundary layers developed by Santa Barbara County (Santa Barbara County, Citation2021); vineyard field boundary data obtained from the Santa Barbara County Agricultural Commissioner’s Office; current and historical information about parcels enrolled in the Williamson Act program from the County’s Assessor’s Office; and land cover data from the Cropland Data Layer (USDA National Agricultrual Statistics Service, Citation2014). We used these data to (1) establish all parcels that were greater than 100 acres (40 ha) and contained rangeland in 1984, the first year of our study; (2) identify parcels that had some amount of conversion from rangelands to vineyards by 2019; and (3) to calculate precise vineyard acreage in the county as of 2019.
In order to identify if a parcel that converted to vineyards during the period 1984–2019 still had grazing on other portions of the parcel that did not convert to vineyards, we relied on a process derived from participatory mapping methodology (Brown & Weber, Citation2011; Ernoul et al., Citation2018). First, invitations were sent to key informants within the ranching community who were known to have extensive and geographically diverse knowledge of land use patterns and parcel history. Second, we used the 1984 FMMP data to identify grazing lands in 1984 and assumed that rangeland parcels enrolled in the Williamson Act indeed had active grazing. Participants confirmed grazing on non-enrolled lands of interest. During these sessions, interactive maps of rangeland-to-vineyard conversion were displayed using the Data Basin platform (Conservation Biology Institute, Citation2021b) and each parcel with vineyard conversion (as observed in the 2019 Ag Commissioners maps which provide vineyard boundaries) was reviewed individually. Participants were shown relevant parcel information (location, size, available ownership information, etc.) and were asked to identify if grazing was continuing on the parcel. If there was any disagreement or uncertainty amongst session participants, we employed a snowball sampling technique (Karimi & Brown, Citation2017) and asked them to provide names and contact information for other informants who would be able to provide more direct knowledge. In total, 13 individuals participated during three separate working sessions. All data elicited from key informants were input directly into the Data Basin map interface and subsequently exported as ArcGIS shapefiles containing the information.
For our econometric analysis, we used several spatially distributed variables. First, we used a dataset provided by the Santa Barbara’s Assessors office to identify, for each parcel, if it had ever been enrolled in the Williamson Act. Because our dataset is for a relatively long time period – 1984-2019 – some parcels enrolled in the Williamson Act partway through the study period, while other parcels unenrolled. In our analysis, we consider any parcel that was ever enrolled in the Williamson Act, as an enrolled parcel, although a small number (<10%) of parcels unenrolled from the Williamson Act during our study time period.
The profitability of a vineyard is related to a host of variables that may impact the quality of its wine as well as access for tourists and to markets. Therefore, for each parcel, we calculated precipitation, elevation, distance to ocean, ruggedness, distance to nearest main road, distance to nearest town, and distance to lake. In addition, we thought that development might be hindered by the presence of endangered species and so we calculated for each parcel whether an endangered species was present. The spatial data used to produce the endangered species dataset came from the California Natural Diversity Database (CNDDB, accessed 02/02/2020) and the US Fish and Wildlife Service Threatened & Endangered Species Active Critical Habitat (accessed 11/05/2019) database.
Econometric analysis
The primary goals of our econometric analysis were threefold. First, we were interested in identifying which spatial determinants and climatic factors were associated with rangeland conversion to vineyards. Second, we wanted to know, given that conversion had taken place, which factors were associated with rangeland fallowing. Third, we wanted to estimate the arguably causal relationship between the Williamson Act and the likelihood of fallowing. To answer these questions, we were faced with two econometric challenges (1) the non-random enrollment of Williamson Act parcels and (2) unobserved correlation between the decision to convert to vineyards and the decision to fallow grazing.
The non-random placement of land use policy creates difficulty in determining if the policy is having an impact, or alternatively, if land participating in the program would have been used in the same way, regardless of program participation (Jones & Lewis, Citation2015). Examples of this phenomena are the placement of protected areas on economically marginal land (Joppa et al., Citation2008), participant enrollment in Conservation Reserve Program projects, and the potential for zoning to ‘follow the market’ (Butsic et al., Citation2011; Wallace, Citation1988). One powerful way to account for this non-random placement is to use matching algorithms to pre-process data such that treated and control parcels are similar in observed covariates, which suggest they may be similar in unobserved variables as well (Butsic et al., Citation2011; Ho et al., Citation2006). Here, we used propensity score matching on the nearest neighbor without replacement to create a dataset of similar parcels, half of which were enrolled in the Williamson Act, and half that were not.
To estimate the propensity score, we parameterized a probit model with 10 variables: parcel size, number of fires in last 50 years occurring on the parcel, ruggedness, precipitation, mean elevation, threatened species, distance to ocean, distance to roads, distance to nearest town, and distance to the city of Santa Barbara. We used the natural log of parcel size and the distance variables to make data distributions more normal. We used the command psmatch2 in Stata (StataCorp, Citation2021), with a caliper set to 0.03 and conducted a post-matching analysis using the pstest command to verify that covariates of our matched dataset were similar between treated and control observations. The caliper size impacts the range of propensity scores that are considered matches. In this case, we tested calipers from 0.02 − 0.1. Our results were not sensitive to caliper size.
To address the sample selection problem presented by potentially correlated unobservables between the conversion and fallowing decisions, we used a variation of the two-step Heckman sample selection model where both the selection equation (does a parcel convert to vineyards?) and the outcome equation (does a parcel that converts fallow grazing?) have binary dependent variables (Heckman et al., Citation1996). We implemented this model in Stata using the heckprobit command.
We parameterized the model using several variables that we hypothesized were jointly associated with the decision to convert to vineyards and to fallow grazing. We included location information (distance to ocean, distance to lake, and distance to the nearest town) which relate to accessibility for tourists and to markets. We also included variables that likely influence wine quality including precipitation and elevation. Distance to ocean may also impact wine quality to the extent that fog is an important feature in establishing vineyards (Haeger, Citation2020). We also included features which may make conversion more expensive including: ruggedness of the property, if there is a threatened or endangered species present on the property, and risk of wildfire (measured by the number of fires on the property in the last 50 years). We also included parcel size, as larger parcels may be better suited for grazing than vineyard production. Finally, we included whether a parcel had ever been enrolled in the Williamson Act. We took the natural logarithm of all distance variables and parcel size to improve model fit.
Validation, accuracy assessment, robustness check
Our primary model uses a matched dataset without replacement and a caliper set at 0.03. While we believe that this model does a sufficient job to address all potential forms of bias, it has the disadvantage of dropping nearly 40% of the observations from our already modestly sized dataset. Therefore, we also ran the model on the full dataset, which may suffer from some bias from the non-random placement of Williams Act enrollment but provides more precise estimates due to increased sample size. Finally, we tested alternative caliper sizes to verify that our results are not sensitive to our choice of matched datasets.
Results
Vineyard conversion and rangeland fallowing
The full dataset contained 1,376 parcels (230,639 ha) that were rangeland in 1984 and over 100 acres (40 ha) in size (). Most parcels, 947 (69.9%; 171,017 ha), were enrolled in the Williamson Act. Of all the parcels in our dataset, 171 (12.6%; 31,590 ha) converted to vineyards, 139 of which were in the Williamson Act covering 26,103 ha. Further, of the parcels that converted to vineyards, 118 (69.0% of conversions; 13,809 ha) fallowed grazing even though over 80% of these parcels still have over 100 acres (40 ha) of rangeland remaining. On average, only 18% of land on parcels that converted to vineyards was used to grow grapes. Of the parcels that converted to vineyards, 90.6% of non-Williamson Act parcels and 64% of Williamson Act parcels fallowed grazing.
Table 1. Tabulations of vineyard conversion and graze fallowing by Williamson Act enrollment for the full and matched dataset (in number of parcels).
Matching algorithm
Propensity score matching using a nearest neighbor algorithm without replacement did a good job of alleviating bias between Williamson Act parcels and non-Williamson Act parcels. Of the 10 variables included in the probit regression used to calculate the propensity score, 9 were statistically significant at the 95% level, with only distance to ocean being insignificant. The pseudo r-squared of the probit model was 0.11. After matching, running the same regression with the matched data produces a pseudo r-squared of 0.01, and the full model is insignificant at the 95% level (although significant at the 90% level). Mean bias is reduced for each variable in the regression by at least 35%. After matching, the means of each variable are not significantly different for 9 out of 10 variables (distance to Santa Barbara being the exception; ). Together these results suggest that the matching algorithm sufficiently pre-processes the data such that the matched dataset can act as a quasi-experiment.
Table 2. Comparison of means between matched and unmatched datasets, % bias reduction, T-value and p-statistic.
The matching algorithm produced a dataset with 706 observations and included an even number (353) of parcels enrolled and not enrolled in the Williamson Act. Of these, 706 parcels, 84 (11.8%) converted to vineyards, with 63.1% of conversions coming from Williamson Act properties. Of the 84 conversions, 65 (77.3%) were fallowed. About 90.3% of non-enrolled parcels and 79.8% for enrolled parcels that converted were fallowed.
Econometric results – conversion to vineyards
The results of the selection equation (i.e. which parcels converted to vineyards) showed that conversion decisions were primarily associated with locations that may produce high quality wines while also being accessible to tourist and markets. Areas with high precipitation and areas further from the ocean were more likely to convert to vineyards, while areas far from towns and at high elevations were less likely to convert. Parcel size and past fire history were not statistically significant in our regression results. The Williamson Act had a positive effect on the conversion, although the result was not precisely estimated (p = 0.088; , ).
Table 3. Regression Results for the Heckman model for matched and full dataset. Reported coefficients are the marginal effects (i.e, dy/dx) for each independent variable. These marginal effects can be interpreted as the percentage point change in the probability of conversion or fallowing, for a one unit change in the variable of interest. Standard errors are presented in parenthesis.
Econometric results – rangeland fallowing
Different variables were associated with rangeland fallowing than with conversion to vineyards (). Larger parcels were less likely to fallow grazing. For example, our model predicts that a 50 ha parcel that converted to vineyards had a 87% chance of fallowing, a 100 ha parcel had a 78% chance of fallowing and a 500 ha parcel had a 44% chance of fallowing. Likewise, more rugged parcels were also less likely to fallow grazing, as were parcels further from towns. Parcels that were enrolled in the Williamson Act were less likely to fallow grazing (, ). No other variables were statistically significant predictors of fallowing. Combining the positive effect of the Williamson Act on conversion and negative impact on fallowing once conversion occurs, we found overall that the Williamson Act did not have a statistically significant impact on the probability of fallowing ().
Table 4. Predicted Probability of (1) conversion to vineyard (2) fallowing, given a conversion has happened and (3) Joint probability of coverting and fallowing.
Results of robustness checks
Running the econometric model on the full dataset resulted in similar model outputs (). All signs of significant variables were the same between datasets and the magnitudes of the coefficients were generally similar. As expected, the coefficients in the full dataset model were more precisely estimated since the model used nearly twice as many observations. Models that used alternative calipers for matching (0.04, 0.02) likewise had similar results.
Discussion
Land cover and land use change combine to drive environmental and social outcomes. Here, however, we aimed to de-couple land cover (conversion to vineyards) from land use (rangeland fallowing) in Santa Barbara, CA, in order to analyze their separate patterns and drivers. Our results demonstrate the value of investigating land cover and land use separately and suggest that for land covers that can have a high variety of uses, such as rangelands, researchers should consider ways to identify land use instead of just land cover. This is particularly important when assessing the impacts of land use policies, as in the case of the Williamson Act; here, an analysis of land cover alone does not tell a complete story.
The results of our models of conversion and fallowing generally follow economic intuition. Areas that are well suited for wine production – not too high of elevation, higher precipitation – are more likely to convert to vineyards, as are areas that are close to towns and markets. Likewise, in the fallowing model, larger parcels are less likely to fallow. This makes sense given the generally low productivity of rangelands in Santa Barbara County, where animal stocking rates average only 10–25 acres per animal unit (4–10 ha/AU). In such a setting, grazing livestock is only marginally economically rational and may only be profitable on the largest parcels.
Beyond the economic value of these fallowed areas, grazing also may play an important part in reducing fire risk (Colantoni et al., Citation2020; Davies et al., Citation2015; Leonard et al., Citation2010; Diamond et al., Citation2009; Tsiouvaras et al., Citation1989). We found that rangelands were more likely to experience grazing fallowing if they were located closer to towns, raising the possibility that increased wildfire risk following the loss of grazing may threaten infrastructure and structures including homes, wine tasting rooms, and fermentation equipment. Some of the parcels with the highest predicted probability of grazing fallowing are located around the towns of Santa Ynez, Los Alamos, Lompoc, and Sisquoc (Figure 5), areas with significant wildland-urban interface (Santa Barbara Office of Emergency Management, Citation2017). The loss of grazing may facilitate the spread of future wildfires in these settings that have existing vulnerabilities to fire.
Methodologically, we addressed the dual concerns of non-random application of land use policy and unobserved correlation between the conversion and the fallowing decisions through the use of matching and Heckman’s two-stage sample selection model. While we believe these models do a good job of addressing most sources of bias, our dataset is quite small, and using the matching algorithm reduces it even further. In the matched data, we only have 84 vineyard conversions and 65 fallowed observations. While this is enough observations for model estimation, some of the imprecision of our estimates may simply be due to the small sample size.
From a policy perspective the Williamson Act did not appear to have an overall effect on the likelihood of grazing fallowing. It should be noted that conversions from one agricultural use to another are allowed under the Williamson Act, and therefore conversions to vineyards coupled with grazing fallowing are allowed. However, it is also important to note that the Williamson Act is an area-based policy. That is, the goal of this policy is to keep land area in agriculture. When a small portion of a rangeland parcel is converted to vineyard while grazing is fallowed on the remainder of the parcel, the land area actively used for agriculture is greatly reduced. In this way, grazing removal on Williamson Act lands may be seen as a loophole, one that is difficult to document and thus challenging to enforce from the county’s perspective. Put more directly, our results provide evidence that the structure of the Williamson Act in Santa Barbara County does not adequately protect grazing as a form of agricultural production. At least 27,000 acres (10,926 ha) (or, 6% of all rangeland in Williamson Act) in Santa Barbara County benefiting from Williamson Act taxation relief are not actually in agriculture, i.e. do not have grazing.
In total, we calculate that grazing was removed from over 27,000 (10,926 ha) acres of rangeland as a result of nearby vineyard conversions on the same parcel. Using a conservative average, it takes approximately 20 acres (8 ha) in Santa Barbara County to support a single cow and her calf for 1 year. Tallied over all fallowed lands, this leads to a reduction of over $1 million dollars (4.3%) annually to Santa Barbara’s counties livestock industry (Santa Barbara, Citation2020). Beyond economic impacts, these changes have been part of a larger shift in rural culture, such as the reduction of participation rates in ranching-related organizations or activities that have traditionally supported the industry (e.g. Future Farmers of America, FFA; 4 H; Junior Rodeo; county-based fairs; membership in the Santa Barbara County Cattlemen’s Association; etc.) and an increased focus on the region’s identity as ‘wine country’ (Pikkemaat et al. Citation2009). Rangeland fallowing is just one aspect of an interlocking and complex set of phenomena that are leading to substantial but largely undocumented changes in land use. Examining shifts in land cover alone fails to document other, subtler, but important on-the-ground changes.
Disclosure statement
The authors report there are no relevant financial or non-financial competing interests to declare.
Additional information
Funding
References
- Andersen, M.A., Blank, S.C., LaMendola, T., & Sexton, R.J. (2002). California’s cattle and beef industry at the crossroads. Calif. Agric, 56(5), 152–156. https://doi.org/10.3733/ca.v056n05p152
- Arnold, M.A., Duke, J.M., & Messer, K.D. (2013). Adverse selection in reverse auctions for ecosystem services. Land Econ, 89(3), 387–412. https://doi.org/10.3368/le.89.3.387
- Barbara, S. (2020). Agricultural Production Report.https://content.civicplus.com/api/assets/8fbef220-212b-4724-96d0-8a234f01ac4c?cache=1800
- Bartolome, J.W., Allen-Diaz, B.H., Barry, S., Ford, L.D., Hammond, M., Hopkinson, P., Ratcliff, F., Spiegal, S., & White, M.D. (2014). Grazing for biodiversity in Californian Mediterranean grasslands. Source: Rangelands, 36(5), 36–43 doi:10.2111/Rangelands-D-14-00024.1.
- Brown, G., & Weber, D. (2011). Public Participation GIS: A new method for national park planning. Landsc. Urban Plan, 102(1), 1–15. https://doi.org/10.1016/j.landurbplan.2011.03.003
- Buchman, L.T. (1956). Historical backgrounds of range land use in California. Rangel. Ecol. Manag. Range Manag. Arch, 9(2), 81–86 https://journals.uair.arizona.edu/index.php/jrm/article/viewFile/4711/4322.
- Butsic, V., Lewis, D.J.D.J.J., & Ludwig, L. (2011). An econometric analysis of land development with endogenous zoning. Land Econ, 87(3), 412–432. https://doi.org/10.3368/le.87.3.412
- California Department of Conservation. (2021). Farmland Mapping & Monitoring Program [WWW Document]. URL https://www.conservation.ca.gov/dlrp/fmmp/
- Cason, T.N., & Gangadharan, L. (2004). Auction design for voluntary conservation programs. Am. J. Agric. Econ, 86(5), 1211–1217. https://doi.org/10.1111/j.0002-9092.2004.00666.x
- Colantoni, A., Egidi, G., Quaranta, G., D’Alessandro, R., Vinci, S., Turco, R., & Salvati, L. (2020). Sustainable land management, wildfire risk and the role of grazing in Mediterranean urban-rural interfaces: A regional approach from Greece. Land, 9(1), 21. https://doi.org/10.3390/land9010021
- Conservation Biology Institute. (2021a). Land use and vegetation- santa barbara county [WWW Document]. URL https://sbcblueprint.databasin.org/
- Conservation Biology Institute. (2021b). Data basin [WWW Document]. URL https://databasin.org/
- Davies, K.W., Boyd, C.S., Bates, J.D., & Hulet, A. (2015). Winter grazing can reduce wildfire size, intensity and behaviour in a shrub-grassland. Int. J. Wildl. Fire, 25(2), 191–199. https://doi.org/10.1071/WF15055
- Diamond, J.M., Call, C.A., & Devoe, N. (2009). Effects of targeted cattle grazing on fire behavior of cheatgrass-dominated rangeland in the northern Great Basin, USA. Int. J. Wildl. Fire, 18(8), 944–950. https://doi.org/10.1071/WF08075
- Didonato, J. (2006). CAL-PAC society for range management symposium-grazing for biological conservation-Endangered Amphibian Research within Grazed Grasslands. https://doi.org/10.1074/jbc.M609039200
- Ernoul, L., Wardell-Johnson, A., Willm, L., Béchet, A., Boutron, O., Mathevet, R., Arnassant, S., & Sandoz, A. (2018). Participatory mapping: Exploring landscape values associated with an iconic species. Appl. Geogr, 95, 71–78. https://doi.org/10.1016/j.apgeog.2018.04.013
- Foley, J.A., DeFries, R., Asner, G.P., Barford, C., Bonan, G., Carpenter, S.R., Chapin, F.S., Coe, M.T., Daily, G.C., Gibbs, H.K., Helkowski, J.H., Holloway, T., Howard, E.A., Kucharik, C.J., Monfreda, C., Patz, J.A., Prentice, I.C., Ramankutty, N., & Snyder, P.K. (2005). Global consequences of land use. Science (80-.), 309(5734), 570–574. https://doi.org/10.1126/science.1111772
- Foley, J.A., Ramankutty, N., Brauman, K.A., Cassidy, E.S., Gerber, J.S., Johnston, M., Mueller, N.D., O’Connell, C., Zaks, D.P.M., Tilman, D., Siebert, S., Sheehan, J., Rockström, J., Polasky, S., Monfreda, C., Hill, J., Carpenter, S.R., Bennett, E.M., Balzer, C., … Ray, D.K. (2011). Solutions for a cultivated planet. Nature, 478(7369), 337–342. https://doi.org/10.1038/nature10452
- Haeger, J.W. (2020). Pacific Pinot Noir (Univ of California Press).
- Heckman, Ichimura, H., Smith, J., & Todd, P. (1996). Sources of selection bias in evaluating social programs: An interpretation of conventional measures and evidence on the effectiveness of matching as a program evaluation method. Proc. Natl. Acad. Sci. U. S. A, 93(23), 13416–13420. https://doi.org/10.1073/pnas.93.23.13416
- Hellerstein, D.M. (2017). The US conservation reserve program: The evolution of an enrollment mechanism. Land Use Policy, 63, 601–610. https://doi.org/10.1016/j.landusepol.2015.07.017
- Ho, D.E., Imai, K., King, G., & Stuart, E.A. (2006). Matching as nonparametric preprocessing for reducing model dependence in parametric causal inference. Polit. Anal, 15(3), 199–236. https://doi.org/10.1093/pan/mpl013
- Jones, K.W., & Lewis, D.J. (2015). Estimating the counterfactual impact of conservation programs on land cover outcomes: The role of matching and panel regression techniques. PLoS One, 10(10), e0141380. https://doi.org/10.1371/journal.pone.0141380
- Joppa, L.N., Loarie, S.R., & Pimm, S.L. (2008). On the protection of “protected areas”. Proc. Natl. Acad. Sci. U. S. A, 105(18), 6673–6678. https://doi.org/10.1073/pnas.0802471105
- Karimi, A., & Brown, G. (2017). Assessing multiple approaches for modelling land-use conflict potential from participatory mapping data. Land Use Policy, 67, 253–267. https://doi.org/10.1016/j.landusepol.2017.06.004
- Keeley, J.E., & Fotheringham, C.J. (2001). Historic fire regime in southern California shrublands. Conserv. Biol, 15(6), 1536–1548. https://doi.org/10.1046/j.1523-1739.2001.00097.x
- Keeley, J.E., & Zedler, P.H. (2009). Large, high-intensity fire events in Southern California shrublands: Debunking the fine-grain age patch model. Ecol. Appl, 19(1), 69–94. https://doi.org/10.1890/08-0281.1
- Keeley, J.E., & Syphard, A.D. (2018). South coast bioregion. In J.W. Wagtendonk, N. Sugihara, S.I. Sephens, A.E. Thode, K.E. Shaffer, & J.A. Fites-Kaufman (Eds.), Fire California’s Ecosyst (University of California Press) (pp. 319–351).
- Leonard, S., Kirkpatrick, J., & Marsden-Smedley, J. (2010). Variation in the effects of vertebrate grazing on fire potential between grassland structural types. J. Appl. Ecol, 47(4), 876–883. https://doi.org/10.1111/j.1365-2664.2010.01840.x
- Liu, J., Daily, G., Ehrlich, P., & Luck, G. (2003). Effects of household dynamics on resource consumption and biodiversity. Nature, 421(6922), 530–533. https://doi.org/10.1038/nature01359
- Liu, J., Hull, V., Batistella, M., DeFries, R., Dietz, T., Fu, F., Hertel, T.W., Izaurralde, R.C., Lambin, E.F., Li, S., Martinelli, L.A., McConnell, W.J., Moran, E.F., Naylor, R., Ouyang, Z., Polenske, K.R., Reenberg, A., de Miranda Rocha, G., Simmons, C.S., and Zhu, C. (2013). Framing sustainability in a telecoupled world. Ecol. Soc, 18(2), 26. https://doi.org/10.5751/ES-05873-180226
- Maher, A.T., Quintana Ashwell, N.E., Maczko, K.A., Taylor, D.T., Tanaka, J.A., & Reeves, M.C. (2021). An economic valuation of federal and private grazing land ecosystem services supported by beef cattle ranching in the United States. Transl. Anim. Sci, 5(3), 1–15. https://doi.org/10.1093/tas/txab054
- Meyfroidt, P., Rudel, T.K., & Lambin, E.F. (2010). Forest transitions, trade, and the global displacement of land use. Proc. Natl. Acad. Sci. U. S. A, 107(49), 20917–20922. https://doi.org/10.1073/pnas.1014773107
- Meyfroidt, P. (2016). Approaches and terminology for causal analysis in land systems science. J. Land Use Sci, 11(5), 501–522. https://doi.org/10.1080/1747423X.2015.1117530
- Meyfroidt, P., Roy Chowdhury, R., de Bremond, A., Ellis, E.C., Erb, K.H., Filatova, T., Garrett, R.D., Grove, J.M., Heinimann, A., Kuemmerle, T., Kull, C.A., Lambin, E.F., Landon, Y., le Polain de Waroux, Y., Messerli, P., Müller, D., Nielsen, J., Peterson, G.D., Rodriguez García, V., and Verburg, P.H. (2018). Middle-range theories of land system change. Glob. Environ. Chang, 53, 52–67 doi:10.1016/j.gloenvcha.2018.08.006.
- Nader, G., Henkin, Z., Smith, E., Ingram, R., & Narvaez, N. (2007). Planned herbivory in the management of wildfire fuels. Rangelands, 29(5), 18–24. https://doi.org/10.2111/1551-501X(2007)29[18:PHITMO]2.0.CO;2
- Pikkemaat, B., Peters, M., Boksberger, P., & Secco, M. (2009). The staging of experiences in wine tourism. Journal of Hospitality Marketing & Management, 18(2–3), 237–253.
- Plieninger, T., & Huntsinger, L. (2018). Complex rangeland systems: Integrated social-ecological approaches to silvopastoralism 2 3. Rangel. Ecol. Manag, 71(5), 519–525. https://doi.org/10.1016/j.rama.2018.05.002
- Robinson, N.P., Allred, B.W., Naugle, D.E., & Jones, M.O. (2019). Patterns of rangeland productivity and land ownership: Implications for conservation and management. Ecol. Appl, 29(3), 1–8. https://doi.org/10.1002/eap.1862
- Santa Barbara County. (2021). Mapping and GIS Resources [WWW Document]. URL https://www.countyofsb.org/mapping.sbc
- Santa Barbara Office of Emergency Management. (2017). Santa Barbara County Multi-Jurisdictional Hazard Mitigation Plan.
- StataCorp. (2021). Stata statistical software: release 17 https://www.stata.com/company/.
- Tsiouvaras, C., Havlik, N., & Bartolome, J. (1989). Effects of goats on understory vegetation and fire hazard reduction in a coastal forest in California. Forest Science, 35(4), 1125–1131 doi:10.1093/forestscience/35.4.1125.
- Turner, B.L., Lambin, E.F., & Reenberg, A. (2007). The emergence of land change science for global environmental change and sustainability. Proc. Natl. Acad. Sci, 104(52), 20666–20671. https://doi.org/10.1073/pnas.0704119104
- USDA- Forest Service. (2009). CALVEG. https://www.fs.usda.gov/detail/r5/landmanagement/resourcemanagement/?cid=stelprdb5347192
- USDA National Agricultrual Statistics Service. (2014). Cropland data layer [WWW Document]. URL http://nassgeodata.gmu.edu/CropScape/
- USDA NRCS. (2021). Range and pastureland overview [WWW Document]. URL https://www.nrcs.usda.gov/wps/portal/nrcs/detail/national/landuse/rangepasture/?cid=nrcsdev11_001074
- USDA-NASS. (2017). 2017 census of agriculture- county data Table 11
- Wallace, N.E. (1988). The market effects of zoning undeveloped land: does zoning follow the market? J. Urban Econ, 23(3), 307–326. https://doi.org/10.1016/0094-1190(88)90021-6
- Wolf, K.M., Baldwin, R.A., & Barry, S. (2017). Compatibility of livestock grazing and recreational use on coastal California public lands: importance, interactions, and management solutions. Rangel. Ecol. Manag, 70(2), 192–201. https://doi.org/10.1016/j.rama.2016.08.008