ABSTRACT
Objectives
To identify early indicators for invasive mechanical ventilation utilization among COVID-19 patients.
Methods
This retrospective study evaluated COVID-19 patients who were admitted to hospital from 20 September 2020, to 8 August 2021. Multivariable logistic regression and machine learning (ML) methods were employed to assess variable significance.
Results
Among 1,613 confirmed COVID-19 patients, 365 patients (22.6%) received invasive mechanical ventilation (IMV). Factors associated with IMV included older age >65 years (OR,1.46; 95%CI, 1.13–1.89), current smoking status (OR, 1.71; 95%CI, 1.22–2.41), critical disease at admission (OR, 1.97; 95%CI, 1.28–3.03), and chronic kidney disease (OR, 2.07; 95%CI, 1.37–3.13). Laboratory abnormalities that were associated with increased risk for IMV included high leukocyte count (OR, 2.19; 95%CI, 1.68–2.87), low albumin (OR, 1.76; 95%CI, 1.33–2.34) and high AST (OR, 1.71; 95%CI, 1.31–2.22).
Conclusion
Our study suggests that there are several factors associated with the increased need for IMV among COVID-19 patients. These findings will help in early identification of patients at high risk for IMV and reallocation of hospital resources toward patients who need them the most to improve their outcomes.
1. Introduction
The coronavirus disease 2019 (COVID-19) caused by the novel severe acute respiratory syndrome coronavirus-2 (SARS-CoV-2) is a rapidly emerging disease. The initial cases of COVID-19 disease were reported in December 2019 in Wuhan, China. In March 2020, the World Health Organization (WHO) declared the novel coronavirus (COVID-19) outbreak to be a global pandemic [Citation1]. As of 6 March 2022, there were more than 440 million confirmed cases of COVID-19 globally, leading to more than 5 million deaths [Citation2]. Although many cases appear to be mild, severe consequences such as pulmonary infection, acute respiratory distress syndrome (ARDS), and multi-organ failure may occur. Epidemiological studies have shown that around 14% of patients develop a severe form of the COVID-19 disease, while 5% of patients develop a critical form of the disease [Citation3].
Several studies have examined the association between baseline characteristics and outcomes in patients with COVID-19 and found that age, smoking, male gender and underlying comorbidities (i.e. cardiovascular disease, diabetes mellitus, hypertension, chronic lung disease, cancer and chronic kidney disease) may influence the clinical course during hospitalization [Citation4–6]. Moreover, recent data from the Center for Disease Control and Prevention (CDC) confirmed that many factors including age, gender, and comorbidities are associated with the severity of the disease [Citation7]. However, few studies have examined the risk factors and clinical characteristics associated with the increased need for invasive mechanical ventilation (IMV) among COVID-19 patients.
Given the overwhelming number of admissions of COVID-19 patients to hospitals, COVID-19 has caused an unprecedented burden on healthcare systems, rendering intensive care unit care and ventilator availability focal points of resource allocation and planning. Hence, identifying patients at higher risk for IMV is thus important to optimize the reallocation of hospital resources toward patients who need them the most to improve their outcomes.
2. Patients and methods
2.1. Study design and patients
This retrospective study was conducted at King Abdullah University Hospital (KAUH) in Jordan, which is a tertiary hospital and one of the largest medical structures in the country with a 750-bed capacity.
This study has included all patients with COVID-19 confirmed by nasopharyngeal swabs for SARS-CoV-2 nucleic acid by polymerase-chain reaction (PCR) who were admitted to KAUH between 20 September 2020, and 8 August 2021. Patients who were less than 18 years of age and those with asymptomatic or mild disease were excluded. All data were retrospectively extracted from hospital electronic medical records. This study followed ‘Strengthening the Reporting of Observational Studies in Epidemiology’ (STROBE) reporting guidelines [Citation8]. The study was approved by the Institutional Review Board (IRB) committee of Jordan University of Science and Technology, Irbid, Jordan (27/137/2021).
3. Study variables
3.1. Patient characteristics
Patients’ clinical data, including comorbidities, radiological and laboratory results, as well as the hospitalization course, were extracted from the hospital’s electronic medical records. Other patient characteristics including age, gender, smoking status, height and weight were also included. Body mass index (BMI) was classified according to the WHO classification [Citation9]. Baseline comorbidities were identified based on related International Classification of Diseases (ICD) codes [Citation10]. All laboratory test results were interpreted based on the hospital laboratory reference values. The disease severity status on admission was classified according to the National Institute of Health (NIH) Clinical Spectrum of SARS-CoV-2 Infection [Citation11].
Patients with positive PCR test without showing symptoms were considered ‘asymptomatic’ patients showing signs and symptoms of COVID-19 with no shortness of breath, dyspnea, or abnormal chest imaging were considered having ‘mild illness’. Patients with evidence of lower respiratory disease including shortness of breath and dyspnea and who had an oxygen saturation (SpO2) ≥94% on room air were considered having ‘Moderate illness’. Patients who had SpO2 < 94%, respiratory rate >30 breaths/min, or lung infiltrates >50% were considered having ‘severe illness’. Patients who had respiratory failure, septic shock, or multiple organ dysfunction were considered having ‘Critically illness’ [Citation11].
3.2. Clinical outcomes
The primary endpoint that was assessed in this analysis was the requirement for IMV, defined as delivery of positive pressure to the lungs via an endotracheal tube. Invasive mechanical ventilation use was assessed using ICD-10 procedure codes or Current Procedural Terminology codes [Citation12]. Prevalence of IMV use was defined as the percentage of hospitalized COVID-19 patients who required IMV.
4. Statistical analysis
Analysis involved transforming categorical variables into binary vectors via encoding categorical data using a one-hot approach. The proportion of inpatients with COVID-19 were examined by age group, gender, and clinical characteristics, and results were presented using summary tables. χ2 tests were used to analyze the IMV rates associated with each characteristic, and to evaluate statistical differences in the frequencies of the categorical groups. Univariate logistic regression was used to produce the odds-ratio and statistical significance for each one-hot transformed characteristic. A 2-sided P ≤ .05 was considered statistically significant.
Analysis started with examining the variable importance of each characteristic with the increased IMV rate for COVID-19 inpatients. Following this, variable importance was assessed comprehensively using multivariable logistic and machine learning (ML) methods. We used the following ML methods: Random Forest (RF), Multivariate Adaptive Regression Splines (MARS), K-Nearest Neighbor (KNN), Extreme Gradient Boosting (XGB), and Classification and Regression Trees (CART). A ten-fold cross-validation method was used in this analysis. This was needed to train the ML methods and to extract the combined relative importance of the variables identified in each fold. Based on the number of times the variable appeared across folds and the magnitude of the variable predictive power, the results from the 10 folds were then combined to produce a collective measure of variable importance. The importance measures were scaled between 100 and 0 to combine variable importance across multiple methods and the variables’ importance for all methods were combined into a single importance measure that was presented using a lollipop chart. A multivariable logistic regression model was built and refined, using the reduced set of variables, that could quantify potential risk factors that result in IMV use, adjusted for other confounding characteristics. Variables included in the final multivariable logistic regression model were determined using the backward stepwise variable selection method. A significance level of P < 0.05 was used to retain variables in the final logistic model.
The analysis involved assessing the classification accuracy of the final multivariable logistic model compared to other ML methods by randomly partitioning the data into a training set (70% of data) and a testing set (30% of the data). A stratified method was used to ensure consistency in distributions. We created the Receiver Operator Characteristic (ROC) chart to assess classification accuracy. The curve plots the True Positive Classification Rate versus the False Positive Classification Rate. The area under the curve (AUC) is an aggregate measure of performance across all possible classification thresholds. All analyses were carried out using the 4.1.0 release of the R package.
5. Results
Between 20 September 2020, and 8 August 2021, a total of 1,613 patients, mean age 61.8 years old, with confirmed COVID-19 were admitted to KAUH and were included in this study. Within these hospitalized patients, there were 945 (58.6%) males, 669 (41.5%) were obese and 223 (13.8%) were current smokers. Hypertension was the most common comorbid condition 963 (59.7%), followed by diabetes mellitus 802 patients (49.7%), ischemic heart disease 291 patients (18.0%) and chronic kidney disease (CKD)127 (7.9%). According to disease severity, 865 (53.6%) and 522 (32.4%) patients presented with critical and severe disease, respectively.
Of 1,613 patients, 518 (32.1%) patients were admitted to the intensive care unit (ICU) and 365 (22.6%) received IMV. Detailed demographic and baseline characteristics are shown in . Higher proportions of IMV use were observed among patients who were older than 65 years of age (n = 205, 28.32%; p-value<0.001), current smokers (n = 66, 29.6%; p-value = 0.007) and who presented with critical disease (with acute respiratory distress syndrome (ARDS), septic shock, cardiac dysfunction, or multiple organ dysfunction) (n = 241, 27.9%; p-value<0.001). Additionally, high proportion of IMV use was observed among patients with chronic kidney disease (CKD; n = 47, 37.0%; p-value<0.001).
Table 1. Characteristics, comorbidities, and disease severity for patients with COVID-19 (N = 1,613).
According to laboratory results, among all hospitalized patients, we observed high levels of C-reactive protein (>0.5 mg/L) (CRP; n = 1,129; 70.0%), high D-dimer level (>0.5ug/ml) in 1,192 patients (73.9%), and high lactate dehydrogenase (LDH) (>441.1 U/L) in 1,112 patients (68.9%). However, among patients who required IMV, it was observed that higher proportions of patients had high leucocyte count (>11.0x103/mm3) (n = 154, 36.7%; p-value<0.001), low albumin (<35 g/l) (n = 234, 31.3%; p-value<0.001), and high aspartate aminotransferase (AST) (>40.0 U/L) (n = 181, 30.7%; p-value<0.001). Findings concerning laboratory results among mechanically ventilated patients are shown in .
Table 2. Laboratory characteristics of COVID-19 patients (N = 1,613).
Using the multivariate logistic regression model, it was found that that older age (>65 years) (odds ratio [OR],1.46; 95%CI, 1.13–1.89; p-value = 0.003) and current smoking status (OR, 1.71; 95%CI, 1.22–2.41; p-value = 0.002) were strongly associated with IMV use (). Furthermore, the odds of severity status were almost doubled for IMV use among critical cases (OR, 1.97; 95%CI, 1.28–3.03; p-value = 0.002). The comorbid condition that was significantly associated with IMV use was CKD (OR, 2.07; 95%CI, 1.37–3.13; p-value = 0.001). Additionally, several laboratory tests were identified to be associated with the increased risk for IMV including high leukocyte count (OR, 2.19; 95%CI, 1.68–2.87; p-value<0.001), low albumin (OR, 1.76; 95%CI, 1.33–2.34; p-value<0.001) and high AST (OR, 1.71; 95%CI, 1.31–2.22; p-value<0.001).
Table 3. Multivariate logistic regression results assess the risk factors associated with in-hospital mortality among inpatients with confirmed COVID-19.
shows variable reduction based on the consensus variable importance extracted from multiple ML methods. Logistic regression was then applied to the consensus. The variables selected for the final multiple logistic regression model are highlighted. A sunburst chart with nested rings illustrating the hierarchical breakdown of identified risk factors segmented by patients’ outcome, i.e. IMV versus no IMV, is shown in . The panel of Receiver Operator Characteristic (ROC) charts is shown in and ordered by predictive accuracy. The multivariable logistic regression model has the best predictive classification power among the other methods considered.
Figure 1. Shows variable reduction based on the consensus variable importance extracted from multiple machine learning (ML) methods.
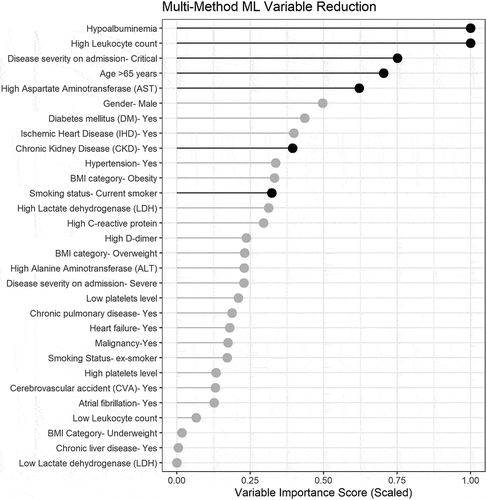
6. Discussion
Our study identified several risk factors associated with the increased need for IMV among hospitalized COVID-19 patients including older age, current smoking status, critical disease on admission, baseline comorbidities, and initial laboratory abnormalities. To our knowledge, this study is the first to examine the clinical characteristics and risk factors associated with the increased need for IMV among COVID-19 patients in the Middle East.
In our study cohort, we observed a high prevalence of IMV use (23.3%) among hospitalized COVID-19 patients. According to recent studies, the rates of IMV utilization among hospitalized COVID-19 patients ranged from 2.3% in one Chinese study to 33.1% in a study from the USA [Citation4,Citation5]. Several studies have found that older age was strongly associated with an increased risk of IMV. Consistent with our findings, a recent study of 10,131 veterans with SARS-CoV-2 infection found that older age was strongly associated with risk of mechanical ventilation (adjusted hazard ratio [aHR] 4.32; 95%CI = 2.88–6.47) [Citation13]. The increased risk of IMV utilization among elderly patients may be attributed to the aging-related chronic pro-inflammatory status of the immune system and the weak immune defense against infections [Citation14]. In addition to that, higher levels of pro-inflammatory cytokines with increasing age may contribute to the development of severe disease [Citation15].
In our study cohort, chronic kidney disease (CKD) was reported in 7.9% of hospitalized COVID-19 patients and was found to be significantly associated with the increased risk of IMV. Consistent with our findings, a systematic review and metanalysis of 73 studies, including 171,108 COVID-19 patients, found that patients with CKD were more likely to develop severe COVID-19 (OR = 1.84; 95% CI, 1.47–2.30) [Citation16]. Furthermore, another study of 210 CKD patients with COVID-19 patients found that CKD patients were at higher risk for intubation and mechanical ventilation [Citation17]. Another study of 7,341 COVID-19 patients showed that CKD was associated with severe clinical outcomes including the need for mechanical ventilation [Citation18]. A possible explanation for these findings is the immune dysfunction in patients with CKD which may lead to increased lung injury [Citation19].
Current smoking status was significantly associated with the increased risk of IMV. Several studies have identified smoking as a risk factor for disease severity and the need for mechanical ventilation. A systematic review and metanalysis of 40 studies including 369,287 patients with confirmed COVID-19 showed that current smoking significantly increases the risk of disease severity (OR = 1.58; 95% CI: 1.16–2.15, p = 0.004) [Citation19]. Another study showed that current smoking status is associated with the increased need for intubation among COVID-19 patients (OR 10.33, p = 0.02) [Citation20].
Other risk factors for IMV use reported in earlier studies did not reach statistical significance in the current analysis including male gender, comorbidities such as hypertension, diabetes mellitus, cerebrovascular accidents (CVA), and chronic liver disease [Citation13,Citation16]. Several studies have reported that the male gender is associated with increased mortality [Citation16]. However, there was no association between gender and need for IMV in this study. Consistent with our findings, a study of 223 critically ill COVID-19 patients showed that no difference was observed in gender in mechanically ventilated patients [Citation21]. Despite the high prevalence of diabetes mellitus and hypertension in our cohort, there was no significant association with IMV identified. A recent systematic review and meta-analysis of 29 studies including 12,258 patients with confirmed COVID-19 infection found that diabetes mellitus (OR: 1.02; 95% CI: 0.94–1.11; p = 0.6027) and hypertension (OR: 1.00; 95% CI: 0.94–1.06; p = 0.9685) were not associated with the increased needs for IMV, which is consistent with our findings [Citation16].
For the laboratory findings, high AST, high leucocyte count and low albumin were significantly associated with increased risk of IMV. Consistent with our findings, a recent study showed that high AST (aHR, 2.92; 95% CI, 2.13–4.02), high total white blood cell count (aHR, 2.34; 95% CI, 1.74–3.14), and low albumin (aHR, 1.90; 95% CI, 1.36–2.67) were significantly associated with the need for mechanical ventilation among COVID-19 patients [Citation13].
To our knowledge, this is the first relatively large population study in the Middle East that shows potential associations between several factors and the need for IMV among patients with confirmed COVID-19 infection. In our analysis, we employed a robust method by combining the machine learning and logistic regression methods to assess for potential associations between several factors and IMV use. However, in this study, the retrospective observational nature of this study might have limited our ability to infer causality. The results of the study would benefit from being replicated and assessed using different populations and healthcare settings. Moreover, lack of association between hypertension and diabetes mellitus and the increased need for IMV should be further evaluated in future studies.
7. Conclusion
Our study indicates that several factors are associated with increased risk of IMV including older age, critical disease status on admission and laboratory biomarkers. Among baseline comorbid conditions, it was found that patients with underlying CKD are at increased risk for IMV. Our study findings may help in early identification of high-risk patients who may need IMV and appropriate reallocation of hospital resources toward patients who need them the most to improve their outcomes. Despite the high prevalence of hypertension and diabetes mellitus in our study cohort, no associations were identified with the risk of IMV. Future studies are required to recognize any potential associations between these comorbidities and risk for IMV and adverse outcomes among COVID-19 patients.
Declaration of interest
The authors have no relevant affiliations or financial involvement with any organization or entity with a financial interest in or financial conflict with the subject matter or materials discussed in the manuscript. This includes employment, consultancies, honoraria, stock ownership or options, expert testimony, grants or patents received or pending, or royalties.
Reviewer disclosures
Peer reviewers on this manuscript have no relevant financial or other relationships to disclose.
Ethical approval
Ethical approval for this study was obtained from the Institutional Review Board (IRB) committee of Jordan University of Science and Technology, Irbid, Jordan (27/137/2021).
Author contributions
Conceptualization, all authors; methodology, S Kabbaha, S Al-Azzam, R Karasneh, BY Khassawneh, A-H Al-Mistarehi, SS Hasan, and MA Aldeyab; validation, S Kabbaha, S Al-Azzam, R Karasneh, BY Khassawneh, A-H Al-Mistarehi, and MA Aldeyab; formal analysis, S Kabbaha, W Lattyak, M Aldiab, and MA Aldeyab; interpretation of data, all authors; writing—original draft preparation, S Kabbaha; writing—review and editing, all authors; All authors critically revised the report and approved the final version to be submitted for publication.
Additional information
Funding
References
- Cucinotta D, Vanelli M. DNA-Protein Crosslinks and Their Resolution. Ann Rev Biochem [Internet]. 2022;91:157. [cited 2022 Mar 16]. Available from: /pmc/articles/PMC7569573/
- World Health Organization. WHO Coronavirus (COVID-19) Dashboard. WHO Coronavirus (COVID-19) dashboard with vaccination data [Internet]. WHO; 2021:1–5. Available from: https://covid19.who.int
- Wu Z, McGoogan JM. Characteristics of and important lessons from the coronavirus disease 2019 (COVID-19) Outbreak in China: summary of a Report of 72 314 cases from the Chinese center for disease control and prevention. JAMA [Internet]. 2020;323:1239–1242. [cited 2022 Mar 16]. Available from: https://pubmed.ncbi.nlm.nih.gov/32091533
- Wang Y, Lu X, Li Y, et al. Clinical course and outcomes of 344 intensive care patients with COVID-19. Am J Respir Crit Care Med [Internet]. 2020;201:1430–1434. [cited 2022 Mar 16]. Available from. https://pubmed.ncbi.nlm.nih.gov/32267160/
- Richardson S, Hirsch JS, Narasimhan M, et al. Presenting characteristics, comorbidities, and outcomes among 5700 patients hospitalized with COVID-19 in the new york city area. JAMA [Internet]. 2020;323:2052–2059. [cited 2022 Mar 16]. Available from: https://pubmed.ncbi.nlm.nih.gov/32320003/
- Chen TL, Dai Z, Mo P, et al. Clinical characteristics and outcomes of older patients with coronavirus disease 2019 (COVID-19) in Wuhan, China: a Single-Centered, Retrospective Study. J Gerontol A Biol Sci Med Sci [Internet]. 2020;75:1788–1795. [cited 2022 Jul 15]. Available from: https://pubmed.ncbi.nlm.nih.gov/32279081/
- People with certain medical conditions | CDC [Internet]. [cited 2022 Mar 16]. Available from: https://www.cdc.gov/coronavirus/2019-ncov/need-extra-precautions/people-with-medical-conditions.html
- STROBE - Strengthening the reporting of observational studies in epidemiology [Internet]. [cited 2022 Mar 16]. Available from: https://www.strobe-statement.org/
- WHO/Europe | nutrition - body mass index - BMI [Internet]. [cited 2022 Feb 3]. Available from: https://www.euro.who.int/en/health-topics/disease-prevention/nutrition/a-healthy-lifestyle/body-mass-index-bmi.
- The web’s free 2022 ICD-10-CM/PCS medical coding reference [Internet]. [cited 2022 Mar 16]. Available from: https://www.icd10data.com/.
- Clinical spectrum | COVID-19 treatment guidelines [Internet]. [cited 2022 Jan 31]. Available from: https://www.covid19treatmentguidelines.nih.gov/overview/clinical-spectrum
- Coding tips for mechanical ventilation | premier medical coding & Consulting [Internet]. [cited 2022 Mar 16]. Available from: https://pmccbilling.com/coding-tips-for-mechanical-ventilation
- Ioannou GN, Locke E, and Green P, et al. Risk factors for hospitalization, mechanical ventilation, or death among 10 131 US veterans with SARS-CoV-2 Infection. JAMA Network Open [Internet]. 2020;3:e2022310. [cited 2022 Mar 16]. Available from: https://pubmed.ncbi.nlm.nih.gov/32965502
- Wu T, Zuo Z, Kang S, et al. Multi-organ dysfunction in patients with COVID-19: a systematic review and meta-analysis. Aging Dis [Internet]. 2020;11:874. [cited 2022 Mar 16]. Available from: /pmc/articles/PMC7390520/
- Jin ZJ, Dong X, Hui LG, et al. Risk and protective factors for COVID-19 morbidity, severity, and mortality. Clin Rev Allergy Immunol [Internet]. 2022;1:1. [cited 2022 Jan 30]. Available from: /pmc/articles/PMC8767775/
- Patel U, Malik P, and Usman MS, et al. Age-Adjusted risk factors associated with mortality and mechanical ventilation utilization amongst COVID-19 Hospitalizations—a systematic review and meta-analysis. Sn Compr Clin Med [Internet]. 2020;2:1740. [cited 2022 Mar 16]. Available from: /pmc/articles/PMC7456201/
- Yamada T, Mikami T, Chopra N, et al. Patients with chronic kidney disease have a poorer prognosis of coronavirus disease 2019 (COVID-19): an experience in New York City. Int Urol Nephrol [Internet]. 2020;52:1405. [cited 2022 Mar 16]. Available from: /pmc/articles/PMC7250261/
- Kang SH, Kim SW, and Kim AY, et al. Association between chronic kidney disease or acute kidney injury and clinical outcomes in covid-19 patients. J Korean Med Sci. [Internet]. 2020;35. [cited 2022 Mar 16. Available from: https://pubmed.ncbi.nlm.nih.gov/33372426/
- Umnuaypornlert A, Kanchanasurakit S, and Lucero-Prisno DE, et al. Smoking and risk of negative outcomes among COVID-19 patients: a systematic review and meta-analysis. Tob Induc Dis. [Internet]. 2021;19. [cited 2022 Mar 16]. Available from: /pmc/articles/PMC7857247/
- Monteiro AC, Suri R, Emeruwa IO, et al. Obesity and smoking as risk factors for invasive mechanical ventilation in COVID-19 respiratory failure: a retrospective, observational cohort study. medRxiv. 2020. [cited 2022 Mar 16]. Available from: /pmc/articles/PMC7430603/
- Roedl K, Jarczak D, Thasler L, et al. Mechanical ventilation and mortality among 223 critically ill patients with coronavirus disease 2019: a multicentric study in Germany. Aust Crit Care [Internet]. 2021;34:167–175. [cited 2022 Mar 16]. Available from: https://pubmed.ncbi.nlm.nih.gov/33250401