Abstract
In this paper, we rigorously analyse an ordinary differential equation system that models fighting the HIV-1 virus with a genetically modified virus. We show that when the basic reproduction ratio ℛ0<1, then the infection-free equilibrium E 0 is globally asymptotically stable; when ℛ0>1, E 0 loses its stability and there is the single-infection equilibrium E s. If ℛ0∈(1, 1+δ) where δ is a positive constant explicitly depending on system parameters, then the single-infection equilibrium E s that is globally asymptotically stable, while when ℛ0>1+δ, E s becomes unstable and the double-infection equilibrium E d comes into existence. When ℛ0 is slightly larger than 1+δ, E d is stable and it loses its stability via Hopf bifurcation when ℛ0 is further increased in some ways. Through a numerical example and by applying a normal form theory, we demonstrate how to determine the bifurcation direction and stability, as well as the estimates of the amplitudes and the periods of the bifurcated periodic solutions. We also perform numerical simulations which agree with the theoretical results. The approaches we use here are a combination of analysis of characteristic equations, fluctuation lemma, Lyapunov function and normal form theory.
1. Introduction
In recent years, mathematical modelling has contributed greatly to the understanding of HIV-1 infection in host and has provided valuable insight into HIV-1 pathogenesis. Among various models is the class by differential equations, which quantitatively describe the dynamics of the HIV-1 virus, healthy and infected cells and even possibly the immune responses. By studying these models, researchers have gained much knowledge about the mechanism of the interactions of these components within a host, and have thereby enhanced the progress in understanding the HIV-1 infection (see, e.g. Citation14–17). Such understanding in turn may offer guidance for developing new drugs and for designing optimal combination of existing therapies (see, e.g. Citation6 Citation10 Citation18 and the references therein).
A standard and classic differential equation model for HIV infection is the following system of ordinary differential equations (ODEs) (see, e.g. Citation10 Citation14 Citation16):
It is known that the HIV-1 virus load is a crucial measurement of the severity of an HIV-1 carrier. When the load exceeds certain level after a clinically latent phase, the CD + T-cell count declines drastically, indicating a transition from HIV to AIDS (see, e.g. Citation5
Citation20). Most of the existing therapies for HIV and/or AIDS employ inhibitors of the enzymes required for replication of HIV-1 virus to reduce the load (see, e.g. Citation3
Citation8). Recent progress in genetic engineering has offered an alternative approach: modification of a viral genome can produce recombinants capable of controlling infections by other virus. Indeed, this method had been used to modify rhabdovirus, including the rabies and the vesicular stomatitis, making them capable of infecting and killing cells previously attacked by HIV-1 (for details, see, e.g. Citation9
Citation13
Citation19
Citation22). To understand this approach of fighting a virus with a genetically modified virus, Revilla and Garcia-Ramos Citation18 proposed a mathematical model which is a result of incorporating into EquationEquation (1) two more variables: the density w of the recombinant (genetically modified) virus and the density z of doubly infected cells.
In Citation18, the authors only analysed the structure of the equilibria of the system Equation(2) and performed some numerical simulations. System Equation(2)
has a dimension higher than 2, and it is well known that for systems with higher dimensions, equilibria cannot determine solutions’ long-term behaviours, and complicated dynamics (periodic solutions and even chaos) may occur which would make the system unpredictable. Therefore, theoretically determining the global dynamics of EquationEquation (2)
is an important yet challenging problem, and this constitutes the purpose of this paper. In Section 2, we will justify the well-posedness of the model by showing the positivity and boundedness of solutions of EquationEquation (2)
and review the existence result on equilibria and the basic reproduction number ℛ0. In Section 3, we show that when
, the disease-free equilibrium is globally asymptotically stable, and when
it is unstable. In Section 4, we prove that there is a δ>0 depending on all parameters except for λ, such that if
, then the single-infection equilibrium exists and is globally asymptotically stable, and when
, this equilibrium loses its stability. Note that
is also the condition for the existence of double-infection equilibrium. In Section 5, we analyse the stability of the double-infection equilibrium E
d and prove that when ℛ0 is slightly larger than 1+δ, solutions of the model converge to the E
d; but when ℛ0 is further increased in some way, E
d loses its stability via Hopf bifurcation, giving rise to stable periodic solutions. This theoretical result confirms what we stated earlier: equilibria cannot fully determine the solution dynamics, and stability analysis is important and indeed necessary. Through a numerical example, we illustrate in Section 6 how to obtain more information about the Hopf bifurcation, including the bifurcation direction, the stability, amplitude and frequency of the bifurcated periodic solution. Such information is crucial for giving good estimates of the virus load and healthy cells’ density in the case that these quantities are periodic in time variable t (i.e. sustained fluctuations), based on which, a therapy is usually determined. It may also provide guidance for designing a optimal clinical sampling strategy, such as the best time intervals of sampling. The approaches we used here are a combination of analysis of characteristic equations, fluctuation lemma, Lyapunov function and normal form theory. Our numerical simulations agree with the theoretical results. In the last section, we summarize the main results and discuss possible modifications of the model.
2. Well-posedness, equilibria and basic reproduction numbers
Since the variables are densities which cannot be negative, one expects that starting from non-negative initial values, the corresponding solution remains non-negative. This can be easily confirmed as below. First, from the first equation of EquationEquation (2), we have
Solutions to EquationEquation (2) also remain bounded. To see this, let
be a non-negative solution. Choose
and
, and let
Model system Equation(2) always has the infection-free equilibrium
. The other two possible biologically meaningful equilibria are
The double-infection equilibrium exists (biologically meaningful) if and only if Q>0 where
In order to analyse local stability of EquationEquation (2) at an equilibrium E, we need to calculate the the Jacobian matrix of EquationEquation (2)
at
as below:
3. Stability of the infection-free equilibrium
For the infection-free equilibrium E=E 0, some fundamental calculations give the corresponding characteristic equation
Indeed, by employing the fluctuation lemma (see, e.g. Citation4), we can prove the global asymptotic stability of the infection-free equilibrium E
0 under the condition . For this purpose, we first introduce some basic notations. For a continuous and bounded function
, let
In Section 2, we have shown that for any initial values , the corresponding solution
remains non-negative and is bounded from above. Therefore, the
and
exist for all these five component functions. By the fluctuation lemma (see, e.g. Citation4), there exists a sequence t
n
with
as n→∞ such that
Summarizing the above results, we have proved the following theorem.
Theorem 3.1
When
the infection-free equilibrium E
0
is globally asymptotically stable implying the virus cannot invade regardless of initial load; when
becomes unstable implying that virus may persist.
4. Stability of the single-infection equilibrium
From Section 3, we know that when ℛ0 increases to pass the value 1, the disease-free equilibrium loses its stability and the single-infection equilibrium E s comes into existence. In this section, we study the stability of E s.
For the local stability of E
s, the characteristic equation of the linearized system of the model Equation(2) at E
s is given by
For the quadratic Equationequation (13), by a similar argument to that for EquationEquation (5)
, we know that the two roots of EquationEquation (13)
have negative real parts if and only if
Indeed, by constructing a Lyapunov function and applying the LaSalle's invariance principle, we can show that when and
is globally asymptotically stable. To this end and for convenience for notation, denote by
and v
s the three positive components of the single-infection equilibrium E
s, that is,
and
. Define
Summarizing the above analysis, we have established the following theorem.
Theorem 4.1
When
and
(equivalently Q<0 or Equation
Equation(17)
holds) hold, then the single-infection equilibrium E
s
is globally asymptotically stable implying that the recombinant virus cannot survive but the pathogen virus can; when
becomes unstable implying that recombinant virus may persist.
5. Stability of the double-infection equilibrium E d and Hopf bifurcation from E d
When (equivalently Q>0 or
), the single-infection equilibrium becomes unstable and there occurs the double-infection equilibrium E
d. Unlike for E
0 and E
s at which the characteristic equations can be factored into product of lower degree polynomials, we are unable to factor the characteristic equation at E
d, and thus cannot determine the stability of E
d by the same way as in Sections 3 and 4. On the other hand, our preliminary simulations show that for certain parameter values satisfying
, solutions of EquationEquation (2)
converge to E
d, while for other parameter values the solutions of EquationEquation (2)
do not converge to E
d; instead they converge to a periodic solution. This observation shows the necessity and importance of some theoretical analyses on the stability of E
d as well as on possible Hopf bifurcation.
For convenience in the following analysis, we first do the following rescalings to reduce the number of parameters:
In order to examine the stability of E
d for EquationEquation (21), we compute the Jacobian matrix of system Equation(21)
as
It is obvious that a 1>0 and a 2>0 for any positive parameter values. Here we apply the Routh–Hurwitz criterion to find the stability of the equilibrium solution E d. The necessary and sufficient conditions for E d to be stable are given by
For Δ3 and Δ4, the signs are not easy to determine for general ℛ0, and hence we use a continuity argument below. At , using EquationEquation (27)
and by direct calculations, we have
Theorem 5.1
There is an R
2>R
1
such that when
the double-infection equilibrium E
d
is asymptotically stable.
When ℛ0 is further increased, Δ1 and Δ2 remain positive, but Δ3 and Δ4 may become negative (so may Δ5 by EquationEquation (29) and a
5>0 as
). The following lemma identifies the order of possible sign switches for Δ3 and Δ4.
Lemma 5.1
If Δ3 and Δ4 can change signs from positive to negative as ℛ0 is further increased after the value R 2 in Theorem 5.1, then Δ4 will change before Δ3 does.
Proof
Assume, for the sake of contradiction, that Δ3 will change sign no later than Δ4 does. Then there exists an R 3>R 2 such that
The following lemma tells that when Δ4 crosses zero, Δ3 must remain positive.
Lemma 5.2
For any
if Δ4=0, then Δ3>0.
Proof
Suppose Δ4=0 at . Then,
The above discussion and the results in Yu Citation24 imply that there are no static bifurcation, Hopf-zero bifurcation, double Hopf bifurcation and double-zero Hopf bifurcation, emerging from the equilibrium solution E d; and the only possibility for E d to lose stability is occurrence of Hopf bifurcation when Δ4 crosses zero from positive to negative as ℛ0 further increases from R 2 (see Theorem 5.1).
In order to show that Hopf bifurcation can occur, we need to show that Δ4 can change sign from positive to negative as ℛ0 further increases after R
1. To this end, we notice that and
, implying that as
while
remains valid (actually, as long as 1/a>dp+bq/c). The above observation suggests considering small values of a and large values of c. Indeed, by EquationEquations (27)
, Equation(29)
and tedious but straightforward expansion, we may obtain
Combining the above and the results in Yu Citation24, we have proved the following theorem.
Theorem 5.2
For some large values of c and small values of a, together with d<d
1
or d>d
2 (d
1, d
2
as given in Equation
Equation(40)) (hence
the double-infection equilibrium E
d
loses its stability through Hopf bifurcation, giving rise to a family of periodic solutions.
By the above theorem, E
d can lose its stability through Hopf bifurcation when ℛ0 is further increased from R
1 in some way. It should be pointed out that the conditions obtained above for Δ4 to change sign (i.e. for large values of c and small values of a) are only sufficient conditions for the requirement , which may be quite conservative. There may be many other choices of the parameters that can satisfy this requirement. For some special choice, we may even find the critical value R
h for ℛ0 precisely at which Hopf bifurcation occurs. This will be illustrated numerically in the following section.
6. Numerical illustrations
In this section, we use a numerical example and some simulations to demonstrate the theoretical results obtained in the previous sections. Due to the larger number of parameters, there are many choices for this purpose. For convenience, we will work on the scaled model Equation(21) instead of the original model Equation(2)
. Throughout this section, we fix
The infection-free equilibrium becomes
For the given parameter values, the coefficients of the characteristic polynomial for E d become
In what follows, we show, via this numerical example, how to obtain more information about the Hopf bifurcation, such as bifurcation direction and stability, magnitudes and periods of the bifurcated period solutions. To this end, we apply the normal form theory and the program using computer algebra system Maple developed by Yu Citation23, and Yu and Huseyin Citation25 to analyse the Hopf bifurcation of system Equation(21) from the critical point
(with other parameters given by EquationEquation (41)
).
The general normal form can be written in polar coordinates as
Let , where μ is small perturbation (bifurcation) parameter, and
By the linear transformation
By Yu and Huseyin Citation25, the coefficients v 0 and τ0 are given by
The solution r¯=0 actually corresponds to the equilibrium solution E
d of EquationEquation (21). A simple linearization of the first equation of EquationEquation (51)
indicates that
) is stable for μ<0, as expected. When μ increases from negative to cross zero, a Hopf bifurcation occurs and the amplitude of the bifurcation periodic solutions is given by the non-zero steady-state solution
We have performed some numerical simulations for EquationEquation (21) by using a fourth-order Runge–Kutta method. We take the parameter values given in EquationEquation (41)
, giving
and
. We choose four different values for d (and so for ℛ0 ):
The simulated time history and phase portraits for the above four cases are shown in , respectively, where the initial conditions are taken as
Figure 1. Simulated time history of system Equation(21) for d=0.21, a=0.93, c=40, b=p=q=5.6 with the initial condition: x(0)=5.0, y(0)=1.0, z(0)=2.0, v(0)=0.5, w(0)=4.0, converging to the stable equilibrium solution E
0.
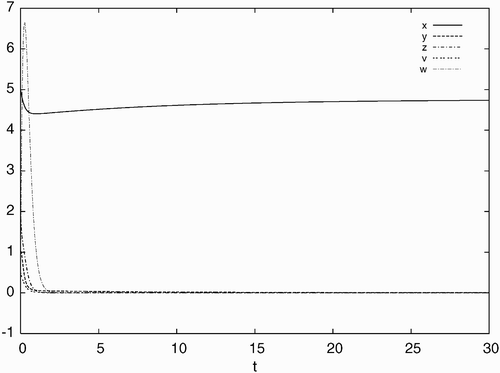
Figure 2. Simulated time history of system Equation(21) for d=0.10, a=0.93, c=40, b=p=q=5.6 with the initial condition: x(0)=5.0, y(0)=1.0, z(0)=2.0, v(0)=0.5, w(0)=4.0, converging to the stable equilibrium solution E
s.
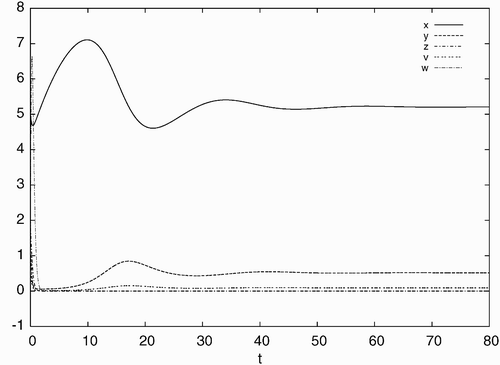
Figure 3. Simulated time history of system Equation(21) for d=0.04, a=0.93, c=40, b=p=q=5.6 with the initial condition: x(0)=5.0, y(0)=1.0, z(0)=2.0, v(0)=0.5, w(0)=4.0, converging to the stable equilibrium solution E
d.
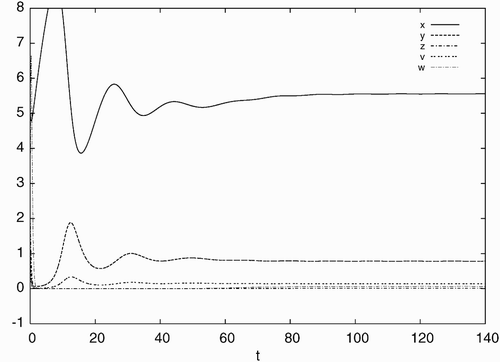
Figure 4. Simulation results of system Equation(21) for d=0.022, a=0.93, c=40, b=p=q=5.6 with the initial condition, x(0)=5.0, y(0)=1.0, z(0)=2.0, v(0)=0.5, w(0)=4.0: (a) time history showing convergence to a stable periodic solution and (b) phase portrait projected on x−y plane indicating a stable limit cycle.
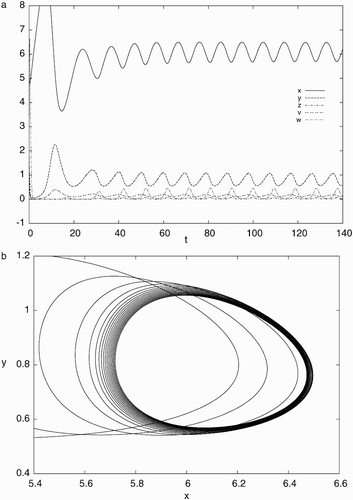
Figure 5. Simulation results of system Equation(21) for d=0.012, a=0.93, c=40, b=p=q=5.6 with the initial condition, x(0)=5.0, y(0)=1.0, z(0)=2.0, v(0)=0.5, w(0)=4.0: (a) time history showing convergence to a stable periodic solution and (b) phase portrait projected on x−y plane indicating a stable limit cycle.
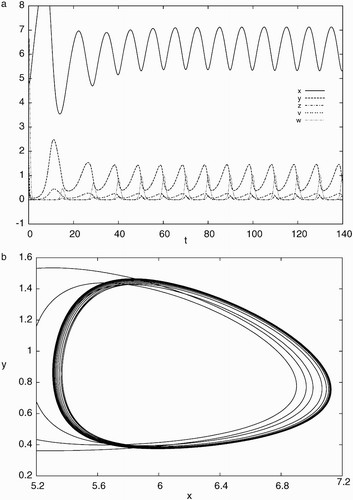
7. Conclusion and discussion
Revilla and Garcia-Ramos Citation18 proposed a model to describe the interaction of HIV-1 virus, a genetically modified virus, healthy T-cells and infected T-cells, and in terms of theory, they only analysed the structure of the equilibria of the model. As we emphasized in Section 1, for a higher-dimensional system, its dynamics cannot be fully determined by the structure of equilibria, and stability analysis is crucial and necessary. In this paper, we have fully analysed the stability of the infection-free equilibrium E 0, the single-infection equilibrium E s and the double-infection equilibrium E d and theoretically proved following:
-
when
, the disease-free equilibrium E 0 is globally asymptotically stable;
-
when
, E 0 becomes unstable and there occurs the single-infection equilibrium E s.
-
when
, E s is globally asymptotically stable;
-
when
(equivalently
), E s becomes unstable, and there is the double-infection equilibrium E d;
-
there is a
, such that E d is asymptotically stable when
;
-
when ℛ0 is further increased in some appropriate ways, E d loses it stability, giving rise to some stable periodic solution via Hopf bifurcation.
The above descriptions reveal the role that each parameter plays in determining the global dynamics of the model and give some quantitative criteria in terms of the parameters for controlling the infection.
Note that EquationEquation (2) is a result of incorporating the variables z and w (recombinant related) into EquationEquation (1)
. It can be easily seen that both EquationEquations (1)
and Equation(2)
share the same basic reproduction number ℛ0, and hence the parameters in z and w equations have no impact on ℛ0. In this sense, introducing the recombinant into to the host does not help completely eliminate the HIV virus. However, by comparing the healthy CD4+ T-cell populations and the wild HIV virus loads in the single-infection equilibrium
and the double-infection equilibrium
, we can see that the recombinant can increase the healthy cell populations and reduce the virus load. To see this, assume
. Then large c and small k will guarantee that ℛ0 is slightly large than
, so that the double-infection equilibrium is asymptotically stable. Now simple calculations show the condition
implies (is indeed equivalent to)
There have been various models for HIV drug therapies. Comparing the model Equation(2) for the generic therapy with existing models for drug therapies, one finds that the latter is much simpler, because they are usually the result of replacing a parameter by another one reflecting the drug efficacy. For example, in considering the effectiveness of a reverse transcriptase inhibitor, the usual way is to replace the parameter β in EquationEquation (1)
by
(see, e.g. Citation16), leading to
We point out that Citation18 only numerically explored solution behaviour of model Equation(2). Now after some rigorous analysis, we have obtained conditions in terms of the model parameters on the stability of the equilibria
and E
d, given by the descriptions Equation(1)–(5) above. Moreover, the description Equation(4)
above (or Theorem 5.2) identifies an important class of solutions: periodic solutions. Considering the fact that the sustained fluctuation of the virus load and/or the healthy cells’ population in vivo will make the clinic measurements of these two quantities less reliable, this finding is of particular theoretical and practical significance. For example, without excluding the period dynamics, a very lower load of virus in the clinic sampling may right be the value of a periodic solution at the lowest moment and hence does not imply that virus is dying out. In order to avoid misleading and to make good use of clinical data in such a periodic situation, information about the frequencies and magnitudes of the oscillating densities is necessary. Through a numerical example, we have demonstrated how to obtain such information. Knowledge on the periods of oscillation of solutions may help design optimal clinical sampling strategy, e.g. the time interval for sampling.
The interaction of HIV virus and T-cells is a complicated process, which involves cell production, virus attachment to the cells and penetration into the cells, virus replication inside cells and release from cells. Now with a recombinant virus added in, the process becomes more complicated. The model we consider here is just a simple one, and there is more room to improve and expand the model. For example, one may consider the situation when there is an external source of recombinant virus. One may also consider the situation when the recombinant virus also infects susceptible cells but at a lower rate (this may occur due to possible mutation of the recombinant virus). One may also incorporate the infection latent into the model, as in Citation11 Citation12, that leads to models of delay differential equations. Such modifications should more precisely describe the reality and give us more insights into the infection process, but would lead to much more challenging mathematical problems.
Acknowledgements
This research was supported by NSERC of Canada, PREA of Ontario and by China Scholarship Council.
References
- Castillo-Chavez , C. and Thieme , H. R. 1995 . “ Asymptotically autonomous epidemic models ” . In Mathematical Population Dynamics: Analysis of Heterogeneity, I. Theory of Epidemics , Edited by: Arino , O. 33 – 50 . Canada : Wuerz, Winnipeg .
- Gantmacher , F. R. 1959 . The Theory of Matrices , Vol. 2 , New York : Chelsea .
- Haase , A. T. 1999 . Population biology of HIV-1 infection: virus and CD+ 4 T cell demography and dynamics in lymphatic tissues . Ann. Rev. Immunol. , 17 : 625 – 656 .
- Hirsch , W. M. , Hanisch , H. and Gabriel , J.-P. 1985 . Differential equation models of some parasitic infections: methods for the study of asymptotic behaviour . Comm. Pure Appl. Math. , 38 : 733 – 753 .
- Janeway , C. and Travers , P. 2005 . Immunobiology: The Immune System in health and Disease , New York : Garland .
- Kepler , T. and Perelson , A. 1998 . Drug concentration heterogeneity facilitates the evolution of drug resistance . Proc. Natl. Acad. Sci. USA , 95 : 11514 – 11519 .
- LaSalle , J. P. 1976 . The Stability of Dynamics Systems , Philadelphia : SIAM .
- Levy , J. 1998 . HIV and the Pathogenesis of AIDS , Washington, DC : AMS .
- Mebatsion , T. , Finke , S. , Weiland , F. and Conzelmann , K. 1997 . A CXCR4/CD4 pseudotype rhabdovirus that selectively infects HIV-1 envelope protein-expressing cells . Cell , 90 : 841 – 847 .
- Nelson , P. , Mittler , J. and Perelson , A. 2001 . Effect of drug efficacy and the eclipse phase of the viral life cycle on estimates of HIV-1 viral dynamic parameters . J. Acquir. Immune. Defic. Syndr. , 26 : 405 – 412 .
- Nelson , P. , Murray , J. and Perelson , A. 2000 . A model of HIV-1 pathogenesis that includes an intracellular delay . Math. Biosci. , 163 : 201 – 215 .
- Nelson , P. and Perelson , A. 2002 . Mathematical analysis of delay differential equations for HIV . Math. Biosci. , 179 : 73 – 94 .
- Nolan , G. P. 1997 . Harnessing viral devices as pharmaceuticals: fighting HIV-1s fire with fire . Cell , 90 : 821 – 824 .
- Nowak , M. and May , R. 2000 . Virus Dynamics , Oxford : Oxford University .
- Perelson , A. , Kirschner , D. and De Boer , R. 1993 . Dynamics of HIV infection of CD4 + T cells . Math. Biosci. , 114 : 81 – 125 .
- Perelson , A. and Nelson , P. 1999 . Mathematical models of HIV dynamics in vivo . SIAM Rev. , 41 : 3 – 44 .
- Perelson , A. , Neumann , A. , Markowitz , M. , Leonard , J. and Ho , D. 1996 . HIV-1 dynamics in vivo: Virion clearance rate, infected cell life-span, and viral generation time . Science , 271 : 1582 – 1586 .
- Revilla , T. and Garcia-Ramos , G. 2003 . Fighting a virus with a virus: a dynamic model for HIV-1 therapy . Math. Biosci. , 185 : 191 – 203 .
- Schnell , M. J. , Johnson , E. , Buonocore , L. and Rose , J. K. 1997 . Construction of a novel virus that targets HIV-1 infected cells and control HIV-1 infection . Cell , 90 : 849 – 857 .
- Stilianakis , N. I. and Schenzle , D. 2006 . On the intra-host dynamics of HIV-1 infections . Math. Biosci. , 199 : 1 – 25 .
- van den Driessche , P. and Watmough , J. 2002 . Reproduction numbers and sub-threshold endemic equilibria for compartmental models of disease transmission . Math. Biosci. , 180 : 29 – 48 .
- Wagner , E. K. and Hewlett , M. J. 1999 . Basic Virology , New York : Blackwell .
- Yu , P. 1988 . Computation of normal forms via a perturbation technique . J. Sound Vib. , 211 : 19 – 38 .
- Yu , P. 2005 . Closed-form conditions of bifurcation points for general differential equations . Int. J. Bifurcation Chaos Appl. Sci. Eng. , 15 : 1467 – 1483 .
- Yu , P. and Huseyin , K. 1988 . A perturbation analysis of interactive static and dynamic bifurcations . IEEE Trans. Automat. Control , 33 : 28 – 41 .