Abstract
We investigate two cases of a sharp change of incidencec functions on the dynamics of a susceptible-infective-susceptible epidemic model. In the first case, low population levels have mass action incidence, while high population levels have proportional incidence, the switch occurring when the total population reaches a certain threshold. Using a modified Dulac theorem, we prove that this system has a single equilibrium which attracts all solutions for which the disease is present and the population remains bounded. In the second case, an increase of the number of infectives leads to a mass action term being added to a standard incidence term. We show that this allows a Hopf bifurcation to occur, with periodic orbits being generated when a locally asymptotically stable equilibrium loses stability.
Keywords:
1. Introduction
One of the most critical aspects in modelling disease propagation is the choice of the incidence function, which describes the rate at which new infectives are produced. If there are S susceptible individuals and I infective individuals in a population, then the rate of creation of new infectives typically involves a product of terms involving S and I. The most common incidence functions are mass action SI, proportional incidence SI/N, where N is the total population, and general incidence S p I q , but there are many other functions that have been used. There has been a debate for quite some time about the nature of the functions to use; see, for example, McCallum et al. Citation9 and the references therein. Most studies conducted to this day assume that the form of the incidence function is fixed for a given model. In models where there are several groups, parameters of the incidence function can vary from group to group, but the general nature of the function is unique. In Fromont et al. Citation3, a model is introduced where different geographical locations have different types of incidence functions; in this case, this is justified by the fact that some of the locations are farms, others are villages, leading to different contact structures. In single populations Citation15, a number of SI and SIS models are formulated with various types of demographic components, with a smooth, density-dependent incidence function. Further mathematical results on some of those models are provided in Zhou Citation14. In Brauer Citation1, the incidence takes the form β(N)SI, with β(N) differentiable. In Zegeling and Kooij Citation13, an SI model is studied for micro- and macro-parasitic diseases, with strong dependence of the incidence on the population size. In Liu et al. Citation8, an incidence function that takes into account the number of hospitalized infectives is used; in both Liu et al. Citation8 and Zegeling and Kooij Citation13, it is shown that such dependence leads to oscillations. See also, for instance, Citation4 Citation10 Citation11.
In the present paper, we consider an SIS model for the spread of an infectious disease in a single population. These models, with the population split between susceptible and infective individuals, constitute the most elementary description of the spread of a disease in a population, and have been extensively studied in the literature. However, we suppose that there is a population level across which the contact structure is fundamentally modified. Hence, contrary to models with varying contact levels Citation1, there is a sharp threshold where the nature of the incidence function itself changes. A similar situation was considered in Kribs-Zaleta Citation7, with logistic dynamics.
We consider here an SIS model in an exponential population dynamics framework, with no self-regulation effects due to intra-specific competition (crowding). Thus, if the birth rate is too low or too high, the population can go extinct or become unbounded. In Section 2, we consider this general model with unspecified incidence and establish some elementary properties.
We then specialize this framework to two different incidence functions with switching.
The first case we consider, in Section 3, has the same incidence form as in Kribs-Zaleta Citation7. The switching occurs when the total population reaches a certain threshold, with mass action incidence for small populations and standard incidence for large populations. This can model a global (and abrupt) modification of the contact structure for high population levels. To study the stability of the endemic equilibrium, we use an extension of Dulac's theorem that is developed in Appendix A.
The second switching scenario addresses the situation where treatment facilities are overcome. At low incidence levels, standard incidence is used; when a certain threshold number of infectives is reached, a mass action type term is added to the incidence function. This describes the situation where, for example, a community has a limited number of hospital beds. When this capacity is overcome, infectives are treated at home rather than in hospital, where their contact pattern is more prone to the propagation of the disease. This change in the incidence is manifested in the incidence function; if the number of infectives is below a threshold value then the incidence is given by proportional mixing, whereas the additional incidence due to any amount of infectives above the threshold is of mass action form. We formulate and analyse this model in Section 4, studying in particular the occurrence of periodic solutions.
We now call attention to an important difference in the dynamics exhibited by the two models. For the second model, it is possible to have a Hopf bifurcation, whereas this cannot happen for the first model.
2. The general framework
We make the following assumptions. There is no vertical transmission of the disease, so all newborns are susceptibles; birth occurs with rate constant b>0, proportional to the total population. The mortality coefficient for non-disease-related reasons is d>0. Infective individuals are subject to disease-induced death, which occurs with rate constant δ≥0. Infective individuals may recover after an average duration of infection of 1/γ time units. Finally, contacts between susceptible and infective individuals result in new infection with rate F(S, I). We thus consider the following SIS epidemic model,
2.1. Equilibria
Disease-free equilibria are obtained by setting I=0 in Equation (1). This yields the extinction equilibrium (S, I)=(0, 0), which is present for all parameter values; whereas, if b=d, then (S, I)=(S, 0) is an equilibrium for any S>0. In this paper, we assume that b≠d and so we ignore this latter case.
From EquationEquation (2), endemic equilibria (EEP), that is, equilibria (S*, I*) such that I*>0, can only exist if
, and must satisfy
Proposition 1
A necessary condition for the SIS model (1) to have endemic equilibria is that
2.2. The system in proportions
In order to simplify the problem, we consider from now on the system in proportions. We let
The system under consideration is then
Under the dynamics of Equation (4), i(t) remains in [0, 1] for all positive times since f is zero if i is 0 or 1. If a solution starts with i(0) less than or equal to one, then i(t) is strictly less than one for all positive time. Also, if N(0)≥0, then N(t) remains non-negative for all time, and satisfies , giving the following result.
Proposition 2
Solutions to system (4) beginning in the positively invariant rectangular half-strip of the (i, N)-plane defined by
Equation Equation(4b) is separable, yielding
-
If b<d, then
for some
.
-
Since i(t)≤1 for all t,
, and it follows that if b>d+δ, then
for some
.
The following result has been proved.
Proposition 3
If b<d, then solutions of Equation (4) satisfy
. If b>d+δ and N(0)>0, then solutions of Equation (4) satisfy
.
The Jacobian matrix associated with system (4) will often be used. At an arbitrary point (i, N) for which f is differentiable, the Jacobian is given by
3. The model with switching on population density
The novelty of the model discussed here lies in the incidence function F. In this section, we assume that the incidence is a continuous function of the form
3.1. The system in proportions
The incidence function f for the system in proportions is as follows:
3.2. Exact solution in 𝒟 H
Let us first remark that the nature of those parts of a solution of Equation (4) that lie in 𝒟 H are known. Indeed, we have the following theorem.
Proposition 4
Suppose that, at some instant τ≥0, we have
. Then there exists a potentially infinite interval ℐ with left endpoint τ such that, for all
, we have
with
Proof
Assume that for some τ≥0. Then consider the initial value problem consisting of Equation (9) together with the initial condition
and
. Integration of Equation Equation(9b)
gives, for t≥τ,
There are two ways for a solution curve to intersect 𝒟
H
. It can start in 𝒟
H
, that is, correspond to an initial condition in 𝒟
H
, or it may correspond to a switching of incidence functions because N=1 is crossed as N increases. To take this information into account, we introduce the sequence {t
k
} of switching times, which we define as follows. For k=1, 2, …, the are the times at which there is a change in EquationEquation (6)
, that is, when N crosses the value 1, with
if there is no such crossing for t>t
k
. We take t
0=0 to be the initial time; so N(t
0) is the initial condition. For subsequent t
k
, N(t
k
)=1. So if the solution enters 𝒟
H
at t
k
, then the integral Equation(11)
can be written as follows:
3.3. Local stability analysis
Theorem 1
For all values of the parameters, the disease-free equilibrium is given by
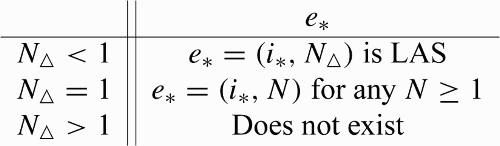
Proof
For all parameter values, e
0 is an equilibrium of the lower system. The point e
* is an equilibrium of the lower system if , guaranteeing that i
*∈(0, 1), and N
*<1. A third solution to the equilibrium equations for the lower strip exists, but it is not biologically relevant since it occurs at
, which lies outside of 𝒟. The higher system (9) only yields equilibria for which N
*>1 if
. In this case, there is a continuum of equilibria of the form
for N>1. However, the condition
is satisfied with probability zero, and so these equilibria are not considered to be biologically significant.
Evaluating the Jacobian matrix Equation(5) at e
0, we have
In the case b<d, Theorem 3 implies that N(t)→0 as t→∞. Now rewrite Equation Equation(8a) as follows:
For , the sign pattern of J(e
*) is
3.4. Ruling out periodic solutions
Note that under the flow (4), the set {i=0} is positively invariant, as is the set {N=0}. Also, when i=1. This implies that any periodic solution of Equation (4) is bounded away from {i=0}, {N=0} and {i=1}, that is, any periodic solution lies entirely in the interior of 𝒟.
We apply Theorem 6 (Appendix 1), taking , with
,
and
. Then hypotheses (H1) and (H2) in Appendix 1 are satisfied.
We use the Dulac function getting
Theorem 2
Suppose
. If
, then each bounded solution in the interior of 𝒟 limits to the endemic equilibrium e
*. If
, then there are no bounded solutions in the interior of 𝒟.
We note that if i=0 is attracting for N>1, then for d<b there are solutions for which i tends to zero and N tends to infinity. Thus, the presence of e * does not guarantee that it is globally stable.
This is distinct from the behaviour of the system studied in Kribs-Zaleta Citation7, where the endemic equilibrium can be shown (using the extended Dulac theorem in Appendix 1) to be globally stable whenever it is present. The difference in dynamics stems from the fact that the model in Kribs-Zaleta Citation7 includes logistic demographics which prevents solutions from being unbounded.
4. A model where infection overcomes treatment facilities
In this section, we consider the case where instead of switching for a given value of N, the switching occurs for a given value of I.
We want to model a situation, such as would occur in the event of overflow of treatment capacities. Suppose that a given population, say a city, has a certain number of hospital beds available, and that the disease under consideration requires hospitalization. While the number of infectives remains lower than the number of beds, infectives are cared for in the hospital, in what can be considered ideal conditions: their contacts with susceptibles from the general population are much less frequent. Then, as the epidemic progresses, there is a point where not enough beds are available, and some patients are sent home. In this situation, the contact pattern is much less favourable, with some infectives mixing more freely in the population (or, at least, in a less controlled setting). We suppose that the incidence function takes the form
Note that this is a toy model. It is generally assumed (as is done, for example, in Section 3) that proportional incidence is more appropriate to describe high population densities. Here, however, we want to focus on the effect of a sudden change in the contact pattern that results in the contacts becoming much more frequent. Hence the form chosen for EquationEquation (15).
The basic analysis is very similar to the case of Section 3, and many results can be adapted.
4.1. The system in proportions
We consider the system in proportions, and so the incidence function is given by
One difference with the case of switching on the population density lies in the nature of the low and high strips, 𝒟 L and 𝒟 H . Here, the boundary Γ is given, for i∈(0, 1], by the hyperbola N=ˆ I/i. Once the strips are thus defined, some of the analysis of Section 3 carries through to the present model with little adaptation.
Note that Theorem 4 holds in 𝒟 L , so that an explicit expression of the solution can be found in the lower strip.
In the lower strip 𝒟
L
, there are two nullclines. The i nullcline, resulting from Equation Equation(17a), is given by
Evidently, if i
LS=i
N
, then there is a continuum of equilibria in the lower strip. However, the set of parameters for which this happens has measure zero in parameter space, and we choose to ignore this situation. So the equilibria are e
0=(0, 0), ,
), when
.
Theorem 3
System (4) with incidence function
Equation(16)
potentially has three equilibria, whose existences and stabilities are summarized in the following table
where
Proof
The equilibrium e
* does not exist if i
N
does not belong to (0, 1), hence the cases for e
*. (e
* might not exist even when i
N
∈(0, 1), which is treated in Theorem 4). The extinction equilibrium e
0=(0, 0) always exists. At e
0, the Jacobian matrix Equation(5) takes the form
Note that ℰ resembles the classical basic reproduction number ℛ0 in its interpretation. Indeed, is the mean time spent in the infective class, if natural death is replaced with birth as is the case when working in proportions. Multiplied by the rate β1 at which individuals enter the infective class, this gives an estimation of the average number of new infections produced by an infective individual. However, for b<d, as ℰ passes through 1, the stability merely passes rom one equilibrium with N
*=0 to another. Since this role is very different from the classical role of ℛ0, we use a different notation.
From now on, we choose to consider only the cases when all three nullclines exist in the interior of 𝒟, that is, . There are three cases to consider, which are shown in . The following theorem summarizes these cases.
Theorem 4
Suppose that
. Then, for system (4) with incidence function
Equation(16)
, e
0
is unstable, and the stability of ē and the existence and stability of e
*
are summarized in the table where
Figure 1. Phase plane situations when the incidence function takes the form Equation(16), with the equilibria represented (circles are unstable, squares are stable). In each panel, the switching curve is the monotone decreasing curve, the nullcline for N′ is given by i=i
N
, and the nullcline for i′ is the curve which has i=1 as an asymptote (given by N=N
HS(i)).
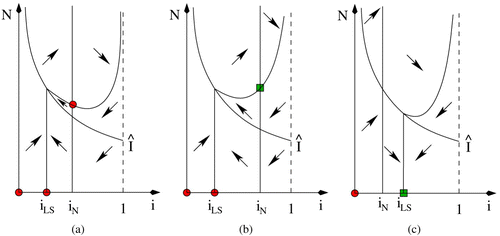
Proof
That e
0 is unstable is established in Theorem 3. When evaluated at and to the left of i
N
(i.e. when i
LS<i
N
), the sign pattern of the Jacobian Equation(5)
is
Interestingly, when it exists the stability of e * is thus determined by the sign of the slope of the tangent to the nullcline N HS at e *. In fact, we show in the next section that when the sign of this slope changes, a Hopf bifurcation occurs at e *.
4.2. Existence of periodic solutions
Theorem 4 states that the loss of stability of e
* occurs when 𝒮
N
changes sign. The following result relates this change in stability to a Hopf bifurcation. We treat 𝒮
N
as a bifurcation parameter, noting that by using β2, we may consider 𝒮
N
to be independent of b, d, δ, i
LS and i
N
. In the theorem statement and proof, denotes the open ball centred at e
* with radius ε.
Theorem 5
Suppose that
and i
LS<i
N
. Then for any ε>0 and any
, there exists
such that system (4) with incidence function
Equation(16)
has a non-trivial periodic orbit in
for
.
Proof
Using Lemma 1 in Appendix 2 with , we see that the eigenvalues of the Jacobian matrix at e
* are purely imaginary conjugates when
, and the real part of these eigenvalues decreases with 𝒮
N
in a neighbourhood of
. By using 𝒮
N
as a bifurcation parameter (or β2 as a proxy for 𝒮
N
), the transversality as it passes through 0 is immediate. It follows that a Hopf bifurcation occurs at
Citation5. In particular, e
* is attracting for
and is repelling for
. The Hopf bifurcation is occurring at e
* as 𝒮
N
passes through 0, but always with the underlying assumption that i
LS<i
N
. Since 𝒮
N
is the derivative of N
HS(i) at i=i
N
(as is the case at e
*), it follows that the bifurcation is happening in the interior of the high strip where the vector field is C
3. Any points of non-differentiability on the periodic orbit are the result of further (minor) bifurcations as the expanding periodic orbit crosses the switching curve. It remains to be shown that periodic orbits exist for negative 𝒮
N
. As seen in , any solution for which N(0) is sufficiently large begins in one of the Regions I, II, III or IV (where the divisions between the regions are the isoclines and the switching curve). In any case, the solution will eventually advance to Region IV. In Region IV, however, N is bounded below by N
1. Then, after leaving Region IV, the N coordinate of the positive semi-trajectory is bounded above by N
2. Thus, solutions are bounded and do not approach e
0 or ē. However, for 𝒮
N
negative, e
* is repelling. Thus, by the Poincaré–Bendixson Theorem, the omega limit set of a solution starting near e
*, or in Regions I, II, III or IV, must be a periodic orbit. In fact, aside from e
*, any solution for which i(0) and N(0) are non-zero must limit to a periodic orbit. ▪
Figure 2. Phase plane diagram showing the nullclines, directions (empty headed arrows) as well as two solutions (filled head arrows), for the system with incidence function Equation(16), in parameter regions where a periodic solution exists.
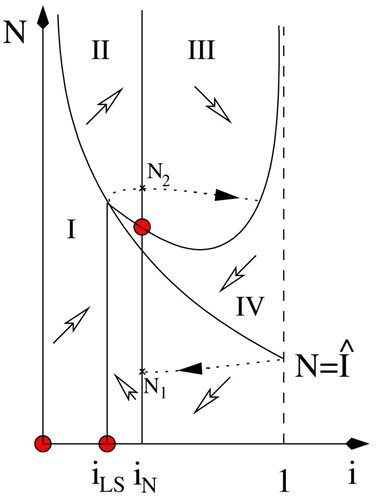
In , the parameters are 1/b=20 years, 1/d=80 years, , γ=1/3, β1=0.4 and
. The initial population is N(0)=1000, with one infective individual, and the switching occurs at ˆ I=150.
Figure 3. (a) Phase plane diagram showing the nullclines (dashed curves), switching boundary Γ (dash-dotted curve), as well as one solution along the hysteresis loop, for the system with incidence function Equation(16). Parameters are indicated in the text. (b) A solution along the hysteresis loop in the case of . The left vertical axis shows the proportion of infectives in the population, corresponding to the solid curve, while the right vertical axis shows the total population, corresponding to the dashed curve.
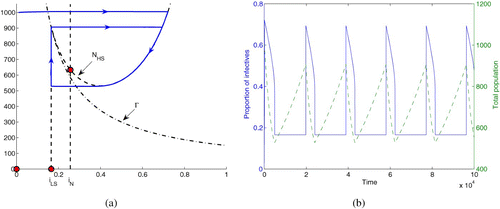
Note () that the periodic orbit intersects both the lower and the upper strips. This is the situation after a so-called grazing bifurcation Citation2, where the periodic orbit whose existence is established in Theorem 5 hits a smooth switching boundary.
5. Discussion
The model we introduce here is, in essence, quite different from other models that were published on the subject. It does share some common properties with other models with exponential demography and disease induced death, in which two extreme cases occur when the birth rate is either too low or too high. For b<d, the population size limits to zero and extinction is inevitable, independent of the disease dynamics. For b>d+δ, the population size grows without bounds. In this case, it is impossible for the disease to limit the population growth. In between these two extreme cases is a region where the disease persists.
For the model with switching on the population density of Section 3, if the population does not become extinct because of excessive disease-induced death, then the disease remains endemic. This result could be foreseen: we assume that the system switches between two well-studied vector fields, with the upper vector field having no equilibrium per se. To show that the endemic equilibrium attracts all bounded solutions, we use an extension of Dulac's theorem for switching vector fields developed in Appendix 1.
A different situation arises in the model of Section 4, with switching on the number of infectives. An analysis of the phase plane, for the case when , shows that there are three major configurations. In each of them, there are two equilibria where the population becomes extinct, one that is always unstable, and one whose local stability depends on the sign of an ℛ0-type quantity. In two of the configurations, there also exists an endemic equilibrium. We show that either this endemic equilibrium is locally asymptotically stable, or it is unstable and there is a periodic orbit. In Zhou and Hethcote Citation15, periodic orbits are not present. There are several major differences between the model presented here and that of Zhou and Hethcote Citation15, so pinpointing one single cause for these oscillations is hard. However, it is likely that the single most important difference between the models lies in the fact that in Citation15, the incidence function is a non-decreasing function of the total population, whereas here it is a function of the infective population. Note that the expression of 𝒮
N
suggests that oscillations occur when β2 and ˆ I are rather large. This means that in order to observe oscillations, the prevalence of the disease in the population and the ‘cost’ of being treated outside the hospital must both be quite high. If, as in Zhou and Hethcote Citation15, incidence depended on the total population, then prevalence cannot reach such high levels as it does here.
It is clear that the period of the oscillations obtained in Section 4 are unrealistic (e.g. 120 years in the case of ). However, a more thorough exploration of the parameter space may reveal areas where such behaviours occur with more realistic frequencies. The models we develop here are toy models; SIS models are seldom used in real case studies, because of their simplicity. More realistic SEIRS models would most likely exhibit the same type of behaviour as our models here, if the same incidence functions were used, with perhaps more realistic oscillation periods. Furthermore, the oscillations we observe seem quite robust to the type of incidence functions used, provided the switching occurs as I passes through some threshold. For example, another way to model the saturation of treatment resources of Section 4 would be to use an incidence of the form
Acknowledgements
The authors thank two anonymous referees for helpful suggestions. The work of both the authors was supported in part by NSERC. The work of J.A. is supported in part by MITACS.
References
- Brauer , F. 2006 . Some simple epidemic models . Math. Biosci. Eng. , 3 ( 1 ) : 1 – 15 .
- di Bernardo , M. , Budd , C. J. , Champneys , A. R. and Kowalczyk , P. 2008 . Piecewise-smooth Dynamical Systems , Berlin : Springer .
- Fromont , E. , Pontier , D. and Langlais , M. 2003 . Disease propagation in connected host populations with density-dependent dynamics: The case of the Feline Leukemia Virus . J. Theor. Biol. , 223 : 465 – 475 .
- Gao , L. Q. and Hethcote , H. W. 1992 . Disease transmission models with density-dependent demographics . J. Math. Biol. , 30 ( 7 ) : 717 – 731 .
- Hale , J. K. and Koçak , H. 1991 . Dynamics and Bifurcations , New York : Springer-Verlag . Texts in Applied Mathematics Vol. 3
- Jeffries , C. , Klee , V. and van den Driessche , P. 1987 . Qualitative stability of linear systems . Linear Algebra Appl. , 87 : 1 – 48 .
- Kribs-Zaleta , C. 2004 . To switch or taper off: The dynamics of saturation . Math. Biosci. , 192 : 137 – 152 .
- Liu , R. , Wu , J. and Zhu , H. 2007 . Media/psychological impact on multiple outbreaks of emerging infectious diseases . Comput. Math. Methods Med. , 8 ( 3 ) : 153 – 164 .
- McCallum , H. , Barlow , N. and Hone , J. 2001 . How should pathogen transmission be modelled? . Trends Ecol. Evol. , 16 ( 6 ) : 295 – 300 .
- Mena-Lorca , J. and Hethcote , H. W. 1992 . Dynamic models of infectious diseases as regulators of population size . J. Math. Biol. , 30 : 693 – 716 .
- Thieme , H. R. 1992 . Epidemic and demographic interaction in the spread of potentially fatal diseases in growing populations . Math. Biosci. , 111 ( 1 ) : 99 – 130 .
- Wang , W. 2006 . Backward bifurcation of an epidemic model with treatment . Math. Biosci. , 201 ( 1–2 ) : 58 – 71 .
- Zegeling , A. and Kooij , R. E. 1998 . Uniqueness of limit cycles in models for microparasitic and macroparasitic diseases . J. Math. Biol. , 36 : 407 – 417 .
- Zhou , J. 1994 . An epidemiological model with population size dependent incidence . Rocky Mt. J. Math. , 24 ( 1 ) : 429 – 445 .
- Zhou , J. and Hethcote , H. W. 1994 . Population size dependent incidence in models for diseases without immunity . J. Math. Biol. , 32 : 809 – 834 .
Appendix 1. A Dulac-type theorem
We give here an extension of Dulac's Theorem that can be used to rule out periodic solutions when the vector field is C 1 everywhere except on a curve Γ on which it is non-differentiable. It was brought to our attention that this result is quite similar to a result of Wang Citation12. However, the result here covers the more general case of the non-differentiability occurring along a curve rather than along a straight line.
Let D be a simply connected open subset of ℝ2. Let be a simple curve such that
consists of two components D
1 and D
2. We require the following:
-
(H1) For each ε>0, there exist simple C 1 curves
in D j , j=1, 2, such that the C 1 distance
is less than ε.
A sufficient (but not necessary) condition for (H1) to be satisfied is that Γ be C 2.
Consider the differential equation
-
(H2) Solutions to Equation Equation(A1)
are unique, and are transverse to Γ except possibly at a finite number of points.
Theorem 6
(A Dulac-type Theorem)Let α be a scalar function on D, bounded on compact subsets of D. If
has constant sign on D
1∪D
2, then there are no piecewise smooth, simple closed curves in D which are invariant under (A1)}.
Proof
Suppose is a piecewise smooth, simple closed curve which is invariant under Equation Equation(A1)
. The possibility of γ being contained entirely in D
1 or D
2 is precluded by Dulac's Theorem. Thus we may assume that γ intersects Γ. Let
(see Figure A1). By the Jordan Curve Theorem, there is a connected region U which is bounded by γ. Let
. (We note that U
1 and U
2 may not be connected.) Take ε>0 small enough so that
intersects each component of U
j
. Let U
jε be the maximal subset of U
j
which is bounded by γ
j
and
and let C
jε be its boundary. Finally, let
and
be the parts of C
jε which coincide with γ
j
and
, respectively. Then denoting g=(u, v) and
, and using Green's Theorem, we have
Appendix 2. A small lemma on eigenvalues
Lemma 1
Let
Proof
The characteristic equation is