Abstract
Evolutionary games, here modelled by systems of ordinary differential equations, encapsulate Darwin's theory of evolution by natural selection. An evolutionary game can reach a local minimum, allowing invasion by mutants to drive the system out of its minimum and engender speciation. Games based on optimality considerations do not resist invasion. Nash equilibrium does not resolve to evolutionary stable strategies – mutants can invade existing communities of species.
1. Classical vs. evolutionary games
Evolutionary games mimic evolution by natural selection, as presented by Darwin Citation4. It can be distilled to three axioms Citation3:
-
(1) Variation in trait values which in turn cause
-
(2) fitness differences that are
-
(3) inherited.
Unlike classical games, strategy values are passed on by organisms via inheritance. These values are inherited with small variation. Players are not required to be rational. They are not required to know the rules. They are not required to know the solution concept. And they are not even aware that they are playing a game. The rules are dictated by evolution by natural selection. They consist of the sum effect of population densities of growth and decline ratesFootnote1 and of strategy values. These are influenced by the environment and interactions with contemporary individuals of species populations (players). The dynamics of the game are followed by the species mean value of the set of phenotypic traits. The mean is unique for a species. The phenotypic traits are subject to natural selection.
Throughout, we use strategy and strategy-values interchangeably. The meaning should be clear from its context. Except here and there, we discuss scalar strategies only. Expansion to vector strategies is (almost) trivial Citation2.
1.1 Some variations of classical games
In a static game, n players choose their strategies to maximize
A dynamic game consists of a set of ODE
We require that is a subset from a strategy set 𝒰.Footnote2 The set 𝒰 is a subset of the n-dimensional Euclidean space ℝ
n
. If 𝒰 is finite, then
is said to be a set of pure strategies. If 𝒰 is continuous, then
is a set of continuous strategies.
1.2. Game theory milestones
The game theory generalizes the optimization theory. It was first introduced by the engineer/economist Pareto Citation10 with the so-called non-commensurable multi-criteria payoff functions. With it, the optimization problem becomes one of maximizing an m vector of payoff functions subject to the same conditions on
as above. One may think of this problem in two ways. As a single player with m payoff criteria such as cost, weight, and reliability. Or as m players, each controlling a single component of
that maximizes a single component of
. Thus, Pareto introduced the first static game.
The publication of ‘Theory of Games and Economic Behavior’ by Von Neumann and Morgenstern Citation15 marked a significant progress in game theory. They began with the two-player matrix game. There is a large and growing body of literature on the theory and applications of matrix games, particularly in economics, where the evolutionary perspective is frequently adopted Citation13 Citation23.
Currently, classical games are identified with one of three categories: matrix games, continuous static games, and differential games. Matrix games are static with a finite number of pure strategies. After each choice of strategy, the payoff to each player is determined as an element of a matrix. One player's strategy corresponds to the selection of a row. The other player's strategy corresponds to the selection of a column. All possible payoffs are given by the elements of the matrix. The particular payoff a player receives is determined by this combination of strategies. In continuous static games, the strategies are drawn from a continuous set. The strategies and payoffs are related in a continuous manner Citation19. The game is static in the sense that an individual's strategy is a constant parameter. Differential games Citation6 are characterized by continuously time-varying strategies and payoffs. They are determined from a dynamic system of ODE equations. Differential games generalize the optimal control theory Citation11. The latter may be perceived as a dynamic game with a single player.
Maynard Smith and Price Citation8 and Maynard Smith Citation7 introduced the concept of an evolutionarily stable strategy (ESS). Thus began the evolutionary game theory. Evolutionary games are dynamic with a solution concept (ESS) and unspecified target set. Strategies evolve by means of natural selection. They represent adaptive phenotypic traits – those traits that are subject to natural selection. A player's strategy set is the set of all evolutionarily feasible strategies. Payoffs correspond to fitness.
Fitness refers to the relative density of a species population (compared with other species populations that participate in the game) at a particular time. When the population is at the stable-point equilibrium, fitness is constant. Otherwise, we have the so-called instantaneous fitness. The fitness of an individual is defined as fitness divided by the total population. It influences changes in the strategy's frequency within the population.
Evolutionary games differ from classical games in some fundamental features. Classical games focus on strategies that optimize players payoffs. Evolutionary games focus on strategies that persist through time. Through births and deaths, players come and go. But their strategies pass on from generation to generation. In classical games, players choose strategies from a well-defined strategy set. This set is part of the game's definition. In evolutionary games, players inherit their strategies with small mutations. The strategy set, determined by physical and environmental constraints, may change with time. In classical games, each player may have a separate strategy set. Separate payoffs may be associated with separate strategies. In evolutionary games, there are groups of evolutionarily identical individuals. Players are evolutionarily identical if they share the same strategy set and the same payoffs from using the same strategy Citation16 Citation17. In classical games, the optimizing rule is based on rational or self-interest choice of strategy. Consequently, players select predictable strategies. In evolutionary games, the optimizing rule is based on natural selection. Consequently, players do not select strategies. In classical games, the concepts of inner and outer games do not exist. In evolutionary games they do Citation16. In evolutionary games, the inner game involves short-term ecological dynamics. It corresponds to classical game dynamics. Within the inner game, players interact with others in a dynamic setting. They receive payoffs based on their own and others’ strategies. The outer game refers to dynamic evolutionary processes. Its time scale is usually long. Natural selection engenders the outer game.
2. Nash equilibrium, optimization and ESS
2.1. The inner game
The ecological dynamics associated with the evolutionary game are somewhat similar to EquationEquation (2). Here, however, the dynamics are written in terms of a so-called fitness function H
i
, i=1, …, n. The function encapsulates the instantaneous per capita growth rate of a group of individuals (species) whose density is x
i
.
Throughout, we address the single phenotypic trait only; but see Citation2. Consider n species and let . Individuals in a population are identified by their value of a heritable phenotypic trait that is subject to natural selection. Individuals with approximately equal trait-values belong to a single species. A species is identified by the mean of these trait values. Strategy dynamics refers to changes in this mean. Let u
i
be the strategy of individuals of species i. In general, population dynamics of species i depends on the density of all other species and on the strategies of all species. Consequently, the ecological dynamics are given by
Denote by the processes that encapsulate the rate of growth of species i’s population. Similarly, denote by
the processes that lead to the rate of decline. Then EquationEquation (3)
becomes
EquationEquation (4) includes various special cases. For example, in
2.2. The G-function
Vincent and Brown Citation18 developed the concept of a G-function as a means to describe the fitness of many species by means of a single mathematical expression. A function is said to be a fitness generating function (G-function) of the population dynamics Equation(3)
if
Consequently, we can write the population dynamics Equation(4) as
2.3. The outer game of the dynamics of evolution by natural selection
Consider solving EquationEquation (6) for fixed
. Then in general, one obtains solutions that are neither evolutionarily stable nor at maximum fitness Citation14. The outer game determines how components of the strategy vector evolve through time. The outer game is played by simultaneously solving the population dynamics equation with a strategy dynamics equation (here called Darwinian dynamics Citation18). (One must keep in mind that each u
i
represents the average of phenotypes’ distribution around this average. The distribution is assumed symmetric with small variance Citation22, where strategy dynamics were first introduced.) The solutions may not result in ESS or maximum fitness. They encapsulate the rules of evolution by natural selection.
To obtain an expression for the strategy dynamics, recall the definition of the G-function in EquationEquation (5) and the population dynamics given by EquationEquation (6)
. Strategies evolve according to the dynamics
2.4. Evolutionary game landscapes
The fitness of individuals of a species is a function of its strategy. A plot of fitness vs. strategy provides insight into why some strategies ‘win’ and others ‘lose’. This plot defines a landscape. We distinguish between two types of landscapes. The first, the ‘fitness landscape’, is derived from optimality principles Citation14. The second, the ‘adaptive landscape’, is derived from the $G$-function Citation18. The fitness landscape is a plot of fitness (vertical axis) in EquationEquation (1)
vs. strategy. The adaptive landscape is a plot of the G-function (vertical axis), in EquationEquation (5)
vs. the strategy. The fitness function and the G-function may arrive at the same solution. However, plotting both illustrates the difference between optimally derived and evolutionary derived landscapes.
2.4.1. The fitness landscape
If one chooses a strategy vector , and initial conditions for the densities
, EquationEquation (3)
may be integrated to produce a solution for
as a function of time. Typically, there exists an equilibrium solution with
and at least one
. It follows from the population dynamics that the fitness for those species with
must be negative. The fitness for those with
must be zero. Given that the strategy vector
and its corresponding equilibrium solution
, we define the fitness landscape by varying a component u
i
of
while holding all other components and the equilibrium
constant.
Definition 1
(Fitness landscape) A fitness landscape for a surviving species i is a plot of H
i
(w) vs. w. This function is obtained by replacing u
i
in
by w holding all other strategies fixed.
For example, consider EquationEquation (8): n denotes the number of species; r the intrinsic growth rate common to all species; K(u
i
) the carrying capacity of species i; and
the interference (competition) coefficients. Definition 1 implies that
only when w=u
i
. When w=u
i
, equilibrium requires that
. As explained below, a strategy leading to a higher population density will not be evolutionarily stable if it is not at a maximum point on the adaptive landscape.
2.4.2. Adaptive landscape
The adaptive landscape comes about using the methods of evolutionary games as developed in Citation18. There are as many fitness landscapes as there are species (n). There is only one adaptive landscape for all species. It is given by the G-function Equation(9).
Definition 2
(Adaptive landscape) For a given strategy vector
, assume that there exists an equilibrium solution
with at least one
. The adaptive landscape corresponds to a plot of
vs. v where
and
are held fixed.
One way to think about this landscape is that it is a plot of the fitness of individuals using the strategy v at a density near zero when played against all other strategies at their respective equilibrium population densities. It can be shown that a necessary condition for a given strategy to be evolutionarily stable is that it must correspond to a maximum point on the adaptive landscape Citation18. As we shall see below, optimization-based solutions do not always satisfy this condition. Hence, such solutions are not evolutionarily stable. Any individual that can benefit by not using the group optimal strategy will increase their own fitness at the expense of others. The principal property of an ESS is that if all individuals in a population are using it, then other individuals using a ‘mutant’ strategy cannot invade. The adaptive landscape provides a useful tool for determining the evolutionary stability of a given strategy.
2.4.3. Optimization-based and evolutionary-based solutions
Assume that the optimization-based solution is obtained by playing a dynamic Nash game. Then we must modify the Nash Citation9 definition for a static game to:
Definition 3
(Nash equilibrium solution) A Nash equilibrium solution
for the payoff functions H
i
(·) is such that if any player makes a unilateral change in his strategy (to some other point in 𝒰), then his payoff can be no higher.
From the definition we immediately obtain
Lemma 1
(Nash equilibrium solution) Let
with u
i
in
replaced by
. It follows from Definition 3 that a point
is a Nash equilibrium solution for the functions H
i
(·) if and only if for each i=1, … n
Thus, Nash equilibrium solution applies to the dynamic game given by EquationEquation (3). Consequently, we extend Definition 3 to
Definition 4
(Dynamic Nash equilibrium solution) A strategy vector
is said to be a global dynamic Nash solution for the evolutionary game if and only if system
Equation(3)
at equilibrium satisfies Definition 3; i.e. given an initial condition
and a strategy
, the solution of Equation
Equation(3)
results in an equilibrium solution for
such that
In Definition 4, we assume that exists. Both solutions (based on Definitions 3 and 4) reduce to a simple maximum definition when n=1.
Rearrange such that x
1>0,
and x
c+1=0,
. Set
and similarly for
. Now n=c+m with 1≤c≤n. Let
be the catenation of two vectors,
and
, associated with
and
with the corresponding elements of
so that
Definition 5
(ESS) A strategy vector
is said to be an ESS if and only if system
Equation(3)
is resistant to invasion. This means that for every initial condition
and
, the solution of Equation
Equation(3)
tends to the equilibrium
where
. If
lies in the neighbourhood of
then
is said to be a local ESS.
Maynard Smith's Citation7 original definition is a local ESS with both and
scalars. Definition 5 represents a generalization of his idea in the context of evolutionary games governed by a system of ordinary differential equations.
Lemma 2
(ESS) It follows from Definition 5 that if
is an ESS then
It follows from the Lemma 2 that for any i=1, …, c and any ,
3. Evolutionary games and Darwinian dynamics
Recall that a fitness landscape is based on optimality design, which in turn corresponds to Nash dynamics (see Definition 4). An adaptive landscape is based on Darwinian dynamics that satisfy the ESS maximum principle. The difference between these dynamics (even if the solutions are the same!) is highlighted by their generated landscapes. In both cases, analytical solutions cannot be expected. Consequently, one must rely on numerical solutions, using algorithms such as ‘hill climbing’.
The dynamics of strategy for Darwinian dynamics is given by EquationEquation (11). For optimality design, the gradient algorithm Citation20 of the form
3.1. Landscape layouts
Figures that provide landscape layout plots are instructive. They emphasize the difference between adaptive and fitness landscapes. In the following examples, when results do not depend on initial conditions, they are not specified.
Example 1 (Frequency dependence)
The term frequency dependence implies that fitness of any individual depends on the strategies used by all individuals in the population.Footnote4 Let us use the standard probability density function Beta to mimic Y in EquationEquation (4) (see also Example 1). The Beta density with shape parameters p and q is
Example 1 illustrates the difference between fitness (H-based) and adaptive (G-based) landscapes. The maximum principle is a necessary condition. Therefore, the numerical solution of G-based landscapes may settle on a minimum. This minimum is not an ESS. It happens to be identical to the maximum of the fitness landscape. Here is an example.
Figure 1. With frequency dependence given in EquationEquation (14), the ESS and maximum fitness solutions are different.
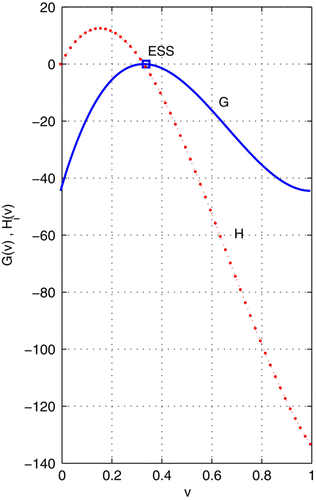
Example 2
Let
Figure 2. Left: local minimum on the adaptive landscape and maximum on the fitness landscape coincide. Right: Y as in EquationEquation (13) and N as in EquationEquation (15)
.
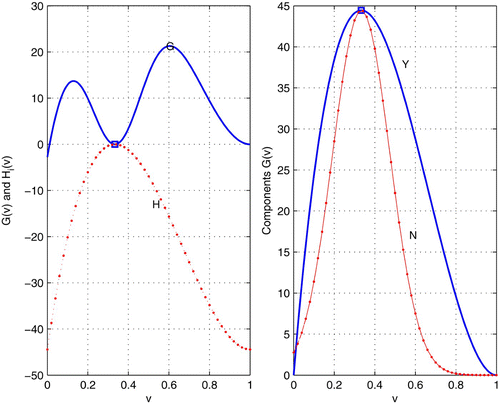
3.2. Future research
The G-function approach lends itself to interesting future research. Among others: (i) Replace Y in EquationEquation (13) with a biologically motivated growth function. (ii) Are there interpretable relations between the maximum of G in the adaptive landscape,
, and
? (iii) Similarly for
and
? (iv) Are there relations between maximum G and maximum H based on (i) and (ii)? These derivatives may shed light on the requirements for minimum or maximum for G and H.
Casting the theory in stochastic framework will represent a significant progress.
4. Discussion
The ESS maximum principle is constructive with respect to the global maximum (G=0) and with respect to the size of the ESS coalition (number of species, n). Consequently, the maximum principle dictates species diversity at ESS in the evolutionary game. The crucial parameter in the models we use is the phenotypic plasticity with respect to carrying capacity; i.e., σ
k
in EquationEquation (7). The larger σ
k
is, the larger the coalition. Local minima (as in ) shed light on speciation in the evolutionary game. An invading mutant, close to the minimum, breaks the local strategy that minimizes the value of the G-function in two and thereby causes two uphill climbing species.
Coexistence, adaptation and speciation remain fundamental research topics in biology. All three shed light on the complex nature of life. Some of this complexity (speciation, invasion, stability) can be illustrated with the G-function method. Fitness landscapes cannot reveal whether or not species can invade the system. Adaptive landscapes can.
There are situations where Darwinian dynamics ends at a minimum and optimal dynamics at a maximum where both coincide (). This situation, where frequency dependence is not a factor, could serve as a model for the numerous cell types coexisting in complex organisms.
In some cases, where an ESS does not exist at equilibrium because the G-function is flat, an infinite number of species can be added to the system (via invasion or mutation). Such a case is not realistic. Here, the mean strategies carrying heritable variation may exhibit random walk (so much so, because there is no advantage among strategies) which represents genetic drift. In a game theoretic context, if the G-function has portions that are flat and portions that are not, drift combined with Darwinian dynamics can drive the system to maximal points in the G-function Citation14.
The journey from optimization, to game theory, to evolutionary game theory provides insights into the differences between optimal design and Darwinian evolution. In optimal design, individuals are not constrained by others. As soon as individuals become constrained by others (as is the case with frequency dependence), the evolutionary game takes over. Evolutionary dynamics can drive species to a stable maximum or minimum. However, if the maximum is not an ESS, novel strategies may evolve until the size of the ESS coalition is achieved. Such is the case at the minimum of the G-function.
The strength of the G-function approach to evolutionary game theory lies in its flexibility. The G-function provides potential explanations of phenomena such as unoccupied niches, limiting similarity, invasion, relative abundance Citation21, and the emergence of cancer Citation5. The $G$-function approach allows for modelling different biological communities with scalar strategies, vector strategies Citation2, multiple G-functions Citation1, multistage G-functions Citation12, and even intelligent design Citation14.
Finally, in the modelling of cell growth using evolutionary game methods, Gatenby and Vincent Citation5 discovered an underlying reason why the strategies used by cells are ‘cooperative’. This may be a situation where Darwinian evolution (under competition) and optimization (under cooperation) yield the same strategy. Unless carefully controlled (e.g. via DNA), a cooperative optimal strategy is subject to ‘cheating’. The onset of cancer appears to be associated with a breakdown in the optimal control structure which, consequently, allows for the coexistence of mutant cells. Also, mutant cells change their own local environment. Thus, Darwinian evolution of the mutant cells breaks the cooperative nature of the game. The mutant cells evolve to a destructive state that defines cancer that can lead to the destruction of the individual.
Additional information
Notes on contributors
Thomas L. Vincent
Unfinished manuscripts by the late T.L. Vincent, compiled and interpreted by T.L.S. Vincent.Notes
Professor Vincent was fond of calling growth and decline rates Yin and Yang, respectively, reflecting the ancient Chinese philosophy of two primal opposing but complementary forces in all things in nature.
Strictly speaking, 𝒰 is dense.
The formal definition as given in Citation18 requires, in addition, that an ESS be convergent stable.
The frequency at which a given strategy is present in a population is proportional to the fraction of individuals using that strategy.
References
- Brown , J. S. and Vincent , T. L. 1992 . Organization of predator-prey communities as an evolutionary game . Evolution , 46 : 1269 – 1283 .
- Brown , J. S. , Cohen , Y. and Vincent , T. L. 2007 . Adaptive dynamics with vector-valued strategies . Evol. Ecol. Res. , 9 : 1 – 38 .
- Cohen , Y. Darwinian evolutionary distributions with time-delays . Nonlinear Dyn. Syst. Theory , in press
- Darwin , C. 1859 . The Origin of Species , London : Avenel Books .
- Gatenby , R. A. and Vincent , T. L. 2003 . An evolutionary model of carcinogenesis . Cancer Res. , 63 : 6212 – 6220 .
- Issacs , R. 1965 . Differential Games , New York : Wiley .
- Maynard Smith , J. 1982 . Evolution and the Theory of Games , Cambridge : Cambridge University Press .
- Maynard Smith , J. and Price , G. R. 1973 . The logic of animal conflict . Nature , 246 : 15 – 18 .
- Nash , J. F. 1951 . Noncooperative games . Ann. Math , 54 : 286 – 295 .
- Pareto , V. 1896 . Cours d'Economie Politique , Lausanne : Rouge .
- Pontryagin , L. S. , Boltyanskii , V. G. , Gamkrelidze , R. V. and Mishchenko , E. F. 1962 . The Mathematical Theory of Optimal Processes , New York : Wiley .
- Rael , R. C. , Constantino , R. F. , Cushing , J. M. and Vincent , T. L. 2009 . Using stage-structured evolutionary game theory to model the experimentally observed evolution of genetic polymorphism . Evol. Ecol. Res. , 11 : 141 – 151 .
- Samuelson , L. 1997 . Evolutionary Games and Equilibrium Selection , Cambridge : MIT Press .
- Scheel , D. and Vincent , T. L. 2009 . Life: Optimality, evolutionary, and intelligent design? . Evol. Ecol. Res. , 11 : 589 – 610 .
- Von Neumann , J. and Morgenstern , O. 1944 . Theory of Games and Economic Behavior , New York : Wiley .
- Vincent , T. L. and Brown , J. S. 1984 . Stability in an evolutionary game . Theor. Popul. Biol. , 26 : 408 – 427 .
- Vincent , T. L. and Brown , J. S. 1988 . The evolution of ESS theory . Annu. Rev. Ecol. Syst. , 19 : 423 – 443 .
- Vincent , T. L. and Brown , J. S. 2005 . Evolutionary Game Theory, Natural Selection, and Darwinian Dynamics , Cambridge : Cambridge University Press .
- Vincent , T. L. and Grantham , W. J. 1981 . Optimality in Parametric Systems , New York : Wiley .
- Vincent , T. L. and Grantham , W. J. 1982 . Trajectory following methods in control system design . J. Global Optim. , 23 : 267 – 282 .
- Vincent , T. L.S. and Vincent , T. L. 2009 . Predicting relative abundance using evolutionary game theory . Evol. Ecol. Res. , 11 : 265 – 294 .
- Vincent , T. L. , Cohen , Y. and Brown , J. S. 1993 . Evolution via strategy dynamics . Theor. Popul. Biol. , 44 : 149 – 176 .
- Weibull , J. W. 1997 . Evolutionary Game Theory , Cambridge : MIT Press .