Abstract
The influence of a resource subsidy on predator–prey interactions is examined using a mathematical model. The model arises from the study of a biological system involving arctic foxes (predator), lemmings (prey), and seal carcasses (subsidy). In one version of the model, the predator, prey and subsidy all occur in the same location; in a second version, the predator moves between two patches, one containing only the prey and the other containing only the subsidy. Criteria for feasibility and stability of the different equilibrium states are studied both analytically and numerically. At small subsidy input rates, there is a minimum prey carrying capacity needed to support both predator and prey. At intermediate subsidy input rates, the predator and prey can always coexist. At high subsidy input rates, the prey cannot persist even at high carrying capacities. As predator movement increases, the dynamic stability of the predator–prey-subsidy interactions also increases.
1. Introduction
Sustained temporal fluctuations in natural population densities are common Citation59, and predator–prey systems in particular are often noted for their oscillations Citation34 Citation39 Citation61.Footnote Murdoch Citation41 suggested that such interactions can be stabilized when predators are able to switch from a preferred prey that is rare to alternatives that are more common Citation10. Early mathematical modelling of communities involving one predator feeding on multiple prey species produced theoretical evidence validating this conjecture Citation11 Citation42, and the resulting predator-mediated interaction was soon identified as apparent competition between the prey because their common predator can potentially increase numerically when feeding on either species Citation24. Whether this interaction must always be negative to both prey species has been a source of intense research for three decades Citation2 Citation60. Indeed, when one predator consumes two nutritionally substitutable and self-reproducing (i.e. logistic-type) prey species, then in some cases, the prey may benefit from the interaction (apparent mutualism) Citation4. The properties and shapes of predator functional and numerical responses may be predictors for the kind of apparent interaction observed Citation7.
For predator–prey systems that are dynamically unstable, the indirect effects of a second resource are often different from those predicted by equilibrium dynamics Citation6 Citation7 Citation46. Adaption of predator behaviours, such as consumption rate constants and relative time spent in searching in distinct patches, can hinder predators from achieving an ideal free distribution and may instead promote oscillation or even chaos Citation3. An alternative prey available at constant density can have both stabilizing and destabilizing effects on predator–prey dynamics Citation9 Citation32 Citation56. In unstable systems, a predator's ability to adapt its attack rate can be either beneficial or harmful to a prey species when it is at low density Citation5. For a predator having fixed fractional population abundances in two different patches, switching food sources can destabilize dynamics and, in some cases, cause chaotic dynamics to occur at sufficiently high prey carrying capacities Citation33. Oscillation can also occur due to a Hopf bifurcation when predators switch because of group defense in prey Citation31 Citation40.
Many empirical studies suggest that the introduction of allochthonous resources (or resource subsidies) may disrupt otherwise stable food web linkages Citation12 Citation20 Citation22. Such knowledge is particularly important for resource management purposes; for example, reintroduced wolves in Yellowstone Park will switch to bison when their preferred ungulate prey, namely elk, are rare Citation16. In a theoretical study, seasonal subsidies can stabilize a consumer–resource relationship when external subsidy inputs complement the availability of in situ resources through time Citation57. The persistence of a predator whose dynamics are slow relative to a preferred prey species having fast dynamics and a constant resource subsidy (i.e. one having infinitely fast dynamics) can be threatened, for a short time only, by rare subsidies but never by more common ones Citation48. The timing of subsidy availability in part of a habitat can result in mixed (i.e. positive and negative) indirect effects on an in situ resource that occurs throughout the habitat Citation58. Allochthonous inputs can also have striking effects on larger food webs Citation13 Citation28 Citation29.
Holt, in studying a model involving a predator that can move between a patch containing prey and a second prey-free patch, notes that ‘passive dispersal can stabilize an otherwise unstable predator–prey interaction’ Citation26. The results remains true when logistic-type prey occur in both patches Citation27, although if the movement rate is very small, then the effect may be destabilizing Citation36. Still, non-diffusive movement (caused, e.g. by prey-handling time effects) can by itself generate oscillation Citation1 Citation27. The rapid evolution of migration probabilities can also produce non-equilibrium dynamics Citation62.
In this paper, we examine a series of predator–prey models in which the predator can also consume a non-living resource subsidy. Our motivating example shall be that of the predation of arctic foxes (Alopex lagopus) on lemmings (Cricetidae family). In coastal habitats, arctic foxes are believed to partially subsist in the winter on both the local lemming population and a resource subsidy, namely seal (Phocidae family) carcasses, which are provided by polar bear (Ursus maritimus) predation on the sea ice Citation52. Our primary interest is to determine situations in which dynamical stability and instability occur so as to understand more fully how resource subsidies may impact predators and their prey.
We begin in Section 2 by examining two differential equation models for the interactions between a predator species (e.g. arctic foxes) and either a prey species (e.g. lemmings) or a donor-controlled resource subsidy (e.g. seal carcasses). In Section 3, we study a model that includes all three components (predator, prey, and subsidy) in a single spatial location. We then study in Section 4 a spatial model in which the predator must move between two separate locations, one containing the prey species and the other containing the resource subsidy. The different predictions made by these models, as well as their biological implications, are considered in Section 5. In Section 6, we extend the model to include seasonal and long-term variations in subsidy amount, as well as spatial separation between prey and subsidy. We also relate our results to the original arctic system and make some additional conclusions.
2. A pair of elementary models
Before introducing either three-species model in full, we mention first some properties possessed by two relevant sub-models.
2.1. Predator and prey system
Let x(t) and y(t) be the population size of a prey and predator species, respectively, at time t≥0, and suppose that these functions obey the Gause-type model Citation17
We can predict the kind of ecological outcome that will occur by defining two critical values of k. Toward this end, let
Figure 1. Outcome of (a) the predator–prey model (I) for different carrying capacities k and (b) the predator-subsidy model (II) for different subsidy input rates i.
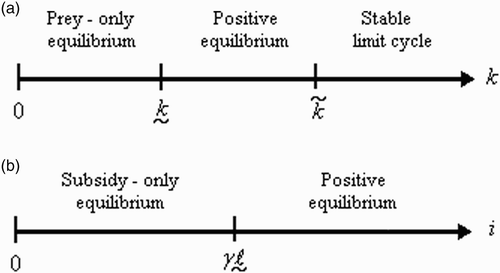
Table 1. Equilibrium properties of the predator–prey model (I).
2.2. Predator and subsidy system
Let s(t) be the amount of a donor-controlled resource subsidy, and y(t) be the population size of a species that consumes the subsidy (henceforth known simply as predator), at time t≥0. Suppose that these functions obey
where i is the rate at which the subsidy appears, γ is the rate at which it disappears or otherwise becomes inedible, ψ is the maximum rate at which predators can exploit the subsidy, c is a half-saturation constant, η is a conversion factor, and δ is the mortality rate for the predator. It is assumed that all constants are positive, that η is no greater than unity, and that s(0)>0 and y(0)>0. As before, we assume that so that the predator's per-capita growth rate is positive for sufficiently large values of s.
We can distinguish cases in which the predator persists from those in which it does not by defining
The equilibrium properties of model (II) are summarized in and portrays the behaviour of the model for different values of the subsidy input rate i.
Table 2. Equilibrium properties of the predator-subsidy model (II).
3. Predator and prey system with a non-spatial subsidy
Suppose that the prey and subsidy described above occur in the same habitat, and that they are both exploited by a single generalist predator according to the following equations
Since the form of the predator's functional response allows it to become satiated on a combination of prey and subsidy, we assume that either or
(otherwise, the predator's per-capita growth rate is always negative). From a biological standpoint, it is sensible to restrict attention further to nutrient-rich prey and subsidy, so that the predator can subsist on either resource when it is in unlimited supply. Our interest then is to understand predator strategy for situations in which prey and subsidy abundances are both limited. In view of the preceding remarks, we now assume that both
and
.
It can be seen in that several different outcomes are possible, including (i) a predator-free equilibrium, (ii) a prey-free equilibrium, (iii) an equilibrium in which the predator, prey, and subsidy coexist, and (iv) coexistence under stable limit cycles. Our goal is to distinguish situations in which these different outcomes occur, and to understand the influence of parameter values on these outcomes.
Figure 2. Time-series for the non-spatial predator–prey-subsidy model (III) with the following parameter values: r=0.1, θ=5, e=1, γ=1, ψ=5, ε=0.1, η=0.1, and δ=0.1. In (a) the prey population size approaches a positive equilibrium value, the subsidy amount approaches its natural value, and the predator becomes extinct (k=0.1 and i=0.12). In (b) the prey becomes extinct, the subsidy amount approaches its natural value, and the predator population size approaches a positive equilibrium value (k=0.1 and i=0.3). In (c) the predator and prey population sizes approach positive equilibrium values and the subsidy amount approaches its natural value (k=0.4 and i=0.2). In (d) the predator, prey, and subsidy all persist in a stable limit cycle (k=2 and i=0.05). In all figures, x(0)=0.2, y(0)=0.1, s(0)=0, k =0.25, and ℓ=0.25.
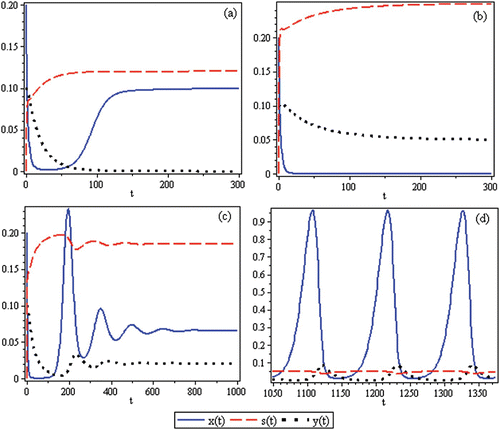
The complete system possesses several equilibrium states (). Their local stability is determined by the eigenvalues of the Jacobian matrix
Here, we define . Observe that although the parameter i does not appear explicitly in the expression for J, it may appear implicitly via the equilibrium states.
Table 3. Equilibrium properties of the predator–prey-subsidy model (III).
3.1. Subsidy-only equilibrium
At the subsidy-only equilibrium , the Jacobian matrix
3.2. Predator-free equilibrium
At the predator-free equilibrium , the associated Jacobian matrix
Theorem 1 (Predator-free equilibrium)
The predator-free equilibrium is stable if and only if
and i<i
*(k).
The case in which a predator-free equilibrium is stable is represented in .
3.3. Prey-free equilibrium
A prey-free equilibrium exists and is unique when
, and so we now assume this to be the case. The corresponding Jacobian matrix
Theorem 2 (Prey-free equilibrium)
A prey-free equilibrium exists if and only if
and is stable if and only if i>i*. When the equilibrium exists, it is unique.
The situation in which a prey-free equilibrium is stable is illustrated in .
3.4. Positive equilibrium
We now turn to equilibrium states , which are positive in each component. These states satisfy
Theorem 3 (Existence and uniqueness of positive equilibrium)
A positive equilibrium (𝒫) for system (III) exists under the following conditions:
(a) If
| |||||
(b) If
|
Furthermore, when 𝒫 exists then it is unique.
Proof
First, we examine conditions under which one or more positive equilibrium states are feasible. It is clear from Equation Equation(7a) that y* can be expressed in terms of x* and s*,
We now obtain a complete description of when 𝒫 exists and whether it is unique. Let
(i) Suppose first that | |||||
(ii) Suppose next that | |||||
(iii) Finally, suppose that k<k* (i.e. B<0). Similar arguments establish that |
In deriving conditions for the existence and uniqueness of 𝒫, it was necessary to discern the behaviour of s* as a function of i. Specifically, we found that
• | s* is increasing and concave downward | ||||
• | s*(i=0)>0 when k<k* and s*(i=0)=0 when k>k* | ||||
• |
| ||||
• |
|
We now consider the behaviour of x* and y* as functions of i. First, it is clear from EquationEquations (9) and Equation(11)
that
• | x* is decreasing and concave upward | ||||
• |
| ||||
• |
| ||||
• |
|
Next, we see from EquationEquation (8) that
• | y*>0 when s*>0 and 0<x*<k | ||||
• |
y* (i=0)<0 when | ||||
• |
| ||||
• |
|
We now have a complete description of how the components of 𝒫 depend on the parameter i. It remains to study the stability of 𝒫, for which our analytical results are only partial. We begin by considering different elements of the Jacobian in EquationEquation (1). Observe from EquationEquation (8)
that
Thus, our results in this direction are based partly on numerical evidence. We conjecture the following statement.
Conjecture 1 (Stability of positive equilibrium) There exists a bifurcation function i **(k) defined for k>[ktilde] (with e in place of a) such that
(a) i **(k) is continuous, increasing, and concave downward | |||||
(b) | |||||
(c) if k<[ktilde] then 𝒫 is stable whenever it exists | |||||
(d) if k>[ktilde] then 𝒫 is stable only for | |||||
(e) if k>[ktilde] then a globally attractive limit cycle occurs for 0<i<i **(k) | |||||
(f) the bifurcation curve i=i
**(k) coincides with the set on which |
Again, all of our numerical investigations support this conjecture (). The parameter region in which 𝒫 is conjectured to be stable is represented in , and the region in which it is conjectured to be unstable is represented in .
Figure 3. Outcome of the non-spatial predator–prey-subsidy model (III) for different values of the carrying capacity (k) and subsidy input rate (i) based on local stability analysis. The system moves to a predator-free equilibrium in the lower-left region (red), to a prey-free equilibrium in the upper region (yellow), to a positive equilibrium in the central region (green), and to a stable limit cycle involving predator, prey, and subsidy in the lower-right region (blue). The lower-left boundary is given by i=i *(k), the upper boundary is given by i=i*, and the lower-right boundary is given by i=i **(k). All results are theoretical except for the stability of the positive equilibrium in the central and lower-right regions which is based instead on numerical evidence. The parameter values used are the same as in .
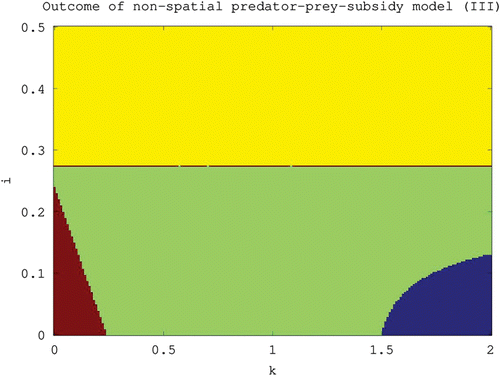
4. Predator and prey system with a spatial subsidy
Here we recast the non-spatial model (III) into a spatial one involving movement of the predator between two patches, one of which contains only the prey and the other containing only the subsidy. Whether a predator consumes prey or the subsidy depends entirely on its location. A related model for predator movement in a continuous habitat Citation45 is described in Section 6.
Let x(t) be the population size of a prey species (in patch 1), s(t) be the amount of a donor-controlled resource subsidy (in patch 2), and y 1(t) and y 2(t) be the population sizes of a predator species (in patches 1 and 2) at time t≥0. Suppose that these functions obey
where all parameters have the same interpretation as before, and the diffusion coefficient α denotes the rate at which predators randomly move between the two patches. It is assumed that all constants are positive, that ε and η are no greater than unity, and that x(0)>0, y
1(0)>0, y
2(0)>0, and s(0)>0. In addition, and for reasons similar to those offered earlier, we will restrict attention to nutrient-rich prey and subsidies, i.e. to parameter combinations in which both and
.
As illustrated in , several different outcomes are possible, including (i) a predator-free equilibrium, (ii) a prey-free equilibrium, (iii) an equilibrium in which the predator, prey, and subsidy all persist, and (iv) the persistence of predator, prey, and subsidy in a stable limit cycle. As with the non-spatial model described earlier, we wish to distinguish situations in which these different outcomes occur, and to understand the influence of parameter values on these outcomes.
Figure 4. Time-series for the spatial predator–prey-subsidy model (IV) with the following parameter values: r=0.1, θ=5, a=1, γ=1, ψ=5, c=1, ε=0.1, η=0.1, δ=0.1, and α=0.8. In (a) the prey population size approaches a positive equilibrium value, the subsidy amount approaches its natural value, and the predator becomes extinct (k=0.1 and i=0.1). In (b) the prey becomes extinct, the subsidy amount approaches its natural value, and the predator population sizes approach positive equilibrium values (k=0.1 and i=1.0). In (c) the predator and prey population sizes approach positive equilibrium values and the subsidy amount approaches its natural value (k=0.4 and i=0.3). In (d) the predator, prey, and subsidy all persist in a stable limit cycle (k=2.4 and i=0.1). In all figures, x(0)=0.2, y 1(0)=0.1, y 2(0)=0, s(0)=0, k (α)=0.25, and ℓ(α)=0.65.
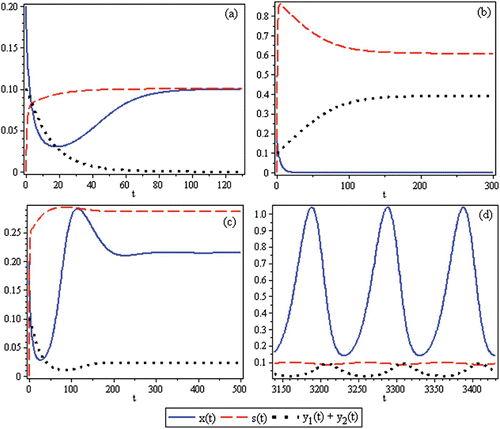
At equilibrium, the equations above become
It is clear from the two equations on the right that if the predator is absent from one patch (say, ) then necessarily it will also be absent from the other
. As we will consider both the feasibility and stability of the different equilibrium states (), we will make frequent use of the Jacobian. With the state variables ordered as (x, y
1, y
2, s), the Jacobian can be written as
Table 4. Equilibrium properties of the spatial predator–prey-subsidy model (IV).
4.1. Subsidy-only equilibrium
At the subsidy-only equilibrium , the Jacobian matrix
4.2. Predator-free equilibrium
At the predator-free equilibrium , the Jacobian matrix has the form
Theorem 4 (Predator-free equilibrium)
The predator-free equilibrium is stable if and only if
and
.
Notice that the equilibrium is stable whenever ; indeed, since
, it is stable whenever α is sufficiently small. The case in which a predator-free equilibrium is stable is represented in (a).
4.3. Prey-free equilibrium
A prey-free equilibrium has the form . We will first determine its form and then its stability. Let us define
Since is finite (
), it follows that
is finite for sufficiently small α. Also,
is an increasing function of α along the interval where it is finite. Similar comments hold for
. Observe also that
, with equality occurring only when α=0 or neither is finite. Next, notice that (i) when
then
, (ii) when
then
, and (iii) when
then
. For parameter combinations satisfying
, then a unique prey-free equilibrium exists and has components
Theorem 5 (Prey-free equilibrium)
A prey-free equilibrium exists if and only if
and is stable if and only if
. When the equilibrium exists, it is unique.
The case in which a prey-free equilibrium is stable is portrayed in .
4.4. Positive equilibrium
In view of Equation (15), a positive equilibrium satisfies the following equations
As can be seen from Equation (23), necessary conditions for an equilibrium to be positive are and 0<x*<k. However, it is not clear whether these conditions are sufficient (yet), and so we will explore this here.
Proposition 1
(a) If
| |||||
(b) If
|
Proof
Observe from Equation (23) that
(i) If | |||||
(ii) If | |||||
(iii) If |
As a reminder, whether k lies above or below depends in a straightforward manner on the quantities
, a, and
. The next results provides a similarly simple description for predicting the relative magnitudes of s*,
, and
.
Proposition 2
Suppose that
and
are finite and that
is infinite.
(a) If
| |||||
(b) If
|
Proof
Suppose that and
are finite and that
is infinite. Substituting the expressions for x*,
, and
in EquationEquation (24)
into the upper left equation of (23) yields
To prove part (a) of the proposition, we assume that and observe that i in EquationEquation (26)
can be solved for as a continuous and differentiable function of s*>0,
To compute the value of i at , we observe from EquationEquation (20)
that
Now, we compute the value of i at . We begin by noticing that
The proof of (b) is similar. Assume first that . It is clear that i=0 at s*=0. The argument showing
at
is the same as above. To establish that i is increasing on the interval
, we recall EquationEquation (28)
Although statements similar to Proposition 2 can be made for situations in which or
is infinite or
is finite, we will make no attempt to exhaust all possibilities. Propositions 1 and 2 above together imply the following.
Theorem 6 (Existence and uniqueness of positive equilibrium)
Suppose that
and
are finite and that
is infinite. Then the positive equilibrium (𝒫) for system (IV) exists under the following conditions:
(a) If
| |||||
(b) If
|
Furthermore, when 𝒫 exists then it is unique.
In proving Proposition 2, we found that the behaviour of s* as a function of i was as follows.
• | s* is increasing along any interval where 𝒫 exists | ||||
• |
| ||||
• |
|
The behaviours of x*, , and
as functions of i can be obtained from Equations (23)– Equation(25)
We now have a complete description of how the components of 𝒫 depend on the parameter i. It remains to study the stability of 𝒫, for which our analytical results are again only partial. Towards this end, it is useful to define functions based on the right-hand side quantities appearing in (IV),
Recall that the stability of the equilibrium is determined entirely by the eigenvalues of J, which in turn are the zeros of its characteristic polynomial. This polynomial has the form
As with the non-spatial model, our stability results are again based partly on numerical evidence.
Conjecture 2 (Stability of positive equilibrium) There exists a bifurcation function
defined for
such that
(a) | |||||
(b) | |||||
(c) if | |||||
(d) if | |||||
(e) if | |||||
(f) the curve i=i
**(k) coincides with the set on which |
Again, all of our numerical investigations support this conjecture (). The parameter regions in which 𝒫 is conjectured to be stable and unstable are represented in and , respectively.
Figure 5. Outcome of the spatial predator–prey-subsidy model (IV) for different values of the carrying capacity (k), subsidy input rate (i), and predator-movement rate (α) based on local stability analysis. The system moves to a predator-free equilibrium in the lower-left region (red), to a prey-free equilibrium in the upper region (yellow), to a positive equilibrium in the central region (green), and to a stable limit cycle involving predator, prey, and subsidy in the lower-right region (blue). The lower-left boundary is given by i=i *(k,α), the upper boundary is given by i=i*(α), and the lower-right boundary is given by i=i **(k,α). The lower-left and upper boundaries both increase as α increases. All results are theoretical except for the stability of the positive equilibrium in the central and lower-right regions which is based instead on numerical evidence. The parameter values used are the same as in unless noted otherwise.
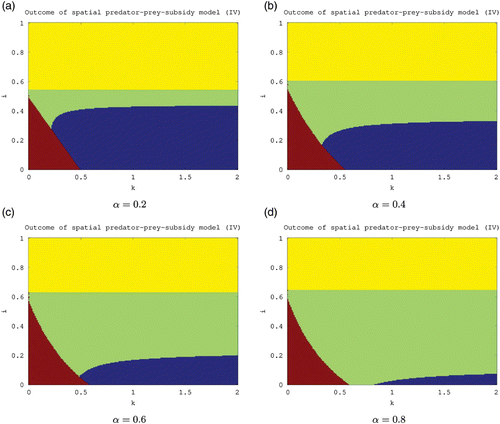
5. Biological implications
Here we compare the features of, and the predictions made by, the predator–prey model (I), the predator-subsidy model (II), the non-spatial predator–prey-subsidy model (III), and the spatial predator–prey-subsidy model (IV).
In keeping with our previous analysis, and the bifurcations diagrams in and , we will usually treat prey carrying capacity (k), subsidy input rate (i), and predator movement rate (α) as variables and all other model parameters as arbitrary but fixed. Also, we remind the reader that the predator is entirely food limited; it can persist only with sufficient food availability as measured by a combination of prey abundance and subsidy amount. In addition, the predator is an efficient consumer of both prey () and subsidy (
) in the sense that it can persist on either food type alone provided that it occurs at sufficiently high density. Finally, so that comparisons between models can be made, we will assume that the half-saturation constants a and c (in I, II, and IV) are interchangeable with e (in III) when appropriate.
For reference, the equilibrium properties of all models are stated in .
5.1. Subsidy-only equilibrium
In (II), the subsidy-only equilibrium is always feasible, and it is stable when the subsidy input rate (i) is too low to support the persistence of a predator which relies on it exclusively. In contrast, the corresponding equilibrium in (III) and (IV) is always feasible but never stable. The instability of this equilibrium in the latter models occurs because the prey can always invade a habitat in which the predator is absent.
5.2. Predator-free equilibrium
The predator-free (or prey-only) equilibrium in Equation (I) is always feasible, but it is stable only when prey carrying capacity (k) does not meet the minimum prey abundance needed to support a predator population (). The corresponding equilibrium in (II) coincides with the subsidy-only equilibrium and is treated above.
In (III), the predator-free equilibrium is always feasible but stable only in habitats where overall food productivity is low (i.e. for combinations of k and i in which both are small). The corresponding region in parameter space where the equilibrium is stable is illustrated in . Notice that the stability condition Equation(3) can be rewritten as
Many of the same remarks apply to (IV), at least in a qualitative way (). Namely, prey carrying capacity must lie below a threshold amount for a mobile but unsubsidized predator to be excluded. If the predator is a relatively inefficient consumer of the subsidy
then, when the subsidy is introduced, the predator will still be excluded because its movement pattern cancels out (although in a complicated manner) any benefit earned from obtaining the subsidy. However, if the subsidized predator is highly efficient
then it can only be excluded when the subsidy input rate is small
. For predators that move slowly
the latter situation will always prevail because
. As the movement rate (α) increases, then the minimum prey carrying capacity for an unsubsidized predator to persist
also increases because of wasted movement effort. In addition, the relation
and EquationEquation (20)
together imply that the stability boundary
increases as α increases. Thus, given a fixed combination of parameters near this boundary, a slow predator (α small) may persist whereas a swift predator (α large) will become extinct.
5.3. Prey-free equilibrium
A prey-free equilibrium is never feasible in Equation (I) because a predator must consume something to persist. In (III), such an equilibrium can occur, but only when the rate of subsidy input is sufficiently high . This condition corresponds perfectly with the condition for predator persistence
in (II). In both models,
and
represent the minimum amount of subsidy (i/γ) needed to support a predator (when c=e they are equal). In (II), the equilibrium is stable whenever it exists. However, the equilibrium in (III) is stable only when i lies above a still higher critical value, namely,
(see ). If prey have no ability to invade (r=0) then the prey-free equilibrium is, naturally enough, stable when feasible
. However, as the ability of prey to invade (r) increases, only highly subsidized predators can continue to exclude them (i>i*). Interestingly, the subsidy amount in a prey-free equilibrium is always
, regardless of the subsidy input rate (i) but provided that it is large enough. Thus, to maintain equilibrium, the predator must skim all subsidy above a fixed amount
. Notice that a similar thing occurs for the predator–prey equilibrium in Equation (I).
As can be seen in , similar remarks apply again to (IV). However, a prey-free equilibrium exists only in situations where the predator is a highly efficient consumer of the subsidy . When this occurs, then the equilibrium is feasible for
and stable for
. Here,
and i*(α) have the same interpretations as
and i* above, although they are somewhat more complicated quantities to define. It can be seen from EquationEquations (21)
and Equation(22)
that the feasibility and stability boundaries
and i*(α) both increase as the predator movement rate (α) increases. Consequently, the parameter region in which the prey cannot invade (i.e. where the prey-free equilibrium is stable) is largest when the predator moves slowly (α small) and it diminishes in area as the predator becomes faster. Also, since
and i*(α) both become infinite at sufficiently high α, it follows that the prey can always invade when the predator is swift (α large). Recalling that the subsidy can never be excluded at equilibrium, our results contradict those of a similar model in which apparent competition is found to be strongest when the predator is swift Citation25. However, the latter model differs significantly from (IV); namely, it assumes that the prey species both increase exponentially and that the predator functional response is linear. We believe that the precise source of conflict between the models’ predictions regarding the strength of apparent competition versus predator mobility warrants further investigation.
5.4. Positive equilibrium
The positive equilibrium of (I) exists when but is stable only for k<[ktilde]. When k>[ktilde] the equilibrium is unstable and is famously surrounded by a unique and globally attractive limit cycle, a phenomenon known as the paradox of enrichment Citation18
Citation19
Citation39
Citation51
Citation54.
A positive equilibrium in (III) can occur as follows. Consider first a predator which cannot rely on prey alone to support it . In this case, a small subsidy input rate (i<i
*(k)) will cause the predator to remain excluded and a large subsidy input rate (i>i*) will cause the prey to be excluded (see above). In these situations, a positive equilibrium cannot occur. However, the equilibrium can occur at intermediate subsidy input rates
. We conjecture that when the positive equilibrium does occur, then it is always stable. Suppose now that an unsubsidized predator can persist with the prey at either a stable equilibrium
or in a stable limit cycle (k>[ktilde]). In this case, small and intermediate sized subsidies (i<i*) will always permit a positive equilibrium to continue to occur, whereas large subsidies (i>i*) will again cause the prey to be excluded. Here, we conjecture that when an unsubsidized predator can persist at equilibrium with the prey
then any amount of subsidy input which allows for a positive equilibrium to occur will enable that equilibrium to be stable; however, when an unsubsidized predator coexists with its prey only in a stable limit cycle (k>[ktilde]), then small subsidy input rates (i<i
**(k)) do not destroy the structural stability of the limit cycle, and only intermediate sized subsidies (i>i
**(k)) enable a positive equilibrium to be stable. Thus, small subsidy inputs cannot eliminate oscillation altogether, whereas larger subsidy inputs can. Furthermore, we conjecture that i
**(k) is an increasing function, i.e. larger carrying capacities require higher subsidy input rates to eliminate oscillation.
Again, similar comments apply for the feasibility and stability of the positive equilibrium in (IV) (). The corresponding stability boundaries and i*(k) are described in detail above. For this model, numerical evidence suggests that an increase in the predator movement rate (α) will enlarge the stability region of the positive equilibrium, with a concomitant decrease in the stability region for the limit cycle (). Thus, it is likely that a slow predator (α small) will persist in oscillation, and a swift predator (α large) will persist at equilibrium.
6. Discussion
The use of secondary resources by species from adjacent habitats or ecosystems is widespread Citation46 Citation50 Citation52. These allochthonous resources, or resource subsidies, can strongly affect the abundance and space use of consumers in recipient ecosystems, with cascading effects on in situ resources Citation28 Citation30 Citation38 Citation50 Citation53. However, the impact of resource subsidies on recipient communities can be affected by several factors, including the productivity of the donor and recipient habitats, amount of input, trophic level receiving the input, and temporal variability in subsidy input rates Citation29 Citation37 Citation55 Citation58. Not only the magnitude, but the direction of the indirect effect on in situ resources can vary. If consumers respond numerically to increased food availability through resource subsidies, local prey could decline because of increased predation, an effect known as apparent competition Citation24. However if predators aggregate toward areas of high subsidy availability, consumption of local prey in other areas may be reduced (apparent mutualism Citation4). Furthermore, local stability of food webs can be affected by the addition of resource subsidies, with a potential loss of species Citation28 Citation29.
6.1. Future directions
We have considered the influence of a donor-controlled resource subsidy on predator–prey interactions by use of a mathematical model. Both a non-spatial model, in which the subsidy occurs in the same location as the predator and prey, and a spatial model, in which the predator must move between two patches (one containing only the prey and the other containing only the subsidy) were considered. Conditions for the feasibility and stability of various equilibrium states were developed for each. In all cases, we find that moderate resource subsidy inputs can promote the stable coexistence of predator and prey, whereas large subsidy inputs can push the prey to extinction.
In our theoretical analysis, we found that the complicated forms of the Routh–Hurwitz conditions precluded a successful local stability analysis for positive equilibria in terms of prey carrying capacity (k), subsidy input rate (i), and predator movement (α). In particular, we were unable to prove that a positive equilibrium is stable in the region of conjectured dynamical stability (the central regions in and ) and unstable in the region of conjectured dynamical instability (the lower-right regions in and ). Yet, we strongly suspect that a positive equilibrium is in fact globally attractive in the former region and that a unique globally attractive limit cycle exists in the latter region.
Our numerical explorations of the non-spatial model (III) indicate that the time required for relaxation to a limit cycle can vary greatly with parameter values. We have even found cases in which a trajectory repeatedly moves from one cycle of finite amplitude to another before finally settling down to the asymptotically attracting cycle. In general, it is unknown how k, i, and α influence the time to relaxation, as well as the amplitude and average predator and prey densities in a limit cycle.
The spatial model (IV) assumes that predator movement occurs via the density-independent process of diffusion. Natural predators probably make use of density-dependent cues as well, involving predator and prey density and subsidy abundance. Whether such rules by themselves can produce dynamical instability remains unknown. Seasonal and migrational effects that produce temporal variation in the predator movement rate (α) may be introduced Citation44. Another extension to the spatial model would be to incorporate allochthonous subsidies transported into several patches differing in prey productivity; it may be that a locally inferior prey species can coexist regionally with a locally superior competitor Citation43.
Below we consider several important modifications to the models that make them more applicable to the arctic system in which arctic foxes (predators) consume both lemmings (prey) and seal carcasses (subsidy).
6.1.1. Seasonal and long-term variability in subsidy amount
In the arctic, seal carcass availability varies in time due to seasonal and long-term changes in temperature, sea ice cover, and polar bear activity. For illustrative purposes, suppose that the mean temperature on day n is given by
6.1.2. Spatial separation of prey and subsidy
Another important feature of the arctic system is that lemmings and seal carcasses are spatially separated, with the former occurring inland and the latter occurring on ice floes surrounding the coastline. In our patch model (IV), the distinct spatial locations are implicit. A more realistic way to include spatial separation of prey and subsidy is to assume that the habitat Ω is a closed and bounded subset of ℝ2 consisting of an inner domain Ω1 on which only the prey occurs and an outer annular domain Ω2 on which only the subsidy occurs. In this case, a system of partial differential equations such as
6.2. Conclusions
Holt Citation25 developed models for two species of immobile prey segregated into two patches coupled by predator movement and suggested that habitat partitioning could be a mechanism allowing alternative prey species to coexist. In these models, one of the prey species may become extirpated by the subsidized predator consuming abundant prey in another patch. In the models considered in the present paper, a highly subsidized predator should indeed drive a prey species to extinction, regardless of whether the prey and subsidy occur in the same location. Though apparent competition Citation24 is still evident in both the spatial and non-spatial models (an increase in subsidy input rate (i) results in lower equilibrium prey density), in the spatial model a predator must travel between patches, which is less efficient than simply consuming both prey and subsidy in the same patch. As such, prey extinction appears more likely in the non-spatial model, in a qualitative sense.
We also find that an increase in predator movement rate (α) can also stabilize the system – a predator having greater access to a subsidy would be more likely to persist when prey are difficult to find. In general, predator movement has a ‘smoothing’ effect, with local differences in patch quality being of less consequence as the movement rate increases. Qualitatively, the two patches behave increasingly as though they were a single patch. Such knowledge can be useful in mitigating local disturbances. For example, in areas of melting coastal ice, foraging arctic foxes must rely increasingly on lemmings inland to survive because of reduced access to seal carcass subsidies along the coast.
Returning to our motivating example, our results suggest that seal carcass availability may have a stabilizing influence on arctic fox and lemming population dynamics – provided that the input rate is relatively moderate. Since arctic foxes must move off the tundra onto the sea ice to exploit the subsidy, our spatial model (IV), in which two distinct habitat patches each contained a different resource for the predator, may better represent this particular biological system. The warming climate and decline in sea ice are already having a negative impact on ringed seals and polar bears Citation14 Citation47, and our results suggest the loss of this subsidy could destabilize the dynamics of coastal fox and lemming populations, in the sense that arctic fox populations relying on the seal subsidy may be greatly reduced in number, or perhaps even pushed to extinction, due to a decline in the subsidy.
Many of our results are qualitatively similar to a previous study using a very different model that found small subsidy inputs stabilized food web dynamics, whereas large subsidy inputs were destabilizing Citation28. However, our model consists of just two trophic levels, with only the top predator receiving additional resources from the subsidy. Previous studies of a non-spatial tritrophic food chain model found the trophic level into which the subsidy entered the community affected species Â' persistence and population-level variance Citation29. A tritrophic model with a spatial component added would likely be analytically intractable, but numerical simulations could be explored to investigate how space and trophic level interact to affect the impact of subsidies on food web stability.
Acknowledgements
We thank J.D. Roth for helping to initiate this project and for comments on early versions of the manuscript. We thank D.J. Kaup and J. Shi for insightful discussions. We thank two anonymous reviewers for their helpful suggestions. R.A. Van Gorder was supported in part by NSF grant DMS-0505566.
Notes
This is a paper based on an invited talk given at the 3rd International Conference on Math Modeling & Analysis, San Antonio, USA, October 2011.
References
- Abdllaoui , A. E. , Auger , P. , Kooi , B. W. , Bravo de la Parra , R. and Mchich , R. 2007 . Effects of density-dependent migrations on stability of a two-patch predator prey model . Math. Biosci. , 210 : 335 – 354 .
- Abrams , P. A. 1999 . Is predator-mediated coexistence possible in unstable systems? Ecology . 80 : 608 – 621 .
- Abrams , P. A. 1999 . The adaptive dynamics of consumer choice . Am. Nat. , 153 : 83 – 97 .
- Abrams , P. A. and Matsuda , H. 1996 . Positive indirect effects between prey species that share predators . Ecology , 77 : 610 – 616 .
- Abrams , P. A. and Matsuda , H. 2003 . Population dynamical consequences of reduced predator switching at low total prey densities . Popul. Ecol. , 45 : 175 – 185 .
- Abrams , P. A. and Roth , J. D. 1994 . The effects of enrichment of three-species food chains with nonlinear functional responses . Ecology , 75 : 1118 – 1130 .
- Abrams , P. A. , Holt , R. D. and Roth , J. D. 1998 . Apparent competition or apparent mutualism? Shared predation when populations cycle . Ecology , 79 : 201 – 212 .
- Ardito , A. and Ricciardi , P. 1995 . Lyapunov functions for a generalized Gause-type model . J. Math. Biol. , 33 : 816 – 828 .
- van Baalen , M. , Krivan , V. , van Rijn , P. C.J. and Sabelis , M. W. 2001 . Alternative food, switching predators, and the persistence of predator–prey systems . Am. Nat. , 157 : 512 – 524 .
- Chesson , P. L. 1984 . Variable predators and switching behavior . Theor. Popul. Biol. , 26 : 1 – 26 .
- Comins , H. N. and Hassell , M. P. 1976 . Predation in multi-prey communities . J. Theor. Biol. , 62 : 93 – 114 .
- Darimont , C. T. , Paquet , P. C. and Reimchen , T. E. 2008 . Spawning salmon disrupt trophic coupling between wolves and ungulate prey in coastal British Columbia . BMC Ecol. , 8 : 1 – 12 .
- Faria , L. D.B. and Costa , M. I.S. 2010 . Omnivorous food web, prey preference and allochthonous nutrient input . Ecol. Complexity , 7 : 107 – 114 .
- Ferguson , S. H. , Stirling , I. and McLoughlin , P. 2005 . Climate change and ringed seal (Phoca hispida) recruitment in western Hudson Bay . Mar. Mamm. Sci. , 21 : 121 – 135 .
- Gantmacher , F. R. 1960 . The Theory of Matrices , Vol II , New York : Chelsea Pub. Co .
- Garrott , R. A. , Bruggeman , J. E. , Becker , M. S. , Kalinowski , S. T. and White , P. J. 2007 . Evaluating prey switching in wolf ungulate systems . Ecol. Appl. , 17 : 1588 – 1597 .
- Gause , G. F. , Smaragdova , N. P. and Witt , A. A. 1936 . Further studies of interaction between predator and prey . J. Anim. Ecol. , 5 : 1 – 18 .
- Gilpin , M. and Rosenzweig , M. 1972 . Enriched predator–prey systems: Theoretical stability . Science , 177 : 902 – 904 .
- Gurney , W. S.C. and Nisbet , R. M. 1998 . Ecological Dynamics , New York : Oxford University Press .
- Halaj , J. and Wise , D. H. 2002 . Impact of a detrital subsidy on trophic cascades in a terrestrial grazing food web . Ecology , 83 : 3141 – 3151 .
- Hale , J. and Kocak , H. 1991 . Dynamics and Bifurcations , New York : Springer Verlag .
- Henden , J.-A. , Ims , R. A. , Yoccoz , N. G. , Hellstrom , P. and Angerbjorn , A. 2010 . Strength of asymmetric competition between predators in food webs ruled by fluctuating prey: The case of foxes in tundra . Oikos , 119 : 27 – 34 .
- Hofbauer , J. and Sigmund , K. 1998 . Evolutionary Games and Population Dynamics , Cambridge : Cambridge University Press .
- Holt , R. D. 1977 . Predation, apparent competition, and the structure of prey communities . Theor. Popul. Biol. , 12 : 197 – 229 .
- Holt , R. D. 1984 . Spatial heterogeneity, indirect interactions, and the coexistence of prey species . Am. Nat. , 124 : 377 – 406 .
- Holt , R. D. 1984 . Population dynamics in two patch environments – some anomalous consequences of an optimal habitat distribution . Theor. Popul. Biol. , 28 : 181 – 208 .
- Huang , Y. and Diekmann , O. 2001 . Predator migration in response to prey density: What are the consequences? J. Math. Biol . 43 : 561 – 581 .
- Huxel , G. R. and McCann , K. 1998 . Food web stability: The influence of trophic flows across habitats . Am. Nat. , 152 : 460 – 469 .
- Huxel , G. R. , McCann , K. and Polis , G. A. 2002 . Effects of partitioning allochthonous and autochthonous resources on food web stability . Ecol. Res. , 17 : 419 – 432 .
- Jefferies , R. L. 2000 . Allochthonous inputs: Integrating population changes and food-web dynamics . Trends Ecol. Evol. , 15 : 19 – 22 .
- Khan , Q. J.A. , Balakrishnan , E. and Wake , G. C. 2004 . Analysis of a predator prey system with predator switching . Bull. Math. Biol. , 66 : 109 – 123 .
- Kimbrell , T. and Holt , R. D. 2005 . Individual behaviour, space and predator evolution promote persistence in a two-patch system with predator switching . Evol. Ecol. Res. , 7 : 53 – 71 .
- Krivan , V. and Eisner , J. 2006 . The effect of the Holling type II functional response on apparent competition . Theor. Popul. Biol. , 70 : 421 – 430 .
- Kolmogorov , A. N. 1936 . Sulla teoria di Volterra della lotta per l'esistenza (On Volterra's theory of the struggle for existence) . Giornale dell Istituto Italiano Degli Attuari , 7 : 74 – 80 .
- Kuang , Y. and Freedman , H. I. 1988 . Uniqueness of limit cycles in Gause-type models of predator–prey systems . Math. Biosci. , 88 : 67 – 84 .
- Kuang , Y. and Takeuchi , Y. 1994 . Predator–prey dynamics in models of prey dispersal in two-patch environments . Math. Biosci. , 120 : 77 – 98 .
- Leroux , S. J. and Loreau , M. 2008 . Subsidy hypothesis and strength of trophic cascades across ecosystems . Ecol. Lett. , 11 : 1147 – 1156 .
- Marczak , L. B. , Thompson , R. M. and Richardson , J. S. 2007 . Meta-analysis: Trophic level, habitat, and productivity shape the food web effects of resource subsidies . Ecology , 88 : 140 – 148 .
- May , R. 1972 . Limit cycles in predator–prey communities . Science , 177 : 900 – 902 .
- Mukhopadhyay , B. and Bhattacharyya , R. 2008 . Bifurcation analysis of an ecological food-chain model with switching predator . Appl. Math. Comput. , 201 : 260 – 271 .
- Murdoch , W. W. 1969 . Switching in general predators: Experiments on predator specicity and stability of prey populations . Ecol. Monogr. , 39 : 335 – 354 .
- Murdoch , W. W. and Oaten , A. 1975 . Predation and population stability . Adv. Ecol. Res. , 9 : 1 – 131 .
- Namba , T. 2007 . Dispersal-mediated coexistence of indirect competitors in source-sink metacommunities . Japan J. Indust. Appl. Math. , 24 : 39 – 55 .
- Namba , T. and Takahashi , S. 1993 . Competitive coexistence in a seasonally fluctuating environment II. Multiple stable states and invasion success . Theor. Popul. Biol. , 44 : 374 – 402 .
- A.L. Nevai and J. Shi, manuscript in preparation (2012).
- Polis , G. A. , Hurd , S. D. , Jackson , C. T. and Pinero , F. S. 1997 . El Nino effects on the dynamics and control of an island ecosystem in the Gulf of California . Ecology , 78 : 1884 – 1897 .
- Regehr , E. V. , Lunn , N. J. , Amstrup , S. C. and Stirling , L. 2007 . Effects of earlier sea ice breakup on survival and population size of polar bears in western Hudson Bay . J. Wildlife Manage. , 71 : 2673 – 2683 .
- Rinaldi , S. and Gragnani , A. 2004 . Destabilizing factors in slow fast systems . Ecol. Model. , 180 : 445 – 460 .
- Rinaldi , S. , Muratori , S. and Kuznetsov , Y. 1993 . Multiple attractors, catastrophes and chaos in seasonally perturbed predator–prey communities . Bull. Math. Biol , 55 : 15 – 35 .
- Rose , M. D. and Polis , G. A. 1998 . The distribution and abundance of coyotes: The effects of allochthonous food subsidies from the sea . Ecology , 79 : 998 – 1007 .
- Rosenzweig , M. 1971 . The paradox of enrichment: Destabilization of exploitation ecosystems in ecological time . Science , 171 : 385 – 387 .
- Roth , J. D. 2002 . Temporal variability in arctic fox diet as reflected in stable-carbon isotopes; the importance of sea ice . Oecologia , 133 : 70 – 77 .
- Roth , J. D. 2003 . Variability in marine resources affects arctic fox population dynamics . J. Anim. Ecol. , 72 : 668 – 676 .
- Roy , S. and Chattopadhyay , J. 2007 . The stability of ecosystems: A brief overview of the paradox of enrichment . J. Biosci. , 32 : 421 – 428 .
- Shurin , J. B. and Seabloom , E. W. 2005 . The strength of trophic cascades across ecosystems: Predictions from allometry and energetics . J. Anim. Ecol. , 74 : 1029 – 1038 .
- Srinivasu , P. D.N. , Prasada , B. S.R.V. and Venkatesulub , M. 2007 . Biological control through provision of additional food to predators: A theoretical study . Theor. Popul. Biol. , 72 : 111 – 120 .
- Takimoto , G. , Iwata , T. and Murakami , M. 2002 . Seasonal subsidy stabilizes food web dynamics: Balance in a heterogeneous landscape . Ecol. Res. , 17 : 433 – 439 .
- Takimoto , G. , Iwata , T. and Murakami , M. 2009 . Timescale hierarchy determines the indirect effects of fluctuating subsidy inputs on in situ resources . Am. Nat. , 173 : 200 – 211 .
- Turchin , P. and Taylor , A. D. 1992 . Complex dynamics in ecological time series . Ecology , 73 : 289 – 305 .
- Vance , R. R. 1978 . Predation and resource partitioning in one predator-two prey model communities . Am. Nat. , 112 : 797 – 813 .
- Volterra , V. 1931 . “ Variations and fluctuations in the number of individuals in animal species living together ” . In Animal Ecology , Edited by: Chapman , R. N. New York : McGraw-Hill .
- Wang , W. and Takeuchi , Y. 2009 . Adaptation of prey and predators between patches . J. Theor. Biol. , 258 : 603 – 613 .
Appendix. Global asymptotic stability of positive equilibrium for predator-subsidy system (II)
Here, we prove that the positive equilibrium of the predator-subsidy system (II) is globally asymptotically stable when . Adapting an argument from Ardito and Ricciardi Citation8 (they consider a logistic-type resource rather than a donor-controlled one), let