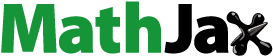
ABSTRACT
A model of an Ebola epidemic is developed with infected individuals structured according to disease age. The transmission of the infection is tracked by disease age through an initial incubation (exposed) phase, followed by an infectious phase with variable transmission infectiousness. The removal of infected individuals is dependent on disease age, with three types of removal rates: (1) removal due to hospitalization (isolation), (2) removal due to mortality separate from hospitalization, and (3) removal due to recovery separate from hospitalization. The model is applied to the Ebola epidemics in Sierra Leone and Guinea. Model simulations indicate that successive stages of increased and earlier hospitalization of cases have resulted in mitigation of the epidemics.
1. Introduction
The 2014–2015 Ebola epidemics in West Africa have been mathematically modelled in many studies with attention to various aspects of the disease epidemiology ([Citation1–30,Citation32,Citation33,Citation37]). In this study we focus attention on the variability of infectiousness of infected individuals throughout their disease course. It is recognized that the period of infectiousness follows a period of incubation lasting from 2 to 21 days, begins with the presentation of symptoms, and that the level of infection transmission coincides with the severity of symptoms [Citation35]. Our analysis of infection transmission is based on the disease age of an infected individual. Here disease age means the time since infection acquisition, which is assigned disease age 0. We assume that the disease age distribution of the level of infectiousness is a Gaussian distribution, rising gradually, peaking, and falling gradually for survivors. The value of tracking disease course by disease age is that removal of infected individuals from the epidemic population can be tied to their level of infectiousness, and earlier removal can have significant benefit for mitigation of the epidemic.
The Ebola epidemics in West Africa have developed with rapid increase in the cumulative number of cases from the spring of 2014 to the present. The most recent WHO data indicate that the epidemics may be subsiding [Citation34]. In this study we analyse the dynamics of the epidemics in Sierra Leone and Guinea with a disease age model. Our goal is to connect the removal rates of infected individuals to the epidemic outcomes. Our simulations indicate that the mitigation of the epidemics is tied to an increased level and earlier hospitalization (isolation) of infectious individuals as the epidemics unfolded. This enhanced hospitalization of identified cases can be tied to increased contact tracing, social awareness, and public health policies. The measure of these epidemics is tracked by the net reproductive values derived from the models. Our simulations show that for both Sierra Leone and Guinea
has decreased significantly to levels which indicate that the epidemics will subside in 2015. We fit our disease age structured model simulations to cumulative case data and extend our simulations forward in time to project the epidemic levels into 2015.
2. The Ebola model with age of infection
The model consists of the epidemic population divided into susceptible , infected
, and removed classes
at time t, with the key feature that infected individuals are structured by a continuous variable a corresponding to time since acquisition. The age of infection is divided into an early stage corresponding to an incubation period, and intermediate stage corresponding to an infectious period, and a late stage corresponding to a recovering period, as determined by the disease age of infected individuals.
Initially there are
susceptible individuals with a small number of infected individuals. The results are valid for any number of initial susceptibles with a given parameterization.
The disease phases of an infected individual are governed by a Gaussian distribution of disease age a. This Gaussian form is consistent with reported cases having a gradual on-set and gradual diminishing of infectiousness. The Gaussian distribution has mean
, standard deviation
, and is multiplied by a scaling factor
. It is assumed that the on-set of symptoms (coincident with infectiousness) is gradual after an incubation (exposed) phase. The values
and
should be viewed as effective average values for a typical infected individual.
Infectious individuals are removed due to hospitalization/isolation at a rate
per day after disease age
. This rate takes into account time lags in identifying presentation of symptoms.
Infectious individuals are removed due to unreported mortality (independent of hospitalization) at a rate
per day after disease age
. This rate allows for delays resultant from improperly handled corpses.
Infectious individuals are (effectively) removed due to recovery (independent of hospitalization) at a rate
per day after disease age
. The values of
and
are significantly greater than the value of
.
Infectious individuals are removed as a result of increased and earlier hospitalization at a rate
per day dependent on disease age a and time
during the course of the epidemic. The time
is chosen based on case data indicating a lessening of the cumulative number of cases. We allow for a succession of times
, when additional increased and earlier hospitalization occurs during the course of the epidemic.
The formulas for ,
,
,
are
(1)
(1)
(2)
(2)
(3)
(3)
(4)
(4)
The probabilities of removal by disease age a for , derived in Appendix 1, are
3. The equations of the model
The equations of the model are based on the disease age density , where a is disease age and t is time. Infected individuals have disease age 0 at time of acquisition. The number of exposed (incubating) infected individuals at time t is
, where
is the approximate disease age at which the infectious phase begins. The number of infectious individuals at time t is
, where
is the approximate disease age at which infectiousness ends. The number of susceptible individuals at time t is
. The model equations are
(5)
(5)
(6)
(6)
(7)
(7)
(8)
(8)
(9)
(9)
An analysis of Equations (Equation5(5)
(5) )–(Equation9
(9)
(9) ) and the formulas below are given in [Citation31]. The ultimate course of the epidemic satisfies
and
. The reproduction number of the epidemic changes at each time point
, when additional increased and earlier hospitalization occurs. The updated reproduction number at these time points take into account the time-dependent removal rates and the decreased susceptible population. The reproduction number
of the model before time
is
(10)
(10) and for
,
is
(11)
(11)
The total cumulative number of cases at time t, both hospitalized (reported) and non-hospitalized (unreported) is
The epidemic attack ratio at time
is
The cumulative number of hospitalized cases at time
, is
The cumulative number of unreported cases at time t is
. The cumulative number of mortality cases (independent of hospitalization) at time
, is
The cumulative number of recovered cases (independent of hospitalization) at time
is
Similar formulas can be obtained for
,
,
, and
for
.
4. Simulations of the model for the Ebola epidemic in Sierra Leone
The model (Equation5(5)
(5) )–(Equation9
(9)
(9) ) is simulated for the 2014–2015 Ebola epidemic in Sierra Leone. For this simulation the epidemic is divided into 3 phases: 27 May 2014 to 1 September 2014, 1 September to 14 December 2014, and 14 December 2014 forward in time. The simulation phase time values are
1 September 2014 and
14 December 2014, where each phase represents an increased removal of cases due to a greater number of cases hospitalized, with earlier hospitalization. The removal rates
,
,
hold for the first, second, and third phases (
). The removal rate
holds for the second and third phases (
). Additionally, there is the removal rate
holding for the third phase
having the form
(12)
(12)
The parameters for the simulation are given in Table . The model parameters are based on cumulative reported case data from WHO situation reports [Citation34]. In Appendix 2, the mean time from symptom to hospitalization is calculated during the three epidemic phases utilizing our parameters, and these values coincide well with estimates derived from patient databases in [Citation36]. The graph of the disease age-dependent transmission function is shown in Figure . The simulation of the model is illustrated in Figure . The phase times
and
are chosen based on fitting this data to a solution of the logistic equation. The concavity change in the data corresponds to the transition over time of
from
to
, which corresponds in turn to the concavity change in the fitted logistic equation solution. The logistic equation and its solution are
(13)
(13) The dashed graph in Figure is the solution of Equation (Equation13
(13)
(13) ) with
,
, and
. The dashed vertical line in Figure is the concavity change in
at
,
. In Figure the simulation is projected forward in time to 1 June 2015. The dependence of
during the first phase between 27 May 2014 and 1 September 2014 as a function of
(the earliest disease age for hospitalization in the first phase) and
(the hospitalization rate after disease age
in the first phase) is illustrated in Figure (all other parameters are as in Table ). Figure demonstrates the importance of smaller values of
and larger values of
for mitigation of the epidemic. The simulation for Sierra Leone is summarized in Table .
Figure 1. The disease age infection transmission function for Sierra Leone with
,
,
, and the removal rate start ages
,
,
,
. The infectious phase begins at
days and ends at
days.
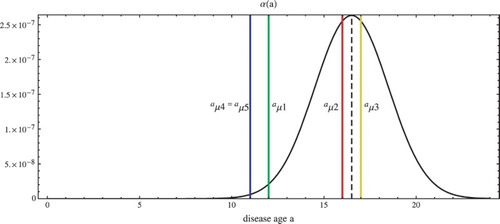
Figure 2. Simulation of the Ebola epidemic in Sierra Leone with the parameters in Table . The dots are reported cumulative case data [Citation34]. The red graph is reported cumulative cases from the simulation. The blue graph is the total cumulative cases from the simulation (both reported and unreported). between May 27, 2014 and September 1, 2014.
between September 1 and December 14, 2014.
between December 14, 2014 and forward in time.
![Figure 2. Simulation of the Ebola epidemic in Sierra Leone with the parameters in Table 1. The dots are reported cumulative case data [Citation34]. The red graph is reported cumulative cases from the simulation. The blue graph is the total cumulative cases from the simulation (both reported and unreported). R0=1.77 between May 27, 2014 and September 1, 2014. R0=1.10 between September 1 and December 14, 2014. R0=0.72 between December 14, 2014 and forward in time.](/cms/asset/16544703-8911-481e-b307-efd9e75d5e9b/tjbd_a_1090632_f0002_c.jpg)
Figure 3. for Sierra Leone during the first phase between May 27, 2014 and September 1, 2014 as a function of
(the earliest disease age for hospitalization) and
(the hospitalization parameter) with all other parameters as in Table . The blue surface is
and the red plane is
. The yellow dot corresponds to the Table values
days,
per day, and
.
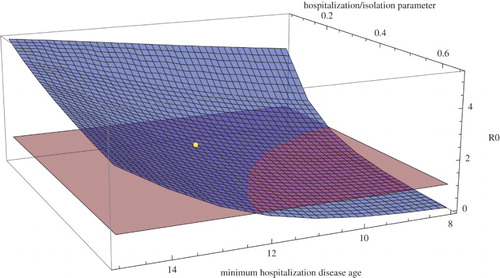
Table 1. Parameters of the model for the Ebola Epidemic in Sierre Leone.
Table 2. Summary of the simulations for Sierre Leone and Guinea.
5. Simulations of the model for the Ebola epidemic in Guinea
The model (Equation5(5)
(5) )–(Equation9
(9)
(9) ) is simulated for the 2014–2015 Ebola epidemic in Guinea. The parameters for the model simulation are chosen as for Sierra Leone, based on WHO cumulative reported case data [Citation34]. For this simulation the epidemic is divided into three phases: 26 March 2014 to 28 September 2014, 28 September 2014 to 21 December 2014, and 21 December 2014 forward in time. The simulation phase time values are
28 September 2014 and
21 December 2014. The parameterization for the simulation is the following:
;
is the same as for Sierra Leone (Table ); the disease age-dependent transmission rate
is the same as for Sierra Leone (Table ) except
;
,
,
,
,
are the same as for Sierra Leone (Table ); the removal rate parameters are
,
,
,
,
. The logistic equation parameters in Equation (Equation13
(13)
(13) ) are
,
, and
, with concavity change at
,
. The simulation of the model is illustrated in Figure . In Figure the simulation is projected forward in time to 1 June 2015. The dependence of
during the first phase between 26 March 2014 and 28 September 2014 as function of
(the earliest disease age for hospitalization in the first phase) and
(the parameter for hospitalization after age disease
in the first phase) is illustrated in Figure . The simulation for Guinea is summarized in Table .
Figure 4. Simulation of the Ebola epidemic in Guinea. The dots are reported cumulative case data [Citation34]. The red graph is reported cumulative cases from the simulation. The blue graph is the total cumulative cases from the simulation (both reported and unreported). between March 26, 2014 and September 28, 2014.
between September 28 and December 21, 2014.
between December 21, 2014 and forward in time.
![Figure 4. Simulation of the Ebola epidemic in Guinea. The dots are reported cumulative case data [Citation34]. The red graph is reported cumulative cases from the simulation. The blue graph is the total cumulative cases from the simulation (both reported and unreported). R0=1.22 between March 26, 2014 and September 28, 2014. R0=1.00 between September 28 and December 21, 2014. R0=0.85 between December 21, 2014 and forward in time.](/cms/asset/8fac1bd9-2549-47c8-bc62-1fb1260f7989/tjbd_a_1090632_f0004_c.jpg)
Figure 5. for Guinea during the first phase between March 26, 2014 and September 28, 2014 as a function of
(the earliest disease age for hospitalization) and
(the hospitalization parameter) with all other parameters as in Figure . The blue surface is
and the red plane is
. The yellow dot corresponds to the values
days,
, and
.
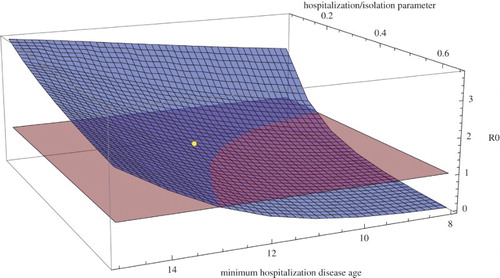
6. Discussion
We have presented a model of the 2014–2015 Ebola epidemics in Sierra Leone and Guinea structured by the disease age of infected individuals. The incorporation of disease age allows refinement of the disease transmission rate based on level of infectiousness. For Ebola the level of infectiousness is correlated to the level of symptoms, which begin gradually over a period of several days. An accurate description of infectiousness levels is critical for evaluating intervention measures such as contact tracing and case isolation in hospitals, Ebola treatment units, and holding centres. The incorporation of disease age also allows refinement of removal rates due to hospitalization, mortality, and recovery correlated to disease age. Consequently, the significant advantage in hospitalization/isolation of infected individuals as soon as possible after presentation of symptoms is emphasized.
Our model parameters are based on cumulative reported case data [Citation34] through 22 February 2015 and projected forward to 1 June 2015. The parameter values were checked for sensitivity and determined not to be overly sensitive to model output. The numerical computations are extremely intensive, and the MATHEMATICA computer code developed for the simulations is available on request to the authors. The data indicate that the Ebola epidemics have subsided and will further subside to effective elimination in 2015 for these two countries. The reasons for the extinction of these Ebola epidemics are complex, but increased identification and isolation of infectious cases played a major role. Our model quantifies the significance of this increased identification/isolation. One way to quantify an epidemic is the epidemic net reproduction number , which is dynamic and evolves with changes in public health interventions and societal behaviour. In our simulations, we modelled this
dynamic with two changes in hospitalization/isolation rates in two successive time periods. These changes correspond to increased social awareness and improved public health response measures. Our model simulations indicate that this enhanced removal of infectious individuals is key to elimination of the epidemic. This removal is quantified in our model in two ways: (1) with respect to the rate of infectious individuals hospitalized per day, and (2) with respect to an earlier disease age of the infectious individuals hospitalized. Both of these considerations are critical for epidemic control and both can be influenced by public health policies and public awareness.
Disclosure statement
No potential conflict of interest was reported by the authors.
References
- C.L. Althaus, Estimating the reproduction number of Zaire ebolavirus (EBOV) during the 2014 outbreak in West Africa, PLOS Currents Outbreaks, September 2, 2014.
- S.E. Bellan, J.R.C. Pulliam, J. Dushoff, and L.A. Meyers, Ebola control: Effect of asymptomatic infection and acquired immunity, Lancet 384 (2014), pp. 1499–1500. doi: 10.1016/S0140-6736(14)61839-0
- H.A. Bolkan, D.A. Bash-Taqi, M. Samai, M. Gerdin, and J. von Schreeb, Ebola and Indirect Effects on Health Service Function in Sierra Leone, PLOS Currents Outbreaks, December 19, 2014.
- C. Browne, H. Gulbudak, and G. Webb, Modeling contact tracing in outbreaks with application to Ebola, J. Theoret. Biol. 384 (2015), pp. 33–49.
- A. Camacho, A. Kucharski, Y. Aki-Sawyerr, M.A. White, S. Flasche, M. Baguelin, T. Pollington, J. R. Carney, R. Glover, E. Smout, A. Tiffany, W. J. Edmunds, and S. Funk, Temporal changes in Ebola transmission in Sierra Leone and Iimplications for control requirements: A real-time modelling study, PLOS Currents Outbreaks, February 10, 2015.
- CDC, Center for disease control and Prevention, What is contact tracing? Contact tracing can stop the Ebola outbreak in its track. Available at http://www.cdc.gov/vhf/ebola/pdf/contact-tracing.pdf.
- D. Chowell, C. Castillo-Chavez, S. Krishna, X. Qiu, and K.S. Anderson, Modelling the effect of early detection of Ebola, Lancet, 15, February 2015.
- G. Chowell, N.W. Hengartner, C. Castillo-Chavez, P.W. Fenimore, and J.M. Hyman, The basic reproductive number of Ebola and the effects of public health measures: the cases of Congo and Uganda, J. Theoret. Biol. 229 (2004), pp. 119–126. doi: 10.1016/j.jtbi.2004.03.006
- G. Chowell and H. Nishiura, Transmission dynamics and control of Ebola virus disease (EVD): A review, BMC Med. 12 (2014), p. 196. doi: 10.1186/s12916-014-0196-0
- G. Chowell, L. Simonsen, C. Viboud, and Y. Kuang, Is West Africa approaching a catastrophic phase or is the 2014 Ebola epidemic slowing down? Different models yield different answers for Liberia, MIT Press, Cambridge, MA, 1984.
- G. Chowell, C. Viboud, J.M. Hyman, and L. Simonsen, The Western Africa Ebola virus disease epidemic exhibits both global exponential and local polynomial growth rates, PLOS Currents Outbreaks, January 21, 2015.
- D. Fisman, E. Khoo, and A. Tuite, Early epidemic dynamics of the West African 2014 Ebola outbreak: Estimates derived with a simple two-parameter model, PLOS Currents Outbreaks, September 8, 2014.
- M. Gomes, A. Pastore, y. Piontti, L. Rossi, D. Chao, I. Longini, M. Halloran, and A. Vespignani, Assessing the international spreading risk associated with the 2014 West African Ebola outbreak, PLOS Currents Outbreaks, September 2, 2014.
- C.N. Haas, On the quarantine period for Ebola virus, PLOS Currents Outbreaks, October 14, 2014.
- H. Inaba. and H. Nishiura, The state-reproduction number for a multistate class age structured epidemic system and its application to the asymptomatic transmission model, Math. Biosci. 216 (2008), pp. 77–89. doi: 10.1016/j.mbs.2008.08.005
- M. Kiskowski, A three-scale network model for the early growth dynamics of 2014 West Africa Ebola epidemic, PLOS Currents Outbreaks, November 13, 2014.
- K. Kuferschmidt, Estimating the Ebola epidemic, Science 345 (2014), pp. 1108–1109. doi: 10.1126/science.345.6201.1108
- A.J. Kucharski and W.J. Edmunds, Case fatality rate for Ebola virus disease in West Africa, Lancet 384 (2014). doi: 10.1016/S0140-6736(14)61706-2
- J. Legrand, R.F. Grais, P.Y. Boelle, A.J. Valleron, and A. Flahault, Understanding the dynamics of Ebola epidemics, Epidemiol. Infect. 135(4) (2007), pp. 610–621. doi: 10.1017/S0950268806007217
- P.E. Lekone and B.F. Finkenstadt, Statistical inference in a stochastic epidemic SEIR model with control intervention: Ebola as a case study, Biometrics 62 (2006), pp. 1170–1177. doi: 10.1111/j.1541-0420.2006.00609.x
- J.A. Lewnard, M.L. Ndeffo Mbah, J.A. Alfaro-Murillo, F.L. Altice, L. Bawo, T.G. Nyenswah, and A.P. Galvani, Dynamics and control of Ebola virus transmission in Montserrado, Liberia: a mathematical modelling analysis, Lancet Infect. Dis 14(12) (2014), pp. 1189–1195. doi: 10.1016/S1473-3099(14)70995-8
- S. Merler, M. Ajelli, L. Fumanelli, M. Gomes, A. Piontti, L. Rossi, D.L. Chao, I.M. Longini, M.E. Halloran, and A. Vespignani, Spatiotemporal spread of the 2014 outbreak of Ebola virus disease in Liberia and the effectiveness of non-pharmaceutical interventions: A computational modelling analysis, Lancet Infect. Dis. January 6, 2015.
- M. Meltzer, C. Atkins, S. Santibanez, B. Knust, B. Petersen, E. Ervin, S. Nichol, I. Damon, and M. Washington, Estimating the future number of cases in the Ebola epidemic Liberia and Sierra Leone, 20142015, CDC Centers for Disease Control and Prevention, September 26, 63 (2014), pp. 1–14.
- H. Nishiura and G. Chowell, Early transmission dynamics of Ebola virus disease (EVD), West Africa, March to August 2014, Eurosurveil. 19(36) (2014). doi: 10.2807/1560-7917.ES2014.19.36.20894
- A. Pandey, K.E. Atkins, J. Medlock, N. Wenzel, J.P. Townsend, J.E. Childs, T.G. Nyenswah, M.L. Ndeffo-Mbah, and A.P. Galvani, Strategies for containing Ebola in West Africa, published online in Science, October 30, 2014.
- C. Rivers, E. Lofgren, M. Marathe, S. Eubank, and B. Lewis, Modeling the impact of interventions on an epidemic of Ebola in Sierra Leone and Liberia, PLOS Currents Outbreaks, November 6, 2014.
- J. Shaman, W. Yang, and S. Kandula, Inference and forecast of the current West African Ebola outbreak in Guinea, Sierra Leone and Liberia, PLOS Currents Outbreaks, October 31, 2014.
- T. Stadler, D. Kuhnert, D.A. Rasmussen, and L. du Plessis, Insights into the early epidemic spread of Ebola in Sierra Leone provided by viral sequence data, PLOS Currents Outbreaks, October 6, 2014.
- S. Towers, O. Patterson-Lomba, and C. Castillo-Chavez, Temporal Variations in the Effective Reproduction Number of the 2014 West Africa Ebola Outbreaks, PLOS Currents Outbreaks. 2014 September 18. 2014.
- E. Volz and S. Pond, Phylodynamic analysis of Ebola virus in the 2014 Sierra Leone epidemic, PLOS Currents Outbreaks, October 24, 2014.
- G.F. Webb, M. Blaser, Y.-H. Hsieh, and J. Wu, Pre-symptomatic influenza transmission, surveillance, and school closings: Implications for novel influenza A(H1N1), Math. Mod. Nat. Phen. 3 (2010), pp. 191–205. doi: 10.1051/mmnp/20105312
- G.F. Webb, C. Browne, X. Huo, O. Seydi, M. Seydi, and P. Magal, A model of the 2014 Ebola epidemic in West Africa with contact tracing, PLOS Currents Outbreaks, January 30, 2015.
- R.A. White, E. MacDonald, B. Freiesleben de Blasio, K. Nygrd, L. Vold, and J.-A. Rttingen, Projected treatment capacity need is Sierra Leone, PLOS Currents Outbreaks, January 30, 2015.
- World Health Organization, Situation reports: Ebola response roadmap, Global Alert and Response (GAR), February 25, 2015, http://apps.who.int/ebola/en/current-situation/ebola-situation-report.
- World Health Organization, Ebola Response Team, Ebola virus disease in West Africa -The first 9 months of the epidemic and forward projections, N. Engl. J. Med, September 23, 2014.
- C.J.M. Whitty, J. Farrar, N. Ferguson, W.J. Edmunds, P. Piot, M. Leach, and S.C. Davies, Infectious disease: tough choices to reduce ebola transmission, Nature 515 (2014), pp. 192–194. doi: 10.1038/515192a
- D. Yamin, S. Gertler, M.L. Ndeffo-Mbah, L.A. Skrip, M.a. Fallah, T.G. Nyenswah, F.L. Altice, and A.P. Galvani, Effect of Ebola progression on transmission and control in Liberia, Ann. Int. Med. 162 (2015), pp. 11–17. doi: 10.7326/M14-2255
Appendix 1. Probability of removal
We derive the probabilities of removal by disease age a for , which is denoted by
. Consider
, the probability of hospitalization by infection age a for an infected individual (when
). This can be calculated by considering the fraction of a cohort of infected individuals who are hospitalized. Consider an initial cohort of infected,
. Let
denote the cumulative number of hospitalized before infection age a. Then the following equations hold:
Then
, where
. Therefore
For an initial cohort, we consider
, where
is the Dirac delta mass centred at 0. Thus
Appendix 2. Mean time from symptom onset to hospitalization
Here we assume that the removal rates correspond to independent exponential processes. In particular, for , the probability of hospitalization by age a (independent of the other removal rates) is given by the cumulative distribution function,
, for the random variable,
, corresponding to age of hospitalization is given by the following formula:
Then the probability density function for
is
Therefore the average age of hospitalization,
, is given by
With the definition of
as given by Equation (Equation1
(1)
(1) ), we obtain
Then, for
(before improved reporting), the mean time from symptom onset to hospitalization is
where
is the length of incubation period. In our work, we have
. Thus with the parameters utilized for Sierra Leone in Table , we find
. This is approximately equal to the 5 days estimated for the time from symptom onset to hospitalization during the early phase of the epidemic in [Citation36]. From a similar calculation, for
, we find that the mean time from symptom onset to hospitalization is
Thus, with the parameters in Table , we find
. For
, we find that the mean time from symptom onset to hospitalization is
. Again this coincides nicely with results from [Citation36], in which the mean time from symptom onset to hospitalization during the later stages of the epidemic is found to be between 3 and 4 days.