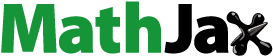
ABSTRACT
One crucial condition for the uniqueness of Nash equilibrium set in vaccination games is that the attack ratio monotonically decreases as the vaccine coverage level increasing. We consider several deterministic vaccination models in homogeneous mixing population and in heterogeneous mixing population. Based on the final size relations obtained from the deterministic epidemic models, we prove that the attack ratios can be expressed in terms of the vaccine coverage levels, and also prove that the attack ratios are decreasing functions of vaccine coverage levels. Some thresholds are presented, which depend on the vaccine efficacy. It is proved that for vaccination games in homogeneous mixing population, there is a unique Nash equilibrium for each game.
1. Introduction
Game theory has been applied to predict human behaviour in the context of epidemiology for a long time. People choose the best strategy to maximize their own payoffs, based on the outcomes of different strategies. Before the outbreak of the epidemic, every individual can choose vaccination, or undertake the risk of infection. Ultimately, a certain percentage of people goes into the vaccinated class, while others remain in the susceptible class. Under these circumstances, the Nash equilibrium is reached; anyone adopting a different strategy will have a lower payoff.
The first paper to discuss game theory and epidemiology is [Citation14] by Fine and Clarkson in 1986. They discussed how individuals decide whether or not to accept vaccination based on their perception of the risks involved. The conclusion of this paper is that rational informed individuals choose a lower vaccine uptake than would the community if it acted as a whole. The topic was also discussed independently by Brito et al. in [Citation10] in 1991. Bauch and Earn described a vaccination game in their PNAS paper [Citation3]. They characterized the Nash equilibrium of the game, proved that the Nash equilibrium is convergently stable; then they used the epidemiological model to make more precise predictions of the expected vaccine coverage level. In many publications, it has been mentioned that if individuals are driven solely by self-interest, the vaccine coverage level of the whole population will be suboptimal, and this level is lower than the herd immunity threshold value (see [Citation3,Citation4,Citation15,Citation18,Citation19,Citation21]). It was also mentioned that in the simple vaccination game for an endemic disease in a homogeneous mixing population, there is a unique Nash equilibrium behaviour in [Citation18] by Reluga. One crucial condition for uniqueness is that the eventual attack ratio of an individual must decrease strictly with the vaccine coverage level [Citation3]. The monotonicity of attack ratio is our focus in this paper. For the case of heterogeneous mixing population, in [Citation18], Reluga considered an epidemiological game with two interacting sub-populations of equal size. He proved that if the mixing is positively assortative, there is a unique stable Nash equilibrium, while if the mixing is disassortative, this may lead to a condition of multiple Nash equilibria. It was proved that the interior Nash equilibria are always unstable. The mixing pattern of this heterogeneous mixing population is the key factor [Citation18]. Chen also pointed out the potential for non-uniqueness in [Citation11].
Along with the growth of interest in game theory and epidemiology, game theory has been applied to many types of infectious diseases. In [Citation22], from the viewpoint of parents, how to make decision on Measles–Mumps–Rubella (MMR) vaccination for their children? Although scientific evidences showed that the MMR vaccine is very safe [Citation12], there are still vaccine sceptics. There are other publications discussing the strategies against influenza [Citation13,Citation15,Citation20,Citation24], smallpox [Citation4], chickenpox [Citation16], measles [Citation22], rubella [Citation23] and other infectious diseases. Other aspects or factors of epidemiology-game theory are also discussed in many publications.
The analysis in this paper is established on the final size relations of several deterministic epidemic models. Final size relation is a very useful tool to predict how serious an epidemic can be, and can be obtained from the epidemic model. The final size relation to the simple susceptible-infected-recovered (SIR) model is
with
being the initial size of the susceptible class, N size of the whole population,
the basic reproduction number. More details can be found in papers [Citation5,Citation6]. This relation can be used to estimate
and the attack ratio. The same technique can be used to derive the final size relations to more complicated deterministic epidemic models. A final size relation was derived for a general class of deterministic models which includes multiple susceptible classes in [Citation1] by Arino et al. For age-of-infection epidemic models, Brauer formulated the final size relation to this type of models in [Citation7]. Brauer also formulated the final size relation to the epidemic model with heterogeneous mixing and treatment in [Citation8]. For the most general age-of-infection models with heterogeneous mixing, Brauer and Watmough formulated the final size relations in [Citation9].
The paper consists of five parts. In Section 2, we demonstrate two vaccination games in homogeneous mixing population and show why monotonicity of attack ratio is important in the analysis. Vaccination games in heterogeneous mixing population are more complicated to analyse completely. In Section 3, the focus is on the homogeneous mixing population, we discuss the relation of ,
and vaccine coverage level p, and the relation between attack ratios and p, some thresholds are discussed. This part of work is able to prove the uniqueness of Nash equilibria in games which we constructed in Section 2. In Section 4, two-subgroup heterogeneous mixing population is analysed, similar analysis is conducted. We use notations
and
in the analysis of heterogeneous mixing population, with the subscript i indicating the subgroup in the population. In Section 5, some possible extensions are discussed. Because of the similarity of forms of final size relations, it is possible to apply the results to the general compartmental deterministic epidemic models. Finally, we propose several possible future work.
2. Vaccination games in homogeneous population
The basic method to predict the expected vaccine coverage level by game theory is coupling game theory and epidemiological models. From the analysis of game theory, an implicit form of the expected vaccine coverage level and the expected attack ratio can be obtained. Through the deterministic epidemic models, the expected attack ratio can be expressed in terms of the vaccine coverage level. Finally, an accurate estimation of vaccine coverage level can be made. We assume that the population is well-mixed/homogeneous and all individuals are provided with the same information and use this information in the same way to assess risks, this is described in [Citation3].
2.1. Vaccination game with perfect vaccine
We formalize the vaccination game with perfect vaccine by setting the elements of the following payoff matrix:
Table
This payoff matrix describes four outcomes of the game: individual i is vaccinated/not vaccinated and interacts with individual j who is vaccinated/not vaccinated. The elements in the payoff matrix are the payoffs to individual i. If individual i is vaccinated, the payoff is the cost of the vaccination . This is true whether this individual is matched with a vaccinated or not vaccinated individual, because the vaccine is fully effective and there is no chance for individual i to get infected. If individual i chooses not to be vaccinated, there are two possibilities. The first possibility is to match with a vaccinated individual. In this case, since individual j is protected by the vaccine and will not be infected, individual i will not be infected either, so the payoff is 0. The second possibility is to match with a not vaccinated individual. We assume for everyone who is susceptible, the probability of being infected is
, and the cost of infection is
. We also assume that if individual j is infected, individual i will be infected through the contact. The costs of vaccination and infection were indicated as negative terms, because they decrease the payoffs. A similar game can be found in [Citation25]. It is noted that if the cost of being vaccinated (
) is larger than the cost of being infected (
), the strategy of not being vaccinated is the dominant strategy. Since to be vaccinated is more risky than being infected.
The second step is to calculate the payoffs of individual i choosing different strategies. Assume that the final fraction of individuals who takes vaccination is p; the fraction of individuals to be vaccinated is and the fraction of individuals not to be vaccinated is
.
is the equilibrium (or evolutionary stable strategy). We have the payoffs of two strategies:
(1)
(1) where
is the payoff for individuals to be vaccinated and
is the payoff for individuals not to be vaccinated.
Then, the replicator equations to this vaccination game can be expressed as
(2)
(2) where
denotes the average payoff. By solving the replicator equations, we find that the equilibrium is
, which implies that the final fraction p of population to be vaccinated is
. If we define the relative cost
(the relative cost reflects the relative risk of being vaccinated and being infected), we can rewrite the expected vaccine coverage level
.
Since is determined by the value of p, we rewrite the relation as
(3)
(3)
2.2. Vaccination game with imperfect vaccine
To construct the vaccination game with imperfect vaccine, we introduce a factor σ, , in the infection rate of vaccinated members.
means that the vaccine is perfectly effective and
means that the vaccine has no effect. We set up the game by setting the entries of the payoff matrix. If individual i is vaccinated and individual j is also vaccinated, then the payoff for individual i is the cost of vaccination plus the risk of being infected, the cost of vaccination is still
; the probability that individual j getting infected is
, since individual i is also vaccinated, the probability of being infected by individual j is reduced by σ, and the cost of infection is
. The other three entries in the payoff matrix can be generated in the same way. It is noted that
is the probability that individual is not vaccinated but infected. The formal partial-effective vaccination game is:
Table
The replicator equations can still be used to describe the dynamics of the game. We have the expression of the fraction p
(4)
(4) where r represents the relative cost
,
is the attack ratio for the susceptible class and
is the attack ratio for the vaccinated class.
2.3. Importance of property of monotonicity
In the vaccination game with perfect vaccine, we have an implicit equation (Equation3(3)
(3) ) of the vaccine coverage level. When the vaccine coverage level p=0, the left side of the equation is
and this value should be larger than r. When the vaccine coverage level
,
is the herd immunity threshold value, the left side of the equation is 0, which is smaller than r. If we differentiate the function in the left side with respect to p, the derivative is
We first assume that if
. In this case,
for all
. Apparently, we have
, which implies
(
is the herd immunity threshold). However, if
, the attack ratio has to be 0. This contradiction gives us
. The same method can show that it is impossible for
. If
is negative, the derivative
is negative, then Equation (Equation3
(3)
(3) ) has a unique solution. Thus, it is important to show that the attack ratio is a decreasing function of the vaccine coverage level p.
In the vaccination game with imperfect vaccine, we have formula (Equation4(4)
(4) ) about the vaccine coverage level p. If the vaccine coverage level p=0, the right side is
, and this value should be larger than
. If the vaccine coverage level
, the right side of the equation is smaller than
. Because the left side of the equation is a constant, if the derivative of the right side is negative, we have one unique solution. We take the derivative of
with respect to p to get
We have
, since the vaccinated individuals are protected by vaccine, the corresponding attack ratio is smaller. If both
and
are negative, then formula (Equation4
(4)
(4) ) has a unique solution. It can be proved that
and
cannot be positive or zero.
Remark 2.1.
is a necessary condition for these two vaccination games to have Nash equilibrium. Otherwise, we have two dominant games. The strategy of not to be vaccinated is dominant, because choosing the strategy of to be vaccinated is always more risky than being infected.
Remark 2.2.
The analysis of these two games implies that the herd immunity cannot be reached if all individuals are purely driven by self-interest. Since the solutions to Equations (Equation3(3)
(3) ) and (Equation4
(4)
(4) ) are absolutely between 0 and the herd immunity threshold
. The vaccine coverage level p we discussed in this paper is lower than
. Once we have
, the attack ratio becomes 0.
We now define the attack ratio in the population under study. This definition will be used through the paper repeatedly.
Definition 2.1.
The attack ratio in the population is defined as
where
is the size of the whole population and
is the number of individuals who are not affected by the epidemic.
3. Mathematical analysis in homogeneous mixing population
Before the outbreak of the epidemic, people can choose to be vaccinated, or not to be vaccinated, based on the estimation of payoffs of such behaviours. We assume
that the population is homogeneous mixing with size N and the expected vaccine coverage level is p, σ is the vaccine efficacy factor, with .
means that the vaccine is perfectly effective and
means that the vaccine has no effect. We also assume that an average member of the population makes contact sufficient to transmit infection with
others per unit time. Infectives leave the infective class at rate α per unit time (see [Citation5,Citation6] for assumptions of simple compartmental models in detail). All individuals remain in the susceptible class or go to the vaccinated class before the outbreak.
3.1. Susceptible-vaccinated-infected-recovered (SVIR) compartmental model
The SVIR model we use to describe such a condition is
(5)
(5) with initial values
,
,
, other parameters are defined as before. Since the population is not completely susceptible, it is accurate to use the terminology control reproduction number instead of basic reproduction number. In this case,
and the final size relation can be expressed by the following equations (a special case of Equation (3) in [Citation9] by Brauer and Watmough):
(6)
(6) It is easy to find out that
(7)
(7) which implies that for every individual, staying in group V is much safer than staying in group S.
3.2. 
, 
, attack ratios and vaccine coverage level
If we change the vaccine coverage level p, how this will affect and
? We take the derivatives of
and
with respect to p to have
(8)
(8) We reorganize Equations (Equation8
(8)
(8) ) to obtasimilar equation systems (by different substitutions)
(9)
(9) and
(10)
(10) It is noted that in Equations (Equation9
(9)
(9) ) and (Equation10
(10)
(10) ),
, so the key to find whether the derivatives are positive or negative is the relation of
,
and
.
We first consider the condition p=0. The SVIR model is simplified to the simple SIR model, the final size relation of such model is
where
. To prove that there is a unique solution of the final size relation, we define
we have
,
, and
if and only if
. The disease can only spread if
. In such a case,
is monotone decreasing from a positive value at
to a minimum at
and then increases to a negative value at
. Thus, there is a unique zero
of
with
, which is
(11)
(11)
Secondly, we consider the case that p=1. In such a case, we have a SVIR model, the final size relation of such model is
where
. If we use the same method before to prove the uniqueness of
, we have
(12)
(12)
Now, we consider the case that 0<p<1. If we have , the second equation in Equation (Equation9
(9)
(9) ) yields
, then from the first equation in Equation (Equation9
(9)
(9) ), this leads to
; the second equation in Equation (Equation10
(10)
(10) ) yields
, finally this assumption leads to
. This is a contradiction to
. So
must hold.
To investigate that how and
will change if p is changed, we still use the final size relation (Equation6
(6)
(6) ). For simplicity, we denote
and
(13)
(13) We take the derivatives of
and
with respect to p to obtain
(14)
(14) Since
, we have
and
. From the fundamental knowledge of calculus, we know that
Now, we know that both
and
are monotone decreasing as p increases from 0 to 1, and for all p, we have
. If when
,
, then for all p, we have
; else if when
,
, as p increases,
will be first smaller than
, then will be bigger than
.
Remark 3.1.
I would like to point out, as the reviewer suggested, it is more intuitive to use the notations and
. We use the notations
and
in the analysis to simplify the calculations, because we assume that the control reproduction number
.
We have two lemmas.
Lemma 3.1.
In the SVIR model (Equation5(5)
(5) ) with infection factor σ, if
, we have
and
.
Proof.
Since the assumption is that the disease can spread among people, the disease-free equilibrium is unstable, we have . And because the endemic equilibrium is asymptotically stable, so
.
For the simple SIR model, can be obtained from the final size relation, we define
as follows:
(15)
(15)
Lemma 3.2.
In the SVIR model (Equation5(5)
(5) ) with infection factor σ, there exists a threshold value
which was defined in Equation (Equation15
(15)
(15) ). And we have that if the infection factor σ is smaller than
holds when the vaccine coverage level is low, and by increasing the vaccine coverage level p,
. If the infection factor σ is bigger than
the inequality
holds.
The analysis establishes the result on and
.
Theorem 3.1.
In the SVIR compartmental model (Equation5(5)
(5) ), the infection factor σ will affect
and
. There are two conditions. If σ is smaller than
there exists a critical value
. As vaccine coverage level p increases from 0 to
,
will decrease and
will still increase; while p increases from
to 1,
and
both increase, where
is the critical value we described in lemma (3.2). If σ is bigger than
when the vaccine coverage level p increases,
will decrease and
will increase.
Based on the definition of attack ratio we introduced before, the attack ratios and
for susceptible class and vaccinated class have the following expressions:
since
and
, we know that
and
. So we reach the result on the change of attack ratios,
Theorem 3.2.
In the SVIR compartmental model, if the vaccine coverage level p increases between 0 and the herd immunity threshold, for vaccinated group, for non-vaccinated group and for the whole population, the attack ratios decrease. The infection factor σ and the attack ratios are independent.
Remark 3.2.
If we consider the vaccine coverage level p in the interval the attack ratio is a piecewise function. The function has maximum at
then decreases as p increasing from 0 to the herd immunity threshold
. If
the attack ratio is 0.
3.3. Case of perfect vaccine
In some game-epidemiology papers, people only consider the case that the vaccine is perfect, which is . In this special case, we only need to analyse the relation between attack ratio for susceptible class and the vaccine coverage level, since the vaccinated group is fully protected. We use the simple SIR model
(16)
(16) with initial values
and
.
The final size relation of Equation (Equation16(16)
(16) ) is
(17)
(17) we take the derivative of
with respect to p to have
(18)
(18)
We have the following results.
Corollary 3.1.
In the SIR compartmental model, when vaccine coverage level p increases from 0 to the herd immunity threshold the final size
of susceptible class will increase, the attack ratio will decrease. If
the whole population is protected completely by the vaccine.
4. Mathematical analysis in heterogeneous mixing population
It is difficult to prove or disprove the uniqueness of Nash equilibrium in vaccination games in heterogeneous mixing population. The analysis for uniqueness consists of the properties of functions of attack ratios in subgroups and the pattern of mixing in the population. We obtain partial results on attack ratios in this section.
We consider the population is divided into subgroups with different activity levels. We will analyse how and
in two subgroups be changed if we increase or decrease the vaccine coverage level of each subgroup. We assume that the sizes of population in the two subgroups are
and
. Group i members make
contacts in unit time and that the fraction of contacts made by a member of group i that is with a member of group j is
, with i,j=1,2 (see [Citation8]).
4.1. SVIR compartmental model
The two-group SVIR vaccination model is
(19)
(19) The mean infective period in group i is
(i=1,2). The rate of infection in group i is
(i=1,2) and the vaccine coverage levels in each subgroup are
and
. The initial condition to this epidemic model is
,
,
,
,
and
.
We use the next-generation matrix approach, which is described in [Citation26], to find the control reproduction number . The final size relation of Equation (Equation19
(19)
(19) ) is
(20)
(20) This final size relation (Equation20
(20)
(20) ) is also a special case of Equation
in [Citation9] by Brauer and Watmough.
Two equations are revealed from Equation (Equation20(20)
(20) )
(21)
(21)
4.2. 
, 
, attack ratios and vaccine coverage level
For simplicity of further calculation, we denote
We take the derivatives of
,
,
and
with respect to
, to find out if we only adjust vaccine coverage level in one subgroup, how this will affect two subgroups.
(22)
(22) with
From Equation (Equation22
(22)
(22) ), we have
(23)
(23) The above relation implies that
and
are both positive or negative, which indicates the change of vaccine coverage level in subgroup 1 having the same impact to classes
and
in subgroup 2.
We state the following lemma, and use the lemma to do further analysis.
Lemma 4.1.
In the SVIR heterogeneous model (Equation19(19)
(19) ), if the epidemic can spread among the population initially, we have
(24)
(24) and
(25)
(25)
and
are defined before.
Proof.
We first linearize the system at the disease-free equilibrium to obtain
This equilibrium is unstable, the
matrix has two positive eigenvalues because the disease can spread in two subgroups.
which lead to
and
We then linearize the system at the endemic equilibrium to obtain
The endemic equilibrium is asymptotically stable, so this
matrix has two negative eigenvalues
which lead to
and
We now substitute expressions in Equation (Equation23(23)
(23) ) into the first and the third equation in Equation (Equation22
(22)
(22) )
(26)
(26) We extract the expression of
(27)
(27) Based on Equation (Equation21
(21)
(21) ), we know that the right-hand side of Equation (Equation27
(27)
(27) ) is positive. And from the second part of the proof of previous lemma, we know that the left-hand side of Equation (Equation27
(27)
(27) ) is also positive, which gives that
(28)
(28) The above analysis implies that if the vaccine coverage level in subgroup 1 is increased, for subgroup 2, both
and
will increase. We now investigate how
and
will be affected, and how the change will relate to the vaccine efficacy. Through some simple calculations, we have the left-hand side of the expression of
be
(29)
(29) The right-hand side of the expression of
is
(30)
(30) By observing the left-hand sides of Equations (Equation27
(27)
(27) ) and (Equation29
(29)
(29) ), they have the same form, so we only need to analyse the positive or negative of
Equation (Equation30
(30)
(30) ), to rewrite Equation (Equation30
(30)
(30) ) as
(31)
(31) If
, then
since the vaccine has no effect. In such a case, we have
(32)
(32) Due to continuity, we have that when the vaccine is low-effective,
is close to 1, then if the vaccine coverage level in subgroup 1 is increased,
will decrease. If we substitute Equation (Equation32
(32)
(32) ) into the first equation of Equation (Equation23
(23)
(23) ), we have
This is the case that
, which vaccine has the lowest efficacy. If the vaccine efficacy is upgraded, people get better protection, so
holds for all
.
If , which is the case that the vaccine is perfect effective, then
(33)
(33) If
is also close to 0, then Equation (Equation33
(33)
(33) )
From the previous lemma, we know that
We establish the conclusion.
Theorem 4.1.
In the heterogeneous SVIR model with imperfect vaccine, if we keep the vaccine coverage of subgroup 2 in a steady level, and only change the vaccine coverage level of subgroup 1. Then, we have that when the vaccine has low effectivity to subgroup 1, if vaccine coverage level of subgroup 1 increases, will decrease, and
will increase; both
and
increase. When the vaccine has high effectivity to subgroup 1, if vaccine coverage level of subgroup 1 increases,
and
will increase.
Remark 4.1.
We notice that in Equation (Equation30(30)
(30) ), if
(34)
(34) there is the change of dynamic behaviour of
, we call Equation (Equation34
(34)
(34) ) the critical-relation equation. We know that both
and
are positive. If
,
may be negative. In such a case, the critical-relation equation does not exit, which means
that the relation between
and
does not affect the change of
, only the value of
matters. This conclusion is reasonable, because if
holds, we have
and
much bigger than
and
, that means two subgroups have few contacts with each other. To the extent, we have two separate homogeneous mixing population. If
is close to 0, or
, the critical-relation equation may exist, that means two different subgroups have frequent contact, then the relation of
and
will affect the results.
For attack ratios, deriving from the final size relation, we have the conclusion.
Theorem 4.2.
In the heterogeneous SVIR model, if the vaccine coverage level in subgroup 1 increases, all attack ratios decrease; for the increase of vaccine coverage level in subgroup 2, the results hold. The infection rates and
are unrelated to the change of attack ratios.
Remark 4.2.
Herd immunity threshold is not considered in the analysis. In heterogeneous mixing population, the herd immunity threshold will be a relation which the vaccine coverage levels must satisfy, instead of a value.
4.3. Case of perfect vaccine
For the special case of perfect vaccine, we hold all other assumptions. By employing the same technique, we obtain the final size relations
(35)
(35) with
and
representing the vaccine level coverage in subgroup 1 and subgroup 2, respectively. By analysing the final size relations, we have similar results.
Corollary 4.1.
In the heterogeneous SIR model, if the vaccine coverage level in any subgroup increases, in both subgroups increase.
Corollary 4.2.
In the heterogeneous SIR model, if the vaccine coverage level in any subgroup increases, the attack ratios in every subgroup also decrease.
5. Extension in general compartmental models
For general compartmental models which may include Exposed class, Quarantine class, Treatment class, etc., we use the general age-of-infection models. By proving the similarity of final size relations obtained from the age-of infection models and from the SIR or SVIR models in homogeneous mixing population and heterogeneous mixing population, we can extend our results in previous sections to the general compartmental models. The same as in [Citation9], we consider a population with a distribution of subpopulation identified by the variable ζ ranging over a ‘state’ space Ω, having sizes , respectively, at time t. Suppose that each group ζ member makes
contacts sufficient to transmit infection per unit time. We assume that the fraction of contacts made by a member of group ζ with a member of group η is
, then:
for each ζ. The total number of contacts made in unit time by member of group ζ with members of group η is
and by balance relation we have
with
.
We assume that there is no permanent movement between groups and that there are no disease deaths, so that is a constant function
of t for all ζ. We consider two cases, which are perfect vaccine and imperfect vaccine.
A multi-group age of infection model with general mixing would be
(36)
(36) The initial conditions are
and the vaccine is perfectly effective.
It is possible to obtain a system of final size relations, which is
(37)
(37)
We now investigate the case which the vaccine are imperfect, and we model this by including factors
, where
. The multi-group age of infection model with general mixing
(38)
(38)
The final size relations of the model (Equation38
(38)
(38) ) are
(39)
(39)
If we compare the final size relations (Equation37(37)
(37) ) and (Equation39
(39)
(39) ) with (Equation35
(35)
(35) ) and (Equation20
(20)
(20) ), it is clear that the forms of final size relations are similar. The final size relation to the homogeneous mixing age-of-infection model is straightforward to obtain. The generality of the final size relation formulas guarantees that we can easily extend our results from previous sections to the general compartmental models. More details of similar work can be found in [Citation17]. As an example, in [Citation5], it has been proved that the standard susceptible-exposed-infected-recovered (SEIR) epidemic model can be written in the form of the age-of-infection model. So, the property of attack ratios can be applied to the SEIR model. Furthermore, it can be argued that the vaccination game of epidemic with exposed stage has a unique Nash equilibrium. By a similar technique, vaccination games of more complicated epidemics have unique Nash equilibria as well.
6. Conclusion and future work
We demonstrated two vaccination games in homogeneous mixing population and find the Nash equilibrium in each game. To prove that the Nash equilibrium in each game is unique, it is important to consider the monotonicity of the attack ratio. We have formulated the deterministic compartmental vaccination models, in homogeneous mixing population and in heterogeneous population. The final size relations can be obtained from the models. By analysing the final size relations, we proved that the attack ratios can be expressed in terms of the vaccine coverage levels, and also proved that the attack ratios are decreasing functions of the vaccine coverage levels. In other words, we have provided the mathematical proof that the vaccine can protect both susceptible population and vaccinated population better, this is the so-called direct and indirect protection stemming from vaccine. The probability of every individual to survive through the epidemic is greater by increasing the vaccine coverage level. We propose some possible extensions:
(A1) Compare the measures of derivatives to conduct the sensitivity analysis. If one derivative is bigger than the other, from the standpoint of public health management, to vaccinate one certain subgroup is the priority, since the same amount of vaccination can lower the attack ratio more.
(A2) The analysis in Section 4 only derives analytical results for the vaccine efficacy factor which is close to 1 and close to 0. Because of too many parameters in the model, there are no explicit formulas to be given. By other methods, we may obtain more accurate results, one possible way is to consider proportional mixing or preferred mixing, instead of general mixing.
(A3) The vaccination game in heterogeneous mixing population is much more complicated to analyse. The uniqueness of Nash equilibrium does not only depend on the monotonicity of attack ratios, but also depend on the mixing patterns of the population. This part of work needs more effort.
(A4) We prove that in heterogeneous mixing population, if two subgroups are mainly separate, the vaccine efficacy for subgroup 1 does not affect subgroup 2, vice versa.
Acknowledgments
This work was first inspired by Professor Fred Brauer. The author thanks Fred for weekly discussions on this subject. The author also thanks one anonymous reviewer for helpful comments. This research was supported by NSERC grant.
Disclosure statement
No potential conflict of interest was reported by the authors.
References
- J. Arino, F. Brauer, P. van den Driessche, J. Watmough, and J. Wu, A final size relation for epidemic models, Math. Biosci. Eng. 4 (2007), pp. 159–175. doi: 10.3934/mbe.2007.4.159
- J. Arino, F. Brauer, P. van den Driessche, J. Watmough, and J. Wu, A model for influenza with vaccination and antiviral treatment, J. Theor. Biol. 253 (2008), pp. 118–130. doi: 10.1016/j.jtbi.2008.02.026
- C.T. Bauch and D.J.D. Earn, Vaccination and the theory of games, Proc. Natl Acad. Sci. USA 101 (2004), pp. 13391–13394. doi: 10.1073/pnas.0403823101
- C.T. Bauch, A.P. Galvani, and D.J.D. Earn, Group interest versus self-interest in smallpox vaccination policy, Proc. Natl Acad. Sci. USA 100 (2003), pp. 10564–10567. doi: 10.1073/pnas.1731324100
- F. Brauer, The KermackMcKendrick epidemic model revisited, Math. Biosci. Eng. 198 (2005), pp. 119–131. doi: 10.1016/j.mbs.2005.07.006
- F. Brauer, Some simple epidemic models, Math. Biosci. Eng. 3 (2006), pp. 1–15. doi: 10.3934/mbe.2006.3.1
- F. Brauer, Age-of-infection and the final size relation, Math. Biosci. Eng. 5 (2008), pp. 681–690. doi: 10.3934/mbe.2008.5.681
- F. Brauer, Epidemic Models with Heterogeneous Mixing and Treatment, Bull. Math. Biol. 70 (2008), pp. 1869–1885. doi: 10.1007/s11538-008-9326-1
- F. Brauer and J. Watmough, Age of infection epidemic models with heterogeneous mixing, J. Biol. Dyn. 3 (2008), pp. 324–330. doi: 10.1080/17513750802415822
- D.L. Brito, E. Sheshinski, and M.D. Intriligator, Externalities and compulsory vaccinations, J. Publ. Econ. 45 (1991), pp. 69–91. doi: 10.1016/0047-2727(91)90048-7
- F.H. Chen, A susceptible-infected epidemic model with voluntary vaccination, J. Math. Biol. 53 (2006), pp. 253–272. doi: 10.1007/s00285-006-0006-1
- W. Chen, S. Landau, P. Sham, and E. Fombonne, No evidence for links between autism, MMR and measles virus, Psychol. Med. 34 (2004), pp. 543–553. doi: 10.1017/S0033291703001259
- D.W. Cornforth, T.C. Reluga, E. Shim, C.T. Bauch, A.P. Galvani, and L.A. Meyers, Erratic flu vaccination emerges from short-sighted behavior in contact networks, PLoS Comput. Biol. 7 (2011), pp. 1–10. doi: 10.1371/journal.pcbi.1001062
- P.E.M. Fine and J.A. Clarkson, Individual versus public priorities in the determination of optimal vaccination policies, Am. J. Epidemiol. 124 (1986), pp. 1012–1020.
- A.P. Galvani, T.C. Regula, and G.B. Chapman, Long-standing influenza vaccination policy is in accord with individual self-interest but not with the utilitarian optimum, Proc. Natl Acad. Sci. USA 104 (2007), pp. 5692–5697. doi: 10.1073/pnas.0606774104
- J. Liu, B.F. Kochin, Y.I. Tekle, and A.P. Galvani, Epidemiological game-theory dynamics of chickenpox vaccination in the USA and Israel, J. R. Soc. Interface 9 (2012), pp. 68–76. doi: 10.1098/rsif.2011.0001
- J. Ma and D.J.D. Earn, Generality of the Final Size Formula for an Epidemic of a Newly Invading Infectious Disease, Bull. Math. Biol. 68 (2006), pp. 679–702. doi: 10.1007/s11538-005-9047-7
- T.C. Reluga, An SIS epidemiology game with two subpopulations, J. Biol. Dyn. 3 (2008), pp. 515–531. doi: 10.1080/17513750802638399
- T.C. Reluga, C.T. Bauch, and A.P. Galvani, Evolving public perceptions and stability in vaccine uptake, Math. Biosci. 204(2) (2006), pp. 185–198. doi: 10.1016/j.mbs.2006.08.015
- E. Shim, G. Chapman, and A. Galvani, Decision making with regard to antiviral intervention during an influenza pandemic, Med. Decision Making 30 (2010), pp. 65–82. doi: 10.1177/0272989X10378699
- E. Shim, G.B. Chapman, J.P. Townsend, and A.P. Galvani, The influence of altruism on influenza on influenza vaccination decisions, J. R. Soc. Interface 0 (2012), pp. 1–10.
- E. Shim, J.J. Grefenstette, S.M. Albert, B.E. Cakouros, and D.S. Burke, A game dynamic model for vaccine skeptics and vaccine believers: Measles as an example, J. Theor. Biol. 295 (2012), pp. 194–203. doi: 10.1016/j.jtbi.2011.11.005
- E. Shim, B. Kochin, and A.P. Galvani, Insights from epidemiological game theory into gender-specific vaccination against rubella, Math. Biosci. Eng. 6 (2009), pp. 841–856. doi: 10.3934/mbe.2009.6.839
- E. Shim, L. Meyers, and A.P. Galvani, Optimal H1N1 vaccination strategies based on self-interest versus group interest, BMC Publ. Health 11(Suppl. 1) (2011).
- T. Tassier, The Economics of Epidemiology, Springer-Verlag, Berlin, 2013.
- P. van den Driessche and J. Watmough, Reproduction numbers and subthreshold endemic equilibria for compartmental models of disease transmission, Math. Biosci. 180 (2002), pp. 29–48. doi: 10.1016/S0025-5564(02)00108-6