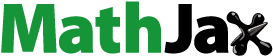
ABSTRACT
Early in a disease outbreak, it is important to be able to estimate the final size of the epidemic in order to assess needs for treatment and to be able to compare the effects of different treatment approaches. However, it is common for epidemics, especially of diseases considered dangerous, to grow much more slowly than expected. We suggest that by assuming behavioural changes in the face of an epidemic and heterogeneity of mixing in the population it is possible to obtain reasonable early estimates.
KEYWORDS:
AMS 2010 SUBJECT CLASSIFICATION:
1. Introduction
It has been standard practice in analysing disease outbreaks to formulate a dynamical system as a deterministic compartmental model, then to use observed early outbreak data to fit parameters to the model, and finally to analyse the dynamical system to predict the course of the disease outbreak and to compare the effects of different management strategies. In general, such models predict an initial stochastic stage (while the number of infectious individuals is small), followed by a period of exponential growth. Measurement of this early exponential growth rate is an essential step in estimating contact rate parameters for the model.
However, instances have been noted where the growth rate of an epidemic is clearly slower than what this approach predicts. One of the earliest examples [Citation4] concerns the growth of HIV/AIDS in the United States, and a possible explanation might be the mixture of short-term and long-term contacts. This could be a factor in other diseases where there are repeated contacts in family groups and less frequent contacts outside the home. Some suggested explanations for slower than expected growth include metapopulation models with spatial structure including cross-coupling and mobility, clustering in spatial structure, dynamic contacts, agent-based models with differences in infectivity and susceptibility of individuals, and reactive behavioural changes early in a disease outbreak.
In Liberia, a country with a population of close to 4,000,000, the 2014 epidemic of the Ebola virus produced fewer than 12,000 cases although estimates of the reproduction number from early data would have predicted more than 1,5000,000 cases. Such large discrepancies led to growth models that are not mechanistic but are very useful for fitting data and making short-term predictions. One example is a discrete model [Citation5] that has been applied to Ebola data in [Citation6]. Another approach has been to formulate a so-called general growth model of the form
where
is the number of disease cases occurring up to time t, and p,
, is a ‘deceleration of growth’ parameter [Citation3,Citation12]. These models have been very successful for fitting epidemic growth, but they are not useful for predicting the final size of an epidemic. The parameter p represents the effect of behavioural responses during the initial growth phase of the epidemic but since without some additional term to model the decreasing phase of the epidemic it cannot be incorporated into a final size relation.
An approach that may be more helpful in estimating the final size of an epidemic from early data is the suggestion of [Citation2] that behavioural change may decrease the basic reproduction number in a severe epidemic.
It has been observed that in many disease outbreaks, the phenomenon of superspreading events, situations in which a small fraction of the population causes more than its share of disease cases, has been significant [Citation9]. For example, superspreading events were important in the SARS outbreak of 2002–2003 [Citation10] in which there was a cluster of at least 125 cases apparently infected by a single index patient as well as another cluster of perhaps 300 cases. For a given basic reproduction number, the number of disease cases is generally fewer if there is superspreading than if the population mixing is homogeneous.
In the 2014–2015 West Africa Ebola epidemic, there is also evidence of superspreading [Citation8]. It is suggested in [Citation7] that commonly roughly of the individuals in a population are responsible for
of the disease cases.
In this note, we suggest that these two effects may give useful early estimates of the final size of an epidemic.
2. Behavioural changes during an epidemic
It is natural to expect that when a disease outbreak begins there may be behavioural response to attempt to protect against infection. This is particularly evident if the disease is considered to be very dangerous, as has been the case in Ebola virus outbreaks, and much less evident with diseases not considered to be dangerous, such as seasonal influenza outbreaks. A model described in [Citation2] for the Ebola virus outbreak of 2014 in West Africa used data from the first 40 weeks of the outbreak to estimate disease model parameters and then assumed a response leading to a decrease by a factor of 0.6 in the reproduction number. This assumption led to a very good fit of the model to continuing disease data and the ultimate final size for the epidemic.
We suggest that assuming a simple model of SIR or SEIR type for an emerging disease outbreak, even though it may fail to capture many of the properties of the disease, together with an assumption of a decrease in the reproduction number after an initial stage, may give a good start on obtaining a reasonable estimate of the epidemic final size. For simplicity, we will assume that the effect of the measures causing this reduction is immediate, even though it would be more realistic to assume a reduction carried out over a given time interval. Clearly, we would expect the reduction factor in the reproduction number and the time of the reduction to be sensitive parameters.
Example 2.1
The 2014 Ebola virus in Liberia has been modelled by a system that includes some specific aspects of the Ebola virus [Citation1,Citation2]. At the beginning of an outbreak of an emerging or re-emerging disease, the specific aspects of the disease may not yet be well understood. In order to make an early estimate of the final size of the outbreak of a disease serious enough that there may be substantial behavioural change in response, we suggest using a generic SEIR model incorporating such response to obtain an initial estimate. We use an SEIR model for the Ebola outbreak in Liberia
(1)
(1) with parameter values [Citation2]
and [Citation1]
giving estimates consistent with those of [Citation2].
Numerical simulations give , indicating 35,345 cases in the first 40 weeks of the outbreak. It is estimated in [Citation2] that after 40 weeks the overall contact rate had dropped to 60 % of its original value. Then, multiplying
by a factor 0.6 and using
as an initial value, we use the final size relation for an SEIR model to estimate
. This final size relation now takes the form
because there are removed members of the population at time t=280. Here,
. We obtain a final size of
, giving a total number of disease cases over the course of the epidemic as 92,228. This is comparable to but considerably larger than the reported number of 10,678 disease cases in Liberia.
3. Heterogeneity in epidemic models
In order to describe a simple superspreading epidemic model, we consider an SEIR epidemic model in a population divided into two groups. Group 1 members (superspreaders), forming a fraction ρ of the total population, have a contact rate and Group 2 members have a contact rate a, with
. Mixing between groups is proportionate. We take
in order to conform to the suggestion in [Citation7] that commonly roughly
of the individuals in a population are responsible for
of the disease cases.
We assume
Total population size is N.
Group 1 population size is
.
Group 2 population size is
.
Group i is divided into susceptibles (
), exposed members (
), infectives (
) and recovered members (
).
Mixing between groups is proportionate. Fraction of contacts of susceptibles with groups 1 and 2 respectively are
Infections in each group recover at rate α.
There are no demographic effects (births, deaths, migration) on the population.
Then
with
Similarly,
Thus the full model is
(2)
(2) The parameter values corresponding to the SEIR model (Equation1
(1)
(1) ) are
(3)
(3) To calculate the reproduction number using the next generation method [Citation11], we write
Thus the next generation matrix is
This matrix has the same non-zero eigenvalues as the
matrix
(the next generation matrix with least domain). The matrix
has determinant zero. Thus the non-zero eigenvalue is the trace of this matrix, and
From the model (Equation2
(2)
(2) ), we see that
from which we deduce that
, and
. Integration gives
Similarly, we see that
from which we deduce that
, and
.
Integration gives
and
Integration of the equation for
in the model gives
We combine the expressions
and
to give
Since
, we may carry out analogous calculations to give
with the matrix K given by
We note that K is the transpose of the next generation matrix with least domain
. We now have the final size relation
(4)
(4) We note that
Because
, we have
which implies
(5)
(5) We could substitute (Equation5
(5)
(5) ) into the final size relation (Equation4
(4)
(4) ) to obtain a single final size equation.
We simulate the superspreader model (Equation2(2)
(2) ) for
, using the parameter values and initial conditions (Equation3
(3)
(3) ), obtaining
The number of disease cases for
is
Next, we use the final size relations (Equation4
(4)
(4) ) using the compartment sizes for t=280 as initial values. This yields
The number of new disease cases after t=280 is 17,718+4531=22,249 , and the total number of cases is 13,215+22,249=35,464. This is still larger than the actual number of observed cases, but considerably closer to the actual number of observed cases than the estimate provided by (Equation1
(1)
(1) ).
The factor σ in the model (Equation2(2)
(2) ) represents the degree of superspreading. To indicate the extent to which the epidemic final size would depend on the value of the parameter σ, we used the final size relation (Equation4
(4)
(4) ) for the model (without incorporating behavioural response).
With , so that 20% of the population is responsible for 80% of the disease cases, we obtain
so that there are 567,490 disease cases overall.
With , so that 20% of the population is responsible for two-thirds of the disease cases, we obtain
so that there are 739,118 disease cases overall.
With , so that 20% of the population is responsible for half of the disease cases, we obtain
so that there are 1,124,251 disease cases overall.
This suggests that superspreading is significant in decreasing the number of disease cases corresponding to a given value of the basic reproduction number.
4. Conclusion
It is useful to have some idea early in a disease outbreak how serious the outbreak will turn out to be. If the disease is viewed as a serious threat, there will surely be substantial behavioural changes as the disease develops. The standard approaches to estimating a disease outbreak using initial exponential growth rates may give predictions which are so large as to be meaningless. We suggest that it may be possible to use relatively simple SIR or SEIR models without detailed assumptions about the particular disease being studied but assuming a decrease in the contact rate and heterogeneity of mixing to obtain more plausible estimates. While a decrease in the contact rate would probably be continuous in reality, we approximate it by a discontinuous decrease at a fixed time. Of course, the results will depend strongly on the size of the assumed decrease and the time at which it is assumed to take place.
Disclosure statement
No potential conflict of interest was reported by the author.
Additional information
Funding
References
- C.L. Allthaus, Estimating the reproduction number of Ebola virus (EBOV) during the 2014 outbreak in West Africa, PLoS Curr. Outbreaks (2014), Edition 1. doi:10.1371/currents.outbreaks.91afb5e0f279e7f29e7056095255b288.
- M.V. Barbarossa, A. Denes, G. Kiss, Y. Nakata, G. Rost, and Z. Vizi, Transmission dynamics and final epidemic size of Ebola virus disease outbreaks with varying interventions, PLoS ONE 10 (2015), p. e0131398. doi: 10.1371/journal.pone.0131398.
- G. Chowell, C. Viboud, J.M. Hyman, and L. Simonsen, The western Africa Ebola virus disease epidemic exhibits both global exponential and local polynomial growth rates, PLOS Curr. 7 (2015), pp. 1–27, Jan. 21 Edition 1. doi:10.1371/currents.outbreaks.8b55f4bad99ac5c5db3663e916803261.
- S.A. Colgate, E.A. Stanley, J.M. Hyman, S.P. Layne, and C. Qualls, Risk-behavior based model of the cubic growth of acquired immunodeficiency syndrome in the United States, Proc. Natl. Acad. Sci. 86 (1989), pp. 4793–4797. doi: 10.1073/pnas.86.12.4793
- D.N. Fisman, T.S. Hauck, A.R. Tuite, and A.L. Greer, An IDEA for short term outbreak projection: near casting using the basic reproduction number, PLOS One 8 (2013), pp. 1–8. doi: 10.1371/journal.pone.0083622
- D.N. Fisman, E. Khoo, and A.R. Tuite, Early epidemic dynamics of the west Africa 2014 Ebola outbreak: estimates derived with a simple two-parameter model, PLOS Curr. 6 (2014), pp. 1–20, September 8. doi: 10.1371/currents.outbreaks.89c0d3783f36958d96ebbae97348d571.
- A.P. Galvani and R.M. May, Dimensions of superspreading, Nature 438 (2005), pp. 293–295. doi: 10.1038/438293a
- M.S.Y. Lau, B.D. Dalziel, S. Funk, A. McClelland, A. Tiffany, S. Riley, C.J.E. Metcalf, and B.T. Grenfell, Spatial and temporal dynamics of superspreading events in the 2014–2015 West Africa Ebola epidemic, Proc. Nat. Acad. Sci. 114 (2017), pp. 2337–2342. doi: 10.1073/pnas.1614595114
- J.O. Lloyd-Smith, S.J. Schreiber, P.E. Kopp, and W.M. Getz, Superspreading and the effect of individual variation on disease emergence, Nature 438 (2005), pp. 355–359. doi: 10.1038/nature04153
- S. Riley, C. Fraser, C.A. Donnelly, A.C. Ghani, J.A. Laith, A. Hedley, G.M. Leung, L.-M. Ho, T.-H. lam, T.Q. Thach, P. Chau, K.-P. Chan, S.-V. Lo, P.-Y. Leung, T. Tsang, W. Ho, K.-H. Lee, E.M.C. Lau, N.M. Freguson, and R.M. Anderson, Transmission dynamics of the etiological agent of SARS in Hong Kong: impact of public health interventions, Science 300 (2003), pp. 1961– 1966. doi: 10.1126/science.1086478
- P. van den Driessche and J. Watmough, Reproduction numbers and sub-threshold endemic equilibria for compartmental models of disease transmission, Math. Biosci. 180 (2002), pp. 29–48. doi: 10.1016/S0025-5564(02)00108-6
- C. Viboud, L. Simonsen, and G. Chowell, A generalized-growth model to characterize the early ascending phase of infectious disease outbreaks, Epidemics 15 (2016), pp. 27–37. doi: 10.1016/j.epidem.2016.01.002