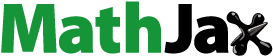
Abstract
In this paper, we use a mean-reverting Ornstein-Uhlenbeck process to simulate the stochastic perturbations in the environment, and then a modified Leslie–Gower Holling-type II predator-prey stochastic model in a polluted environment with interspecific competition and pulse toxicant input is proposed. Through constructing V-function and applying formula, the sharp sufficient conditions including strongly persistent in the mean, persistent in the mean and extinction are established. In addition, the theoretical results are verified by numerical simulation.
1. Introduction
In recent years, with the continuous development of industry, agriculture and fisheries as well as the discharge of waste water, waste gas and wastes, the ecological environment has been seriously damaged. Today's society emphasizes the importance of protecting the environment because these toxins in the polluted environment pose a serious threat to the survival of species such as plankton, animal populations and even human beings themselves. All this has led more scholars to investigate the effects of toxins on populations in polluted environments. For example, Nelson [Citation34] studied the early oil pollution of European seas, Jensen and Marshall [Citation16] described laboratory populations of Daphnia pulex exposed to chronic radiation stress, and Shukla et al. [Citation37] proposed a dynamical model to assess the effects of industrialization on forest biomass degradation in a particular area.
At the same time, many researchers have studied the effects of toxic substances and pollutants on ecological populations from a realistic perspective. In particular, Hallam and his colleagues [Citation9–11] have opened the door to the study of environmental toxins by publishing three papers in a row that explored the effects of toxins on deterministic models of ecosystems. From then on, many deterministic single or multiple population models with toxic effects have been proposed and analysed (see e.g. [Citation5,Citation7,Citation12,Citation28,Citation32,Citation36]), however the underlying assumption is that exogenous inputs of toxins are continuous. Particularly, in real life, toxins are often released into the environment in pulses. A typical example is the regular spraying of pesticides in agriculture and forestry [Citation21], and another example is the massive discharge of heavy metals in industry [Citation20]. Therefore, on the basis of a deterministic population model, some authors have investigated the effects of toxins with pulsed inputs on the population in polluted environments(see e.g. [Citation22,Citation26,Citation31,Citation42]).
Those important and useful studies of deterministic models provide a great way to understand the effects of pollution, but in the real world, populations do not exist in isolation. Therefore, the study of the persistence and extinction of two or more interacting populations under the action of toxic substances has more important biological significance. In recent years, several scholars have proposed more realistic predation models which should take the functional response into account [Citation4,Citation38]. Holling type II functional response is used to measure the average feeding rate of a predator when it spends time looking for and handing the prey, which has served as the basis of many predator-prey theories. As a result, Aziz-Alaoui and Okiye [Citation1] proposed a famous predator-prey model incorporating a modified version of the Leslie–Gower functional response and a Holling-type II one, the model can be shown as follows:
where
and
stand for the size of the prey population and the predator population respectively;
and
denote the intrinsic growth rate of the prey and the predator respectively. a is the internal competition coefficient of the prey; c represents the per capita reduction rate of the prey; h indicates the degree of environmental protection to predator
, that is to say, it represents the capacity of food provision of the environment except prey
; f is the per capita reduction rate of the predator. A growing number of scholars based on the above model have studied the possibility of a reciprocal relationship between the decline of predator populations and the per capita availability of prey(see e.g. [Citation17,Citation18,Citation29,Citation35,Citation39,Citation41]).
Note that the above model is based on a deterministic model independent of random environment fluctuations. In fact, the growth of populations is inevitably affected by environmental noise. For example, May [Citation33] pointed out that the growth rate in population system should be stochastic and the solution of system fluctuated randomly around some mean value. On this basis, many studies focussed on random population models in polluted environment and some good results were obtained(see e.g. [Citation13,Citation24,Citation25,Citation30,Citation40,Citation43,Citation44]). In particular, Liu et al. [Citation27] proposed the following stochastic predator-prey model in a polluted environment with pulse toxicant input
(1)
(1) where
and
denote the concentration of the toxicant in the organism of the prey species, the predator species at time t, respectively;
;
stands for the organisms net uptake rate of toxicant from the environment;
denotes the loss rate of the toxicant in the organism due to the processes such as egestion;
denotes the concentrations of the toxicant in the environment at time t; g is the loss rate of toxicant from environment; q is the toxicant input amount at every time;
stands for the period of the impulsive input of toxicant.
, i = 1, 2 is the intensity of white noise;
and
are mutually independent standard Brownian motions defined on a complete probability space
with a filtration
.
Obviously, there is a limitation in model (Equation1(1)
(1) ), that is, in model (Equation1
(1)
(1) ) there exists an assumption that the growth rate
is linear with respect to the Gaussian white noise
Integrating on the interval
results in
Hence, the variance of the average per capita growth rate
over an interval of length T tends to ∞ as
. It may not be enough to describe the real situation. In recent years, some authors [Citation6,Citation45] have claimed that using the mean-reverting Ornstein-Uhlenbeck process is a more appropriate approach to incorporate the environment perturbations, and due to this approach, one has
i.e.
where
,
,
represents the speed of reversion,
is the intensity of stochastic perturbations. Based on the ideas above, we can derive a new version as follows:
(2)
(2) In the real ecological environment, the predator-prey relationship is a complex biological relationship. Therefore, Gao et al. [Citation8] studied the two-predator one-prey model with pulsed toxin input based on model (Equation2
(2)
(2) ). In the model in paper [Citation8], there is no competition between the two predators. In this paper, we study an impulsive stochastic model with one prey and two predators where the two predators have a competitive relationship. The model is as follows:
(3)
(3) where
denotes the size of the prey population;
and
denote the size of the predators population;
denotes the growth rate of population
;
and
mean the per capita reduction rate of the prey due to the capture by
and
respectively;
and
have similar meanings to
and
respectively;
and
represent the degree of environmental protection to predators
and
, respectively.
Remark 1.1
In model (Equation3(3)
(3) ), the parameters
,
and a satisfy
. The parameter
is the intensity of the white noise on the growth rate of species i, thus
. The parameter
.
represents the decreasing rate of the intrinsic growth rate associated with the uptake of the toxicant. Thus
.
denote the inter-specific competition coefficients of two predators. Thus
. In addition,
and
stand for the concentrations of the toxicant, therefore
and
for
. As a result, the following conditions need to be met:
,
, i = 1, 2, 3.
As far as we know, a very little amount of work has been done about the stochastic three species predation model with toxicants effect, and little is known of the survival impact of competing predators in a polluted environment. In essence, the proof methods and techniques for having two predator species are similar to those for having more predator species. Motivated by these, we deeply analyse the properties of model (Equation3(3)
(3) ).
The arrangement of this paper is as follows. In Section 2, the threshold of persistence and extinction for each species is obtained. In Section 3, we carry out some numerical simulations to verify the theoretical results. Finally, we give some conclusions in Section 4.
2. Main results
For the sake of convenience and simplicity, we define the following notations:
Lemma 2.1
[Citation26,Citation42]
Consider the following subsystem of (Equation3(3)
(3) ):
(4)
(4)
The model has a unique positive γ-periodic solution which satisfies
Notice that in model (Equation4
(4)
(4) ),
and
can be explicitly solved. Then we only need to consider the first three equations in model (Equation3
(3)
(3) ), which is expressed as follows
(5)
(5)
Lemma 2.2
For arbitrary, model (Equation5
(5)
(5) ) possesses a unique positive solution
for all
a.s.(almost surely).
Proof.
Pay attention to the following system:
(6)
(6) and
,
,
. Due to the fact that the coefficients of model (Equation6
(6)
(6) ) satisfy the local Lipschitz condition, model (Equation6
(6)
(6) ) possesses a unique local solution
on
, where
means the explosion time. From the
formula, we derive that
is the unique local positive solution of model (Equation5
(5)
(5) ).
Now let us verify that . Consider the following systems:
(7)
(7)
(8)
(8)
(9)
(9)
(10)
(10)
(11)
(11) On the basis of the comparison theorem for stochastic differential equations [Citation15],we get for
,
(12)
(12) According to Theorem 2.2 in Jiang and Shi [Citation19], we get
(13)
(13)
(14)
(14)
(15)
(15)
(16)
(16)
(17)
(17) Due to the fact that
and
are global, we can know that
.
Lemma 2.3
[Citation30]
Let , where
denotes the family of all continuous positive-valued functions defined on
.
(i) | If there exist three positive constants | ||||
(ii) | If there exist three positive constants |
Definition 2.1
(1) is said to be extinct if
, a.s. (2)
is said to be strongly persistent in the mean if
, a.s. (3)
is said to be persistent in the mean if
, a.s.
Theorem 2.1
For model (Equation5(5)
(5) ), if
then
become extinct,
a.s.,
Proof.
Applying formula to model (Equation5
(5)
(5) )gives
As a consequence,
(18)
(18)
(19)
(19)
(20)
(20) We derive from (Equation18
(18)
(18) ) that, for sufficiently large t,
(21)
(21) Note that
and
, thus
a.s. In the same way,
means that
a.s.,
means that
a.s.
Theorem 2.2
For model (Equation5(5)
(5) ), if
and
, then
become extinct and
is persistent in the mean,
a.s.
Here we only prove the case of , and the proof of
can be obtained similarly.
Proof.
Since
, we then deduce from Theorem 2.1 that
a.s. Then for sufficiently large t,
(22)
(22)
(23)
(23) Making use of Lemma 2.3 to (Equation22
(22)
(22) ) and (Equation23
(23)
(23) ) respectively, we obtain
Therefore, from the arbitrariness of ε, we can derive that
a.s.
Theorem 2.3
For model (Equation5(5)
(5) ), if
and
, then
become extinct and
is persistent in the mean,
a.s.
Proof.
Since , we then deduce from Theorem 2.1 that
a.s. Then for sufficiently large t, by (Equation18
(18)
(18) ), we know
(24)
(24)
(25)
(25) Making use of Lemma 2.3 to (Equation24
(24)
(24) ) and (Equation25
(25)
(25) ) respectively, we obtain
Therefore, from the arbitrariness of ε, we can derive that
a.s.
Theorem 2.4
For model (Equation5(5)
(5) ), if
and
, then
become extinct and
,
satisfies
where
That is to say, the populations
are strongly persistent in the mean.
Proof.
Since , we then deduce from Theorem 2.1 that
a.s.
By (Equation19(19)
(19) ) and (Equation20
(20)
(20) ), we know that for sufficiently large t,
(26)
(26)
(27)
(27)
(28)
(28)
(29)
(29) An application of (i) in Lemma 2.3 to the above inequalities and the arbitrariness of ε, we can deduce
(30)
(30)
(31)
(31) Substituting (Equation30
(30)
(30) , (Equation31
(31)
(31) ) into (Equation29
(29)
(29) ), (Equation27
(27)
(27) ) respectively results in
From Lemma 2.3(ii) we also deduce that
the proof is completed.
Remark 1.1 Theorems 2.1–2.4 reveals some important and interesting impacts of the speed of reversion, the intensity of stochastic perturbations and the toxicant impulsive period on the persistence and extinction of the species. In fact, we can see that the persistence or not of the resource species ,
and
depends on the size of
,i = 1, 2, 3.
3. Numerical simulations
Now we use the Milstein method offered in [Citation14] to verify the theoretical results numerically (here we only provide the functions of since the functions of
can be proffered analogously).
In Figures , choose ,
,
,
,
,
,
, a = 0.25,
,
,
,
,
,
,
,
,
,
,
,
,
,
,
,
, g = 0.9, q = 0.5. The only difference between conditions of Figures – is that the values of
,
and
are different, see Table .
Figure 1. Solutions of system (Equation3(3)
(3) ) for
and
![Figure 1. Solutions of system (Equation3(3) {dy1(t)=y1(t)[r10+(r~10−r10)e−α1t−r11C10(t)−ay1(t)−c2y2(t)h2+y1(t)−c3y3(t)h3+y1(t)]dt+σ1(t)y1(t)dB1(t),dy2(t)=y2[r20+(r~20−r20)e−α2t−r21C20(t)−a23y3(t)−f2y2(t)h2+y1(t)]dt+σ2(t)y2(t)dB2(t),dy3(t)=y3[r30+(r~30−r30)e−α3t−r31C30(t)−a32y2(t)−f3y3(t)h3+y1(t)]dt+σ3(t)y3(t)dB3(t),dCi0(t)dt=kiCe(t)−liCi0(t),i=1,2,3,dCe(t)dt=−gCe(t),t≠nγ,n∈Z+,ΔCe(t)=q,t=nγ,n∈Z+,(3) ) for r10=0.5,r20=0.5,r30=0.3,r~10=0.3,r~20=0.25,r~30=0.2,r11=r21=r31=0.9,a=0.25,c2=0.36,c3=0.4,f2=0.4,f3=0.47,a23=0.2,a32=0.15,h2=h3=1,α1=0.31,α2=0.35,α3=0.42,γ=2,k1=0.2,k2=0.26,k3=0.21,l1=0.8,l2=0.7,l3=0.7,g=0.9,q=0.5, and ξ12=0.55,ξ22=0.9,ξ32=0.5.](/cms/asset/7d0ea4ee-b3ce-4707-89ae-0c65d46fc815/tjbd_a_2155717_f0001_oc.jpg)
Figure 5. Solutions of system (Equation3(3)
(3) ) for
and
![Figure 5. Solutions of system (Equation3(3) {dy1(t)=y1(t)[r10+(r~10−r10)e−α1t−r11C10(t)−ay1(t)−c2y2(t)h2+y1(t)−c3y3(t)h3+y1(t)]dt+σ1(t)y1(t)dB1(t),dy2(t)=y2[r20+(r~20−r20)e−α2t−r21C20(t)−a23y3(t)−f2y2(t)h2+y1(t)]dt+σ2(t)y2(t)dB2(t),dy3(t)=y3[r30+(r~30−r30)e−α3t−r31C30(t)−a32y2(t)−f3y3(t)h3+y1(t)]dt+σ3(t)y3(t)dB3(t),dCi0(t)dt=kiCe(t)−liCi0(t),i=1,2,3,dCe(t)dt=−gCe(t),t≠nγ,n∈Z+,ΔCe(t)=q,t=nγ,n∈Z+,(3) ) for r10=0.5,r20=0.5,r30=0.3,r~10=0.3,r~20=0.25,r~30=0.2,r11=r21=r31=0.9,a=0.25,c2=0.36,c3=0.4,f2=0.4,f3=0.47,a23=0.2,a32=0.15,h2=h3=1,α1=0.31,α2=0.35,α3=0.42,γ=2,k1=0.2,k2=0.26,k3=0.21,l1=0.8,l2=0.7,l3=0.7,g=0.9,q=0.5, and ξ12=0.55,ξ22=0.16,ξ32=0.09.](/cms/asset/be96d79e-17cf-4f81-bf97-781223954610/tjbd_a_2155717_f0005_oc.jpg)
Table 1. The values with and
(i=1,2,3).
From Table , we know the following cases.
In Figure ,
,
,
. According to Theorem 2.1,
,
and
die out. Figure confirms these.
In Figure ,
,
,
. By Theorem 2.2,
and
die out and
See Figure .
becomes extinct and
is persistent in the mean, that is to say, the prey
is not the only food source for the predator
.
In Figure ,
,
,
. By Theorem 2.2,
and
die out and
See Figure .
becomes extinct and
is persistent in the mean, that is to say, the prey
is not the only food source for the predator
.
In Figure ,
,
,
. Thus according to Theorem 2.3,
and
die out and
See Figure . This means that when predators
and
are extinct, the prey
will survive without natural enemies.
In Figure ,
,
,
,
,
,
,
. Thus according to Theorem 2.4,
dies out and
Figure confirms these.
dies out,
and
are strongly persistent in the mean. that is to say, the prey
is not the only food source for predators
and
.
Compared with Figures –, we find that with the increase of intensity of stochastic perturbations
, species
tends to die out while the predator
and
remain persistence. That is to say, the stochastic perturbations on the growth rate of
is harmful for the persistence of
and does not imply the extinction of
and
. Similarly, with the increase of
,
,i = 2, 3, tends to die out while
tends to be persistent. From a biological point of view, this is reasonable.
is intensity of stochastic perturbations, and growth rates are sensitive to these stochastic perturbations. Hence the stochastic perturbations on the growth rate of a species is harmful for the persistence of this species. In addition, although prey
tends to die out, predators
and
can choose other foods, hence the parameter
does not affect the survival of predators.
In Figures and , we choose
,
,
,
,
,
,
,
,
, a = 0.5,
,
,
,
,
,
,
,
,
,
,
,
,
,
,
, g = 0.9, q = 0.16,
,
,
. The only difference between conditions of Figures and is that the values of γ are different, see Table .
From Table , we know the following cases.
In Figure , we choose
. Then
,
,
,
,
,
, and
. By Theorem 2.4, we have
die out and
Figure confirms these.
In Figure , choose
. Then
,
and
. Thus according to Theorem 2.1,
,
and
die out. See Figure .
Figure 2. Solutions of system (Equation3(3)
(3) ) for
and
![Figure 2. Solutions of system (Equation3(3) {dy1(t)=y1(t)[r10+(r~10−r10)e−α1t−r11C10(t)−ay1(t)−c2y2(t)h2+y1(t)−c3y3(t)h3+y1(t)]dt+σ1(t)y1(t)dB1(t),dy2(t)=y2[r20+(r~20−r20)e−α2t−r21C20(t)−a23y3(t)−f2y2(t)h2+y1(t)]dt+σ2(t)y2(t)dB2(t),dy3(t)=y3[r30+(r~30−r30)e−α3t−r31C30(t)−a32y2(t)−f3y3(t)h3+y1(t)]dt+σ3(t)y3(t)dB3(t),dCi0(t)dt=kiCe(t)−liCi0(t),i=1,2,3,dCe(t)dt=−gCe(t),t≠nγ,n∈Z+,ΔCe(t)=q,t=nγ,n∈Z+,(3) ) for r10=0.5,r20=0.5,r30=0.3,r~10=0.3,r~20=0.25,r~30=0.2,r11=r21=r31=0.9,a=0.25,c2=0.36,c3=0.4,f2=0.4,f3=0.47,a23=0.2,a32=0.15,h2=h3=1,α1=0.31,α2=0.35,α3=0.42,γ=2,k1=0.2,k2=0.26,k3=0.21,l1=0.8,l2=0.7,l3=0.7,g=0.9,q=0.5, and ξ12=0.55,ξ22=0.16,ξ32=0.5.](/cms/asset/173f1b19-312a-4482-9b30-9bb2f49e3f59/tjbd_a_2155717_f0002_oc.jpg)
Figure 3. Solutions of system (Equation3(3)
(3) ) for
and
![Figure 3. Solutions of system (Equation3(3) {dy1(t)=y1(t)[r10+(r~10−r10)e−α1t−r11C10(t)−ay1(t)−c2y2(t)h2+y1(t)−c3y3(t)h3+y1(t)]dt+σ1(t)y1(t)dB1(t),dy2(t)=y2[r20+(r~20−r20)e−α2t−r21C20(t)−a23y3(t)−f2y2(t)h2+y1(t)]dt+σ2(t)y2(t)dB2(t),dy3(t)=y3[r30+(r~30−r30)e−α3t−r31C30(t)−a32y2(t)−f3y3(t)h3+y1(t)]dt+σ3(t)y3(t)dB3(t),dCi0(t)dt=kiCe(t)−liCi0(t),i=1,2,3,dCe(t)dt=−gCe(t),t≠nγ,n∈Z+,ΔCe(t)=q,t=nγ,n∈Z+,(3) ) for r10=0.5,r20=0.5,r30=0.3,r~10=0.3,r~20=0.25,r~30=0.2,r11=r21=r31=0.9,a=0.25,c2=0.36,c3=0.4,f2=0.4,f3=0.47,a23=0.2,a32=0.15,h2=h3=1,α1=0.31,α2=0.35,α3=0.42,γ=2,k1=0.2,k2=0.26,k3=0.21,l1=0.8,l2=0.7,l3=0.7,g=0.9,q=0.5, and ξ12=0.55,ξ22=0.9,ξ32=0.09.](/cms/asset/a616060b-2628-4d27-968f-9c556bc9e939/tjbd_a_2155717_f0003_oc.jpg)
Figure 4. Solutions of system (Equation3(3)
(3) ) for
and
![Figure 4. Solutions of system (Equation3(3) {dy1(t)=y1(t)[r10+(r~10−r10)e−α1t−r11C10(t)−ay1(t)−c2y2(t)h2+y1(t)−c3y3(t)h3+y1(t)]dt+σ1(t)y1(t)dB1(t),dy2(t)=y2[r20+(r~20−r20)e−α2t−r21C20(t)−a23y3(t)−f2y2(t)h2+y1(t)]dt+σ2(t)y2(t)dB2(t),dy3(t)=y3[r30+(r~30−r30)e−α3t−r31C30(t)−a32y2(t)−f3y3(t)h3+y1(t)]dt+σ3(t)y3(t)dB3(t),dCi0(t)dt=kiCe(t)−liCi0(t),i=1,2,3,dCe(t)dt=−gCe(t),t≠nγ,n∈Z+,ΔCe(t)=q,t=nγ,n∈Z+,(3) ) for r10=0.5,r20=0.5,r30=0.3,r~10=0.3,r~20=0.25,r~30=0.2,r11=r21=r31=0.9,a=0.25,c2=0.36,c3=0.4,f2=0.4,f3=0.47,a23=0.2,a32=0.15,h2=h3=1,α1=0.31,α2=0.35,α3=0.42,γ=2,k1=0.2,k2=0.26,k3=0.21,l1=0.8,l2=0.7,l3=0.7,g=0.9,q=0.5, and ξ12=0.15,ξ22=0.9,ξ32=0.5.](/cms/asset/ab67f530-d6de-424d-aa22-0e1725373ada/tjbd_a_2155717_f0004_oc.jpg)
Figure 6. Solutions of system (Equation3(3)
(3) ) for
and
![Figure 6. Solutions of system (Equation3(3) {dy1(t)=y1(t)[r10+(r~10−r10)e−α1t−r11C10(t)−ay1(t)−c2y2(t)h2+y1(t)−c3y3(t)h3+y1(t)]dt+σ1(t)y1(t)dB1(t),dy2(t)=y2[r20+(r~20−r20)e−α2t−r21C20(t)−a23y3(t)−f2y2(t)h2+y1(t)]dt+σ2(t)y2(t)dB2(t),dy3(t)=y3[r30+(r~30−r30)e−α3t−r31C30(t)−a32y2(t)−f3y3(t)h3+y1(t)]dt+σ3(t)y3(t)dB3(t),dCi0(t)dt=kiCe(t)−liCi0(t),i=1,2,3,dCe(t)dt=−gCe(t),t≠nγ,n∈Z+,ΔCe(t)=q,t=nγ,n∈Z+,(3) ) for r10=0.2,r20=0.1,r30=0.2,r~10=0.1,r~20=0.05,r~30=0.13,r11=2,r21=1,r31=2.1,a=0.5,c2=0.2,c3=0.2,f2=0.85,f3=0.77,a23=0.2,a32=0.15,h2=h3=1,α1=0.81,α2=0.85,α3=0.79,γ=3,k1=0.11,k2=0.12,k3=0.035,l1=0.6,l2=0.7,l3=0.5,g=0.9,q=0.16, and ξ12=0.75,ξ22=0.006,ξ32=0.29.](/cms/asset/46e017a5-f545-44ab-ba95-7d7f7296b10e/tjbd_a_2155717_f0006_oc.jpg)
Figure 7. Solutions of system (Equation3(3)
(3) ) for
and
![Figure 7. Solutions of system (Equation3(3) {dy1(t)=y1(t)[r10+(r~10−r10)e−α1t−r11C10(t)−ay1(t)−c2y2(t)h2+y1(t)−c3y3(t)h3+y1(t)]dt+σ1(t)y1(t)dB1(t),dy2(t)=y2[r20+(r~20−r20)e−α2t−r21C20(t)−a23y3(t)−f2y2(t)h2+y1(t)]dt+σ2(t)y2(t)dB2(t),dy3(t)=y3[r30+(r~30−r30)e−α3t−r31C30(t)−a32y2(t)−f3y3(t)h3+y1(t)]dt+σ3(t)y3(t)dB3(t),dCi0(t)dt=kiCe(t)−liCi0(t),i=1,2,3,dCe(t)dt=−gCe(t),t≠nγ,n∈Z+,ΔCe(t)=q,t=nγ,n∈Z+,(3) ) for r10=0.2,r20=0.1,r30=0.2,r~10=0.1,r~20=0.05,r~30=0.13,r11=2,r21=1,r31=2.1,a=0.5,c2=0.2,c3=0.2,f2=0.85,f3=0.77,a23=0.2,a32=0.15,h2=h3=1,α1=0.81,α2=0.85,α3=0.79,γ=0.2,k1=0.11,k2=0.12,k3=0.035,l1=0.6,l2=0.7,l3=0.5,g=0.9,q=0.16, and ξ12=0.75,ξ22=0.006,ξ32=0.29.](/cms/asset/55d404e0-452a-4131-adb0-a7be8de28ad6/tjbd_a_2155717_f0007_oc.jpg)
Table 2. The values with γ and (i=1,2,3).
By comparing Figures with , one can observe that the toxicant impulsive period γ in polluted environments affects species survival. Moreover,with the decrease of γ, species tends to die out.
4. Conclusions
In this paper, we take advantage of a mean-reverting Ornstein-Uhlenbeck process to portray the random perturbations in the environment and formulate a modified Leslie–Gower Holling-type II two-predator one-prey stochastic model in polluted environments with impulsive toxicant input. We consider interspecific competition between predators and we obtain sharp sufficient conditions for persistence in the mean, strongly persistent in the mean and extinction for each species of model (Equation3(3)
(3) ).
The theorems that we obtain have some interesting biological interpretations. By these Theorems we can see that each species is either extinct, strongly persistent in the mean or persistent in the mean, relying on the sign of .
We note that the intensity of the perturbation and the speed of reversion
are two key parameters in the Ornstein-Uhlenbeck process. Obviously,
Therefore, we conclude that along with the increase of
(respectively,
),
will increase (respectively, decrease). That is, increasing the reversion rate
is advantageous for the survival of species
, while increasing intensity
is harmful for them.
Some interesting topics remain to be solved. For example, it would be interesting to dissect other random noises such as the telephone noise(see [Citation23]), the Lévy noise(see [Citation3]) or reaction diffusion(see [Citation2]) etc. We leave these questions for future research.
Disclosure statement
No potential conflict of interest was reported by the authors.
Additional information
Funding
References
- M.A. Aziz-Alaoui and M.D. Okiye, Boundedness and global stability for a predator-prey model with modified Leslie–Gower and Holling-type II schemes, Appl. Math. Lett. 16 (2003), pp. 1069–1075.
- C. Bai, Multiplicity of solutions for a class of nonlocal elliptic operators systems, Bull. Korean Math. Soc. 54 (2017), pp. 715–729.
- J.H. Bao, X.R. Mao, G. Yin, and C.G. Yuan, Competitive Lotka–Volterra population dynamics with jumps, Nonlinear Anal. Real World Appl. 74 (2011), pp. 6601–6616.
- J.R. Beddington, Mutual interference between parasites or predators and its effect on searching efficiency, J. Anim. Ecol. 44 (1975), pp. 331–340.
- B. Buonomo, A.D. Liddo, and I. Sgura, A diffusive-convective model for the dynamics of population-toxicant intentions: Some analytical and numerical results, Math. Biosci. 157 (1999), pp. 37–64.
- Y.L. Cai, J.J. Jiao, Z.J. Gui, Y.T. Liu, and W.M. Wang, Environmental variability in a stochastic epidemic model, Appl. Math. Comput. 329 (2018), pp. 210–226.
- H.I. Freedman and J.B. Shukla, Models for the effect of toxicant in single-species and predator-prey systems, J. Math. Biol. 30 (1991), pp. 15–30.
- Y.X. Gao and S.y. Yao, Persistence and extinction of a modified Leslie–Gower Holling-type II predator-prey stochastic model in polluted environments with impulsive toxicant input, Math. Biosci. Eng. 18 (2021), pp. 4894–4918.
- T.G. Hallam, C.E. Clark, and G.S. Jordan, Effffects of toxicant on population: A qualitative approach II. First order kinetics, J. Math. Biol. 109 (1983), pp. 411–429.
- T.G. Hallam, C.E. Clark, and R.R. Lassiter, Effects of toxicants on populations: A qualitative approach I. Equilibrium environmental exposure, Ecol. Model. 8 (1983), pp. 291–304.
- T.G. Hallam and J.L. Deluna, Effffects of toxicant on populations: A qualitative approach III. Environmental and food chain pathways, J. Theor. Biol. 109 (1984), pp. 411–429.
- T.G. Hallam and Z. Ma, Persistence in population models with demographic fluctuations, J. Math. Biol. 24 (1986), pp. 327–339.
- Q.X. Han, D.Q. Jiang, and C.Y. Ji, Analysis of a delayed stochastic predator-prey model in a polluted environment, Appl. Math. Model. 38 (2014), pp. 3067–3080.
- D.J. Higham, An algorithmic introduction to numerical simulation of stochastic differential equations, J. Siam Rev. 43(3) (2001), pp. 525–546.
- N. Ikeda and S. Wantanabe, Stochastic Differential Equations and Diffusion Processes, North-Holland, Amsterdam, 1981.
- A.L. Jensen and S.J. Marshall, Application of a surplus production model to assess environmental impacts on exploited populations of daphnia pulex in the laboratory, J. Environ. Pollut. 28 (1982), pp. 273–280.
- C. Ji, D. Jiang, and N. Shi, Analysis of a predator-prey model with modified Leslie–Gower and Holling type II schemes with stochastic perturbation, J. Math. Anal. Appl. 359 (2009), pp. 482–498.
- C. Ji, D. Jiang, and N. Shi, A note on a predator-prey model with modified Leslie–Gower and Holling-type II schemes with stochastic perturbation, J. Math. Anal. Appl. 377 (2011), pp. 435–440.
- D.Q. Jiang and N.Z. Shi, A note on non-autonomous logistic equation with random perturbation, J. Math. Anal. Appl. 303 (2005), pp. 164–172.
- E.L. Johnston and M.J. Keough, Field assessment of effects of timing and frequency of copper pulses on settlement of sessile marine invertebrates, Mar. Biol. 137 (2000), pp. 1017–1029.
- E.L. Johnston, M.J. Keough, and P.Y. Qian, Maintenance of species dominance through pulse disturbances to a sessile marine invertebrate assemblage in port shelter, Mar. Ecol. Prog. Ser. 226 (2002), pp. 103–114.
- J. Liang, S. Tang, J.J. Nieto, and R.A. Cheke, Analytical methods for detecting pesticide switches with evolution of pesticide resistance, Math. Biosci. 245 (2013), pp. 249–257.
- M. Liu, Dynamics of a stochastic regime-switching predator-prey model with modified Leslie–Gower Holling-type II schemes and prey harvesting, Nonlinear Dyn. 96 (2019), pp. 417–442.
- M. Liu and C.Z. Bai, Persistence and extinction of a stochastic cooperative model in a polluted environment with pulse toxicant input, Filomat 29 (2015), pp. 1329–1342.
- Q. Liu and Q.M. Chen, Dynamics of stochastic delay Lotka–Volterra systems with impulsive toxicant input and Lévy noise in polluted environments, Appl. Math. Comput. 256 (2015), pp. 52–67.
- B. Liu, L. Chen, and Y. Zhang, The effects of impulsive toxicant input on a population in a polluted environment, J. Biol. Syst. 11 (2003), pp. 265–274.
- M. Liu, C. Du, and M. Deng, Persistence and extinction of a modified Leslie–Gower Holling-type II stochastic predator-prey model with impulsive toxicant input in polluted environments, J. Nonlinear Anal. Hybrid. Syst. 27 (2018), pp. 177–190.
- H. Liu and Z. Ma, The threshold of survival for system of two species in a polluted environment, J. Math. Biol. 30 (1991), pp. 49–51.
- M. Liu and K. Wang, Dynamics of a Leslie–Gower Holling-type II predator-prey system with Lévy jumps, Nonlinear Anal. 85 (2013), pp. 204–213.
- M. Liu, K. Wang, and Q. Wu, Survival analysis of stochastic competitive models in a polluted environment and stochastic competitive exclusion principle, Bull. Math. Biol. 73 (2011), pp. 1969–2012.
- B. Liu and L. Zhang, Dynamics of a two-species Lotka–Volterra competition system in a polluted environment with pulse toxicant input, Appl. Math. Comput. 214 (2009), pp. 155–162.
- Z. Ma and T.G. Hallam, Effects of parameter fluctuations on community survival, Math. Biosci. 86 (1987), pp. 35–49.
- R.M. May, Stability and Complexity in Model Ecosystems, Princeton University Press, Princeton (NJ), 2001.
- A. Nelson-SmithThe Problem of Oil Pollution of the Sea, Academic Press, 1971.
- A.F. Nindjin, M.A. Aziz-Alaoui, and M. Cadivel, Analysis of a predator-prey model with modified Leslie–Gower and Holling-type II schemes with time delay, Nonlinear Anal. RWA 7 (2006), pp. 1104–1118.
- J. Pan, Z. Jin, and Z. Ma, Thresholds of survival for an n-dimensional volterra mutualistic system in a polluted environment, J. Math. Anal. Appl. 252 (2000), pp. 519–531.
- J.B. Shukla, I.H. Freedman, V.M.. Pal, O.P.. Misra, M. Agarwal, and A. Shukla, Degradation and subsequent regeneration of a forestry resource: A mathematical model, J. Ecol. Modell. 44 (1989), pp. 219–229.
- G.T. Skalski and J.F. Gilliam, Functional responses with predator interference: Viable alternatives to the Holling type II model, Ecology 82 (2001), pp. 3083–3092.
- X. Song and Y. Li, Dynamic behaviors of the periodic predator-prey model with modified Leslie–Gower Holling-type II schemes and impulsive effect, Nonlinear Anal. RWA 9 (2008), pp. 64–79.
- F.Y. Wei, S.A.H. Geritz, and J.Y. Cai, A stochastic single-species population model with partial pollution tolerance in a polluted environment, Appl. Math. Lett. 63 (2017), pp. 130–136.
- Y. Xu, M. Liu, and Y. Yang, Analysis of a stochastic two-predators one-prey system with modified Leslie–Gower and Holling-type II schemes, J. Appl. Anal. Comput. 7 (2017), pp. 713–727.
- X. Yang, Z. Jin, and Y. Xue, Weak average persistence and extinction of a predator-prey system in a polluted environment with impulsive toxicant input, Chaos Solit. Fractals 31 (2007), pp. 726–735.
- S.W. Zhang and D.J. Tan, Dynamics of a stochastic predator-prey system in a polluted environment with pulse toxicant input and impulsive perturbations, Appl. Math. Model. 39 (2015), pp. 6319–6331.
- W.C. Zhao, J. Li, T.Q. Zhang, X.Z. Meng, and T.H. Zhang, Persistence and ergodicty of plant disease model with Markov conversion and impulsive toxicant input, Commun. Nonlinear Sci. Numer. Simul. 48 (2017), pp. 70–84.
- Y. Zhao, S.L. Yuan, and J.L. Ma, Survival and stationary distribution analysis of a stochastic competitive model of three species in a polluted environment, Bull. Math. Biol. 77 (2015), pp. 1285–1326.