Abstract
The volume of publically available geospatial data on the web is rapidly increasing due to advances in server-based technologies and the ease at which data can now be created. However, challenges remain with connecting individuals searching for geospatial data with servers and websites where such data exist. The objective of this paper is to present a publically available Geospatial Search Engine (GSE) that utilizes a web crawler built on top of the Google search engine in order to search the web for geospatial data. The crawler seeding mechanism combines search terms entered by users with predefined keywords that identify geospatial data services. A procedure runs daily to update map server layers and metadata, and to eliminate servers that go offline. The GSE supports Web Map Services, ArcGIS services, and websites that have geospatial data for download. We applied the GSE to search for all available geospatial services under these formats and provide search results including the spatial distribution of all obtained services. While enhancements to our GSE and to web crawler technology in general lie ahead, our work represents an important step toward realizing the potential of a publically accessible tool for discovering the global availability of geospatial data.
1. Introduction
Vast amounts of data are generated every day from satellites, ground sensors, computer simulations, and mobile devices, all of which are driving the current data-intensive paradigm of science (Hey, Tansley, and Tolle Citation2009). This paradigm is one where scientists, professionals, and the public can collectively contribute data in an array of formats to the web where they are stored, analyzed, synthesized, and visualized through a multitude of web-based applications. Geospatial data representing digital information about the Earth’s surface is a significant component in this new paradigm, as creating geospatial data has become easier than ever before (Elwood Citation2010), especially with the availability of web-based platforms that provide mapping tools and server architecture to enable flexible opportunities for hosting and sharing data. Web-based platforms for sharing digital data are essential for enhancing knowledge about the Earth’s surface and the processes by which it is governed. Platforms provide access to various digital representations of the Earth, not only facilitating knowledge discovery through examining the data itself but also by enhancing the availability of data that can be used as input in models that predict or simulate Earth system processes at a variety of scales. In this sense, platforms for data access and sharing are an integral component of a broader Digital Earth framework. As a result, the Open Geospatial Consortium (OGC) has increasingly improved protocols for standardizing how geospatial data are stored and shared over the web (Whiteside and Greenwood Citation2010), which has led to a large number of government organizations, research institutions, and private companies deploying Web Map Services (WMS) and implementing Open Web Services to provide both server and client functions for searching and accessing data (Yang and Tao Citation2006).
The most prominent avenue for accessing geospatial data on the web has been through geoportals: web-based applications of organized directories, search tools, support, rules, and processes aimed at the acquisition and sharing of geospatial data (Tait Citation2005; Yang et al. Citation2007). Geoportals are typically part of an organization’s spatial data infrastructure (SDI) that are developed for arranging technology, standards, rules, and processes for data handling (Maguire and Longely Citation2005). The United States National Spatial Data Infrastructure (NSDI), for example, was developed in 1992 by the Federal Geographic Data Committee to provide a set of standards for describing and accessing government data. As part of the NSDI, a geoportal called the Geospatial One-Stop was developed to provide hundreds of geospatial records to thousands of users at multiple levels of government and non-government organizations (Goodchild Citation2009). While the One-Stop geoportal has become part of a larger government data clearinghouse called Data.gov, several countries around the world and numerous agencies and academic institutions continue to utilize geoportals to share geospatial data both internally and publically. Yet, geoportals are increasingly critiqued for being out-of-sync with the evolving nature of geospatial data and the needs of its users. Some critiques cite cognitive and security issues (Goodchild Citation2007), while others have found the principles of geoportals irrelevant to the evolving needs of local governments (Harvey and Tulloch Citation2006). At the same time, some have criticized the one-directional flow of information in typical SDIs from main geospatial data products to geospatial data consumers (Elwood Citation2008). This has led to efforts for rethinking the relationship between the web and SDIs in a way that can facilitate the contributions of volunteered geographic information and the concept of the Web 2.0 (Craglia Citation2007; Goodchild, Fu, and Rich Citation2008; Budhathoki, Bruce, and Nedovic-Budic Citation2008; De Longueville Citation2010; Masó, Pons, and Zabala Citation2012).
This is not to say that SDIs and their related geoportals are irrelevant in today’s data-driven society. On the contrary, they facilitate the existence of numerous enterprises and have allowed the sharing of content across the web. Furthermore, there have been several key recent developments in geospatial data access, such as portal development based on spatial web-based architectures (Yang et al. Citation2007), grid-based computing (Wang and Liu Citation2009; Zhang and Tsou Citation2009), multi-threading techniques that improve client-side performance (Yang et al. Citation2005), and performing content analysis of geospatial metadata (Vockner, Richter, and Mittlbock Citation2013), all of which facilitate enhanced means of data access. Yet, it can be argued that data-driven science also requires a means to search for geospatial data beyond the frameworks of silo infrastructures and, instead, across the web in order to discover unknown data residing on servers outside of geospatial enterprise initiatives.
A solution to this need has been the more recent development of web crawler techniques that can search the entire web for geospatial data (Li et al. Citation2010; Walter, Luo, and Fritsch Citation2013). Web crawlers are Internet applications that browse the web with the main purpose of indexing web-based content. Crawlers initially visit a list of specified uniform resource locators (URLs), and then expand this list by identifying hyperlinks and adding those URLs to a ‘frontier’ list. Sites on the frontier list are recursively visited based on a defined set of rules to determine whether they still contain search criteria. In doing so, a web crawler provides a deep yet up-to-date list of sites that meet some criteria while eliminating URLs that become irrelevant or broken over time. With regard to geospatial data, web crawlers are used to search for services as well as websites containing data in various spatially related formats, and cataloging the results in a database (Sample et al. Citation2006; Schutzberg Citation2006; Li et al. Citation2011). Once services are obtained, it is possible for crawlers to further interrogate data, metadata, and server-level information to build a knowledgebase describing many aspects of the data. Furthermore, web crawlers have multiple uses in addition to searching for geospatial data, such as determining the number of OGC services online at a given point in time (López-Pellicer et al. Citation2012a), building geospatial products such as an orthophoto coverage database (Florczyk et al. Citation2012), and serving as a method for spatial analysis to monitor the spatial distribution of some geographically dependent event (Galaz et al. Citation2010).
Web crawlers have previously been developed using sophisticated reasoning and discovery algorithms to strategically search and catalog available services. One of the earliest viable examples is Mapdex, a commercial search engine that focused on Esri ArcIMS resources. The cataloging of a large number of services by Mapdex and similar applications generated much interest in utilizing existing search engines for locating services across the web. Refractions Research (Sample et al. Citation2006), for example, provides a crawler that utilizes Google application programming interface (API) for discovering WMS for Esri’s ArcView software. Similarly, the Naval Research Laboratory’s Geospatial Information Database (GIDB) utilizes, but does not entirely depend upon, the Google search engine API in its web crawler that locates and interrogates WMS metadata documents (see López-Pellicer et al. [Citation2012a] for a more in depth description of GIDB and related search engines). Other search engines have since been developed (Walter, Luo, and Fritsch Citation2013; Chen et al. Citation2011; Patil, Bhattacharjee, and Ghosh Citation2014), some utilizing existing search engines like Google and others developing customized search applications to enhance efficiency, with a range of success in the number of WMS returned to the client. López-Pellicer et al. (Citation2012a) report that the early developed Mapdex returns 129 WMS services and 51,865 ArcIMS services, while more recent search engines focus only on cataloging WMS services, such as Refractions Research (612 WMS services), Skylab (904 WMS services), Li et al. Citation2010 (1126 WMS services), and GIDB (1187 WMS services). However, while the number of located services is an important feature, several other factors are necessary for maintaining an active and current geospatial service catalog and providing a useful search engine. These include prioritizing seed URLs during crawling, continually updating the catalog by adding newly found services and dropping invalid services, locating a range of mapping services, and providing technical design and performance evaluation metrics in order that for the broader scientific community to understand how the search engine was developed and how it performs. As Li et al. (Citation2010) highlight, few, if any, search engines accomplish all these tasks. Furthermore, not all search engines are publically available on the web, which confines the availability of services to specific commercial software applications and constrains the types of cataloged services to those that are located by proprietary search engines.
This paper presents a new concept in geospatial search engines (GSE) by overcoming these limitations and accomplishing all of the following tasks: (1) the use of a seeding mechanism to prioritize searches; (2) updates to the catalog of services by adding new services and removing invalid services; (3) locates a range of data and data sources by returning WMS, ArcGIS services, and ArcIMS services, as well as websites containing data in shapefile formats; and (4) catalogs metadata and server information. While none of these concepts in and of themselves are novel, our GSE provides a more comprehensive set of search strategies and documentation than previously developed search engines. In addition, the developed GSE is publically available for any client to use for searching the web for data – a service not provided by all previously developed GSEs. We provide extensive documentation to illustrate how our GSE was developed and searches for geospatial data. We provide a system overview and an explanation of the major functionality, along with an example query, and use the system to report on the current status of server numbers as wells as the spatial distribution of data obtained by the GSE. We also evaluate the effectiveness of the GSE by comparing advantageous and disadvantageous elements to other crawling techniques, and conclude by discussing potential future enhancements as well as opportunities to leverage intelligent web crawlers with conventional search engines and geospatial catalogs.
2. The geospatial search engine
The development of the GSE was directed by the USDA Forest Service’s Western Wildland Environmental Threat Assessment Center (WWETAC). The center was established in 2005 with the mission of facilitating the development of tools and methods for the assessment of multiple interacting disturbance processes (fire, insects, disease, climate change, etc.) that may affect western US forests and wildlands. The mandate of WWETAC is to carry out a program using geospatial and information management technologies, including remote sensing imaging and decision support systems to inventory, monitor, characterize, and assess forest conditions. This requires careful assessment of current geospatial technologies and geographic data, and the context within which they are used.
2.1. System overview
The GSE is a web application that combines a searchable database of geospatial servers and layers, with a web crawler for locating new geospatial data as well as updating an existing geospatial database. The conceptual configuration of the GSE is presented in . The client application runs in any standard web browser, and is built using JavaScript and open source mapping components such as Open Layers (Citation2010) and GeoExt (Citation2010). The GSE is currently a standalone application, but there are plans to build a user friendly API to allow further access to the search engine. The server hosts an ASP.NET application for searching and updating the database. The application is hosted in the Amazon Web Services cloud and is available, along with documentation, at http://www.wwetac.us/GSE/GSE.aspx.
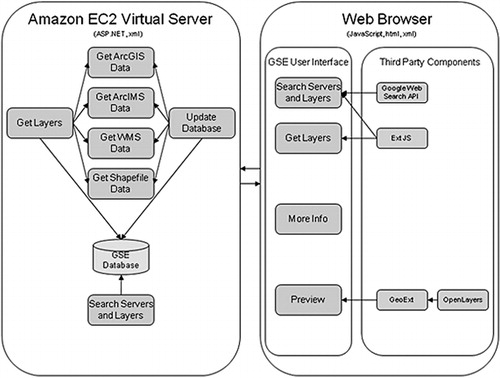
The GSE uses a single-level web crawler built on top of the Google search engine. The term single level implies that the crawler only searches services returned through the search, and not any links contained in these services. A crawler seeding mechanism combines search terms entered by users with predefined keywords that identify map servers. An update procedure runs daily to refresh map server layers and metadata, and to eliminate servers that go offline. The GSE also has an option to manually enter a known map server URL or seed the system with a list of known map server URLs.
2.2. Web crawler design
A web crawler is incorporated into the GSE application to both update web server data and locate new geospatial services on the Internet. When a user searches for map services and layers, the user-specified terms are combined with predefined terms and sent to the Google search engine in order to retrieve web pages related to both the user terms and potential geospatial data. The Google search engine results are added to a list of URLs stored on the server. The list of URLs is analyzed daily to find map services mentioned in the websites (). Analyzed URLs are added to a list and are only analyzed again after a predefined time period to improve overall performance. Map services mentioned in the website that are not already in the database are contacted to retrieve metadata. If the metadata are successfully returned, these data are added to the database (). A listing of the database fields can be obtained in the GSE technical description in Bunzel (Citation2012).
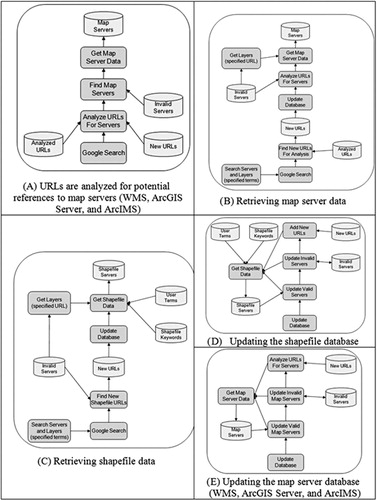
When a user searches for shapefiles, Google returns a list of websites that mention the user-specified terms, and that also mention shapefiles available for download (). The list of URLs is analyzed daily to find shapefile websites that are not already in the database. These websites are contacted and the website text is analyzed. The application has a list of keywords that are likely to be found in websites that have shapefiles available for download. The system will search for these keywords in the website text and rank the website according to how many keywords were found (described in detail in the next section). Keywords found in the web page title, URL, or description hold a greater weight in the ranking algorithm. If the website is ranked high enough, it is added to the database. The website plain text (without HyperText Markup Language [HTML] markup) is stored on the server to improve performance with future search operations. Shapefile websites are returned to the user interface sorted by rank. The process of updating the shapefile database is presented in .
The server database built by the web crawler is updated on a daily basis as depicted in . This process contacts all servers in the database and reloads metadata to ensure the latest changes are included in the system. Servers that do not respond or have an invalid response are added to a list of invalid servers. These servers are ignored in the future to improve performance. Invalid servers are periodically tested for validity; those that are found to be valid are moved to the main database, while those that remain invalid are removed entirely. This process will eventually remove old inactive servers from the system. During database updates, the system will also process the list of new URLs that were collected from Google search results.
2.3. Searching and ranking
Users can search the GSE database by entering terms in the user interface. As in most search engines, common words (i.e. stop words) are eliminated from the search terms unless they are enclosed in quotes. Users will also need to enclose terms in quotes in order to search for a specific phrase. For example, if the term ‘Climate Change’ is not enclosed in quotes, the search engine will return all items that include both the words ‘climate’ and ‘change’ anywhere in the text. The application will search for these terms in the title, abstract, and keywords at both the server level and the map layer level. For shapefiles, the application will search for the terms in the web page title, description, and main text.
Services and layers that meet search criteria, t, are ranked and returned to the client browser in EXtensible Markup Language (XML) format. The services are ranked higher if t is located in the server abstract, keywords, URL, or title. The rank is increased for each layer that contains t in the layer abstract, keywords, or title. The ranking procedure takes place by assigning integer weights to service s, in the list of servers found, S, if s contains t. If so, s is added to the list of services, S k, that contain matches to t. The weighting system is further described by the following pseudocode:
for s in S:
search for t in abstract, keywords, or title
if t is found:
ws = ws + 1000
for l in s:
search for t in abstract, keywords, or title
if terms is found:
ws = ws + 10
Add l node to L for this service
if the w s > 0
add s to Sk
The list of ranked servers, S k, is then returned to the GSE user interface.
Websites containing shapefiles are identified and ranked using a system involving multiple predefined keywords and a keyword weight system. The keywords are stored in a table on the server where each keyword is assigned weights according to the importance of that keyword in identifying a website. For example, the keywords ‘Shapefile’ and ‘Download’ are both assigned a weight of 10, whereas the keyword ‘data’ is assigned a weight of 8. The weighting of keywords was determined by a calibration procedure that produced the following weighting framework: shape file = 10; shape file = 10; shp = 10; download = 10; GIS = 9; ftp = 10; zip = 10; tar = 10; data = 8; layer = 7; map = 7; esri = 7.
Calibration was performed ad hoc, where each parameter setting was varied while holding other parameters constant. The final setting for each parameter was selected based on those that provided the highest number of returned services over a given period of evaluation. While future planned developments of our GSE include an advanced automated calibration procedure, the methods employed here are suitable due to the relatively small number of parameters. Each website is examined for the existence of any of the predefined keywords and the total weights are calculated. The keyword and weight table can be modified to calibrate the system for maximum efficiency in identifying websites with shapefiles for download. The following describes in detail the process used for analyzing and ranking a website, q, in the list of websites, Q, for potential shapefile download:
for q in Q:
for each t:
if t is found in q text:
wq = wq + wt
if t is found in q title or URL
wq = wq + 1000
The ranking of q is recalculated and displayed to the user in the GSE user interface that is a browser application built using open source components such as Ext JS, GeoExt (Citation2010), and Open Layers (Citation2010). The Google Web Search API is integrated to obtain web search results based on user-specified terms that are used to help build the GSE database. The interface allows users to specify search terms in order to retrieve a list of map services and layers. For shapefiles, a web page link is provided so that the user may review the web page containing shapefiles available to download. The GSE results are returned in a tree view where a separate node is used for each of the three service types (WMS, ArcGIS Server, and ArcIMS) and websites with shapefiles. Below the server type node is a node for each map service, and each map service has nodes for each layer that meet the search criteria. It is possible to have a map service node with no layer sub nodes, which occurs when the map service abstract or keywords contain the search terms but none of the layer abstracts or keywords contain the terms. As the user selects nodes in the tree view, the total number of layers and the number of layers that meet the search criteria are displayed.
2.4. Spatial data search engine database
The GSE database is currently stored in XML format. The XML files can be retrieved and stored locally as needed. For each server, the database stores the type of service (WMS, ArcGIS, ArcIMS, or shapefile), URL, title, abstract, and keywords. At the layer level, the database stores the name, title, abstract, keywords, latitude/longitude bounding box, and spatial reference. There is also a list of invalid servers that do not respond as expected, which are temporarily stored in this list until they are eventually removed after multiple failed attempts to contact them. For shapefile websites, the GSE database stores the rank of the website as well as the filename of the locally stored file containing the text of the website.
2.5. Discovering layer-level metadata and server statistics
A utility function was developed to process the search engine results. This utility cycles through the servers returned from a search in the catalog. For each unique service, the utility requests the capabilities document from the server. This document stores all metadata for the map service in XML format in order to comply with a standard document format defined by the OGC (Citation2010). The XML document is read and parsed to obtain detail information on the map server such as the service abstract and layer-level metadata. The parsed data are summarized and stored in a table for each keyword search. The outputs from separate searches were combined in a spreadsheet for further summary statistics. We used the system described above to perform keyword queries and examine the number of unique services, matched layers, the presence of an abstract that described the layer, and the mean abstract length.
3. GSE results
The GSE was evaluated on 9 January 2014 to enumerate the population of geospatial services that it could locate on the web. The GSE located a total of 1893 servers from which it cataloged a total of 66,551 WMS, ArcGIS, and ArcIMS services, several of these containing multiple data layers. These results are comparable to the results of several crawler-based search engines presented by López-Pellicer et al. (Citation2012a). Our GSE catalogs a greater number of services than those listed in the review by López-Pellicer et al. (2Citation012b), largely due to the reason that we search a greater variety of services, and also because of the greater availability of geospatial services that are now available relative to the number of services available when previous search engines were evaluated. We interrogated the efficiency of the GSE by calculating the number of servers contacted within a specific time period:
Servers with WMS services: 823 contacted in 15 minutes (0.91/second) Servers ArcGIS services: 41 contacted in 15 minutes (0.05/second) Servers with ArcIMS services: 103 contacted in 15 minutes (0.11/second) Websites containing shapefiles: 857 contacted in 15 minutes (0.95/second).
We retrieved all geographic datasets located by the GSE across all available servers and combined them in order to visualize the spatial extent of all available data ().
To demonstrate the search capabilities of the GSE, a search for geospatial services using the term ‘Fire’ was performed on 24 February 2014, returning data layers in 42 services, and several hundred websites that contained downloadable shapefile data. From these results, we selected a specific service from the Global Risk Data Platform (http://preview.grid.unep.ch/), a United Nations initiative for providing geospatial data on global risk from natural hazards. The service is displayed in the map viewer (), which provides the user with a visualization of the available data. Narrowing the search to ‘Fire’ and ‘US’ provided few results that were focused over the USA, such as the selected 2009 fire centroids hosted by the State of California’s Natural Resource Agency (). These results demonstrate the ability of the GSE to search for both global- and national-level geospatial data, and in doing so return a broad variety of services and websites containing links to data.
4. Discussion
The ability to efficiently collect geospatial data across the web is a central component to the advancement of geospatial cyberinfrastructure (Yang et al. Citation2010). In this paper, we described our efforts to build an operational GSE to facilitate the discovery of online geospatial resources, and to provide a tool for rapidly assessing the state of online global geospatial data. The GSE described here is a tool that can facilitate the systematic examination of online geospatial data that can facilitate examination of Earth system processes.
Our system leverages Google search capabilities for locating putative geospatial resources, and subsequently interrogates each candidate site for both server-level metadata and layer-specific data. By relying on Google to build the database, we avoid performance issues associated with systems that attempt to crawl the entire web. Building on top of Google ensures a complete coverage of the web, whereas a custom crawler that does not use Google results may miss some sites due to the difficulty in crawling the vast population of Internet servers. Although our system is likely missing some map server sites that do not contain the specific service identity keywords, we believe these omissions are minor since the lack of proper keywords would suggest either poor documentation or simply that the data are not intended for public access. Moreover, the Google ranking mechanism helps identify the most popular map services, and these are more likely to be relevant to the searched terms and also intended for public use.
As described in Li et al. (Citation2010), it is possible to search for certain keywords (‘WMS server’) that may indicate the possibility of a map service, and then test all the related URLs on the same page to see if they might contain a service. This method is hit or miss, but would likely result in additional map services found. We plan to explore the possibility of including this type of additional crawling in the future. The system would still rely on the Google search results, but additional searches would be performed using these keywords. The results of these searches would be crawled at only one level, but analyzed and tested for the presence of map servers. This is especially important for servers with WMS since the keyword we are using to identify a server may not be included anywhere on the website. The situation is less complex for servers with ArcGIS and ArcIMS services since the search keyword is a standard part of the URL for ArcGIS websites, and thus will be found by Google regardless of the other content on the website.
An advantage of our system is that, unlike other crawlers used for discovering web services, the GSE has the ability to locate shapefiles available for downloading. Map services are typically provided in data formats that are not amenable for spatial analysis functions that require data in vector or raster format. While non-spatial engines can also be used to locate websites containing shapefiles, the GSE offers several advantages. First, the user-specified terms are automatically combined with a set of keywords optimized to locate websites that have shapefile data for download. Although the user could type in these same keywords along with their terms if they wanted, the list of keywords and the logical operators between them is complex. Second, after Google returns the results from the above search, the GSE will download and analyze each web page in the results. This process is essentially equivalent to the user clicking on each link in the results and manually scanning the page for its relevance to their search. By automating this process, we are able to provide more refined results than what is returned directly from a Google search. Third, the process of analyzing web pages for relevance is aided by the use of a set of keywords designed specifically to locate web pages containing shapefiles for download. The keyword list is optimized with points for each keyword to help the system reliably locate relevant web pages as the most reliable keywords have the most points. These points are used to rank the web page internally in the GSE database for its likelihood of containing shapefiles for download. Finally, the system also utilizes frequently used terms provided by other user searches. These terms are searched in each web page, and additional weights are added to the rank when these terms are found. Since most GSE users are likely searching for map layers, when there are many other user terms found, this likely indicates we have a site with a list of layers available.
While the GSE provides a suitable means for discovering multiple geospatial data formats on the web, there exists opportunity to improve the ranking algorithm by utilizing existing approaches to crawler development. For example, Li et al. (Citation2010) developed a crawler using a conditional probability model for prioritizing crawling and incorporated an automated procedure for updating metadata of identified services. Such advancements to our crawler would leverage the efficiency and accuracy of our ranking, while providing enhanced knowledge about server metadata. Additionally, including ontology generators such as those employed by Chen et al. (Citation2011) could allow our GSE to facilitate reasoning-based queries.
In future work, we intend to explore how to incorporate OGC catalog interface standards (referred to as Catalog Services for the Web [CSW]) that specify a framework for publishing and accessing metadata for geospatial data in order to leverage the GSE’s potential for increasing the number of services it can locate on the web. These standards rely on metadata as the general properties that search engines can query to evaluate and potentially retrieve services. The GSE is a suitable candidate for adopting CSW as it interrogates resource metadata; however, additional considerations would need to be made for how the CSW framework could handle websites that contain shapefile data. Such resources do not currently conform to CSW query methods, mostly because shapefile metadata are not directly accessible from a website client interface. Other future enhancements include performing an explicit spatial search for data, rather than relying on keywords to narrow the search to specific geographic locations, and broadening search capabilities to include alternative services such as Web Feature Services and data in Keyhole Markup Language format.
5. Conclusion
The main advantage with the GSE is that it strategically incorporates existing methods to provide an efficient engine for searching a multitude of geospatial data formats. Whereas previously developed GSEs do not collectively provide seeding mechanisms to prioritize searches, continually update their catalog of services, or provide sufficient description of the search engine process, the GSE described in this paper accomplishes all these tasks while simultaneously searching for a broader range of geospatial data. Furthermore, the GSE presented here is publically available. It is currently being applied to address a number of issues related to the availability and access to geospatial data within a broader Digital Earth framework, including data quality, spatial distribution of available spatial data across the web, trends in spatial data subject matter, and creation of a global online data catalog with an API to allow further access to the search engine from outside the current user interface. All of these enhancements will enable wider data access that will facilitate a more in depth and informed examination of Earth system processes. While future enhancements lie ahead, our work represents an important step toward realizing the potential of discovering global availability of geospatial data.
References
- Budhathoki, N. R., B. Bruce, and Z. Nedovic-Budic. 2008. “Reconceptualizing The Role of the User of Spatial Data Infrastructure.” Geojournal 72 (3–4): 149–160. doi:10.1007/s10708-008-9189-x.
- Bunzel, K. 2012. Geospatial Search Engine Technical Description. Accessed January 8, 2014. http://www.wwetac.net/docs/GSE%20Technical%20Description.pdf.
- Chen, N., Z. Chen, C. Hu, and L. Di. 2011. “A Capacity Matching and Ontology Reasoning Method for High Precision OGC Web Service Discovery.” International Journal of Digital Earth 4: 449–470. doi:10.1080/17538947.2011.553688.
- Craglia, M. 2007. Volunteered Geographic Information and Spatial Data Infrastructures: When Do Parallel Lines Converge? http://www.ncgia.ucsb.edu/projects/vgi/participants.html.
- De Longueville, B. 2010. “Community-based Geoportals: The Next Generation? Concepts and Methods for the Geospatial Web 2.0.” Computers, Environment and Urban Systems 34: 299–308. doi:10.1016/j.compenvurbsys.2010.04.004.
- Elwood, S. 2008. “Grassroots Groups as Stakeholders in Spatial Data Infrastructures: Challenges and Opportunities for Local Data Development and Sharing.” International Journal of Geographical Information Science 22 (1): 71–90. doi:10.1080/13658810701348971.
- Elwood, S. 2010. “Geographic Information Science: Emerging Research on the Societal Implications of the Geospatial Web.” Progress in Human Geography 34: 349–357. doi:10.1177/0309132509340711.
- Florczyk, A. J., J. Nogueras-Islo, F. J. Zaraaga-Soria, and R. Bejar. 2012. “Identifying Orthoimages in Web Map Services.” Computers and Geosciences 47: 130–142. doi:10.1016/j.cageo.2011.10.017.
- Galaz, V., B. Crona, T. Daw, O. Bodin, M. Nystrom, and P. Olsson. 2010. “Can Web Crawlers Revolutionize Ecological Monitoring?” Frontiers in Ecology and the Environment 8 (2): 99–104. doi:10.1890/070204.
- GeoExt. 2010. GeoExt - JavaScript Toolkit for Rich Web Mapping Applications. http://geoext.org/.
- Goodchild, M. F. 2007. “Citizens as Voluntary Sensors: Spatial Data Infrastructure in the World of Web 2.0.” International Journal of Spatial Data Infrastructures Research 2: 24–32.
- Goodchild, M. F. 2009. “Geographic Information Systems and Science: Today and Tomorrow.” Annals of GIS 15 (1): 3–9. doi:10.1080/19475680903250715.
- Goodchild, M., P. Fu, and P. Rich. 2008. “Sharing Geographic Information: An Assessment of the Geospatial One-Stop.” Annals of the Association of American Geographers 97 (2): 250–266. doi:10.1111/j.1467-8306.2007.00534.x.
- Harvey, F., and D. Tulloch. 2006. “Local-government Data Sharing: Evaluating the Foundations of Spatial Data Infrastructures.” International Journal of Geographical Information Science 20: 743–768. doi:10.1080/13658810600661607.
- Hey, T., S. Tansley, and K. Tolle. 2009. The Fourth Paradigm: Data-intensive Scientific Discovery. Redmond, WA: Microsoft Corporation.
- Li, W., C. Yang, and C. Yang. 2010. “An Active Crawler for Discovering Geospatial Web Services and their Distribution Pattern – A Case Study of OGC Web Map Service.” International Journal of Geographical Information Science 24: 1127–1147. doi:10.1080/13658810903514172.
- Li, Z., C. Yang, H. Wu, W. Li, and L. Miao. 2011. “An Optimized Framework for Seamlessly Integrating OGC Web Services to Support Geospatial Sciences.” International Journal of Geographical Information Science 25: 595–613. doi:10.1080/13658816.2010.484811.
- López-Pellicer, F. J., R. Béjar, and F. J. Soria. 2012a. Providing Semantic Links to the Invisible Geospatial Web. Universidad de Zargoza, Germany.
- López-Pellicer, F. J., W. Rentería-Agualimpia, J. Nogueras-Iso, F. J. Zarazaga-Soria, and P. R. Muro-Medrano. 2012b. “Towards an Active Directory of Geospatial Web Services. In Bridging the Geographic Information Sciences, edited by J. Gensel, D. Josselin, and D. Vandenbroucke, 63–79. Berlin: Springer.
- Maguire, D., and P. Longley. 2005. “The Emergence of Geoportals and their role in Spatial Data Infrastructures.” Computers, Environment and Urban Systems 29 (1): 3–14. doi:10.1016/j.compenvurbsys.2004.05.012.
- Masó, J., X. Pons, and A. Zabala. 2012. “Tuning the Second-generation SDI: Theoretical Aspects and Real Use Cases.” International Journal of Geographical Information Science 26: 983–1014. doi:10.1080/13658816.2011.620570.
- OGC (Open Geospatial Consortium). 2010. Web Service Common. Accessed June 6, 2004. http://www.opengeospatial.org/standards/common.
- Open Layers: Free Maps for the Web. 2010. OpenLayers. Accessed December 11, 2013. http://openlayers.org/.
- Patil, S., S. Bhattacharjee, and S. Ghosh. 2014. “A Spatial Web Crawler for Discovering Geo-servers and Semantic Referencing with Spatial Features.” Distributed Computing and Internet Technology: Lecture Notes in Computer Science 8337: 68–78. doi:10.1007/978-3-319-04483-5_7.
- Sample, J. T., R. Ladner, L. Shulman, E. Ioup, F. Petry, E.,Warner, K. B. Shaw, and F. P. McCreedy. 2006. “Enhancing the US Navy’s GIDB Portal with Web Services.” Internet Computing 10 (5): 53–60. doi:10.1109/MIC.2006.96.
- Schutzberg, A. 2006. “Skylab Mobilesystems Crawls the Web for Web Map Servies.” OGC User 8: 1–3.
- Tait, M. 2005. “Implementing Geoportals: Applications of Distributed GIS.” Computers, Environment and Urban Systems 29 (1): 33–47. doi:10.1016/j.compenvurbsys.2004.05.011.
- Vockner, B., A. Richter, and M. Mittlbock. 2013. “From Geoportals to Geographic Knowledge Portals.” ISPRS International Journal of Geo-Information 2 (2): 256–275. doi:10.3390/ijgi2020256.
- Walter, V., F. Luo, and D. Fritsch. 2013. “Automatic Map Retrieval and Map Interpretation in the Internet.” In Advances in Spatial Data Handling, Advances in Geographic Information Science, edited by S. Timpf and P. Laube, 209–221. Berlin Heidelberg: Springer.
- Wang, S., and Y. Liu. 2009. “TeraGrid GIScience Gateway: Bridging Cyberinfrastructure and GIScience.” International Journal of Geographical Information Science 23: 631–656. doi:10.1080/13658810902754977.
- Whiteside, A., and J. Greewood eds. 2010. OGC Web Services Common Standard. Version 2.0.0., OGC 06-121r9.
- Yang, C., and C. V. Tao. 2006. “Distributed Geospatial Information Service (Distributed GIService).” In Frontiers in Geographic Information Technology, edited by S. Rana and J. Sharma, 103–120. New York: Springer.
- Yang, C., D. Wong, R. Yang, and M. Kafatos. 2005. “Performance-improving Techniques in Web-based GIS.” International Journal of Geographical Information Science 19: 319–342. doi:10.1080/13658810412331280202.
- Yang, C., R. Raskin, M. Goodchild, and M. Gahegan. 2010. “Geospatial Cyberinfrastructure: Past, Present and Future.” Computers, Environment and Urban Systems 34: 264–277. doi:10.1016/j.compenvurbsys.2010.04.001.
- Yang, P., J. Evans, M. Cole, S. Marley, N. Alameh, and M. Bambacus. 2007. “The Emerging Concepts and Applications of the Spatial Web Portal.” Photogrammetric Engineering and Remote Sensing 73: 691–698. doi:10.14358/PERS.73.6.691.
- Zhang, T., and M.-H. Tsou. 2009. “Developing a Grid-enabled Spatial Web Portal for Internet GIServices and Geospatial Cyberinfrastructure.” International Journal of Geographical Information Science 23: 605–630. doi:10.1080/13658810802698571.