Abstract
When a farm that was using a conventional milking system introduces an automatic milking system (AMS) possible risk factors can affect milk quality. The aim of the study was to investigate the influence of milking with automatic milking systems on milk quality variables over a long time-period post-installation. Bulk milk total bacterial count (BMTBC) and somatic cell count (BMSCC) were analysed and compared from 2 years before introduction of automatic milking until 4 years after. Differences regarding these quality parameters were contrasted using t-test and one-way analysis of variance (ANOVA) and post hoc comparisons were performed. A significant increase in BMTBC was observed during the first three months after introduction of AMS, counts then declined to equivalent levels pre-AMS installation, from 25,000 to 50,000 cfu mL−1. Although differences were significant for the first two years post-installation, they became non-significant during the following two years. The difference in BMSCC was not statistically significant between pre and post-AMS installation time periods, but by grouping data into annual periods, significantly higher values of BMSCC were found during the first year after introduction. Nevertheless, these values decreased over time and even showed a significant improvement in the third year with respect to pre-introduction. The data show that the installation of AMS had a marked impact on milk quality. However, as soon as farmers become accustomed to managing the new equipment and the adaption of cows is real, a level of milk quality which can be maintained over time is achievable.
Keywords:
Introduction
With the change from conventional milking to automatic milking there are many factors which can affect milk quality including management, milking routine and mastitis detection. Van der Vorst et al. (Citation2003) analysed in a full study the possible risk factors affecting milk quality on these farms. Therefore, it is normal for there to be a transition period following AMS installation as farmers have to become adept at managing and maintaining the new equipment and cattle have to familiarise themselves with the process of being milked in an AMS unit.
The AMS identifies cows with voluntary attendance and also records whole udder or quarter milk yield and can flag cows that deviate from a predicted yield based on the milking interval. In an AMS, cleaning of the teats before milking is carried out automatically without visual examination by the milker. Successful use of the AMS depends on the cows visiting the robot frequently, but a certain percentage of the cows visit the robot less than twice a day, reducing production and possibly increasing the risk of mastitis (Munksgaard et al. Citation2011). Mollenhorst et al. (Citation2011) showed that the variation in milking intervals correlated positively with somatic cell count (SCC), showing that this variation is more important than the milking interval itself.
At each milking, the cow’s teats are spray cleaned with warm water or cleaned with brushes, then dried, milked, and disinfected. Such actions could have a potentially negative effect on teat condition, increasing teat end callosity and skin damage, nevertheless, some writers have shown that the increased milking frequency did not significantly influence teat end condition in AMS animals (Vliegher et al. Citation2003), in fact, the overall teat condition can improve after changing to an automatic milking system (Neijenhuis et al. Citation2004). More recently, dairy cow hygiene has been linked with SCC in milk and udder health (Dohmen et al. Citation2010; Sant’Anna & Paranhos da Costa Citation2011).
Automatic milking is quarter-based, which prevents the spread of intramammary infection (IMI) between teats of a cow and reduces overmilking. Possible spread of IMI between cows can no longer be prevented by milking order, but can be reduced by flushing or steaming of the liners between individual milkings (Hovinen & Pyörälä Citation2011). Feedback from producers' experience in managing herds with AMS allows manufacturers to modify equipment to improve milk quality. Earlier replacement of liners tended to correspond with a lower BMSCC (de Koning et al. Citation2003), so it could prevent and reduce IMI. On average BMTBC levels were also lower with earlier replacement, but this relationship was not significant.
In an AMS, sensors are required to monitor milk quality and to detect mastitis. Although there is no consensus in literature, it is generally accepted by the majority of researchers that the milk quality on farms with an automatic milking system is significantly poorer than before its introduction (Klungel et al. Citation2000; de Koning et al. Citation2004) as well as udder health (Hovinen et al. Citation2009). Rapid mastitis detection is very important in order to avoid a decrease in milk quality and economic losses (Fröhling et al. Citation2010). A self-monitoring programme can significantly reduce the BMSCC by helping farmers to detect cows with abnormal foremilk at the start of automatic milking and then to work efficiently with the alarm lists (Rasmussen et al. Citation2002), identifying those cows and quarters that are likely to have clinical mastitis. Another study, performed over 25 weeks, concluded that udder health in an AMS is equivalent to conventional parlour milking and in some respects better, as significant differences in SCC were not found and the quarter milk from AMS cows had fewer positive bacterial tests (Berglund et al. Citation2002). Helgren and Reinemann (Citation2006) found no significant differences in BMSCC, although the BMTBC of milk from automatic milking farms was significantly lower than that of conventional farms.
The milk quality of farms that have introduced an AMS is often evaluated from comparing conventional herds with different AMS herds. In contrast to different herds without taking account their milk quality during periods with the other milk system it does not necessarily reflect the true variation in time of milk quality. The levels of milk quality may be studied on a daily basis in bulk milk, hence giving the status of the farm according to the period of time. The differences between before and after AMS introduction for each farm reflects whether herd management with AMS could negatively affect the levels of BMSCC and BMTBC.
On the other hand, previous researches have concentrated mostly on the few months around the introduction of an AMS covering approximately two year. Analysis over a longer period of time of the milk quality may provide additional information knowing that the initial situation of each farm is different, and that there is a transition period when going from conventional to automatic milking.
The overall goal of this study was to examine the effects of transitioning from conventional milking to AMS on milk quality in the long term, through the variability of BMTBC and BMSCC.
Materials and methods
Data collection
The study was performed on milk quality parameters (BMSCC and BMTBC), which are taken into consideration by the milk industry for dairy farmer payments.
Data were collected in 13 farms with 15 AMS for the period between January 2004 and June 2013 from the Galicia Interprofessional Laboratory of Milk analysis (LIGAL) database. This laboratory is a private non-profit organisation dedicated to determining the quality parameters of all the milk produced in Galicia (NW of Spain), to determine the price of the milk. Bulk milk samples were collected and handled in accordance with Spanish official regulations (MPR Citation2008). LIGAL monthly collected a minimum of three samples per farm, milk was homogenised by stirring and a sample of 40 ml of milk was taken and held between 0 and 6° C at the laboratory. Fluoro-opto-electronic with flow cytometry methods was performed on every sample to determine its hygienic quality (BMTBC) and udder health quality (BMSCC).
Milk quality data were analysed from all farms starting 2 years before until 4 years after AMS installation. The AMS have been installed between 2006 and 2009. Before their analysis, all data was checked for unlikely values such as outliers or readings indicating measurement error. The farms included in this study represent approximately 25% of the total population of AM farms in Galicia. Eight farms used Lely Astronaut (Lely, Rotterdam, Netherlands), 4 farms used DeLaval VMS (DeLaval, Tumba, Sweden) and 1 farm used Galaxy (Insentec, Marknesse, Netherlands). This resulted in a total of 5944 valid samples, 1429 from before AMS installation and 4515 from after.
On all farms the cows, of which 99% were Holstein-Friesian, were kept indoors with free access to a total mixed ration and in the AMS gained access to additional feed concentrate. Table shows the average data of the number of cows per AMS, days in milk, parity, percentage of primiparous cows, milkings per cow and day and also annual milk yield per AMS unit from the point of AMS installation.
Table 1. Mean, standard deviation, minimum and maximum values for descriptive variables from 13 herds during transition to use of AMSa.
A new milking was allowed between 240 to 390 min after the previous milking, depending on milk yield, days in milk and the strategy of the farmer. Cows whose milking interval exceeded 720–840 min (depending on the farm) were marked as overdue and were fetched for milking. Cows identified as infrequent milkers based on the lag times between milkings were collected and moved to the AMS twice per day by the farmers following their own criteria.
Depending on the brand of AMS, the milking routines may differ. First the teats were cleaned with a cleaning teat cup (DeLaval VMS and Insentec Galaxy) or with rotating brushes (Lely Astronaut) then milking teat cups were immediately attached. After complete milking of each milk quarter, the teat cups were automatically detached and the teats were sprayed with teat dip. After detachment, each teat cup was rinsed with warm water, water vapour or with diluted peracetic acid.
Statistical analyses
All data were processed using IBM SPSS 18.0.0 for Windows (SPSS Citation2008). Values of milk quality parameters were used without any transformation: BMSCC in cells mL−1; BMTBC in cfu mL−1. Firstly, the possible effect of seasonality on milk quality was analysed using a one-way analysis of variance (ANOVA) mean comparison test, dividing a year in spring, summer, autumn and winter months. Then the differences, regarding these quality parameters, between the two groups (before and after the installation of AMS), were contrasted by a t-test. The period after introduction was divided in shorter periods of time (one year) and once again an ANOVA was performed. ANOVA is an extension of the independent t-test and in the t-test we must take into account that normality and homogeneity of variance are assumed. Homogeneity of variance was tested by the Levene test and normality by examining graphs and the Kolmogorov–Smirnov test. However, since the size of the groups (after vs. before) was so large we may consider that the robustness of the test is maintained even if the normality assumption has not been met (SPSS Citation2008). Because de ANOVA only performs a total analysis of the overall differences, we performed a post hoc comparison. A number of post hoc comparison techniques can be used to test differences between specific groups. We performed a Tukey’s Honestly Significant Difference (HSD) test, but since the assumption of homogeneity of variance was not met (Levene’s test: p <.001), and the number of samples were different in every group, we performed a Games–Howell test to contrast the results. Finally, the frequency of samples in intervals of BMSCC and BMTBC values were checked with the Chi squared test to determine the differences between the time periods. Intervals were determined with respect to the official limits (MPR Citation2008) taken into account as a reference for setting milk prices for Spanish farmers (for BMTBC; <50 × 103 bonus price; 50–100 × 103 base price; >100 × 103 penalised price; and for BMSCC; <200 × 103 bonus price; 200–400 × 103 base price; >400 × 103 penalised price).
Results
It was observed that throughout the whole period of time studied, the mean values of Summer months were greater (p = .000) for BMSCC (272–287 × 103 cells mL−1) and BMTBC (41–48 × 103 cfu mL−1) than the Winter months (233–246 × 103 cells mL−1 and 30–32 × 103 cfu mL−). BMTBC was greater (p = .001) after AMS installation whereas, regarding BMSCC, differences were not significant (p = .275). Although it was slightly lower after AMS (Table ). Individual farm averages ranged from 14,230 to 49,880 cfu mL−1 and from 140,450 to 403,680 cells mL−1 before AMS installation. After AMS installation the averages ranged from 24,850 to 66,710 cfu mL−1 and from 157,720 to 376,570 cells mL−1.
Table 2. Statistics of bulk milk somatic cell count (BMSCC) and bulk milk total bacterial count (BMTBC) obtained from bulk milk from before and after the introduction of an automatic milking system.
Data samples from the introduction of AMS can be divided into shorter periods in order to detect an adaptation period. If we evaluate the results graphically, BMTBC was higher for approximately three months following AMS installation before falling to levels equivalent to pre-AMS installation (Figure ). BMSCC variation is not so clear (Figure ). ANOVA indicates whether the mean values for the five different time periods (years) differ significantly (p = .001), but it does not indicate the location, or rather, the year in which this difference is observed. Differences or likeness between specific years are shown in Table for BMTBC mean values and Table for BMSCC mean values. The mean values for BMTBC and BMSCC were increased for the first year after AMS installation. BMTBC for the second year continued being significantly higher than the pre-installation period, but the mean values for the third and fourth year were not significantly different, forming a homogeneous subset with the pre-installation period (p = .411). BMSCC for the second and fourth year after installation form a homogeneous subset with the pre-installation period because there were not significant differences between these three periods. In the third year after AMS installation BMSCC was significantly lower than in the situation before (Table ).
Figure 1. Monthly average BMTBC from 13 dairy farms between 24 months before AMS installation and 48 months after.
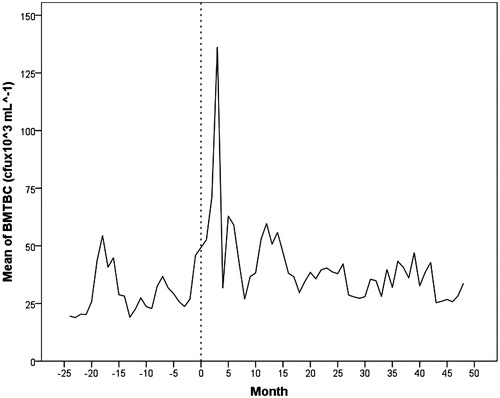
Figure 2. Monthly average BMSCC from 13 dairy farms between 24 months before AMS installation and 48 months after.
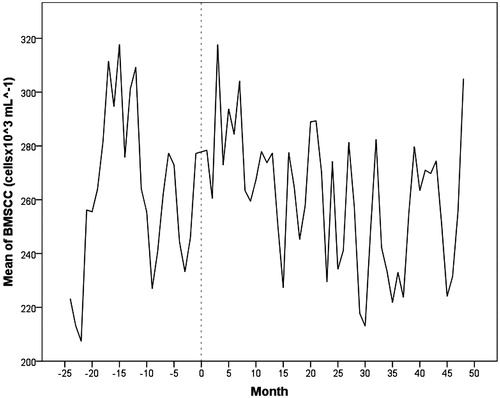
Table 3. Bulk milk total bacterial count (BMTBC) averages for homogeneous subsets based on the time periods studied with significant differences.
Table 4. Bulk milk somatic cell count (BMSCC) averages for homogeneous subsets based on the time periods studied with significant differences.
There were no differences in BMSCC when analysed in intervals which are taken into account as a reference for setting milk prices (p = .172), nevertheless, in the BMTBC intervals, all differences (overall and in groups), were significant (Table ).
Table 5. Number and percentage of samples before versus after the introduction of an automatic milking system by intervals of BMSCC and BMTBC values.
Discussion
From a general overview, our results agree with other researchers in that there is evidence of seasonal variation in the quality of milk. The most liable period with the highest values for both BMTBC and BMSCC, were the summer months, as also observed by Helgren and Reinemann (Citation2006) in United States. Another study using 25 herds in Spain showed the distribution of clinical mastitis cases was 32, 26, 22 and 20% for winter, summer, spring and autumn seasons, respectively (Pérez-Cabal et al. Citation2008). Summer and winter are seasons preceded by spring and autumn respectively in which the fieldwork increases due to forage planting and harvesting. For this reason, mastitis cases may not have been as accurately recorded leading to the seasonal variations presented above.
Regardless of the time of year, if we were to only compare the time period before installation of the AMS with the time period after, we would say that the introduction of an AMS resulted in an increase in the BMTBC and overall unchanged levels of BMSCC, coinciding with what was shown before by Klungel et al. (Citation2000). Bacterial contamination can originate from multiple sources, such as mastitis in cows, dirty teats and udders, and poorly cleaned milking equipment (de Koning et al. Citation2003; van der Vorst et al. Citation2003). Equally important is the milk holding temperature and the duration of milk processing since increased bacterial growth can occur in the bulk tank (de Koning et al. Citation2003; Helgren & Reinemann Citation2006). However, a major reason for the large increase in BMTBC during the first three months after installation (Figure ) could be unhygienic milk harvesting practices due to the animals’ lack of hygiene and co-operation. Wenzel et al. (Citation2003) indicated that cows in the automatic milking system showed higher step-frequency during milking than cows in the milking parlour. Besides, during the transition period cows have to adapt to the new system, they are more restless and kicking is very common making it difficult to maintain the milk harvesting process sufficiently hygienic. Jacobs and Siegford (Citation2012) concluded as ‘puzzling’ the increase, after two weeks of milking in AMS, in the number of steps and kicks following teat attachment during the milking process. Cow behaviour such as steps with swinging, kicking or lifting have a serious negative impact on the automatic milking process as they cause 55% of failures in the milking process, of which 28% were failures during washing (Kaihilahti et al. Citation2007). Poor hygiene in those parts of the robot which come into contact with the udder and in the waiting area can also increase the chances of bacterial contamination (van der Vorst et al. Citation2003). Moreover, the system is in use 24 h per day which results in different machine cleaning requirements. Over time it seems that the risk factors can be partly controlled by management actions taken by the farmer (Helgren & Reinemann Citation2006) and differences become non-significant (Table ), so mostly management factors (like hygiene in the clean part of the AMS and the waiting area) are responsible for a sudden increase in BMTBC (de Koning et al. Citation2004). All factors should be controlled because they are complementary. For example, good bedding practices are important but of little use if udder hair is not kept short. Long udder hair has been related to unsuccessful teat cleaning in AMS since an excessive amount of clean, dry bedding material was attached to the long udder hair (Hovinen et al. Citation2005). However, BMTBC levels remain slightly higher than pre-installation levels and although these differences are small and well within acceptable milk quality, additional care is needed to maintain the milk quality in AMS.
As indicated, a generalised observation of average BMSCC values between pre and post installation time periods didn’t show significant differences. This is consistent with data reported from 33 German herds (van der Vorst et al. Citation2002), however, this study also showed that BMSCC increased following AMS installation on farms in Denmark and Holland. The average BMSCC was higher during the first year after introduction of AMS and decreased over time, even becoming significantly lower (Table ). An adaptation period of a few months was also seen by previous research and, although SCC decreased after the first year, it continued to be higher than before the installation (Rasmussen et al. Citation2002; Hovinen et al. Citation2009). However, these studies focussed on short-term data, whereas our research includes data from 4 years following AMS installation. BMSCC is related to the number of high SCC cows or the occurrence of mastitis. Due to the multifactorial nature of udder health, BMSCC may be affected by a wide range of factors: dairy cow hygiene, overmilking, possible spread of IMI between cows, mastitis detection (van der Vorst et al. Citation2003; Neijenhuis et al. Citation2004; Sant’Anna & Paranhos da Costa Citation2011; Mollenhorst et al. Citation2011), among others. Further aspects must be considered to determine why the variations in BMSCC were not significant. For instance, the effect of culling strategies applied when the AMS is installed due to teat shape or placement or willingness to come to the AMS (Østergaard et al. Citation2002) and the replacement of these cows with younger animals which would typically have lower SCC. Nonetheless, in our study only 4 of the 13 farms had to cull some of the cows (a total of 7 cows) when transitioning to an AMS and the percentage of primiparous cows (Table ) was similar to that shown in Official Milk Records of Galician dairy farms (Africor Lugo Citation2013).
On the other hand, in routine conversations with the farmers during the study some common drying strategies were discussed. On some farms when the AMS was installed cows that were near the end of their lactation period were dried to decrease the size of the herd because this reduced the farm’s workload during the adaptation period. This drying period could also be used to treat cows with persistent mastitis problems in order to improve udder health at calving (in terms of SCC). These actions can be considered as part of a complete programme for mastitis control. These programmes can be based on supplementary requirements for AMS established to help farmers in the transition period going from conventional to automatic milking (Rasmussen et al. Citation2002).
These results regarding the evidence of a decrease in BMSCC in automatic milking farms agree with those reported in Helgren and Reinemann (Citation2006), which could occur from the eighth month after installation. These authors confirm the importance of an increased knowledge in AMS milking management since the overall frequency of milk-quality failures is reduced as the producers and farm employees become more experienced.
It is also likely that this point coincides with the adaptation of the cows to the AMS; adaptation of the cows to the AMS can take on average 188.4 days according to 38 Spanish dairy farms (Castro et al. Citation2015). Changes in management especially in the early post-installation period would be stressful for cattle used to a regular routine. Burnett et al. (Citation2014) showed that acute stress responses in dairy cows due to management practices can influence SCC.
The lower SCC in the AMS periods, once the adaptation period had passed, could probably also be a consequence of the more frequent milkings in AMS, since an increased milking frequency has generally been associated with less mastitis. Milking three times a day was shown to result in decreased cow SCC compared with twice-a-day milking (Hovinen & Pyörälä Citation2011), but Köhn et al. (Citation2007) reported a slightly negative correlation between cow SCC and milking frequency in 10 farms with AMS.
It has been noted that levels of SCC stabilise to similar averages to those of conventional farms due to improvement of mastitis detection systems and because of the use of quarter milking with on-line measurements (de Koning et al. Citation2004). Milk from clinically infected cows and cows with high SCC is diverted to a similar degree in automatic milking and in conventional milking.
Furthermore, if effective preventive measures to maintain correct BMSCC levels are used without AMS, the same performance could be expected in the new situation. Therefore, it is possible for farmers who experienced milk quality problems to work with milk quality consultants to develop a mechanism to reduce mastitis and improve milk quality. BMSCC values can respond rapidly to interventions (Rodrigues et al. Citation2005), such as using milk recording data to identify problem cows, allowing the milk with poor quality to be removed in time so as not to affect milk payments. But at this time, little is known about practices associated with SCC in AMS herds; dairy producers who were more aggressive regarding maintenance and cleaning of the AMS seemed to be able to mitigate the elevated SCC (Dufour et al. Citation2011).
Conclusions
Milk quality was affected after introduction of an AMS in terms of BMTBC. However, when data was analysed for shorter periods of time, an adjustment period during the first year post-installation was observed where BMSCC were at their highest too. But when analysis of AMS operation increased the data revealed evidence of a decrease in BMTBC and BMSCC almost back to levels from before AMS. Our reasons for this are not conclusive but possibly, when farmers gain experience with the new situation, using suitable hygiene and cleanliness protocols, the adaptation in cows reduces their stress levels, allowing them to manage and control factors which impact milk quality. This suggests that greater support, information and training for the farmer should be given during this transition period by the distributors’ technical staff. On the other hand, farmers should work with consultants to develop specific programmes for milk quality control in dairy farms with automatic milking systems.
Acknowledgements
The authors are grateful to the dairy farmers who facilitated access to their data and the Galicia Interprofessional Laboratory of Milk analysis.
Disclosure statement
No potential conflict of interest was reported by the authors.
References
- Africor Lugo. 2013. Memoria 2013. [Internet]. [cited 2014 Sep 1]. Available from: http://www.africorlugo.com
- Berglund I, Pettersson G, Svennersten-Sjaunja K. 2002. Automatic milking: effects on somatic cell count and teat end-quality. Livest Prod Sci. 78:115–124.
- Burnett TA, Madureira AML, Silper BF, Nadalin A, Tahmasbi A, Veira DM, Cerri RLA. 2014. Short communication: factors affecting hair cortisol concentrations in lactating dairy cows. J. Dairy Sci. 97:7685–7690.
- Castro A, Pereira JM, Amiama C, Bueno J. 2015. Typologies of dairy farms with automatic milking system in northwest Spain and farmers’ satisfaction. Ital J Anim Sci. 14:207–219.
- de Koning K, Slaghuis B, van der Vorst Y. 2003. Robotic milking and milk quality: effects on bacterial count, somatic cell count, freezing point and free fatty acids. Ital J Anim Sci. 2:291–299.
- de Koning K, Slaghuis B, van der Vorst Y. 2004. Milk quality on farms with an automatic milking system. In: Meijering A, Hogeveen H, de Koning K, editors. Automatic milking; a better understanding. Wageningen, The Netherlands: Wageningen Academic Publishers; p. 311–320.
- Dohmen W, Neijenhuis F, Hogeveen H. 2010. Relationship between udder health and hygiene on farms with an automatic milking system. J Dairy Sci. 93:4019–4033.
- Dufour S, Fréchette A, Barkema HW, Mussell A, Scholl DT. 2011. Invited review: effect of udder health management practices on herd somatic cell count. J Dairy Sci. 94:563–579.
- Fröhling A, Wienke M, Meierhöfer SR, Schlüter O. 2010. Improved method for mastitis detection and evaluation of disinfectant efficiency during milking process. Food Bioprocess Technol. 3:892–900.
- Helgren JM, Reinemann DJ. 2006. Survey of milk quality on dairy farms utilizing automatic milking systems. Trans ASABE. 49:551–556.
- Hovinen M, Aisla AM, Pyörälä S. 2005. Visual detection of technical success and effectiveness of teat cleaning in two automatic milking systems. J Dairy Sci. 88:3354–3362.
- Hovinen M, Pyörälä S. 2011. Invited review: udder health of dairy cows in automatic milking. J Dairy Sci. 94:547–562.
- Hovinen M, Rasmussen MD, Pyörälä S. 2009. Udder health of cows changing from tie stalls or free stalls with conventional milking to free stalls with either conventional or automatic milking. J Dairy Sci. 92:3696–3703.
- Jacobs JA, Siegford JM. 2012. Lactating dairy cows adapt quickly to being milked by an automatic milking system. J Dairy Sci. 95:1575–1584.
- Kaihilahti J, Suokannas A, Raussi S. 2007. Observation of cow behavior in an automatic milking system using web-based video recording technology. Bios Engin. 96:91–97.
- Klungel GH, Slaghuis BA, Hogeveen H. 2000. The effect of the introduction of automatic milking systems on milk quality. J Dairy Sci. 83:1998–2003.
- Köhn F, König S, Gauly M. 2007. Influence of milk production traits and genetic effects on milking frequency in automatic milking system. Zuchtungskunde. 79:287–297.
- Ministerio de la Presidencia (MPR). 2008. REAL DECRETO 1728/2007, de 21 de diciembre, por el que se establece la normativa básica de control que deben cumplir los operadores del sector lácteo [Internet]; [cited 2014 Sep 4]. Available from: http://www.boe.es/boe/dias/2008/01/17/pdfs/A03508-03519.pdf
- Mollenhorst H, Hidayat MH, van den Broek J, Neijenhuis F, Hogeveen H. 2011. The relationship between milking interval and somatic cell count in automatic milking systems. J Dairy Sci. 94:4531–4537.
- Munksgaard L, Rushen J, de Passillé AM, Krohn CC. 2011. Forced versus free traffic in an automated milking system. Livest Sci. 138:244–250.
- Neijenhuis F, Bos K, Sampimon OC, Poelarends J, Hillerton JE, Fossing C, Dearing J. 2004. Changes en teat condition in Dutch herds converting from conventional to automated milking. In: Meijering A, Hogeveen H, de Koning K, editors. Automatic milking; a better understanding. Wageningen, The Netherlands: Wageningen Academic Publishers; p. 141–147.
- Østergaard S, Sørensen JT, Hindhede J. 2002. Culling strategies in herds with automatic milking systems analysed by stochastic simulation. First North American Conference on robotic milking, Toronto, Canada.
- Pérez-Cabal MA, Yaici S, Alenda R. 2008. Clinical mastitis in Spanish dairy cows: incidence and costs. Span. J Agric Res. 6:615–622.
- Rasmussen MD, Bjerring M, Justesen P, Jepsen L. 2002. Milk quality on Danish farms with automatic milking systems. J Dairy Sci. 85:2869–2878.
- Rodrigues ACO, Caraviello DZ, Ruegg PL. 2005. Management of Wisconsin dairy herds enrolled in milk quality teams. J Dairy Sci. 88:2660–2671.
- Sant’Anna AC, Paranhos da Costa MJR. 2011. The relationship between dairy cow hygiene and somatic cell count in milk. J Dairy Sci. 94:3835–3844.
- SPSS. 2008. SPSS for Windows Release 17.0.0. Somer (NY): IBM Corporation.
- van der Vorst Y, Knappstein K, Rasmussen MD. 2002. Milk quality on farm with an automatic milking system. Effects of Automatic Milking on the Quality of Produced Milk [Internet]; [cited 2012 Jul 5]. Available from: http://www.automaticmilking.nl
- van der Vorst Y, Bos K, Ouweltjes W, Poelarends J. 2003. Milk quality on farm with an automatic milking system. Farm and Management Factors Affecting Milk Quality [Internet]; [cited 2012 Jul 5]. Available from: http://www.automaticmilking.nl
- Vliegher S, Laevens H, Barkema HW, Opsomer G, Hemling T, de Kruif A. 2003. Short-term effect of transition from conventional to automated milking on teat skin and teat end condition. J Dairy Sci. 86:1646–1652.
- Wenzel C, Schönreiter-Fischer S, Unshelm J. 2003. Studies on step–kick behavior and stress of cows during milking in an automatic milking system. Livest Product Sci. 83:237–246.