Abstract
The implementation of an animal welfare assurance programme for dairy cattle in South Tyrol (Eastern Italian Alps) faces particular feasibility constraints due to the outstanding volume of travel associated with routine on-farm audits of remote mountain farms. Therefore, this study aims to estimate the inter-rater reliability of the expert’s and farmers’ welfare outcome assessment regarding recommendations to involve milk producers in animal welfare assurance within South Tyrolean dairy farming. A formal training programme containing a classroom session and an on-farm observation became mandatory for all 188 participating farmers, which was offered by the expert, applied as reference standard. On-farm data collected on the farmers’ cows (data set of 1719 dairy cows) were compared at animal level. Cohen’s kappa, respectively, weighted kappa, examined for several welfare indicators, range from slight to moderate agreement (κ = 0.018 − 0.416; κω = 0.163 − 0.310). These findings are further confirmed by results at farm level (ICC = 0.018 − 0.577). Continuous repeatability checks as part of routine audits are therefore proposed to substantially reduce the variability between the raters and to avoid significant bias in the welfare outcome assessment. In this way, the competence for regular and standardised monitoring could be increasingly transferred to dairy farmers in order to reduce the need for costly and time-consuming inspections by external auditors, which are in long-term perspective also harmful to the alpine environment. Additionally, the promotion of welfare assessment as an instructive management tool would intensify farmers’ commitment to the assessment process.
Farmers’ self-assessment of welfare outcomes is cost-effective and eco-friendly, but reliability must be ensured.
Inter-rater reliability of welfare outcome assessment by an expert and farmers presented a slight to moderate level.
Repeatability assessment at regular intervals is proposed to reduce data variability and, thus, prevent bias in the welfare outcome assessment.
Highlights
Introduction
Recently, the image of dairy farming is under threat (Weary and von Keyserlingk Citation2017). The social acceptance of livestock production is closely linked to the fulfilment of animal welfare-friendliness on both consumer and trade sides (EFSA Citation2015); therefore, milk producers are required to meet an increasing number of animal welfare standards (Rushen et al. Citation2011). In this context, animal welfare assurance schemes are becoming more popular in order to address the growing public concerns by creating transparent information and evidence about the welfare credentials in food production (de Vries et al. Citation2014). Such programmes aim to reflect an objective and accurate picture of animal welfare underpinned by regular, standardised on-farm assessment (van Os et al. Citation2018) and, thus, play an essential role in confirming and continuing to strengthen and improve animal welfare (van Dijk et al. Citation2018). A survey on animal welfare in dairy cattle farms in South Tyrol (Eastern Italian Alps) highlighted some important welfare problems mainly related to the provision of resources and the prevalence of integument alterations especially in tie-stalls (Katzenberger et al. Citation2020). In response to these findings, Katzenberger et al. (Citation2020) emphasised the urgent need for the establishment of an animal welfare assurance programme. Farm compliance with welfare requirements in the mountainous area of South Tyrol is an indispensable prerequisite for future maintenance of traditional livestock farming. Livestock farming is one of the fundamental pillars supporting the preservation of the heterogeneous landscape, contributing to the sustainability of agro-biodiversity (Battaglini et al. Citation2014), while generating income for local communities.
However, the implementation of on-farm assessment faces feasibility constraints. Farm audits that are ordinarily conducted by third-party independent inspectors require a large number of assessors (van Os et al. Citation2018) and pose challenges in assessing behaviour-related indicators in a comprehensive as well as time-efficient way (Knierim and Winckler Citation2009). Behavioural measures have to be assessed independently from time because some may require a long wait to be observed (e.g. getting up behaviour since the animal has to lie down first). Thus, certification visits are time-consuming and expensive (de Vries et al. Citation2014; van Os et al. Citation2018). In the Alpine region, however, costs arise not only from the required service but also from the outstanding volume of travel in mountainous terrain caused by the limited development of infrastructure, compared with the plain (Bätzing Citation2015). This problem is exacerbated by the fact that mountain farms are mostly decentralised and settled in geographically and topographically isolated districts. Given these circumstances, it was suggested to transfer the competence for regular and standardised welfare assessment to dairy farmers in order to reduce the need for routine farm inspections by external auditors. From both an economic and an ecological point of view, costs as well as environmental emissions associated with continuous field trips to all 4509 milk suppliers of the South Tyrolean dairy sector (Sennereiverband Südtirol Citation2020) would be saved. Furthermore, the promotion of welfare assessment as an instructive management tool would be beneficial to raise the awareness among livestock keepers to identify existing weaknesses and, thus, intensify farmers’ commitment to the welfare monitoring. Notwithstanding this, self-assessment of animal welfare by farmers has already been adopted in Germany by the Animal Welfare Act from 2014 (paragraph 11(8); Animal Welfare Act Citation2006) and emphasised by the report of the Scientific Advisory Board on Agricultural Policy, Food and Consumer Health Protection of the Federal Ministry of Food and Agriculture (WBABMEL Citation2015).
The increased public concern on farm animal welfare has resulted in the development of several instruments to measure dairy cattle welfare on farms. These protocols rely on different indicators. Resource-based indicators are related to the physical environment and resources available to the cows (e.g. water provision), while management-based indicators concern the conduction of the farm (e.g. disbudding/dehorning). However, these indicators can only provide indirect welfare measures, since they are not able to give information on how the animals are coping with their environment. More recently, assessment tools have therefore shifted their emphasis from resource and management indicators to animal-based indicators dealing with health (e.g. integument alterations) and behaviour (e.g. getting up behaviour). Cow-related indicators represent direct measures of dairy cattle welfare as they are more closely linked to the animal’s well-being and, thus, allow the assessment of variations in the environmental input (EFSA Citation2012). For instance, the Welfare Quality protocol for dairy cattle (WQ; Welfare Quality Citation2009) focuses on animal-based indicators, most of which have already been evaluated with regards to validity, reliability, and feasibility (e.g. Knierim and Winckler Citation2009).
For these reasons, an outcome-based approach in animal welfare assurance is now preferred (EFSA Citation2012). Due to the high risk of subjectivity during data collection of animal-related indicators (Schenkenfelder and Winckler Citation2017), however, good inter-rater agreement is paramount (Gibbons et al. Citation2012). Therefore, the objective of the study is to estimate the inter-rater reliability of welfare outcome assessment by an expert and farmers regarding recommendations to involve milk producers in animal welfare assurance within South Tyrolean dairy farming. Observer variability assessment was thereby applied as a part of quality control (Popović and Thomas Citation2017) to check for a lack of credence in truthfulness of the farmers’ data reported.
Materials and methods
Recruitment of farmers
A one-page factsheet was sent out to all milk producers by the South Tyrolean dairy plants. In addition, a brief notice was issued to advertise the project at the 12th annual agricultural conference Südtiroler Berglandwirtschaftstagung in January 2019. As the farmers’ active involvement was required (i.e. assessment of indicators), farmers had first to express their interest in participating. To this end, those who have been interested in participating had to register directly with their responsible local dairy representative.
In total, 188 mountain farmers (87 tie-stalls with a herd size of (mean ± SD) 14.2 ± 7.5 dairy cows; 101 loose housings with a herd size of 23.9 ± 17.0 dairy cows) located in the neighbouring regions South Tyrol (Italian Alps, North-Eastern Italy; Autonomous Province of Bolzano) and North Tyrol (Austrian Alps, Western Austria, Tyrol) participated in the study. North Tyrolean farmers (24 farmers) were included as well, because they are employed with the South Tyrolean dairy plant in Vipiteno, as the milk produced in Austria is processed and refined across borders and finally labelled with provenance of South Tyrol.
Development of protocol
In order to meet the specific operative conditions regarding welfare assessment on small-scale farms (EFSA Citation2015) and data collection by farmers, a robust protocol for application in an animal welfare assurance programme was developed and elaborated based on previous fieldwork. Three different recording methods were tested by 15 dairy farmers and the expert during pilot visits in South Tyrol in 2018 with regard to the feasibility of on-farm application and the likelihood of a willing implementation by agricultural producers. As the time investment necessary to complete the assessment is a crucial factor for the acceptance and success of welfare protocols (Vasseur et al. Citation2015), it was first defined as a key objective that the evaluation can be holistically performed within a time frame of two hours. Secondly, it was a desire that farmers would consider this tool beneficial in encouraging improvements in dairy cattle welfare by detection of improvable health and welfare areas. Moreover, data collection was aimed to be performed in the same way by multiple observers to guarantee a highly reliable measurement. Once the targets were established, several animal-based indicators that are explicitly recommended for dairy farmer’s self-assessment by the German association Kuratorium für Technik und Bauwesen in der Landwirtschaft e. V. (KTBL; Brinkmann et al. Citation2016) were defined. Van Dijk et al. (Citation2018) acknowledged that the principle of endeavouring to monitor an agricultural operation based on health and behavioural observations of animals rather than relying upon the assessment of resources and management practices was well received by farmers. In addition, two resource-based criteria were selected to be able to estimate the impact of such environmental inputs on the animals themselves and to provide insights for any improvements to be made. Finally, measures included in the pilot phase were the same as in the final protocol. However, present analyses focused exclusively on the cow-related indicators that had been assessed (Table ).
Table 1. Animal-based indicators used to determine inter-rater reliability.
Training programme
A training programme, including a classroom session and an on-farm session, was mandatory for all participants, since there is a great emphasis on the importance of training for welfare observers to reduce inter-rater variation of animal-based measures and to maintain the integrity of the assessment (Rushen et al. Citation2011; EFSA Citation2012). Differences in welfare assessment had to be expected due to observer-related influences such as education, experience and personal biases.
Trainer
The trainer was a veterinarian with extensive experience in welfare assessment on commercial dairy cattle farms. She was responsible for the elaboration of the protocol, the design of the training materials and the training itself, i.e. the classroom sessions and the continued welfare outcome assessment on all farms. In doing so, the trainer set the reference standard against which each farmer was evaluated throughout the on-farm observation. This was consistent with previous studies, e.g. in assessing pig welfare (Mullan et al. Citation2011), where the trainer was also used as the reference point. Due to the trainer’s education, intra-observer reliability testing was waived. If repeatability checks are carried out at short intervals, there is a high risk of recognising individual animals. If a long interval is chosen instead, findings may have changed in the meantime.
Classroom session
The basic knowledge required for welfare assessment was conveyed to all farmers using a PowerPoint presentation accompanied by photographs and video clips in identical 2-h classroom sessions, which were offered at 12 locations throughout South and North Tyrol in February 2019. On this occasion, the protocol was given to the participants in addition to a take-home reminder containing a clear definition of the scores along with representative photographs, and a detailed description of the recording procedure both put on reference cards for each indicator.
On-farm session
A sample of 10 randomly selected dairy cows including lactating as well as dry cows was assessed to balance accuracy and feasibility for the number of animals to be scored. Animals were selected by the farmer, unless the sample had already been drawn by the expert’s previous template. In detail, the selection was made in tie-stalls by choosing every second animal, whereas in loose housings the animals had to be fixed in the feeding fence first before being selected in the same way (Brinkmann et al. Citation2016). If herd size was equal to or less than 10 dairy cows, all animals were considered accordingly.
Animal-based indicators (Table ) were assessed individually for each cow, identified by ear tag number, based on visual examination at a maximum distance of two metres (Brinkmann et al. Citation2016). BCS was scored from behind on appearance of the lumbar region of the vertebral column (spinous processes and transverse processes), tuber coxae (hip or hook bones), tuber ischii (pin bones) and the cavity around the tail head (Brinkmann et al. Citation2016). All factors considered together provided a score based on a five-point system proposed by Wildman et al. (Citation1982). Avoidance distance was estimated as the distance between the assessor’s hand and the muzzle of the cow when the observed animal showed the first withdrawal. To this end, the cow was approached from the front by the observer, who held the arm outstretched at an angle of about 45° in front of the body and slowly walked towards the animal at a speed of one step per second and a step length of approximately 60 centimetres (Brinkmann et al. Citation2016). When cows were tied-up head-to-wall, avoidance behaviour was similarly estimated by standing next to the cow's head and moving the outstretched arm towards her muzzle (non-validated test). Further, the presence of skin alterations with a minimum diameter of two centimetres at the largest extent (Brinkmann et al. Citation2016) was monitored, distinguishing between hair loss, swelling and lesion. Dirtiness was assessed based on the presence of separate or continuous plaques of dirt amounting to at least the size of the palm of the hand per region observed (Brinkmann et al. Citation2016). Moreover, claw conformation covering the presence of overgrown claws and other disorders, e.g. ulcers or digital dermatitis, was noted. According to the specifications of the KTBL, skin alterations, dirtiness and claw conformation were examined from one side of the body only, in the present case always from the right side (Brinkmann et al. Citation2016). In tie-stalls, lameness was recorded from behind, whereby the front feet were viewed as best as possible. Following the recommendations of Leach et al. (Citation2009) and Welfare Quality (Citation2009) for assessing lameness in cows confined in tie-stalls, the animal was first observed while standing undisturbed. Thereby, lameness was scored on appearance of repeated shifting of weight from one foot to another, rotation of feet from the line parallel to the midline of the body, standing on the edge of a step and resting a foot (one foot more than another). Then the cow was encouraged to move to the left and to the right (applying hand pressure to the hindquarter if necessary). When moving from side to side, uneven weight bearing between feet, demonstrated by more rapid movement by one foot to relieve another or reluctance to bear weight on one foot, as well as the position the cow returned to after movement were considered. In free stalls, the same criteria were applied to assess lameness while standing, whereas the cow’s step length, head bob and arched back were recorded from the side and from behind during gait scoring in the corridors (Brinkmann et al. Citation2016). All factors considered while standing and moving resulted in two separate scores each based on a three-point scale described by Brinkmann et al. (Citation2016). To observe getting up behaviour, the animal was motivated to stand up by addressing or slightly touching the hindquarter (Brinkmann et al. Citation2016). In general, loose housed cows were headlocked at the feed bunk during the assessment and only released for examination of lameness (when moving) and getting up behaviour.
There was no specification on the exact time of the assessment within daily routine (e.g. before milking or after feeding). Farmers were only instructed to carry out the observation once between March and April 2019 to minimise and standardise the time gap between classroom session and on-farm session. However, the majority of farmers disregarded the predefined time window and, despite reminders (via email), continued to further postpone the assessment. As a result, on-farm observation was ultimately performed between February and August 2019.
The expert exercised the assessment in the same way during the overlapping time frame from March to October 2019. Data collected enabled some comparison of indicators used to determine inter-rater reliability. In total, the data set comprises 1719 dairy cows (759 cows in tie-stalls; 960 cows in free stalls). Only those animals that had been assessed by both expert and farmer were included. If one of the coders failed the measurement, e.g. if an animal was out to mountain ranges at the time of the expert’s farm visit, was sold or died during the time interval between the farmer's and expert’s assessment, data were not considered. This time interval averaged 70 days (69.6 ± 56.5 days) due to the large number of time-consuming field trips in North and South Tyrol, all of which were executed by the same expert.
Statistical analysis
A combination of coefficients that are advised in literature for reliability assessment was chosen to make it easier to cross-reference with previous studies. Analyses were done using IBM SPSS Statistics 26, except for confidence intervals, which were performed using BiAS. for windows 11.10. Significant levels were consistently related to p < 0.05. Missing values were generally not addressed. If one of the coders failed to report a specific indicator in the assessment of a cow, data comparison at animal and farm level was excluded.
Reliability assessment at animal level
Cohen’s kappa (κ) and weighted kappa (κω) statistics indicate the extent to which the proportion of agreement between expert and farmer is better than chance. While Cohen’s kappa treats differences between observers equally, Cohen’s weighted kappa is adapted in the way that large differences between the assessors are treated as more significant than smaller ones. For this reason, coefficients were calculated as follows:
Dichotomous variables: Reliability of dichotomous measures was quantified by Cohen’s kappa.
Polytomous variables:
Reliability of polytomous variables was also calculated by Cohen’s kappa after the measures had been collapsed to form dichotomous variables (normal versus all other categories).
In addition, reliability of the multi-category nominal variable (getting up behaviour) was calculated by Cohen’s kappa, while reliability of multi-category ordinal measures was quantified by Cohen’s weighted kappa.
The interpretation of coefficients was < 0.0 = poor, 0.0 to 0.20 = slight, 0.21 to 0.40 = fair, 0.41 to 0.60 = moderate, 0.61 to 0.80 = substantial, and 0.81 to 1.00 = almost perfect according to Landis and Koch (Citation1977). The chance level of agreement between expert and farmer depends on the relative prevalence of each classification in the sample population. The probability of agreement by chance increases in a more homogenous sample (Burn and Weir Citation2011). Accordingly, the relative prevalence of cows affected was determined for each indicator when considered as a dichotomous variable. In addition, McNemar-chi-squared-test was performed for dichotomous scales and otherwise McNemar-Bowker-test in order to check for significant differences between the raters.
Reliability assessment at farm level
The Intraclass correlation coefficient (ICC; two-way mixed-effects model, absolute-agreement, single-measurement) that reflects both the degree of correlation and the agreement between measurements was quantified. Its interpretation was 0.0 to 0.30 (0.0 to −0.30) = negligible, 0.30 to 0.50 (−0.30 to −0.50) = low, 0.50 to 0.70 (−0.50 to −0.70) = moderate, 0.70 to 0.90 (−0.70 to −0.90) = high, and 0.90 to 1.00 (−0.90 to −1.00) = very high (Hinkle et al. Citation2003). To help understand the level of reliability, the farm-level prevalence (mean %) of animals affected as well as the relative difference (mean %) between the expert’s and farmer’s assessment were calculated for each indicator when considered as a dichotomous variable.
Results and discussion
Reliability assessment at animal level
BCS
Inter-rater reliability of the assessment of BCS was fair when, in the interest of better comparability with all other indicators, the multi-category ordinal scale was collapsed to form a dichotomous variable (). When considering the scale of five categories, Cohen’s weighted kappa consistently indicated fair reliability (κω = 0.310 [0.260 − 0.359]; p < 0.001). In comparison, data published by Vasseur et al. (Citation2013) for the first live observation showed moderate inter-rater reliability of BCS scored on a 14-point chart. The more the BCS of an animal deviated from normal in the opinion of the expert, the more frequently the farmers disagreed (). Qualitative analyses of farmers’ assessment against the reference standard showed that there was a trend among participants to score their cows towards normal body condition (), possibly due to operational blindness that has developed over the years in daily routine work.
Figure 1. Occurrence of farmer’s disagreement and agreement presented in each BCS score reported by the experta. aDisagreement and agreement between expert and farmer were tallied for each combination of scores and converted to a percentage of the expert’s total.
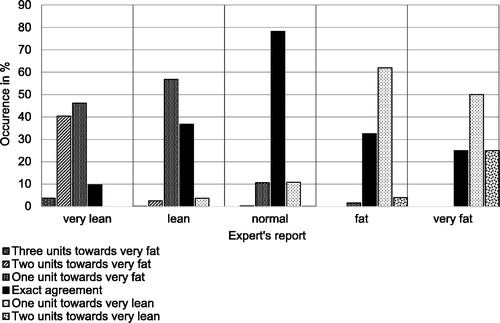
Table 2. Inter-rater reliability at animal level.
Avoidance distance
The assessment of avoidance distance obtained slight inter-rater reliability when the ordinal scale was summarised to form a dichotomous variable (). Inter-rater reliability was also slight (κω = 0.163 [0.111 − 0.215]; p < 0.001) when the scale of three categories was addressed. However, as the avoidance behaviour of cows is influenced by whether the observer is familiar or unfamiliar to the animal (Waiblinger et al. Citation2006), differences between the external’s and stockperson’s observation had to be assumed. Accordingly, the expert recorded avoidance behaviour in 783 out of 1650 cows, while farmers inconsistently claimed to be able to touch 559 of these animals on the muzzle.
The use of a non-validated test in head-to-wall tie-stalls can be justified by the fact that the primary aim was to evaluate inter-rater reliability, so the reliability of the test itself was of secondary importance.
Skin alterations
Regarding skin alterations on the neck, inter-rater reliability of the assessment of hairless patches was moderate, whereas the evaluation of swellings demonstrated slight reliability (Table ). In accordance with Gibbons et al. (Citation2012), it was recognised that scoring outstretched necks during eating compared to scoring relaxed necks when cows are in a head-up position resulted in different assessments of swelling. This may therefore have contributed to disagreement between the observers, since no information was provided on the exact time of the evaluation. Looking at integument alterations at the knee, inter-observer reliability of the assessment of hair loss and swellings was slight (Table ). In contrast to the neck region, there were hardly noticeable differences between hair loss and swellings, possibly as the accuracy of evaluation was basically dependent on farmer’s efforts to bend down to the knee for an optimal visibility. In addition, good lighting may have been an important factor in the assessment of the carpal joint, because the focal area is much smaller than the neck, for example. Accordingly, it was sometimes necessary to turn on the lights in the barn, but possibly farmers did not do this for reasons of convenience. Inter-rater reliability of the assessment of hairless patches at the hock was fair, whereas the evaluation of swellings at the hock obtained slight reliability (). There are only few studies available on observer agreement in tarsal joint injury. For instance, Rutherford et al. (Citation2008) demonstrated moderate to high reliability between assessors. Similar to the carpal region, disagreement may have been due to the small focal area, which requires more effort to make an accurate assessment. Regarding the observation of swellings, however, it must also be considered that it may have been difficult to achieve a good reliability coefficient, because the prevalence of swellings was only 3.6%. In each region observed, Cohen’s kappa was consistently lower for swelling than for hair loss (Table ) pointing to greater difficulties of the farmers in the assessment of swellings as they were likely to be less obvious in the visual examination without manual palpation. Lesions were holistically not considered, because their prevalence reported by both expert and farmers was generally < 1.0%.
Dirtiness
The assessment of dirtiness at the udder demonstrated slight reliability between the coders, whereas fair inter-rater reliability was determined for dirtiness at the upper and lower hind leg (Table ). As the prevalence of animals showing dirt at the udder was lower than 10.0% (Table ), however, the probability of chance agreement was high. In addition, the comparability of the observers’ data at animal level was limited due to the short-term stability characterising the measurement. When dirtiness at the lower and dirtiness at the upper hind leg were summarised to address this concern, inter-rater reliability was still fair (κ = 0.276 [0.231 − 0.320]; p < 0.001) indicating that farmers may have had a different understanding of dirtiness despite the precise instructions to take account of dirt resulting in a palm-size area.
Claw conformation
Inter-observer reliability of the evaluation of overgrown claws and other claw disorders at the front and hind leg was slight (Table ). Chance agreement was high due to the low prevalence of cows with other claw disorders at the front and hind leg (Table ), which could at least explain the low values of Cohen’s kappa regarding the assessment of other claw disorders. In general, due to the small focal area to be observed, the assessment may have been dependent on farmer’s efforts to ensure an optimal visibility (e.g. good lighting). While a high amount of bedding material may have covered the claws in tie-stalls, heavy dirtiness of the claws, likely caused by poor management regarding the quantity of manure present in the corridors, may have been a relevant factor in loose housings. Given the time interval between the expert’s and farmer’s assessment, disagreement may also have been due to claw trimming, as it was not checked whether claw trimming had been performed between the assessments.
Lameness
When the ordinal scale was collapsed to form a dichotomous variable, the assessment of lameness when standing and when moving showed fair reliability between the observers (Table ). Conversely, taking the three-point scale into account, Cohen’s weighted kappa consistently indicated fair reliability (κω = 0.213 [0.069 − 0.356] when standing, κω = 0.298 [0.187 − 0.410] when moving; p < 0.001). 22.6% of cows assessed as lame when standing were recorded consistently by the farmers, while the respective percentage was 32.3% in movement. Indeed, various studies have already shown that recognising locomotion difficulties poses challenges to farmers. Whay et al. (Citation2002) published that farmers on average detected a quarter of their lame animals, while Šárová et al. (Citation2011) asserted that farmers only identified a fifth of the lameness cases.
Getting up behaviour
Inter-observer reliability of the assessment of getting up behaviour was slight when the indicator was considered as a dichotomous variable (Table ). When considering the multi-category scale, reliability was also slight (κ = 0.117 [0.061 − 0.172]; ns). Besides individual discomfort (e.g. due to disease or age of the animal), shortcomings in housing structure (e.g. small lunging space) can potentially cause abnormal getting up behaviour. In response to inadequacies in stall design, all cows kept on the farm may exhibit similar disturbances in getting up behaviour. In such cases, there is no point of comparison and, therefore, it is even more difficult for the farmer to detect the abnormal behaviour. This could result in operational blindness, which may have been a reason for the slight level of agreement.
Only 908 out of 1719 dairy cows were monitored by both observers, because the expert’s assessment was not feasible in an acceptable time frame, if cows to be scored were standing all the time, e.g. due to feeding.
Reliability assessment at farm level
Irrespective of the factors mentioned above that may have influenced the inter-rater reliability, the time interval between the expert's and farmer's assessment must also be kept in mind. Due to the long distances to travel, an appropriate route planning (i.e. two to four neighbouring farms per day) was required without being able to react to the time of the farmer’s observation in order to save costs and environmental emissions. Thus, this issue in itself is a consequence of the problem to which the paper refers.
It could have been solved by using more than one expert if the inter-observer reliability between the experts had been established as sufficiently high in advance. However, apart from practical constraints (e.g. costs), it seemed to be an advantage that only one well-trained person performed the on-farm assessment. Alternatively, long intervals could have been avoided by asking the farmers to exercise their assessment shortly before or after the expert’s visit. In that case, farmers would have had to receive financial compensation for carrying out the assessment at the exact time needed, which was impossible. The study therefore relied entirely on the farmers’ willingness. Nevertheless, a large gap between farmers’ classroom session and on-farm assessment was planned to be avoided by predefining the time window for self-evaluation from March to April 2019. It should be ensured that farmers still remember the knowledge acquired in theory. However, the majority of participants did not meet the time limit.
For these reasons, the average time interval between the expert’s and farmer’s assessment was 70 days. The comparability of the expert's and farmer’s data at animal level was limited, as there might indeed have been changes in which individual animals suffered from any specific condition. However, the farm-level prevalence of cows affected may have been stable. Given the objective of welfare assurance schemes in determining how a farm performs overall in terms of welfare outcomes, farm-level reliability was analysed. In this way, it was examined whether expert and farmer reported the same prevalence at farm level, even if there was disagreement regarding individual cows. Analyses revealed that the ICC ranged from negligible to moderate reliability (Table ), in line with present results at animal level. In some cases, disagreement between the raters could in fact have been a reflection of actual changes in the percentage of animals affected over the time, e.g. the percentage of cows with overgrown claws due to claw trimming performance. Overall, however, it does not seem plausible that differences between the expert’s and farmer’s assessment were only due to true changes in the prevalence of cows affected. Therefore, it must be argued that there have been great challenges in the farmers’ welfare assessment.
Table 3. Inter-rater reliability at farm level.
Recommendations to improve data quality
The farmers’ self-assessment faces obstacles that must be overcome because it has not led to reliable welfare outcomes. One way to secure and improve data quality is to reconsider the content of the protocol used. Gibbons et al. (Citation2012) stated that when considering welfare outcome assessment, information is generally required at farm level, for which a binary scale of indicators may be sufficient. In light of this, they demonstrated that simpler scoring scales can provide more reliable results compared to a more precise scale for injury assessment. Vasseur et al. (Citation2013), who used a 14-point BCS chart, also acknowledged that it may be arguable whether such a fine level of precision is needed, if the sole intention of this indicator is to detect cows with extreme conditions. BCS was therefore classified on a five-point scoring system with one-point increments. Although, for example, the WQ protocol relies on a three-point BCS scale, five categories have been retained, as the ideal BCS profile for dairy cows varies between lean, normal, and fat score depending on the cow’s point of production cycle. Very lean and very fat cows, however, always represent extremes that must be detected to implement corrective measures. From a statistical point of view, Cohen’s kappa and weighted kappa consistently indicated fair inter-rater reliability of BCS assessment, even though Cohen’s weighted kappa was slightly higher. For all other ordinal variables, Cohen’s weighted kappa was lower compared to Cohen’s kappa. Thus, these results confirmed that inter-rater reliability can be improved by using binary scales. Looking on the drawbacks of having binary scales instead of ordinal scales for conditions that e.g. can vary from mild to severe, there is a substantial loss of information.
Additionally, the training programme has to be intensified to ensure that farmers will achieve better reliability with the expert in future. In this regard, appropriate and repetitive theoretical training is recommended, as before in classroom or online (e.g. by offering webinars with pop-up questions) based on the findings of Schenkenfelder and Winckler (Citation2017). In order to work towards standardising the assessment through on-farm training, it is suggested that an expert undertakes formal scoring together with the farmer during the routine on-farm inspections. This is modelled on an initiative in the UK called joint-scoring, which has been included for scientific purposes in farm certification visits under the Soil Association and Freedom Food Scheme (van Dijk et al. Citation2018). According to internal review studies on farmers’ opinion, the majority of British farmers reported that the process led to a useful discussion with the assessor on allocated scores, which offered on-farm learning opportunities, avoided conflict and built rapport with the auditor, who was increasingly considered as an important source of advice (van Dijk et al. Citation2018). Therefore, on-farm repeatability assessment may substantially reduce the variability in the data collected and secure high data quality by ensuring that the reference standard is maintained over time (Gibbons et al. Citation2012; Vasseur et al. Citation2013). Typically, repeatability assessment in terms of refresher-courses and mid-way-checks is performed during the training of welfare assessors (e.g. Gibbons et al. Citation2012; Vasseur et al. Citation2013). In this research, however, it could not be carried out for reasons of feasibility. Multiple trips from and back to mountain farms for comparative repeatability assessment of a cattle research unit could not be arranged due to the long distances to travel. Mountain farmers also face particular time constraints, especially if the farm is run alone or as a sideline. Conversely, only one expert was involved, which is why on-farm repeatability checks were not possible. Given these practical conditions, which require compromises, the on-farm session under the training programme had to be limited to a single assessment of the farmers’ cows.
Thus, if a high standard of training is received with regular repeatability assessment, farmers should be able to produce more accurate and reliable data (Mullan et al. Citation2011). In response to the learning process, the frequency of external audits could be gradually reduced in the medium- to long-term by increasingly transferring the competence for welfare assessment to dairy farmers.
However, the record on which agricultural assessors potentially make compliance decisions would not be honest or accurate in all cases. There was recognition that farmers could just write down what they wanted when undertaking self-assessment, which was also substantiated by findings of van Dijk et al. (Citation2018). In any case, provision must therefore be made for occasional unannounced checks of randomly selected farms to be able to guarantee a realistic assessment of indicators and to ensure the programme’s credibility to consumers and retailers. Further, consultation exercises relating to farmers’ perception of welfare outcome assessment conducted by van Dijk et al. (Citation2018) have also shown an ignition of criticisms of the self-assessment approach, such as the perceived bureaucracy and unnecessary duplication of something farmers feel they are daily working for as a matter of course, and were proud and passionate about. Accordingly, if self-assessment were to be mandatory for all dairy farmers in South Tyrol, its implementation would probably be hampered by some skeptics, who oppose self-assessment and, therefore, need to be convinced of the benefits.
Conclusions
Accredited assurance schemes prefer to use an outcome-based approach to measure dairy cattle welfare. However, farmers’ self-assessment of animal-based indicators is challenging. Inter-rater reliability of welfare outcome assessment by an expert and farmers was slight to moderate. These findings were consistent with results at farm level. In order to improve the quality of the farmers’ data, recommendations were drawn up as follows: (1) optimisation of the recording method by simplifying the scales of indicators, (2) intensification of the theoretical training sessions and, (3) implementation of on-farm repeatability assessment. In this way, the competence for regular and standardised monitoring of welfare indicators could be increasingly transferred to dairy farmers in order to reduce the need for costly and time-consuming external inspections, which are also harmful to the alpine environment.
Ethical approval
The experimental and notification procedures were carried out in compliance with Directive 86/609/EEC.
Acknowledgements
The authors wish to deeply thank the Autonomous Province of Bolzano, which supported this study within the action plan Mountain Agriculture, Ms. Kaser and Ms. Steinmayer from the Sennereiverband Südtirol for their excellent cooperation as well as all South Tyrolean dairies integrated in the project. Finally, the authors are grateful to all the mountain farmers who participated for their active engagement and commitment to improve dairy cattle health and welfare in South Tyrol.
Disclosure statement
No potential conflict of interest was reported by the authors.
Additional information
Funding
References
- Animal Welfare Act. 2006. Animal Welfare Act published on 18 May 2006 (BGBl. I p. 1206, 1313), last amended by Article 3 of the Act published on 28 July 2014 (BGBl. I p. 1308). [accessed 2020 Jun 25]. http://www.gesetze-im-internet.de/tierschg/BJNR012770972.html.
- Battaglini L, Bovolenta S, Gusmeroli F, Salvador S, Sturaro E. 2014. Environmental sustainability of Alpine livestock farms. Ital J Anim Sci. 13:431–443.
- Bätzing W. 2015. Die Alpen: Geschichte und Zukunft einer europäischen Kulturlandschaft [The Alps: History and future of a European cultural landscape]. 4th rev. ed. Munich: C. H. Beck. Chapter III.3. Landwirtschaft in den Alpen – unverzichtbar, aber zukunftslos?; p. 152–163. German.
- Brinkmann J, Ivemeyer S, Pelzer A, Winckler C, Zapf R. 2016. Milchkühe. In: Tierschutzindikatoren: Leitfaden für die Praxis – Rind [Animal welfare indicators: practical guide – cattle]. 1st ed. Darmstadt: Silber Druck; p. 10–29.
- Burn CC, Weir AAS. 2011. Using prevalence indices to aid interpretation and comparison of agreement ratings between two or more observers. Vet J. 188(2):166–170.
- de Vries M, Bokkers EAM, van Schaik G, Engel B, Dijkstra T, de Boer IJM. 2014. Exploring the value of routinely collected herd data for estimating dairy cattle welfare. J Dairy Sci. 97(2):715–730.
- [EFSA] European Food Safety Authority. 2012. Panel on Animal Health and Welfare (AHAW) Scientific Opinion on the use of animal-based measures to assess welfare of dairy cows. Efsa J. 10:2554–2634.
- [EFSA] European Food Safety Authority. 2015. Scientific Opinion on the assessment of dairy cow welfare in small-scale farming systems. Efsa J. 13:4137–4239.
- Gibbons J, Vasseur E, Rushen J, de Passillé AM. 2012. A training programme to ensure high repeatability of injury scoring of dairy cows. Anim Welf. 21(3):379–388.
- Hinkle DE, Wiersma W, Jurs SG. 2003. Applied statistics for the behavioral sciences. 5th ed. Boston (MA): Houghton Mifflin.
- Katzenberger K, Rauch E, Erhard M, Reese S, Gauly M. Forthcoming 2020. Evaluating the need for establishment of an animal welfare assurance programme in South Tyrolean dairy farming. Ital J Anim Sci.
- Knierim U, Winckler C. 2009. On-farm welfare assessment in cattle: validity, reliability and feasibility issues and future perspectives with special regard to the Welfare Quality approach. Anim Welf. 18:451–458.
- Landis JR, Koch GG. 1977. The measurement of observer agreement for categorial data. Biometrics. 33(1):159–174.
- Leach KA, Dippel S, Huber J, March S, Winckler C, Whay HR. 2009. Assessing lameness in cows kept in tie-stalls. J Dairy Sci. 92(4):1567–1574.
- Mullan S, Edwards SA, Butterworth A, Whay HR, Main DCJ. 2011. Inter-observer reliability testing of pig welfare outcome measures proposed for inclusion within farm assurance schemes. Vet J. 190(2):e100–e109.
- Popović ZB, Thomas JD. 2017. Assessing observer variability: a user's guide. Cardiovasc Diagn Ther. 7(3):317–324.
- Rushen J, Butterworth A, Swanson JC. 2011. Animal behavior and well-being symposium: Farm animal welfare assurance: science and application. J Anim Sci. 89(4):1219–1228.
- Rutherford KMD, Langford FM, Jack MC, Sherwood L, Lawrence AB, Haskell MJ. 2008. Hock injury prevalence and associated risk factors on organic and nonorganic dairy farms in the United Kingdom. J Dairy Sci. 91(6):2265–2274.
- Šárová R, Stěhulová I, Kratinová P, Firla P, Spinka M. 2011. Farm managers underestimate lameness prevalence in Czech dairy herds. Anim Welf. 20:201–204.
- Schenkenfelder J, Winckler C. 2017. Development and evaluation of an online training-tool for the assessment of animal-based welfare parameters in cattle. Agriculturae Conspectus Scientificus. 82:201–204.
- Sennereiverband Südtirol. 2020. Tätigkeitsbericht 2019. [accessed 2020 Jun 15]. https://www.suedtirolermilch.com/CustomerData/655/Files/Documents/2018_taetigkeitsbericht_milchsektor.pdf.
- van Dijk L, Elwes S, Main DCJ, Mullan SM, Jamieson J. 2018. Farmer perspectives on welfare outcome assessment: learnings from four farm assurance scheme consultation exercises. Anim Welf. 27(1):1–11.
- van Os JMC, Winckler C, Trieb J, Matarazzo SV, Lehenbauer TW, Champagne JD, Tucker CB. 2018. Reliability of sampling strategies for measuring dairy cattle welfare on commercial farms. J Dairy Sci. 101(2):1495–1504.
- Vasseur E, Gibbons J, Rushen J, de Passillé AM. 2013. Development and implementation of a training program to ensure high repeatability of body condition scoring of dairy cows. J Dairy Sci. 96(7):4725–4737.
- Vasseur E, Gibbons J, Rushen J, Pellerin D, Pajor E, Lefebvre D, de Passillé AM. 2015. An assessment tool to help producers improve cow comfort on their farms. J Dairy Sci. 98(1):698–708.
- Waiblinger S, Boivin X, Pedersen V, Tosi MV, Janczak AM, Visser EK, Jones RB. 2006. Assessing the human–animal relationship in farmed species: a critical review. Appl Anim Behav Sci. 101(3-4):185–242.
- [WBABMEL] Scientific Advisory Board on Agricultural Policy, Food and Consumer Health Protection of the Federal Ministry of Food and Agriculture. 2015. Wege zu einer gesellschaftlich akzeptierten Nutztierhaltung [Ways towards socially accepted livestock farming]. Expert opinion. Berlin: Federal Ministry of Food and Agriculture (BMEL).
- Weary DM, von Keyserlingk MAG. 2017. Public concerns about dairy-cow welfare: how should the industry respond? Anim Prod Sci. 57(7):1201–1209.
- Welfare Quality. 2009. Welfare Quality assessment protocol for cattle. Lelystad: Welfare Quality Consortium.
- Whay HR, Waterman Pearson AE, Webster AJF. 2002. The use of behavioural observation in the identification and monitoring of lameness. In: Proceedings of the 12th International Symposium on Lameness in Ruminants; Jan 9–13; Orlando, FL. p. 302–305.
- Wildman EE, Jones GM, Wagner PE, Boman RL, Troutt HF, Lesch TN. 1982. A dairy cow body condition scoring system and its relationship to selected production characteristics. J Dairy Sci. 65(3):495–501.