ABSTRACT
Worldwide efforts are underway to replace or repair lost or dysfunctional pancreatic β-cells to cure diabetes. However, it is unclear what the final product of these efforts should be, as β-cells are thought to be heterogeneous. To enable the analysis of β-cell heterogeneity in an unbiased and quantitative way, we developed model-free and model-based statistical clustering approaches, and created new software called TraceCluster. Using an example data set, we illustrate the utility of these approaches by clustering dynamic intracellular Ca2+ responses to high glucose in ∼300 simultaneously imaged single islet cells. Using feature extraction from the Ca2+ traces on this reference data set, we identified 2 distinct populations of cells with β-like responses to glucose. To the best of our knowledge, this report represents the first unbiased cluster-based analysis of human β-cell functional heterogeneity of simultaneous recordings. We hope that the approaches and tools described here will be helpful for those studying heterogeneity in primary islet cells, as well as excitable cells derived from embryonic stem cells or induced pluripotent cells.
KEYWORDS:
Introduction
Diabetes is the result of pancreatic islet β-cell dysfunction and/or death.Citation1,2 Clinical studies demonstrate the therapeutic potential of islet cell replacement for diabetes,Citation3,4 and therefore many academic and industrial laboratories have sought to produce functional β-cells from sources such as embryonic stem cells. Up to this point, single-cell analysis suggests that only a small percentage of partially functional cells have been produced.Citation5 Among the many technical challenges is the fact that even the most sophisticated published protocols generate highly heterogeneous cultures of cells.Citation5 This necessitates high-throughput analysis at the single-cell level. To do this, current functional studies typically compare ‘representative example’ Ca2+ signal traces between stem cell derived cells (or groups of cells) to primary human islet cell preparations of variable quality.Citation5,6 The reason for assaying Ca2+ flux is that the main function of a pancreatic β-cell is glucose-stimulated insulin exocytosis, a process that requires voltage-dependent Ca2+ signals caused by the closure of ATP-sensitive K+ channels.Citation7 The analyses of mRNA, protein, and metabolites can further assist with the comparison of stem cell derived cultures to human islets,Citation5,6,8 but these can be misleading as preparations are typically of mixed cell types. New breakthroughs in single-cell transcriptomics, epigenomics and proteomics promise to enable snapshots of cell-to-cell heterogeneity.Citation9 However, the continuous analysis of single living cells, including real-time measurements of signal transduction events, is still required to assess true phenotypic heterogeneity.
The development of surrogate β-cells is also hampered by limited understanding of the function and functional heterogeneity in fully responsive human islets from healthy donors. In other words, it is not clear exactly what the features are of the target cell type, or cell types, that need to be generated. This question is not trivial, and attempts to answer it reveal critical gaps in the understanding of human islet cell physiology. Indeed, heterogeneity in cell state and cell fate remains a poorly understood aspect of β-cell physiology, despite several studies.Citation10-18 Importantly, it has been proposed that functional heterogeneity itself may be critical for the ideal performance of the intact islet.Citation19-21 Studies in this realm typical focus on rodent β-cells,Citation11,24,25 as the majority of human islet preparations do not match the quality that can be achieved under the controlled conditions of rodent islet isolation, where we and others have documented low levels of cell death in culture.Citation9 Moreover, the majority of human islet donors are older, with β-cells that have endured decades of diets of variable quality.
Here, we present TraceCluster (https://jimjohnsonsci.shinyapps.io/TraceCluster), a software app designed to enable the unbiased, high-throughput analysis of functional heterogeneity between islets cells using model-free and model-based clustering. We demonstrate the utility of these approaches and the new software on a rare set of ∼300 high quality simultaneous recordings of human islet cells from a young donor and find two functional groupings of β-cell like responses and one functional grouping of non-β-cell responses.
Methods
Primary culture of human islet cells
Human islets were isolated at the I.K. Barber Islet Transplantation Facility (Vancouver, Canada) from a healthy 11.8-year old male who died suddenly of head trauma (isolation code HR196). The warm ischemic time was 0 min and the cold ischemic time was 135 min. The pancreas weight was 61 g. The digestion time was 10 min. The final isolation yielded 378,006 IE (islet equivalents) with >90% purity. Some islets were embedded in agar, fixed, sectioned and examined with antibodies to insulin, glucagon or Pdx1 () as described elsewhere.Citation5,22 Once isolated, the islets were transported to the laboratory within a few hours. Islets were gently dispersed as described.Citation23,24 The resulting cells were plated on a coverslip () and incubated in RPMI media (containing 5.5 mM glucose and 100 IU/ml penicillin, 100µg/ml streptomycin, 10% fetal calf serum; pH 7.4 with NaOH) and maintained at 37°C, 5% CO2 and saturated humidity for 2 d.
Figure 1. Cell composition of intact islets and dispersed islet cells of test sample. (A) Representative immunofluorescence staining of insulin, glucagon, and Pdx1 in intact islets from the donor. DNA counterstaining employed DAPI. The percentage of β-cells and α-cells (out of the major endocrine population i.e., β-cells or α-cells) is shown. Intact islets from this preparation contained attached non-endocrine cells; insulin positive cells were 37.3% of all cells and glucagon-positive cells were 23.7% of all cells. (B) Cultures of dispersed islet cells (including all endocrine and non-endocrine cells labeled with DAPI) contained 48.2% cells that stained robustly for insulin.
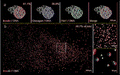
Ca2+ Imaging
Reagents were from Sigma (St. Louis, MO), unless otherwise indicated. Ca2+ imaging was performed in Ringer's solution containing (in mM): 5.5 KCl, 2 CaCl2, 1 MgCl2, 20 HEPES, 141 NaCl and 3 glucose according to standard protocols.Citation10,24,25 Briefly, islet cell cultures were incubated with 1 µM Fura-2-AM (Molecular Probes, Eugene, OR) in RPMI for 30 minutes and rinsed in Ringer's solution for 30 minutes. Coverslips, in a 37°C chamber (∼300 µL), were continuously perfused with pre-heated solutions, to maximize control over the contents of the bath (especially ambient insulin levels). Cells were excited at 340 nm and 380 nm using a 300 W Sutter DG4 and imaged with a Roper CoolSnapHQ2 CCD camera, mounted on a Zeiss 200 m microscope (Intelligent Imaging Innovations). In total, 480 time points were recorded simultaneously for each of 291 islet cells over 80 min. All individual traces are provided in the Supplementary File, which is further explained in the Supplementary Information. Over the course of the experiment, cells were exposed to the following conditions: 20 min in 3 mM (low) glucose, 30 min in 20 mM (high) glucose, 15 min in 3 mM glucose, 10 min in 30 mM KCl, and 5 min in 3 mM glucose. The ratio of emission intensity >510 nm from excitations at 340 nm and 380 nm, was recorded for all cells.
Normalization, quality control and analysis of live cell imaging data
The minor signal drift (as can be seen in the individual traces in the Supplementary File) was corrected per cell by estimating median increase in fluorescence signal per time step under baseline conditions (first 20 minutes). Correction for signal drift did not significantly alter the cell feature estimates described in this work. Fifteen cells (∼5%) were filtered from further analysis due to poor overall signal response, defined as a median KCl response less than 5% over baseline (median signal within the first 20 min). All data normalization, QC and analyses were performed within the R environment, details of which are provided in the Supplementary Information.
Results
Ca2+ imaging
The islets used to demonstrate the utility of our cluster analyses were isolated without complication and dispersed into single cells within 24 h (see Online Methods for details). Prior to dispersion, islets were collected for characterization of cellular composition using immunofluorescence staining for insulin, glucagon and PDX1, a critical regulator of islet-cell health and functionCitation10,26,27 (). In these islets, β-cells accounted for 61.1% of the major endocrine population (i.e. β-cells and α-cells). Beta-cells were robustly positive for the PDX1 transcription factor (). These observations compare favourably with the β-cell percentage reported by othersCitation28,29 and with our typical experienceCitation5 (see also Supplementary Fig. 5). After islet dispersion, coverslips demonstrated a large proportion of β-cells when compared to all cells (endocrine and non-endocrine, ). Collectively, these observations indicate that abundant and healthy dispersed β-cells were prepared from the donor.
Ca2+ signals were simultaneously recorded from 291 cells, in 3 imaging fields, while sequentially exposed to either low glucose (3 mM) or high glucose (20 mM) before depolarization with 30 mM KCl (, Supplementary Fig. 1). The recordings were technically sound and free of significant drift (correction for drift did not alter the main conclusions in this work). Importantly, because all cells were imaged in a single batch run, these data are free of experimental variability. Based on extensive experience working with human islets,Citation12,23,24,26,30-33 it is estimated that these were among the highest quality islet cells we have studied. Therefore, we chose this data set as a test case for detailed analysis and development of a functional clustering strategy. Selected cells from this data set have also been compared to embryonic stem cell-derived cells in a recent publication.Citation5
Figure 2. Ca2+ traces and exploratory data analysis. (A) Raw Ca2+ traces from Fura-2 stained islet cells showing the timed glucose perturbations and KCl depolarisation. (B) Cells were imaged in three microscopy fields (Supplementary Fig. 1). Shown are the five top ranked MDS dimensions of the raw Ca2+ traces based on large mean silhouette width between the fields (details provided in Supplementary Information). Cells are colored red, dark gray or light gray to reflect the three different microscopy fields. We noticed that the cluster of cells circled in yellow enriched for cells of one field due to inhomogeneous dispersion, but more importantly that these cells showed a distinct Ca2+ trace pattern irrespective of field. The traces are shown for these cells which are mostly/only responding during KCl depolarisation.
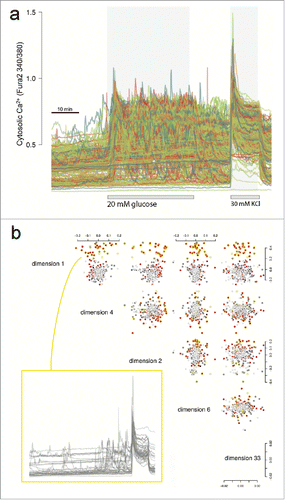
Exploratory analysis
Our goal was to analyze functional responses to defined stimuli of human islet cells without using a priori knowledge of ‘cell type’. Multi-dimensional scaling (MDS) was initially used to explore low dimension projections of the intracellular Ca2+ traces acquired using Fura-2 imaging. The distance matrix for the projections was calculated as (1-ρij)/2, with ρij being the Spearman correlation between cell i and j. As shown in , we noticed that the first few dimensions suggest 2 clusters of cells, largely representing glucose responders (at low or high glucose) and non-responders (Fura-2 response only with or close to KCl perturbation). Non-responders were found to be in different proportions between the three imaging fields, suggesting nonhomogeneous dispersion of the cells (−log10P = 4.98 for the null hypothesis that the proportions across the fields are the same). However, no consistent spatial effect could be detected within the imaging fields (Supplementary Fig. 2).
Ca2+ trace feature extraction and clustering
Next we sought to perform less naïve analyses of glucose responses in our human islet data set. To do this, nine Ca2+ response features were extracted from the Ca2+ imaging traces for further study. ‘High glucose response’ was defined as the median high glucose (20 mM) signal above baseline (median signal during the first 20 min), normalized as a ratio of median KCl signal above baseline. ‘High glucose oscillation’ was defined as the magnitude of oscillations during high glucose exposure, expressed as a ratio of the median absolute deviation (MAD) to median KCl response above baseline. ‘Low glucose oscillation’ was defined as the magnitude of oscillations during the first 2 low glucose exposures (3 mM before and after high glucose respectively), estimated in a similar manner to the high glucose oscillations. ‘Response speed’ was defined as the inverse of time to first oscillation peak with high glucose exposure, a measure of time responsiveness to perturbation. Number of ‘counted peaks’ during high glucose exposure were estimated as local maxima from each trace, with the constraint that a local maximum be considered a true peak if at least one third of the signal difference between median KCl and baseline glucose signal. Peak periodicity was used also to estimate the mean ‘oscillation frequency’. ‘Return to baseline’ was defined as the local minimum during the second low glucose exposure, normalized as a ratio of median KCl signal above baseline. ‘KCl response’ was defined as the maximum KCl response above baseline, normalized as a ratio of median KCl signal above baseline.
Hierarchical clustering of the features, as shown in (and detailed in the Supplementary Information) pointed to 3 ‘feature’ clusters. The first feature cluster was comprised of cells that responded with clear oscillations when exposed to high glucose. The number of high glucose peaks and estimated oscillation frequency were, as expected, found to largely correlate (Supplementary Fig. 3). The lower the number of estimated peaks, the lower this correlation, suggesting a possible relationship between level and variability in oscillation frequency. Future studies will require longer high glucose exposures to study this relationship with sufficient statistical power. Overall, the defining relationship found in this cluster was the correlation between oscillation frequency and oscillation magnitude during high glucose exposure (Spearman ρ −log10P = 15.05). The second observed feature cluster () consists of the correlation between high glucose response, response speed (Spearman ρ −log10P = 11.44) and magnitude of low glucose oscillations after high glucose exposure (Spearman ρ −log10P = 7.61). This suggests a sub-type of predominantly rapid responders, usually with low/no oscillation during high glucose but some oscillation during subsequent low glucose instead of complete return to baseline. The final observed feature cluster () largely contains cells of little/no high glucose response. The same ‘non-responders’ were observed with the exploratory data analysis. Interestingly, these cells demonstrated greater relative low glucose oscillation magnitudes (during the initial 20 min) than high glucose oscillation magnitudes. This, despite their absolute magnitudes typically being much smaller than observed oscillation magnitudes for the oscillators in the first feature cluster. With 3 predominant clusters suggested by the data, we note that 2 features would suffice to capture the gross behavior in this sample. Parametric (Gaussian) mixture models of high glucose response and oscillation are presented in , with the Baysian Information Criterion (BIC) suggesting the same 3 dominant cell behaviors. Although tempting to further sub-divide the cells based on responsiveness to high glucose, as shown in , the agreement between the drift-corrected and raw data breaks down beyond three clusters.
Figure 3. Hierarchical clustering of Ca2+ trace features and cells. The heatmap shown is reproduced from the provided software app (Supplementary Software), where users are able to select similar behaving cells and visualize their traces. In the heatmap, columns are clustered Ca2+ trace features while rows are clustered cells. Example traces from the software are also provided, where the first column of traces are cells from the top black box in the heatmap and the second column of traces are from the bottom black box. These are representative cells for the 2 β-like subtypes observed: oscillators and non-oscillators. The correlations between the features are provided in Supplementary Figure 3.
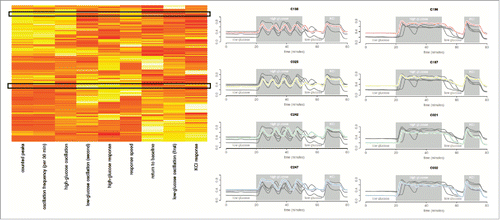
Figure 4. Model-based clustering of high glucose response and oscillations. All scatter-plots are of the same two features, though colored according to suggested clusters for drift-corrected (top row) and raw (bottom row) versions of the data. Gaussian mixture model BICs suggest optimal clustering of 3 major cell sub-types: non-responders (blue), oscillating responders (red) and non-oscillating responders (green). Drift-corrected BIC for four clusters was similar to that for three clusters, it did not suggest the same clustering pattern as the raw data. Three clusters therefore robustly describe the cell sub-type number. The drift-corrected 3-cluster plot highlighted is provided in the Trace Cluster software app (Supplementary Software), where users can upload their own data for analysis.
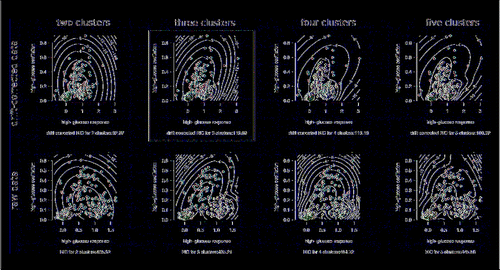
Considering the different clustering strategies and normalized versions of the data, the most robust conclusion is that there is a non-responding (perhaps α-like cells) and responding (β-like cells) population, with a further sub-division of the responders into oscillators and non-oscillators. We anticipate that similar high quality samples from the community will enable the meaningful study of further functional sub-divisions. Moreover, we expect that post-hoc immunostaining and/or single-cell transcriptomics will enable characterization of functional islet cell sub-types. After filtering out cells that did not respond to KCl, the proportion of cells responding to high glucose (71%) was reasonably similar to the expected β-cell frequency within the endocrine cell population of this preparation (). Interestingly, the non-oscillating cells of this population tended to possess a faster glucose response time. The 2 suggested cell states are reminiscent of the 2 β-cell sub-states, immature and mature, we have previously described in adult human and mouse β-cells based on mouse Ins1 promoter activity and gene expression analysis.Citation6-8 We speculate that the non-oscillators provide sensitivity in response while the oscillators syncronise the pulsatile insulin secretion. Worth noting is that the 15 cells (∼5%) filtered out for lack of any Fura-2 response, including to KCl, may represent non-excitable endothelial cells, exocrine cells, or unhealthy cells. However, the Fura-2 imaging technique tends to under-represent unhealthy cells, as they typically do not take up and cleave the Fura2-AM dye efficiently because AM esterase activity is energy-dependent.
Discussion
The goals of the present study were to develop new statistical approaches for the analysis of functional heterogeneity from live-cell imaging data and to develop a software app, called TraceCluster, (Supplementary Software) to allow direct comparative analysis of similar data sets with the presented data. Details for the installation and running of the software app are provided in the Supplementary Information. The thorough characterization of Ca2+ response heterogeneity from simultaneously imaged cells controls for any technical variation in this unique high-quality reference resource of Ca2+ responses from a young healthy donor. We expect that these data may serve as a target for efforts to generate functionally young pancreatic β-cells and for studies of the systems biology of these sub-types using emerging single-cell ‘omic’ technologies. It is important to note that the goal of our study was not to characterize β-cell heterogeneity, but instead to merely provide the tools to do so. Robust characterization of β-cell heterogeneity will require highly powered studies with perhaps hundreds of donors.
Complete characterization of human islet cell functionality is essential for efforts aimed at β-cell replacement in type 1 diabetes or even type 2 diabetes,Citation5,6,34 as it is not exactly clear how the desired output of cells from pluripotent stem cell differentiation protocols should behave. While there remains some controversy around how close the field is to producing true β-cells from human stem cells,Citation5,6,34 it is clear that the field is now at the stage of optimizing the insulin secreting cells such that they exhibit relevant functional characteristics of bona fide human β-cells. Several key questions remain. Should we attempt to generate functionally homogeneous β-cell replacements, and if so, what functional characteristics are ideal? On the other hand, perhaps the goal should be to generate a range of functional islet cell types that more accurately mimics primary human islets. There is also the question of age. The peak age of type 1 diabetes diagnosis is between 10–14 years of age, so perhaps the ideal β-cell replacement cells would respond to glucose in a manner similar to the ‘teen-aged’ cells studied here. Little is known about age-dependent changes in human β-cell function, after the initial post-natal maturation.Citation35 Evidence from rodent studies suggest some important functional differences between young mice, such as those from the most commonly studied ages (8–16 weeks of age), and mice older than 1 y.Citation36 Previous studies have noted important differences in gene expression and proliferative capacity between young and old mice that mimic known differences in humans.Citation37-41 It is notable that the oscillatory glucose responses observed in a large percentage of β-cells in the current study are not typical of published examples of β-cell responses from older human donors, which tend to exhibit brief, disorganized fluctuationsCitation23,32,33,42-45 or no fluctuations at all,Citation18 much like dysfunctional mouse β-cells.Citation45 Moreover, many of the Ca2+ responses in the current case exhibited the transient decrease in Ca2+ that initiates the response to glucose, known as ‘phase 0’,Citation46 which is not typically observed in other studies of human β-cells.Citation32 Collectively, our data demonstrate that β-cells from a healthy young donor can exhibit Ca2+ responses to glucose that resemble those observed commonly in mouse β-cells.Citation25,27,42,43,46,47 Thus, it seems possible that some of the functional differences assumed previously to be species-specific, may actually represent the effects of age on human islets, or their quality. While there may be important morphologic, genomic and functional differences between islets of different species,Citation43,48-50 the effects of age and quality should be considered in all studies. These speculations will require robust replication with multiple human and murine islet donors at multiple ages, which our new tools will enable.
Indeed, an obvious limitation of the experimental work described here is that it was conducted on a single donor, preventing any broad conclusions about the heterogeneity between donors. However, our main goal was to develop a statistical framework for analyzing heterogeneity of individual responses in populations of cells. These approaches and the software tools are modular and can be adapted to a variety of biological problems.
In summary, our statistical clustering to Ca2+ responses from ∼300 human islet cells imaged side-by-side was capable of identifying 2 functional β-cell states within this test sample. The approaches applied to this high-quality data set can be used to examine other β-cell states and in vitro generated replacement β-cells, or any other cell type.
Disclosure of potential conflicts of interest
No potential conflicts of interest were disclosed.
Author contributions
QFW conceived research concept, performed research and co-wrote the manuscript.
TB performed research and edited the manuscript.
AA performed research.
ZA performed research.
GLW provided resources and edited the manuscript.
TJK provided resources and edited the manuscript.
JDJ conceived research concept and co-wrote the manuscript.
Supplementary_data.zip
Download Zip (13.1 MB)Acknowledgments
Work was supported by grants from JDRF and CIHR to J.D.J.
Funding
This work was supported by an operating grant from the JDRF.
References
- Johnson JD, Luciani DS. Mechanisms of pancreatic β-cell apoptosis in diabetes and its therapies. Adv Exp Med Biol 2010; 654:447-62; PMID:20217509; http://dx.doi.org/10.1007/978-90-481-3271-3_19
- Szabat M, Page MM, Panzhinskiy E, Skovso S, Mojibian M, Fernandez-Tajes J, Bruin JE, Bround MJ, Lee JT, Xu EE, et al. Reduced insulin production relieves endoplasmic Reticulum stress and induces β cell proliferation. Cell Metab 2015; 23(1):179-93; PMID:26626461
- Shapiro AM, Ricordi C, Hering BJ, Auchincloss H, Lindblad R, Robertson RP, Secchi A, Brendel MD, Berney T, Brennan DC, et al. International trial of the Edmonton protocol for islet transplantation. N Engl J Med 2006; 355:1318-30; PMID:17005949; http://dx.doi.org/10.1056/NEJMoa061267
- Warnock GL, Thompson DM, Meloche RM, Shapiro RJ, Ao Z, Keown P, Johnson JD, Verchere CB, Partovi N, Begg IS, et al. A multi-year analysis of islet transplantation compared with intensive medical therapy on progression of complications in type 1 diabetes. Transplantation 2008; 86:1762-6; PMID:19104418; http://dx.doi.org/10.1097/TP.0b013e318190b052
- Rezania A, Bruin JE, Arora P, Rubin A, Batushansky I, Asadi A, O'Dwyer S, Quiskamp N, Mojibian M, Albrecht T, et al. Reversal of diabetes with insulin-producing cells derived in vitro from human pluripotent stem cells. Nat Biotechnol 2014; 32:1121-33; PMID:25211370; http://dx.doi.org/10.1038/nbt.3033
- Pagliuca FW, Millman JR, Gurtler M, Segel M, Van Dervort A, Ryu JH, Peterson QP, Greiner D, Melton DA. Generation of functional human pancreatic β cells in vitro. Cell 2014; 159:428-39; PMID:25303535; http://dx.doi.org/10.1016/j.cell.2014.09.040
- Misler S, Barnett DW, Gillis KD, Pressel DM. Electrophysiology of Stimulus-Secretion Coupling in Human Beta-Cells. Diabetes 1992; 41:1221-8; PMID:1397696; http://dx.doi.org/10.2337/diab.41.10.1221
- Hrvatin S, O'Donnell CW, Deng F, Millman JR, Pagliuca FW, DiIorio P, Rezania A, Gifford DK, Melton DA. Differentiated human stem cells resemble fetal, not adult, β cells. Proc Natl Acad Sci U S A 2014; 111:3038-43; PMID:24516164; http://dx.doi.org/10.1073/pnas.1400709111
- Sandberg R. Entering the era of single-cell transcriptomics in biology and medicine. Nat Methods 2014; 11:22-4; PMID:24524133; http://dx.doi.org/10.1038/nmeth.2764
- Szabat M, Luciani DS, Piret JM, Johnson JD. Maturation of adult β-cells revealed using a Pdx1/insulin dual-reporter lentivirus. Endocrinology 2009; 150:1627-35; PMID:19095744; http://dx.doi.org/10.1210/en.2008-1224
- Szabat M, Lynn FC, Hoffman BG, Kieffer TJ, Allan DW, Johnson JD. Maintenance of β-cell maturity and plasticity in the adult pancreas: developmental biology concepts in adult physiology. Diabetes 2012; 61:1365-71; PMID:22618775; http://dx.doi.org/10.2337/db11-1361
- Szabat M, Pourghaderi P, Soukhatcheva G, Verchere CB, Warnock GL, Piret JM, Johnson JD. Kinetics and genomic profiling of adult human and mouse β-cell maturation. Islets 2011; 3:175-87; PMID:21633187; http://dx.doi.org/10.4161/isl.3.4.15881
- Yang YH, Johnson JD. Multi-parameter single-cell kinetic analysis reveals multiple modes of cell death in primary pancreatic β-cells. J Cell Sci 2013; 126:4286-95; PMID:23843629; http://dx.doi.org/10.1242/jcs.133017
- Karaca M, Castel J, Tourrel-Cuzin C, Brun M, Geant A, Dubois M, Catesson S, Rodriguez M, Luquet S, Cattan P, et al. Exploring functional β-cell heterogeneity in vivo using PSA-NCAM as a specific marker. PLoS One 2009; 4:e5555; PMID:19440374; http://dx.doi.org/10.1371/journal.pone.0005555
- Leung YM, Ahmed I, Sheu L, Tsushima RG, Diamant NE, Hara M, Gaisano HY. Electrophysiological characterization of pancreatic islet cells in the mouse insulin promoter-green fluorescent protein mouse. Endocrinology 2005; 146:4766-75; PMID:16109783; http://dx.doi.org/10.1210/en.2005-0803
- Ling Z, Wang Q, Stange G, In't Veld P, Pipeleers D. Glibenclamide treatment recruits β-cell subpopulation into elevated and sustained basal insulin synthetic activity. Diabetes 2006; 55:78-85; PMID:16380479; http://dx.doi.org/10.2337/diabetes.55.01.06.db05-0820
- Silva AM, Dickey AS, Barnett DW, Misler S. Ion channels underlying stimulus-exocytosis coupling and its cell-to-cell heterogeneity in β-cells of transplantable porcine islets of Langerhans. Channels 2009; 3:91-100; PMID:19242122; http://dx.doi.org/10.4161/chan.3.2.7865
- Kenty JH, Melton DA. Testing pancreatic islet function at the single cell level by calcium influx with associated marker expression. PLoS One 2015; 10:e0122044; PMID:25853429; http://dx.doi.org/10.1371/journal.pone.0122044
- Smolen P, Rinzel J, Sherman A. Why pancreatic islets burst but single β cells do not. The heterogeneity hypothesis. Biophys J 1993; 64:1668-80; PMID:8369400; http://dx.doi.org/10.1016/S0006-3495(93)81539-X
- Benninger RK, Hutchens T, Head WS, McCaughey MJ, Zhang M, Le Marchand SJ, Satin LS, Piston DW. Intrinsic islet heterogeneity and gap junction coupling determine spatiotemporal Ca(2)(+) wave dynamics. Biophys J 2014; 107:2723-33; PMID:25468351; http://dx.doi.org/10.1016/j.bpj.2014.10.048
- Markovic R, Stozer A, Gosak M, Dolensek J, Marhl M, Rupnik MS. Progressive glucose stimulation of islet β cells reveals a transition from segregated to integrated modular functional connectivity patterns. Sci Rep 2015; 5:7845; PMID:25598507; http://dx.doi.org/10.1038/srep07845
- Asadi A, Bruin JE, Kieffer TJ. Characterization of antibodies to products of proinsulin processing using immunofluorescent staining of pancreas in multiple species. J Histochem Cytochem 2015; 63(8):646-62; In Press; PMID:26216140
- Johnson JD, Misler S. Nicotinic acid-adenine dinucleotide phosphate-sensitive calcium stores initiate insulin signaling in human β cells. Proc Natl Acad Sci U S A 2002; 99:14566-71; PMID:12381785; http://dx.doi.org/10.1073/pnas.222099799
- Luciani DS, Ao P, Hu X, Warnock GL, Johnson JD. Voltage-gated Ca(2+) influx and insulin secretion in human and mouse β-cells are impaired by the mitochondrial Na(+)/Ca(2+) exchange inhibitor CGP-37157. Eur J Pharmacol 2007; 576:18-25; PMID:17719029; http://dx.doi.org/10.1016/j.ejphar.2007.07.055
- Luciani DS, White SA, Widenmaier SB, Saran VV, Taghizadeh F, Hu X, Allard MF, Johnson JD. Bcl-2 and Bcl-xL suppress glucose signaling in pancreatic β-cells. Diabetes 2013; 62:170-82; PMID:22933114; http://dx.doi.org/10.2337/db11-1464
- Johnson JD, Bernal-Mizrachi E, Alejandro EU, Han Z, Kalynyak TB, Li H, Beith JL, Gross J, Warnock GL, Townsend RR, et al. Insulin protects islets from apoptosis via Pdx1 and specific changes in the human islet proteome. Proc Natl Acad Sci U S A 2006; 103:19575-80; PMID:17158802; http://dx.doi.org/10.1073/pnas.0604208103
- Johnson JD, Ahmed NT, Luciani DS, Han Z, Tran H, Fujita J, Misler S, Edlund H, Polonsky KS. Increased islet apoptosis in Pdx1+/− mice. J Clin Invest 2003; 111:1147-60; PMID:12697734; http://dx.doi.org/10.1172/JCI200316537
- Kilimnik G, Jo J, Periwal V, Zielinski MC, Hara M. Quantification of islet size and architecture. Islets 2012; 4:167-72; PMID:22653677; http://dx.doi.org/10.4161/isl.19256
- Pisania A, Weir GC, O'Neil JJ, Omer A, Tchipashvili V, Lei J, Colton CK, Bonner-Weir S. Quantitative analysis of cell composition and purity of human pancreatic islet preparations. Lab Invest 2010; 90:1661-75; PMID:20697378; http://dx.doi.org/10.1038/labinvest.2010.124
- Jeffrey KD, Alejandro EU, Luciani DS, Kalynyak TB, Hu X, Li H, Lin Y, Townsend RR, Polonsky KS, Johnson JD. Carboxypeptidase E mediates palmitate-induced β-cell ER stress and apoptosis. Proc Natl Acad Sci U S A 2008; 105:8452-7; PMID:18550819; http://dx.doi.org/10.1073/pnas.0711232105
- Dror V, Nguyen V, Walia P, Kalynyak TB, Hill JA, Johnson JD. Notch signalling suppresses apoptosis in adult human and mouse pancreatic islet cells. Diabetologia 2007; 50:2504-15; PMID:17922104; http://dx.doi.org/10.1007/s00125-007-0835-5
- Luciani DS, Johnson JD. Acute effects of insulin on β-cells from transplantable human islets. Mol Cell Endocrinol 2005; 241:88-98; PMID:16099589; http://dx.doi.org/10.1016/j.mce.2005.06.006
- Johnson JD, Kuang S, Misler S, Polonsky KS. Ryanodine receptors in human pancreatic β cells: localization and effects on insulin secretion. Faseb J 2004; 18:878-80; PMID:15033925
- Kushner JA, MacDonald PE, Atkinson MA. Stem cells to insulin secreting cells: two steps forward and now a time to pause? Cell Stem Cell 2014; 15:535-6; PMID:25517460; http://dx.doi.org/10.1016/j.stem.2014.10.012
- Fox JE, Seeberger K, Dai XQ, Lyon J, Spigelman AF, Kolic J, Hajmrle C, Joseph JW, Kin T, Shapiro AM, et al. Functional plasticity of the human infant β-cell exocytotic phenotype. Endocrinology 2013; 154:1392-9; PMID:23449893; http://dx.doi.org/10.1210/en.2012-1934
- Li L, Trifunovic A, Kohler M, Wang Y, Petrovic Berglund J, Illies C, Juntti-Berggren L, Larsson NG, Berggren PO. Defects in β-cell Ca2+ dynamics in age-induced diabetes. Diabetes 2014; 63:4100-14; PMID:24985350; http://dx.doi.org/10.2337/db13-1855
- Rankin MM, Kushner JA. Aging induces a distinct gene expression program in mouse islets. Islets 2010; 2:345-52; PMID:21099336; http://dx.doi.org/10.4161/isl.2.6.13376
- Teta M, Long SY, Wartschow LM, Rankin MM, Kushner JA. Very slow turnover of β-cells in aged adult mice. Diabetes 2005; 54:2557-67; PMID:16123343; http://dx.doi.org/10.2337/diabetes.54.9.2557
- Gunasekaran U, Hudgens CW, Wright BT, Maulis MF, Gannon M. Differential regulation of embryonic and adult β cell replication. Cell Cycle 2012; 11:2431-42; PMID:22659844; http://dx.doi.org/10.4161/cc.20545
- Gunasekaran U, Gannon M. Type 2 diabetes and the aging pancreatic β cell. Aging (Milano) 2011; 3:565-75
- Avrahami D, Li C, Zhang J, Schug J, Avrahami R, Rao S, Stadler MB, Burger L, Schübeler D, Glaser B, et al. Aging-Dependent Demethylation of Regulatory Elements Correlates with Chromatin State and Improved β Cell Function. Cell Metab 2015; 22:619-32; PMID:26321660; http://dx.doi.org/10.1016/j.cmet.2015.07.025
- Gwiazda KS, Yang TL, Lin Y, Johnson JD. Effects of palmitate on ER and cytosolic Ca2+ homeostasis in β-cells. Am J Physiol Endocrinol Metab 2009; 296:E690-701; PMID:19141690; http://dx.doi.org/10.1152/ajpendo.90525.2008
- Cabrera O, Berman DM, Kenyon NS, Ricordi C, Berggren PO, Caicedo A. The unique cytoarchitecture of human pancreatic islets has implications for islet cell function. P Natl Acad Sci USA 2006; 103:2334-9; http://dx.doi.org/10.1073/pnas.0510790103
- De Marchi U, Thevenet J, Hermant A, Dioum E, Wiederkehr A. Calcium co-regulates oxidative metabolism and ATP synthase-dependent respiration in pancreatic β cells. J Biol Chem 2014; 289:9182-94; PMID:24554722; http://dx.doi.org/10.1074/jbc.M113.513184
- Gilon P, Chae HY, Rutter GA, Ravier MA. Calcium signaling in pancreatic β-cells in health and in Type 2 diabetes. Cell Calcium 2014; 56:340-61; PMID:25239387; http://dx.doi.org/10.1016/j.ceca.2014.09.001
- Roe MW, Philipson LH, Frangakis CJ, Kuznetsov A, Mertz RJ, Lancaster ME, Spencer B, Worley JF, 3rd, Dukes ID. Defective glucose-dependent endoplasmic reticulum Ca2+ sequestration in diabetic mouse islets of Langerhans. J Biol Chem 1994; 269:18279-82; PMID:8034570
- Luciani DS, Misler S, Polonsky KS. Ca2+ controls slow NAD(P)H oscillations in glucose-stimulated mouse pancreatic islets. J Physiol 2006; 572:379-92; PMID:16455690; http://dx.doi.org/10.1113/jphysiol.2005.101766
- Kim A, Miller K, Jo J, Kilimnik G, Wojcik P, Hara M. Islet architecture: A comparative study. Islets 2009; 1:129-36; PMID:20606719; http://dx.doi.org/10.4161/isl.1.2.9480
- Steiner DJ, Kim A, Miller K, Hara M. Pancreatic islet plasticity: interspecies comparison of islet architecture and composition. Islets 2010; 2:135-45; PMID:20657742; http://dx.doi.org/10.4161/isl.2.3.11815
- Brissova M, Fowler MJ, Nicholson WE, Chu A, Hirshberg B, Harlan DM, Powers AC. Assessment of human pancreatic islet architecture and composition by laser scanning confocal microscopy. J Histochem Cytochem 2005; 53:1087-97; PMID:15923354; http://dx.doi.org/; http://dx.doi.org/10.1369/jhc.5C6684.2005