Abstract
In women, the uterine cervix and corpus uteri are two main suspects, playing a major role in cancer-associated-mortality. Immunologically, Toll-like receptors (TLRs) associated with the innate immune system, can recognize pathogens and induce immune responses against pathogens. Cellularly, TLR9 expression occurs in immune system cells including macrophages, natural killer cells, dendritic cells, and other antigen-presenting cells. TLR9 recognizes and interacts with viral and bacterial DNA comprising cytosine-phosphate-guanine (CpG) dideoxynucleotide motif. The current study is designed to identify the most deleterious nonsynonymous single nucleotide polymorphisms (nsSNPs) in the TLR9 gene and to delineate their deleterious effect on the structural and functional features of proteins at the molecular level. Based on the implementation of various computational tools and algorithms eight most deleterious nsSNPs (P139H, R257C, C265Y, L283P, G514D, L544Q, H566Y, and W670R) have been identified in the human TLR9 gene as potentially damaging SNPs. Further, our study suggests highly conserved patterns at deleterious nsSNPs sites could influence protein stability and its functional features. Additionally, this study identifies two nsSNPs (G514D and W670R) associated with the severity of Uterine corpus endometrial carcinoma. In support of our computational findings, the validation of key results using polymerase chain reaction and other experimental methods is warranted in the Indian population. In general, this study might be able to delineate the guideline for identifying the most damaging SNPs and enhances the understating of the risk factors for cancer and disease susceptibilities.
Introduction
Worldwide, in gynecological cancers, uterine cervix and corpus uteri (endometrial cancer) play a major role in cancer-associated mortality. In India, enhancement in cervical cancer and corpus uteri cancer cases has been seen with time (Ferlay et al. Citation2021). Recent studies indicate that the involvement of immunological responses plays a crucial role in cancer development (Singhal et al. Citation2015; Bandil et al. Citation2017). Innate immunity works as the first line of defense against antigens i.e., viruses and bacteria. Innate immune cells need to be activated in order to perform effective immune responses. Subsequently, innate immune cells rely on various types of pattern recognition receptors (PRRs) expressed intracellularly or on the cellular surface. Functionally, different PRRs recognize unique structures known as pattern-associated molecular patterns (PAMPs) located on antigen’s surfaces (Pradere et al. Citation2014). One such PRR is Toll-like receptors (TLRs) that comprise a wide range of recognition sensors to initiate the immunological response against the pathogens for quick production of inflammatory cytokines and activation of adaptive immunity (Hemmi et al. Citation2000).
In homo sapiens, based on primary sequence, TLRs are classified into 10 subclasses likewise TLR1–TLR10 (Imler and Hoffmann Citation2001). Physiologically, TLR1, TLR2, TLR4, TLR5, and TLR6 are localized at the plasma membrane, crucially identifying bacterial and mycobacterial populations. Cellularly, TLR3, and TLR7 are allocated in endolysosomes and play a key role in the detection of dsRNA and ssRNA viruses respectively (Kawai and Akira Citation2009). Additionally, TLR9 is also localized into the endolysosome and detects cytosine phosphate-guanine oligodeoxynucleotide (CpG-ODN) of bacteria and viruses (Bauer et al. Citation2001; Kumar Citation2018).
In humans, TLR9 is expressed in mast cells, platelets, T cells, innate lymphoid cells (ILCs), NK cells, neutrophils, epithelial and endothelial cells (Bauer et al. Citation2001; Krug et al. Citation2004; Akira et al. Citation2006; Pradere et al. Citation2014). The CpG motifs comprise a hexamer (RRCGYY) with centrally unmethylated CpG, where R represents purine and Y represents pyrimidine (Park et al. Citation2008). Biologically, the immunostimulatory activity of the CpG-DNA binding motif is influenced by the density of CpG motifs, its length, and amino acid sequences flanking the CpG motif (Krieg et al. Citation1995). TLR9 also comprises long insertion loop (Z-loop), where proteolytic processing occurs to perform its functional activity (Ewald et al. Citation2008; Onji et al. Citation2013). Recent studies show an association of the TLR9 in the progression of various cancer types like interactions between HPV and host immune response facilitated by TLR9 which influences the transformation to cervical cancer malignancy (Janeway and Medzhitov Citation2002; Werner et al. Citation2012; Sharma et al. Citation2019; Moura et al. Citation2022). Clinically, TLR9 is highly polymorphic and represents a crucial drug target to explicate the critical role in the development of endometrial cancer (Krieg Citation2008; Luquez et al. Citation2021).
The presence of single nucleotide polymorphism (SNP) in the TLR9 gene is responsible for amino acid substitutions causing functional changes or deleterious effects caused by respective SNP. The improper function of TLR9 could lead to inappropriate activation of the downstream immune response pathway (Lamphier et al. Citation2006). The deleterious nsSNPs in TLR9 could also change its structural features or the mutations in the promoter region disturb gene regulation (Oubounyt et al. Citation2019). The alterations in gene sequence subsequently affect the alteration of protein function such as protein charge, hydrophobicity, translation, stability, and inter/intra protein interactions leading to serious disease (Kamaraj and Purohit Citation2013; Emadi et al. Citation2020; Rozario et al. Citation2021). Accordingly, the current study is designed to identify the most deleterious nsSNPs in TLR9 and delineate their deleterious effect on the structural and functional features of proteins at the molecular level. In respect, several computational methods and tools have been used to achieve the goal of this study. Subsequently, based on the scientific background and medical importance of TLR9, the key findings of the current study are discussed with the vital role of deleterious nsSNPs in respect of cervical and endometrial cancer.
Results and discussion
Identification of most deleterious nsSNPs in human TLR9 gene sequence using various computational methods
In the TCGA database, of the total 263 SNPs associated with the human TLR9 gene sequence, 21 SNPs are 5′-UTR (8.0%), 7 SNPs are 3′-UTR (2.7%), 156 SNPs are missense (59.3%), and 79 SNPs are others (30.0%) (). In others, 67 SNPs are synonymous, 7 SNPs are stop gained, and 5 SNPs are frameshift (). Here, we have performed a consensus method with SIFT, SNAP2, Align GVGD, PolyPhen-2, PROVEAN, PhD-SNP, and p-MUT to identify the most deleterious nsSNPs from the data set of missense SNPs. Accordingly, the reported 156 missense SNPs data set has been used with every individual SNPs prediction method, and their altogether results have been manually analyzed and compared (Supplementary Table S1).
Figure 1. Three-dimensional pie-chart analysis of human TLR9. The figure shows the association of percentage missense SNPs, 5′-UTR, 3′-UTR, and other types of SNPs. The data set of correlated TLR9 SNPs is retrieved from the TCGA database. The total of retrieved 263 SNPs are comprising the human TLR9 gene sequence, where 21 SNPs are 5′-UTR (8.0%), 7 SNPs are 3′-UTR (2.7%), 156 SNPs are missense (59.3%), and 79 SNPs are others (30.0%).
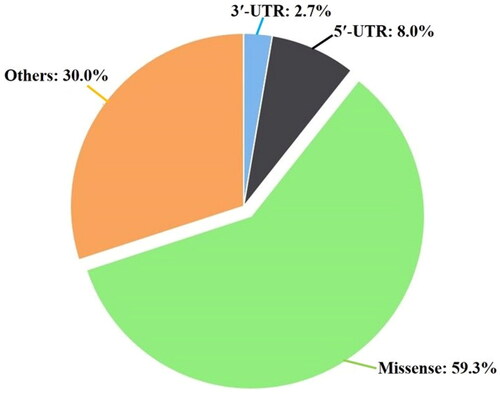
Table 1. Analyzing the most deleterious nsSNPs of human TLR9 gene using various bioinformatics tools.
Of the total 156 missense SNPs, SIFT tool identifies 62 missense SNPs as the most deleterious nsSNPs. Similarly, 40, 22, 79, 61, 63, and 22 nsSNPs have been predicted as the most deleterious nsSNPs by using SNAP2, p-LIGN-GVGD, PLYPHEN, PROVEAN, PhD-SNP, and p-MUT techniques respectively (Supplementary Table S1). Our comparative outcomes suggest that of the total 156 missense SNPs, only 8 are identified as common nsSNPs by all the techniques (). Therefore, we could argue that only eight nsSNPs could be the most deleterious and might be associated with the establishment of various cancer types and diseases (). With respect to the TLR9 protein sequence, the eight identified deleterious nsSNPs have been labeled and are named as P139H, R257C, C265F, L283P, G514D, L544Q, H566Y, and W670R (). Since the identified most deleterious sites have been replaced by the distinct nature of amino acid residues the changes at these sites might influence the structural and functional features of the TLR9 protein.
Analyzing the effect of most deleterious nsSNPs on the structural stability of TLR9 protein
The present study is aimed to identify the most deleterious nsSNPs of the TLR9 gene sequence and delineate their cumulative role in the structural and functional parameters of the protein. Accordingly, the effect of the most deleterious nsSNPs on TLR9 protein stability has been analyzed by the DynaMut2, SDM, and I-MUTANT Suite servers. The findings of the DynaMut2 and I-MUTANT server revealed DDG < 0 for seven deleterious nsSNPs (P139H, R257C, C265Y, L283P, G514D, L544Q, and W670R) suggesting destabilizing the TLR9 protein () (Rodrigues et al. 2018). However, the reverse has been seen for H566Y SNP with positive DDG = 0.41 and 0.37 respectively by both the servers, suggesting an enhancement in the protein stability (). Further, SDM server analysis for all deleterious SNPs revealed negative DDG < 0 values and a reduction in the protein stability (). Here, the comparative outcomes of DynaMut2, SDM, and I-MUTANT servers analysis suggest that point mutations might affect the conformational changes that lead to altering the protein stability.
Table 2. Identifying the effect of deleterious nsSNPs on protein stability and functional features of TLR9.
Conservation profile analysis of most deleterious nsSNPs in TLR9 by ConSurf server
Looking into insight, an evolutionarily conserved amino acid profile of most deleterious nsSNPs provides essential information to understand the possible impact of deleterious nsSNPs on the structural and functional features of the protein. The ConSurf web server identifies whether the position of every individual amino acid is exposed on the protein surface or buried inside the core protein. The outcomes of the ConSurf web server revealed six deleterious nsSNPs (P139H, R257C, L283P, G514D, L544Q, and H566Y) as evolutionary conserved ( and ). However, the other two deleterious nsSNPs C265Y and W670R are predicted to be as moderately conserved (). The two variants (G514D and H566Y) are structurally exposed whereas the other six nsSNPS (P139H, R257C, L283P, L544Q, and W670R) are buried inside the core protein (). Evolutionarily, conserved amino acid residues are known to play a vital role in crucial structural and functional features of the protein. Therefore, it is argued that mutations at highly conserved sites have a direct impact on changes in the structural and functional features of TLR9.
Figure 2. Conservation profile analysis of most deleterious nsSNPs. Figure showing the amino acid conservation profile of human TLR9 protein. Highly conserved residues are directly proportional to the density of dark red color (score varies between 6 and 9), whereas the density of blue color (score varies between 6 and 9) shows variability in respective amino acids. Identified, eight most deleterious nsSNPs sites are highlighted by red arrows.
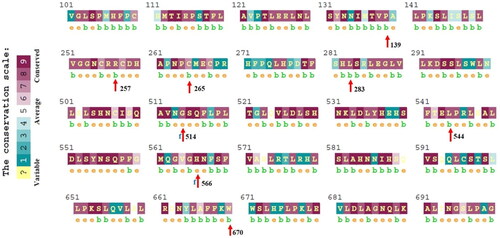
Table 3. Evolutionary conservation profile analysis of high-risk nsSNPs in TLR9.
Analyzing the effect of most deleterious nsSNPs on protein stability and solvent accessibility by NetSurfP server
The solvent accessibility and protein stability are essential physiological parameters to understand the possible impact of most deleterious nsSNPs on the functional behaviors of TLR9 protein. The NetSurfP web server characterized the cumulative impact of every individual deleterious nsSNPs in terms of exposed or buried amino acid along with solvent accessibility parameters i.e., absolute surface accessibility (ASA) and relative surface accessibility (RSA). NetSurfP predicts that five nsSNPs (P139H, C265Y, L283P, L544Q, and W670R) are buried inside the backbone of core protein; however, three (R257C, G514D, and H566Y) are exposed over the protein surface (). The RSA score of most deleterious nsSNPs varies between 0.063 ≤ RSA ≥ 0.49 and ASA score varies between 11.55 ≤ ASA ≥ 105.98. The NetSurfP results showed higher value of RSA and ASA score for three SNPs and these are exposed on protein surface i.e., R257C (RSA = 0.46, ASA= 105.98), G514D (RSA= 0.49, ASA= 39.03), and H566Y (RSA= 0.38, ASA= 45.47) (). Of the total, five most deleterious nsSNPs are buried in core protein and could be responsible for protein folding and functional changes. However, the three most predicted deleterious nsSNPs exposed on the protein surface might alter the physiological parameters of TLR9 protein i.e., protein thermostability, surface charge potential, accessible surface area, and volume. Further, based on the altogether outcomes of Consurf and NetSurfP analysis, we could argue that the identified most deleterious eight nsSNPs might have a direct impact on altering the biological and functional features of TLR9 and that could be leading to the establishment of various types of cancer and other chronic diseases.
Structural assessment analysis of most deleterious nsSNPs on TLR9 by HOPE server
Project HOPE is a web-based application used to identify the effect of amino acid substitution on the native structure of TLR9 protein, including physiological and chemical properties i.e., hydrophobicity, spatial structure, charge, size changes between wild and mutant, and protein functions (). Accordingly, HOPE indicates, of the total three amino acid residues corresponding to the most deleterious nsSNPs (P139H, G514D, and L544Q) are bigger as compared to the wild-type. However, the two most deleterious nsSNPs L283P and R257C are comparatively smaller than the wild-type. Our previous Consurf analysis suggests that the mutation site G514D is exposed on the protein surface. Additionally, the inspection of P139H and L544Q on TLR9 showed mutated residues are bigger than native residues and might not perfectly fit in the core protein. Further, at site P139H, the mutated residue is positively charged whereas the native residue is neutral, which can lead to a problem in protein folding.
Table 4. Interpreting the impact of amino acid changes on TLR9 protein structure, hydrophobicity, charge, and size by HOPE.
Interestingly, of the total, six mutants (P139H, R257C, G514D, L544Q, H566Y, and W670R) showed an increased level of hydrophobicity in mutant-type as compared to the wild-type, whereas the reverse has been observed for mutant L283P (). In addition, the charge potential differences at key point mutations (R257C, C265Y, G514D, W670R, and D683K) are also observed between the wild-type and mutant-type. The inspection at these mutation sites showed that in R257C mutation residue C is neutral, whereas wild-type residue R has a positive charge. Similarly, in the charge potential inspection of other mutants i.e., C265Y, G514D, W670R, and D683K, the mutant residues have negative, negative, positive, and positive charges, respectively, whereas wild-type residues have neutral, neutral, neutral, and negative charges, respectively. Here, our comparative analysis suggests that the distribution of hydrophobicity differences between wild-type and mutant-type residues in most deleterious nsSNPs can alter the side-chain intermolecular hydrogen bonding network within two or more neighboring residues. These changes could be leading to disrupting the mechanism of protein folding and other crucial structural differences in TLR9 protein. In general, we could suggest that above discussed crucial changes might affect the catalytic behavior of TLR9 protein, which could be leading to altering the biological role of the protein.
Protein–protein interaction analysis of TLR9 by STRING database
Globally, the STRING database is a popular tool to understand protein–protein interactions. Any variation in protein structure and function can alter its ability to interact with other molecules. Thus, the interacting neighbors of mutated protein could be leading to phenotypic effects. The investigation of the STRING database revealed that TLR9 protein is related to 10 different proteins likewise MYD88, HMGB1, UNC93B1, TIRAP, TICAM1, PLSCR1, IL6, IL1B, TICAM2, and TLR2 (). Our findings of the STRING database have been associated with some of the previously reported experimental studies where the interactions of TLR9 with other protein molecules are well known (Takeda and Akira Citation2015; Han et al. Citation2018; Kim et al. Citation2020; Dong et al. Citation2022).
Figure 3. Protein–protein interactions of TLR9 protein. The figure shows protein–protein interactions of TLR9 protein with its related proteins MYD88, HMGB1, UNC93B1, TIRAP, TICAM1, PLSCR1, IL6, IL1B, TICAM2, and TLR2. The interaction network has been generated by the STRING database. A brief introduction about all interacting partners of TLR9 has been also highlighted below in the network diagram.
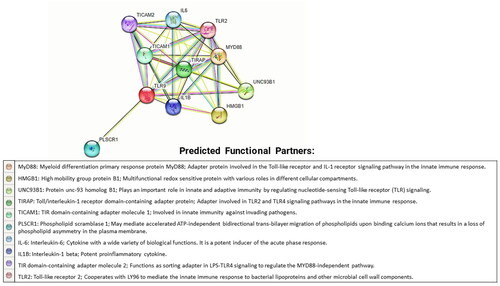
Three-dimensional structural assessment analysis of TLR9 protein
Three-dimensional structural assessment analysis of human TLR9 protein is performed to understand the effect of most deleterious nsSNPs on structural features of wild-type versus mutant-type. The 3D coordinates of wild-type and mutant-type TLR9 are predicted using Modeller10.1 and TLR9 from Equus caballus (PDB ID: 3WPB) as templates (Ohto et al. Citation2015). Ramachandran plot analysis showed that for each structure > 99% of residues have been falling in the favorable region of the Ramachandran plot (data not shown).
Structural superimposition of wild-type and mutant-type suggested that the protein backbone of both structures is aligning perfectly with each other; however, deviations are noted among all points of mutation (). Further, it has been suggested that mutation sites identified as buried inside the core protein, might alter intermolecular hydrogen bonding with their adjacent amino acids. This might be led to altering the protein folding mechanism of TLR9 and might alter interactions between TLR9 and ligand molecules leading to affecting its catalytic processes. Additionally, mutation sites identified at the protein surface of TLR9 affect the protein–protein interactions network with surrounding proteins leading to the phenotype effect. Therefore, our comparative structural assessment analysis of wild versus mutant TLR9 suggests that the positional deviations at mutation sites could lead to structural and functional changes among the mutant-type.
Figure 4. Structural alignment of wild and mutant-type TLR9 proteins: The C-alpha atoms structural superimposition of wild-type (sky blue color) and mutant-type (sand color) TLR9 proteins has been shown in cartoon format. All sites have been shown in green and red color sticks for wild and mutant-type, respectively. The figure shows that both structures are aligning perfectly where slight deviations are noted among all points of mutations. The overall structural alignment shows the very close C-alpha atom’s root mean square deviation (RMSD) of 0.064 Å between the two structures.
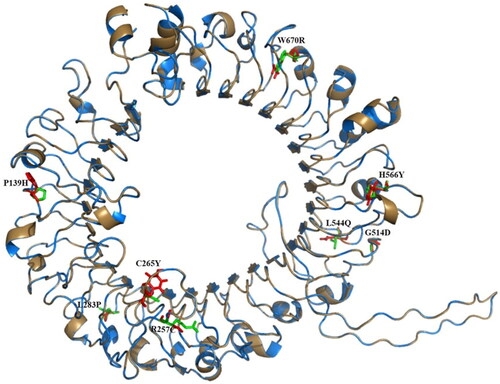
Amino acid network analysis for identifying the effect of mutation on noncovalent intra-molecular interactions of TLR9 protein using the RING 3.0 server
Comparative amino acid network (AAN) analyses for wild and mutant TLR9 proteins have been performed in order to identify the impact of point mutations on noncovalent intramolecular interactions (). The outcomes of the RING 3.0 server suggest changes in the degree of nodes at the point of mutations (). Accordingly, we observed the degree of nodes as 0, 4, 4, 3, 1, 6, and 6 for the amino acids P139, R257, C265, L283, G514, L544, H566, and W670 respectively of the wild TLR9 protein. Whereas the degree of nodes at the points of mutations P139H, R257C, C265Y, L283P, G514D, L544Q, H566Y, and W670R are observed as 5, 3, 7, 2, 4, 6, 7, 6, and 5 respectively for the mutant TLR9 protein (). The altogether outcomes of AAN for wild and mutant TLR9 protein suggests the direct impact of point mutations on various types of noncovalent intramolecular interactions ( and ). Accordingly, we observed 458 and 514 intra-molecular H-bonds for wild and mutant TLR9 protein, respectively (). The higher density of π-π stacking, π-cation, and van der Waal interactions have been observed for mutant as compared to the wild TLR9 protein (). However, three ionic interactions are observed to be conserved in both cases, and eight and seven disulfide bridges have been observed for the wild and mutant TLR9 protein, respectively (). Further, the density of van der Waals forces for the wild and mutant TLR9 protein is observed as 774 and 812, respectively (). Interestingly, we observed the total of noncovalent intramolecular binding interactions as 1277 and 1372 for the wild and mutant TLR9 protein, respectively (). Since our results showed a higher density of noncovalent intramolecular binding interactions for the mutant TLR9 as compared to the wild TLR9, it is suggested that point mutations directly affect the noncovalent intramolecular interactions network of TLR9 protein. The point mutations in TLR9 protein enhance the overall noncovalent intramolecular binding interactions, which might stabilize the structural scaffold of mutant TLR9 protein as compared to the wild case. Further, based on the detailed finding of our analysis we can suggest that the variability in the degree of nodes results in the alteration of the protein conformation leading to changes in protein folding. In general, we can suggest structural changes in TLR9 proteins resulting in variabilities of biophysical and functional features led by point mutations (Yan et al. Citation2014).
Figure 5. Amino acid network (AAN) analysis of wild-type and mutant-type TLR9 proteins. The figure shows comparative AAN analyses for wild and mutant TLR9 proteins. The nodes of interest (point of mutations) have been labeled and highlighted by black circles. The size of solid spheres is directly proportional to the density of the degree of nodes. The various types of noncovalent bonded intramolecular contacts have been shown by lines. The colors of nodes are varying according to the nature of the amino acids (Supplementary Figure S1). (A) The figure shows AAN analysis for the wild-type TLR9 protein. For wild-case TLR9, only seven nodes have been highlighted because an amino acid P139 does not have any degree of nodes. (B) The figure shows AAN analysis for the mutant-type TLR9 protein.
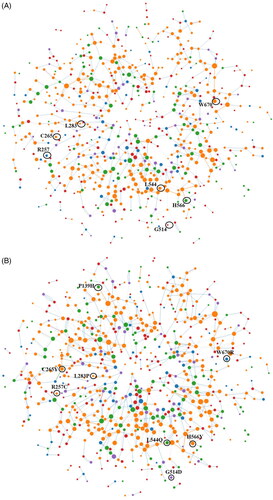
Table 5. Detailed intramolecular noncovalent interactions analysis of wild and mutant TLR9 protein by using online available Ring server.
Association of the damaging nsSNPs with cancer
The cBioPortal and canSAR Black web server are used to identify the cancer-specific nsSNPs. The comparative outcomes of both tools identify two nsSNPs G514D and W670R that are associated with uterine corpus endometrial carcinoma (UCEC). However, none of the nsSNPs are found to be associated with cervical adenocarcinoma (CESC). Further, based on these findings we could suggest that tissue specificity of mutations in canSAR black data at two crucial positions 514 and 670 in TLR9 may result in moderate severity in UCEC.
Analyzing the gene expression profile for TLR9 gene in association with cancer
GEPIA2 database has been used to perform box plot analysis of TLR9 gene with respect to identifying the differential gene expression associated with cancer types. The box plot analysis revealed that CESC arises due to underexpression of TLR9, whereas UCEC results due to the overexpression of the TLR9 gene (). Our results suggest that the majority of cancers occur due to the downregulation of TLR9; alternatively, few are resulted by the upregulation of TLR9. A similar finding has been already confirmed by some previous experimental studies where the downregulation of the TLR9 expression is associated with the establishment of oral cancer (Han et al. Citation2018). Further, our finding has been also strengthened by a study where a population of chronic HBV carriers showed suppressed expression and function of TLR9, assisting in immune escape strategy for HBV (Tout et al. Citation2018).
Figure 6. Gene expression analysis of TLR9. Figure showing detailed Box plot analysis diagram of the TLR9 gene expression in the case of cervical squamous cell carcinoma (CESC) and uterine corpus endometrial carcinoma (UCEC) for both tumor (red color box) and normal (dark grey color box) samples. The analysis showed that UCEC shows the overexpression of the TLR9 gene, whereas underexpression of the gene is observed in CESC.
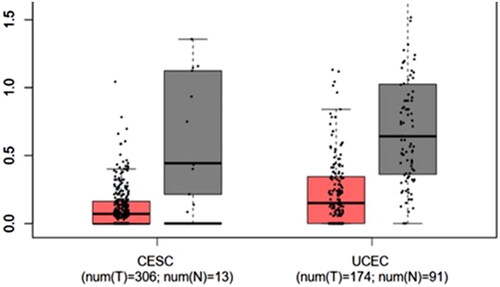
Cumulative TLR9 gene expression and survival analysis in CESC and UCEC patients
The online available GEPIA database has been used to perform detailed survival analyses of patients affected by CESC and UCEC. Depending on the median TLR9 gene expression level, patients are categorized into two groups namely high or low expression level groups. Our results suggest that the abnormal expression of the TLR9 gene is involved in the poor prognosis of patients in association with CESC and UCEC. Additionally, the patients associated with CESC showed long but similar survival time (approximately 200 months) in both under and overexpression of the TLR9 gene (). However, patients associated with UCEC showed a shorter survival time (approximately 125 months) under expressed conditions of the TLR9 gene, whereas a comparatively longer survival time (approximately 140 months) has been observed in the overexpression of the TLR9 gene (). The TLR9 gene expression and survival analysis in CESC and UCEC patients suggest the clinical role of TLR9 in the association of various types of cancers.
Figure 7. Patient’s time-dependent percentile survival rate analysis. The figure shows the overall percent survival rate of the patient for both (A) cervical squamous cell carcinoma (CESC) and (B) uterine corpus endometrial carcinoma (UCEC). The blue color represents low expression and the red color represents the high expression of TLR9. The patients associated with CESC showed longer but similar survival time (approximately 200 months) in both under and overexpression of the TLR9 gene. The patients associated with UCEC showed a shorter survival time (approximately 125 months) during underexpressed conditions, whereas comparatively longer survival time (approximately 140 months) during the overexpression of the TLR9 gene.
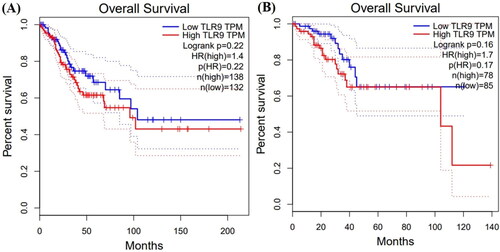
Identifying the effect of most deleterious nsSNPs and expression of TLR9 gene with their neighboring genes
The muTarget tool identifies the effect of mutation on gene expression and gives an expression plot. Here, in the current hypothesis we have used two independent analyses i.e., ‘Genotype’ and ‘Target’ for the TLR9 gene to predict the detailed role of the mutant gene on its expression. In this respect, we performed a ‘Genotype’ run for the TLR9 mutant gene. For uterine cancer, the outcomes of the ‘Genotype’ hypothesis revealed the alteration in the expression of MKNK2, MED18, AEN, C19orf25, OSGIN1, and other genes (). However, cervical cancer mutation in TLR9 could not alter the expression of neighboring genes. Next, we performed a ‘Target’ run by selecting TLR9 as the target gene for both cervical and uterine cancers. Our results showed gene alteration for cervical cancer (SOS1, NMT2, EPB41L3, and PZP) where a higher expression is observed for the MUC4 gene (). Similarly, for uterine cancer the expression of TLR9 can be influenced by a mutation in various genes i.e., TP53, AGO1, GRM8, SCAF4, and MROH8 (). Interestingly, we observed that gene TP53 showed a maximum change in gene expression for uterine cancer as compared to other counterparts’ genes. The comparative outcomes of our muTarget analysis suggest that the expression of various genes has been affected by point mutations in the TLR9 gene. In this respect, with regard to the case of uterine cancer, the expression of MKNK2, MED18, AEN, C19orf25, and OSGIN1 genes have been altered along with mutations in the TLR9 gene (detailed about potential genes are incorporated in Supplementary Data S2).
Figure 8. Genotype analysis of TLR9 gene. The figure represents a genotype run analysis to identify the changes in the TLR9 gene expression for uterine cancer. The figure shows that the expression of MKNK2, MED18, AEN, C19orf25, OSGIN1, etc. neighboring genes in uterine cancer is downregulated in presence of the mutated TLR9 gene (red) and normal expression in presence of wild-type (green) TLR9 gene expression.
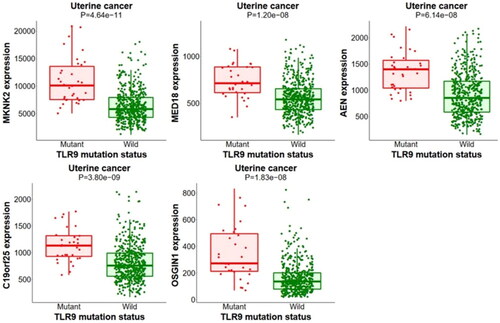
Figure 9. Target run analysis of TLR9. Target run analysis is performed to identify mutations in crucial genes that can alter the expression of the TLR9 gene. (A) Highlighted mutations in significant genes belong to cervical cancer. The figure shows the downregulation of the TLR9 gene in presence of mutated SOS1, NMT2, EPB41L3, and PZP and MUC4 genes in cervical cancer. (B) Highlighted mutations in significant genes belong to uterine cancer. The figure shows the expression of the TLR9 gene is downregulated by a mutation in different genes i.e., AGO1, GRM8, SCAF4, and MROH8 except for mutated TP53 where the TLR9 expression is upregulated.
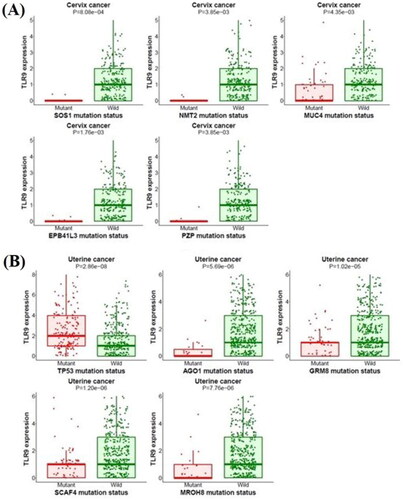
In the current study, we used TLR9 as the target gene where we found that its expression is changed by numerous mutated genes. Accordingly, the tool predicts the mutations in SOS1, NMT2, EPB41L3, and PZP genes, which might change the gene expression of TLR9 in cervical cancer patients. Further, in a uterine cancer patient, mutations in TP53, AGO1, GRM8, SCAF4, and MROH8 genes change the gene expression of TLR9 (details about potential genes are incorporated in Supplementary Data S2). Since all identified genes are known to play a crucial role in various types of metabolic pathways and biological processes, mutations in these key genes could enhance the possibility to cause various types of cancers. Accordingly, based on the comparative outcomes of muTarget analysis, it is suggested that mutations in any interacting gene partners in the gene-gene network also affect their neighboring genes, which could lead to cancer formation.
TLR9 are significant molecules that recognize and interact with DNA viruses. The presence of missense SNP in a gene responsible for amino acid substitutions causes functional changes or deleterious effects in the protein. The improper function of the TLR9 protein could further lead to inappropriate activation of the downstream immune pathway.
Here, we present the first systematic and exhaustive study using various in silico approaches leading to the identification of the most deleterious nsSNPs in the TLR9 gene. With the help of various computational tools and algorithms, we reported the eight most deleterious nsSNPs (P139H, R257C, C265Y, L283P, G514D, L544Q, H566Y, and W670R) in the TLR9 gene. The deleterious nsSNPs at highly conservation sites act as potentially damaging SNPs and could influence protein stability and its functional features. Further, our study identifies two crucial nsSNPs G514D and W670R, specifically associated with the severity of UCEC. Further, the key findings of the current study have been strengthened by some similar experimental studies (as discussed below) where the clinical roles of a few SNPs related to the TLR9 gene are highlighted.
Several previous studies suggest that the presence of SNPs led to hampering of the DNA recognition ability of the TLR9 receptor. In this respect, few of the reports highlighted the medical importance of TLR9 where its disease susceptibility and role are affected by the presence of SNPs. In a Swedish study, the clinical role of TLR9 SNPs (A1635G and G1174A) has been discussed where these SNPs are found to be associated with HIV-1 infection (Bochud et al. Citation2007). Another study showed that the TC genotype at locus -1237 T/C (rs5743836) of TLR9 in mononuclear cells gets upregulated expression and promiscuous response towards the CpG along with profound IL6 and B-cell proliferation (Carvalho et al. Citation2011). Similar SNP has been studied in Brazilian women to find the placental infection, where the presence of the C allele decreased the infection of human cytomegalovirus and another study showed a higher frequency of allele with allergic bronchopulmonary aspergillosis (Carvalho et al. Citation2008; Sánchez et al. Citation2021). A recent study on cervical cancer and cervicitis in India showed a decreased risk of HPV16 infection with three SNPs – 1486 T/C(rs187084), 2848 G/A (rs352140), and 1174 A/G (rs352139) of the TLR9 gene (Pandey et al. Citation2021). Similarly, a study on Han Chinese women with HPV16 infection carrying GA/AA genotype of G2848A (rs352140) showed an increased risk of cervical cancer as compared to women carrying GG genotype (Jin et al. 2017). A simultaneous contradictory meta-genomic study on rs352140 SNP of TLR9 gene with vulnerability to cervical carcinoma showed no association with the disease (Parsaeian et al. Citation2021).
Based on our outcomes and in association with the previous experimental reports highlighting the clinical role of SNPs in TLR9, we could conclude that this is the first systematic study to identify the possible deleterious role of nsSNPs in the TLR9 gene related to cancer. Therefore, the present study might be able to delineate the guidelines for identifying the most damaging SNPs and predicting the risk factors for cancer and disease susceptibilities. The current study has been focused on the most deleterious nsSNPs associated with endometrial and cervical cancer; however, the role of these SNPs could also be checked for other severe diseases. The 3D coordinates for human TLR9 proteins have not been solved by any experimental methods; therefore, the modeled structures have been used for detailed structural assessment analyses that might also slightly fluctuate the accuracy of our concluding remarks. Although, the findings of this study have been calculated by using various popular tools and algorithms, however, an extensive experimental and large-population-based study along with clinical trials are needed before implementing the key findings of this study for clinical purposes.
Materials and methods
The protein sequence of human TLR9 (Q9NR96) has been retrieved from the UniProtKB database and its related TLR9 gene has been retrieved from the NCBI database. The TLR9 gene-related 263 somatic mutations reported SNPs retrieved from the GDC portal of the TCGA database (https://www.cancer.gov/tcga). In the current study, we have used various bioinformatics tools and algorithms (SIFT, SNAP2.0, PROVEAN, and PhD-SNP) to identify the most deleterious nsSNPs. The results of these tools analyze the phenotypic effect of amino acid changes on protein functions as neutral/tolerated or deleterious/effect based on the score (Bromberg and Rost Citation2007; Sim et al. Citation2012) (Supplementary Data S1). Align GVGD works based on multiple sequence alignment and biophysical properties of protein residues where score C15 is less likely to affect and C65 is more likely to affect the protein function (Tavtigian et al. Citation2006). PolyPhen-2 identifies the effect of amino acid substitution on structural and functional features of protein as neutral, possibly damaging, or probably damaging (Ramensky et al. Citation2002). PMut tool is a neural network-based classifier that annotates pathological mutations on protein from 0–0.5 (neutral) to 0.5–1 (pathological) (Costa et al. Citation2005). Additionally, PROVEAN is also used to identify the effect of respective nsSNPs as deleterious or neutral. If the retrieved score is ≤ 2.5, then it is treated as a deleterious effect or otherwise as a neutral effect (Choi et al. 2002). Subsequently, PhD-SNP has been used to identify the role of respective nsSNPs as neutral or deleterious in homo sapiens related to disease formation (Capriotti et al. Citation2006). Next, DynaMut2, SDM, and I-Mutant Suite are employed to identify the impact of nsSNPs on protein stability based on a free energy change value (DDG) (Capriotti et al. Citation2005; Dehury et al. Citation2019, Citation2017, Citation2020; Pandurangan et al. Citation2017; Rodrigues et al. Citation2021). The Consurf server identifies the conservation profile of mutated residues at the nsSNP positions in protein molecules, which are represented in a color scheme (Berezin et al. Citation2004). Further, NetSurfP-2.0 predicts the secondary structure, solvent, and surface accessibility (Klausen et al. Citation2019). Further, HOPE is used to find respective functions such as changes in hydrophobicity, size, charge, etc. of mutation on the protein (Venselaar et al. Citation2010). Additionally, the STRING database has been used to predict related partners of the TLR9 gene (Jensen et al. Citation2009).
A suitable template of Homo Sapiens TLR9 for homology modeling has been searched by using PSI-blast against the PDB database. Further, the popular biomolecular modeling software Modeller10.1 has been used for homology modeling of wild and mutant proteins (Webb and Sali Citation2016). Qualitative analysis, refinement, and structural validation of each modeled structure have been done by using the SAVES server and ProSAweb-based tool (Wiederstein and Sippl Citation2007). Further, PyMOL molecular visualizations tool (pymol.org) has been used for molecular visualizations and structural superimposition (Schrödinger and DeLano Citation2020). Additionally, the RING (Residue Interaction Network Generator) 3.0 server has been used to identify noncovalent interactions at the atomic level in protein structures based on geometrical parameters (Clementel et al. Citation2022).
The present study is designed to identify and delineate the critical role of specific nsSNPs, which could be associated with endometrial and cervical cancer. In this respect, cBioPortal and canSAR Black tools have been used to access cancer genomics data and identify specific nsSNPs associated with cancer (Cerami et al. Citation2012). Further, GEPIA has been used to correlate gene expression and the overall survival rate (Tang et al. Citation2019). Next, we have used the muTarget tool for analyzing the correlation of gene expression (Genotype and Target run) and mutation based on the TCGA data (Nagy and Győrffy Citation2021) (detailed methodology has been given as in Supplementary Data S1).
Ethical approval
This article does not contain any studies with human participants or animals performed by any of the authors.
Authors’ contributions
Experiments, data curation, data analysis, literature review, and writing original draft: H.G.; data curation, data analysis, literature review, and writing original draft: V.V.V.; supervision, review, and editing: S.A.H.; conceptualization, supervision, investigation, project administration, funding acquisition, data interpretation, validation, review, and editing: M.B.
Abbreviations | ||
CpG | = | cytosine-phosphate-guanine |
TLRs | = | Toll-like receptors |
nsSNPs | = | Nonsynonymous single nucleotide polymorphisms |
SIFT | = | Sorting Intolerant from Tolerant |
SNAP2.0 | = | Screening for non-acceptable Polymorphism v2 |
PROVEAN | = | Protein variation effect analyzer |
PhD-SNP | = | Predictor of human deleterious single nucleotide polymorphism |
HOPE | = | Have (y) Our Protein Explained |
STRING database | = | Search Tool for the Retrieval of Interacting Genes/Proteins |
PDB | = | Protein Data Bank |
TCGA | = | The Cancer Genome Atlas |
GDC | = | Genomic Data Commons |
RING | = | Residue Interaction Network Generator |
Supplemental Material
Download MS Word (39.4 KB)Supplemental Material
Download JPEG Image (50.9 KB)Supplemental Material
Download MS Word (20.3 KB)Supplemental Material
Download MS Word (18.5 KB)Acknowledgments
Heena Gautam acknowledges the Council of Scientific and Industrial Research, India 09/1173(001)/2017-EMR/I for financial assistance as a Senior Research Fellowship. Ved Vrat Verma acknowledges the Indian Council of Medical Research, India, BMI/11(27)/2020 for financial assistance as a Research Associate Fellowship.
Disclosure statement
No potential conflict of interest was reported by the author(s).
Data availability statement
The details of third-party software and databases used in this study are provided in Supplementary Table S1.
References
- Akira S, Uematsu S, Takeuchi O. 2006. Pathogen recognition and innate immunity. Cell. 124(4):783–801.
- Bandil K, Singhal P, Dogra A, Rawal SK, Doval DC, Varshney AK, Bharadwaj M. 2017. Association of SNPs/haplotypes in promoter of TNF A and IL-10 gene together with lifestyle factors in prostate cancer progression in Indian population. Inflamm Res. 66(12):1085–1097.
- Bauer S, Kirschning CJ, Häcker H, Redecke V, Hausmann S, Akira S, Wagner H, Lipford GB. 2001. Human TLR9 confers responsiveness to bacterial DNA via species specific CpG motif recognition. Proc Natl Acad Sci USA. 98(16):9237–9242.
- Berezin C, Glaser F, Rosenberg J, Paz I, Pupko T, Fariselli P, Casadio R, Tal NB. 2004. ConSeq: the identification of functionally and structurally important residues in protein sequences. Bioinformatics. 20(8):1322–1324.
- Bochud PY, Hersberger M, Taffé P, Bochud M, Stein CM, Rodrigues SD, Calandra T, Francioli P, Telenti A, Speck RF, et al. 2007. Polymorphisms in Toll-like receptor 9 influence the clinical course of HIV-1 infection. AIDS. 21(4):441–446.
- Bromberg Y, Rost B. 2007. SNAP: predict effect of non-synonymous polymorphisms on function. Nucleic Acids Res. 35(11):3823–3835.
- Capriotti E, Calabrese R, Casadio R. 2006. Predicting the insurgence of human genetic diseases associated to single point protein mutations with support vector machines and evolutionary information. Bioinformatics. 22(22):2729–2734.
- Capriotti E, Fariselli P, Casadio R. 2005. I-Mutant2.0: predicting stability changes upon mutation from the protein sequence or structure. Nucleic Acids Res. 33:W306–310.
- Carvalho A, Osório NS, Saraiva M, Cunha C, Almeida AJ, Teixeira-Coelho M, Ludovico P, Pedrosa J, Pitzurra L, Aversa F, et al. 2011. The C allele of rs5743836 polymorphism in the human TLR9 promoter links IL-6 and TLR9 up-regulation and confers increased B-cell proliferation. PLoS One. 6(11):e28256.
- Carvalho A, Pasqualotto AC, Pitzurra L, Romani L, Denning DW, Rodrigues F. 2008. Polymorphisms in toll-like receptor genes and susceptibility to pulmonary aspergillosis. J Infect Dis. 197(4):618–621.
- Cerami E, Gao J, Dogrusoz U, Gross BE, Sumer SO, Aksoy BA, Jacobsen A, Byrne CJ, Heuer ML, Larsson E, et al. 2012. The cBio cancer genomics portal: an open platform for exploring multidimensional cancer genomics data. Cancer Discov. 2(5):401–404.
- Choi Y, Chan AP. 2015. PROVEAN web server: a tool to predict the functional effect of amino acid substitutions and indels. Bioinformatics. 31(16):2745–2747.
- Clementel D, Del Conte A, Monzon AM, Camagni GF, Minervini G, Piovesan D, Tosatto SCE. 2022. RING 3.0: fast generation of probabilistic residue interaction networks from structural ensembles. Nucleic Acids Res. 50(W1):W651–W656.
- Costa CF, Gelpí JL, Zamakola L, Parraga I, Cruz XDL, Orozco M. 2005. PMUT: a web-based tool for the annotation of pathological mutations on proteins. Bioinformatics. 21(14):3176–3178.
- Dehury B, Behera SK, Negi S. 2017. Overcoming the limitation of GWAS platforms using systems biology approach. CBIO. 12(2):156–170.
- Dehury B, Tang N, Blundell TL, Kepp KP. 2019. Structure and dynamics of γ-secretase with presenilin 2 compared to presenilin 1. RSC Adv. 9(36):20901–20916.
- Dehury B, Tang N, Kepp KP. 2020. Insights into membrane-bound presenilin 2 from all-atom molecular dynamics simulations. J Biomol Struct Dyn. 38(11):3196–3210.
- Dehury B, Tang N, Mehra R, Blundell TL, Kepp KP. 2020. Side-by-side comparison of Notch- and C83 binding to γ-secretase in a complete membrane model at physiological temperature. RSC Adv. 10(52):31215–31232.
- Dong X, Han X, Zhang X, Li S, Li Z, Kang J, Jiang J, Ni S, Lu L, He Z, et al. 2022. A simplified herbal formula improves cardiac function and reduces inflammation in mice through the TLR-mediated NF-κB signaling pathway. Front Pharmacol. 13:865614.
- Emadi E, Akhoundi F, Kalantar SM, Baygi ME. 2020. Predicting the most deleterious missense nsSNPs of the protein isoforms of the human HLA-G gene and in silico evaluation of their structural and functional consequences. BMC Genet. 21(1):94.
- Ewald SE, Lee BL, Lau L, Wickliffe KE, Shi GP, Chapman HA, Barton GM. 2008. The ectodomain of Toll-like receptor 9 is cleaved to generate a functional receptor. Nature. 456(7222):658–662.
- Ferlay J, Colombet M, Soerjomataram I, Parkin DM, Piñeros M, Znaor A, Bray F. 2021. Cancer statistics for the year 2020: an overview. Int J Cancer.
- Han N, Zhang Z, Jv H, Hu J, Ruan M, Zhang C. 2018. Culture supernatants of oral cancer cells induce impaired IFN-α production of pDCs partly through the down-regulation of TLR-9 expression. Arch Oral Biol. 93:141–148.
- Hemmi H, Takeuchi O, Kawai T, Kaisho T, Sato S, Sanjo H, Matsumoto M, Hoshino K, Wagner H, Takeda K, et al. 2000. A Toll-like receptor recognizes bacterial DNA. Nature. 408(6813):740–745.
- Honda K, Yanai H, Mizutani T, Negishi H, Shimada N, Suzuki N, Ohba Y, Takaoka A, Yeh WC, Taniguchi T. 2004. Role of a transductional-transcriptional processor complex involving MyD88 and IRF-7 in Toll-like receptor signaling. Proc Natl Acad Sci USA. 101(43):15416–15421.
- Imler JL, Hoffmann JA. 2001. Toll receptors in innate immunity. Trends Cell Biol. 11(7):304–311.
- Janeway CA, Jr, Medzhitov R. 2002. Innate immune recognition. Annu Rev Immunol. 20:197–216.
- Jensen LJ, Kuhn M, Stark M, Chaffron S, Creevey C, Muller J, Doerks T, Julien P, Roth A, Simonovic M, et al. 2009. STRING 8–a global view on proteins and their functional interactions in 630 organisms. Nucleic Acids Res. 37:D412–416.
- Kamaraj B, Purohit R. 2013. In silico screening and molecular dynamics simulation of disease-associated nsSNP in TYRP1 gene and its structural consequences in OCA3. Biomed Res Int. 2013:697051.
- Kawai T, Akira S. 2009. The roles of TLRs, RLRs and NLRs in pathogen recognition. Int Immunol. 21(4):317–337.
- Kim SK, Park KY, Choe JY. 2020. Toll-like receptor 9 is involved in NLRP3 inflammasome activation and IL-1β production through monosodium urate-induced mitochondrial DNA. Inflammation. 43(6):2301–2311.
- Klausen MS, Jespersen MC, Nielsen H, Jensen KK, Jurtz VI, Sønderby CK, Sommer MOA, Winther O, Nielsen M, Petersen B, et al. 2019. NetSurfP-2.0: improved prediction of protein structural features by integrated deep learning. Proteins. 87(6):520–527.
- Krieg A. 2008. Toll-like receptor 9 (TLR9) agonists in the treatment of cancer. Oncogene. 27(2):161–167.
- Krieg AM, Yi AK, Matson S, Waldschmidt TJ, Bishop GA, Teasdale R, Koretzky GA, Klinman DM. 1995. CpG motifs in bacterial DNA trigger direct B-cell activation. Nature. 374(6522):546–549.
- Krug A, French AR, Barchet W, Fischer JA, Dzionek A, Pingel JT, Orihuela MM, Akira S, Yokoyama WM, Colonna M. 2004. TLR9-dependent recognition of MCMV by IPC and DC generates coordinated cytokine responses that activate antiviral NK cell function. Immunity. 21(1):107–119.
- Kumar V. 2018. Toll-like receptors in immunity and inflammatory diseases: past, present, and future. Int Immunopharmacol. 59:391–412.
- Lamphier MS, Sirois CM, Verma A, Golenbock DT, Latz E. 2006. TLR9 and the recognition of self and non-self-nucleic acids. Ann NY Acad Sci. 1082:31–43.
- Luquez KS, Schadock IC, Gonçalves CV, Tornatore M, Jardim FF, Avila EC, Soares MA, de Martínez AMB, Ellwanger JH, Chies JAB, et al. 2021. Impact of TLR7 and TLR9 polymorphisms on susceptibility to placental infections and pregnancy complications. J Reprod Immunol. 146:103342.
- Moura EL, Santos IFD, Freitas PP, Silva DMD, Santos ACMD, Lira Neto AB, Silva ACPE, Barbosa NR, Nascimento CA, Balliano TL, et al. 2022. Polymorphisms in Toll-like receptors genes changes the host’s immune response and is associated with cervical cancer. Immunobiology. 227(2):152187.
- Nagy Á, Győrffy B. 2021. muTarget: a platform linking gene expression changes and mutation status in solid tumors. Int J Cancer. 148(2):502–511.
- Ohto U, Shibata T, Tanji H, Ishida H, Krayukhina E, Uchiyama S, Miyake K, Shimizu T. 2015. Structural basis of CpG and inhibitory DNA recognition by Toll-like receptor 9. Nature. 520(7549):702–705.
- Onji M, Kanno A, Saitoh SI, Fukui R, Motoi Y, Shibata T, Matsumoto F, Lamichhane A, Sato S, Kiyono H, et al. 2013. An essential role for the N-terminal fragment of Toll-like receptor 9 in DNA sensing. Nature Commun. 4:1949.
- Oubounyt M, Louadi Z, Tayara H, Chong KT. 2019. DeePromoter: robust promoter predictor using deep learning. Front Genet. 10:286.
- Pandey N, Chauhan A, Raithatha N, Patel P, Khandelwal R, Desai A, Choxi Y, Kapadia R, Jain N. 2021. Influence of TLR4 and TLR9 polymorphisms and haplotypes on multiple hrHPV infections and HPV16 copy number in cervical cancer and cervicitis. Microb Pathog. 159:105149.
- Pandurangan AP, Montano BO, Ascher DB, Blundell TL. 2017. SDM: a server for predicting effects of mutations on protein stability. Nucleic Acids Res. 45(W1):W229–W235.
- Park B, Brinkmann MM, Spooner E, Lee CC, Kim YM, Ploegh HL. 2008. Proteolytic cleavage in an endolysosomal compartment is required for activation of Toll-like receptor 9. Nat Immunol. 9(12):1407–1414.
- Parsaeian SF, Asadian F, Karimi ZM, Setayesh S, Javaheri A, Tabatabaie RS, Dastgheib SA, Golestanpour H, Neamatzadeh H. 2021. A meta-analysis for association of XRCC3 rs861539, MTHFR rs1801133, IL-6 rs1800795, IL-12B rs3212227, TNF-α rs1800629, and TLR9 rs352140 polymorphisms with susceptibility to cervical carcinoma. Asian Pac J Cancer Prev. 22(11):3419–3431.
- Pradere JP, Dapito DH, Schwabe RF. 2014. The Yin and Yang of Toll-like receptors in cancer. Oncogene. 33(27):3485–3495.
- Ramensky V, Bork P, Sunyaev S. 2002. Human non-synonymous SNPs: server and survey. Nucleic Acids Res. 30(17):3894–3900.
- Rodrigues CHM, Pires DEV, Ascher DB. 2021. DynaMut2: assessing changes in stability and flexibility upon single and multiple point missense mutations. Protein Sci. 30(1):60–69.
- Rozario LT, Sharker T, Nila TA. 2021. In silico analysis of deleterious SNPs of human MTUS1 gene and their impacts on subsequent protein structure and function. PLoS One. 16(6):e0252932.
- Sánchez LK, Schadock IC, Gonçalves CV, Tornatore M, Finger JF, Avila EC, Soares MA, de Martínez AMB, Ellwanger JH, Chies JAB, et al. 2021. Impact of TLR7 and TLR9 polymorphisms on susceptibility to placental infections and pregnancy complications. J Reprod Immunol. 146:103342.
- Schrödinger L, DeLano W. 2020. PyMOL. http://www.pymol.org/pymol
- Sharma U, Singhal P, Bandil K, Patle R, Kumar A, Neyaz K, Bose S, Dewan AK, Mehrotra R, Sharma V, et al. 2019. Genetic variations of TLRs and their association with HPV/EBV, co-infection along with nicotine exposure in the development of premalignant/malignant lesions of the oral cavity in Indian population. Cancer Epidemiol. 61:38–49.
- Sim NL, Kumar P, Hu J, Henikoff S, Schneider G, Ng PC. 2012. SIFT web server: predicting effects of amino acid substitutions on proteins. Nucleic Acids Res. 40:W452–457.
- Singhal P, Kumar A, Bharadwaj S, Hussain S, Bharadwaj M. 2015. Association of IL-10 GTC haplotype with serum level and HPV infection in the development of cervical carcinoma. Tumour Biol. 36(4):2287–2298.
- Takeda K, Akira S. 2015. Toll-like receptors. Curr Protoc Immunol. 109:14.12.1–14.12.10.
- Tang Z, Kang B, Li C, Chen T, Zhang Z. 2019. GEPIA2: an enhanced web server for large-scale expression profiling and interactive analysis. Nucleic Acids Res. 47(W1):W556–W560.
- Tavtigian SV, Deffenbaugh AM, Yin L, Judkins T, Scholl T, Samollow PB, Silva DD, Zharkikh A, Thomas A. 2006. Comprehensive statistical study of 452 BRCA1 missense substitutions with classification of eight recurrent substitutions as neutral. J Med Genet. 43(4):295–305.
- Tout I, Gomes M, Ainouze M, Marotel M, Pecoul T, Durantel D, Vaccarella S, Dubois B, Loustaud-Ratti V, Walzer T, et al. 2018. Hepatitis B virus blocks the CRE/CREB complex and prevents TLR9 transcription and function in human B cells. J Immunol. 201(8):2331–2344.
- Venselaar H, Beek TAT, Kuipers RK, Hekkelman ML, Vriend G. 2010. Protein structure analysis of mutations causing inheritable diseases. An e-Science approach with life scientist friendly interfaces. BMC Bioinformatics. 11:548.
- Webb B, Sali A. 2016. Comparative protein structure modeling using MODELLER. Curr Protoc Protein Sci. 86:2.9.1–2.9.37.
- Werner J, Decarlo CA, Escott N, Zehbe I, Ulanova M. 2012. Expression of integrins and Toll-like receptors in cervical cancer: effect of infectious agents. Innate Immun. 18(1):55–69.
- Wiederstein M, Sippl MJ. 2007. ProSA-web: interactive web service for the recognition of errors in three-dimensional structures of proteins. Nucleic Acids Res. 35:W407–410.
- Yan W, Zhou J, Sun M, Chen J, Hu G, Shen B. 2014. The construction of an amino acid network for understanding protein structure and function. Amino Acids. 46(6):1419–1439.