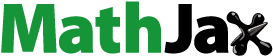
ABSTRACT
Due to increased awareness and strictly imposed legislations, Supply Chain (SC) managers are often forced to include recovery system in their SC. Recovery systems make use of 3 Rs, namely, recycling, reuse and remanufacturing which results in the transformation of traditional SC into closed-loop supply chain (CLSC). Recovery of discarded product in such forms is an effective way to deal with afterlife of product, leading to sustainable development. In this work, a bi-objective CLSC model has been developed for the paper industry under uncertain environment. The first objective of the proposed model is to maximise SC surplus, and the second objective is to incorporate sustainability through minimising carbon content by reducing the number of trucks between various echelons of CLSC network. In the proposed model, sustainability has been considered by two means: first to reduce the demand of next period by refurbishing some of the product collected from the customers and second is to reduce the raw material requirement at vendor side by using some of the recycled and usable product from the recycling centre. Further, various numerical illustrations are being performed and results are interpreted for a case paper industry which validates the application of the proposed model.
1. Introduction
Supply chain management (SCM) has been emerged as one of the most prominent areas among industries and academia in the last two decades. This is due to the fact that unified management of supply chain (SC) can mitigate the unnecessary costs and thus increase the profit of an entire organisation (Singh, Jain, and Mishra Citation2013; Ramezani, Bashiri, and Tavakkoli-Moghaddam Citation2013). A traditional supply chain consists of flow of materials, information and money between different stages and also establishes coordination among supplier, manufacturers and distributors to deliver products and services to customers. Doing so, we need a proper design of SC network which is an important decision for making the strategic-level planning of an organisation (Melo, Nickel, and Saldanha-Da-Gama Citation2009). A strategic issue associated with SCM is the design of a network that has a significant impact on the supply chain performance (Pishvaee, Rabbani, and Torabi Citation2011). The strategic decision is generally related to the number of facility location, capacity and technology to be used at the facility and quantity of flow between each echelon (Chopra and Meindl Citation2007).
Increasing awareness about issues like global warming, increasing pollution, etc., has advocated the need for sustainable development. For this purpose, governments in many countries have brought stringent environmental legislation, resulting in many significant changes in the structure of organisation/industries (Singh and Saxena (Citation2014); Jain and Singh Citation2020). In the wake of such circumstances, traditional supply chain has transformed into closed-loop supply chain (CLSC) to achieve sustainable goals of the organisation. CLSC could be seen as an extension in the form of reverse logistics in conjunction with existing forward SCN. It makes use of 3 Rs, namely, reuse, recycle and remanufacture to incorporate sustainability into the supply chain system. Guide and Van Wassenhove (Citation2009) defined management of CLSC ‘as the design, control, and operation of a system to maximize value creation over the entire life cycle of a product with dynamic recovery of value from different types and volumes of returns over time’ which seems more of business definition. However, in simple terms, CLSC could be termed as zero-waste supply chain which makes use of reuse, recycle and remanufacture. In corporate terminology, it could be a take-back programme where organisations need to dispose what they produce. CLSC accounts for both forward and reverse flows where forward flow consists of transporting products from supplier to consumer, while backward flow consists of proper recovery of product for effective utilisation of resources. CLSC modified the classical definition of traditional SC through establishing synergy between forward and reverse flows (Zeballos et al. Citation2014). The other prominent factor responsible for fostering of CLSC is the need for effective end-life management of product to minimise harmful environmental impacts (Govindan, Soleimani, and Kannan Citation2015). Application of CLSC could be seen in almost all industries from process to consumer goods (Kumar and Yamaoka Citation2007; Stindt and Sahamie Citation2014; Govindan, Soleimani, and Kannan Citation2015). Out of which, manufacturing and electronics industries are the most focused ones in the literature (Stindt and Sahamie Citation2014).
In this work, a CLSC model for the paper industry in the Indian context is designed. It is one of those sectors which need severe attention to protect deforestation as its consumption is very high in India due to huge demand. Pati, Vrat, and Kumar (Citation2008) designed a goal programming model for recycling of paper in the Indian context through increased wastepaper recovery. However, in this paper, we consider sustainability in two different ways. First way is to reduce the demand of next period by refurbishing some of the paper collected from the customers and the second one is to reduce the raw material requirement at paper vendor by using some of the recycled and usable paper from the recycling centre. Furthermore, uncertainty arises due to various real-world circumstances and often affects supply chain performance (Govindan, Fattahi, and Keyvanshokooh Citation2017). Thus, a robust SCN must be able to handle an uncertain environment to withstand in real-life scenario. Because of this, we have considered demand uncertainty in our model. Such type of work in the Indian context is first as per our belief. Also, various test cases are performed for an Indian paper industry to illustrate the applicability of the proposed model.
Further sections of this paper are structured as follows. The next section illustrates the relevant literature, while the problem is defined in Section 3. The model is formulated in Section 4, while Section 5 presents the existing cost details in the industry under our concerned study and solution methodology and result interpretations of various cases are discussed in Section 6, and finally, Section 7 gives the conclusion and future scope of this paper.
2. Literature review
CLSC models aim to address environmental issues to achieve the goal of sustainable development. Such approach not only minimises harmful impacts on the environment but also reaps economic benefits through effective use of resources. Because of this, such models are of great concerns among industries and academia. In this section, we examine such CLSC models, the solution approach and their applications. Also, due to both forward and reverse flows in CLSC, these models are often affected by uncertainty and thus are also discussed in consecutive subsection. Further motivation of this work is also given at the end of this section.
2.1. Closed-loop supply chain
Due to strict legislation and awareness about CLSC in recent times, it has emerged as one of the major interests among industries and academia (Govindan, Soleimani, and Kannan Citation2015; Govindan, Fattahi, and Keyvanshokooh Citation2017; Pant et al. Citation2018). Few reviews of CLSC literature could be found in the work by Akçalı and Cetinkaya (Citation2011); Jena and Sarmah (Citation2016); Govindan, Soleimani, and Kannan (Citation2015); Memari et al. (Citation2016); and Coenen, Van der Heijden, and van Riel (Citation2018). Govindan, Soleimani, and Kannan (Citation2015) extensively reviewed the work in the area of reverse logistic and CLSC from 2007 to 2013. However, Memari et al. (Citation2016) reviewed green SCN modelling in order to minimise CO2 emission. The authors suggested that carbon taxes and emission were the most encountered problems in the literature. However, there are many more tactical decisions whose integration with manufacturing and other philosophies needs to be incorporated to make SCN as real green SCN. Das and Jharkharia (Citation2018) reviewed low-carbon supply chain and found that almost all functions of the supply chain such as inventory planning, supplier selection, logistic decisions and network design have gone through transformations to integrate emission-related issues.
Application of CLSC could be observed in many companies like electronics (Tiwari et al. Citation2016; Talaei et al. Citation2016), automotive (Özceylan and Paksoy Citation2013; Bhattacharya et al. (Citation2018)) and gold (Zohal and Soleimani Citation2016) companies. Few authors worked on the option of multiple recoveries in the case of return in CLSC (Jerbia et al. Citation2018; Li et al. Citation2018). Some of the notable work in the field of CLSC is discussed in the next paragraph.
Amin and Zhang (Citation2013) proposed a multi-echelon mixed-integer linear programming (MILP) model for CLSC which considered minimisation of total cost and handled environmental issues (through the use of renewable and solar energy). A multi-objective problem was solved by using weighted sums and є constraint method. Finally, the author investigated the effect of demand and supply uncertainty in the supply chain by using stochastic programming (scenario based) approach to get different scenarios and sensitivity analysis performed to get optimal solutions. Zhang et al. (Citation2014) proposed a sustainable supply chain framework addressing three main indicators, namely, total cost, Green House Gases (GHG) emission and lead time. A MILP model was developed has prepared to deal with all three objectives such as minimisation of cost, minimisation of total environmental impact and optimisation of responsiveness of a model. To solve the multi-objective problem; the epsilon constraint method was implemented in modelling environment AIMMS and the CPLEX 12.4 solver was used. , the authors minimised the cost, GHG emission and lead time individually and compared the results of these three optimisation problems.
Garg et al. (Citation2015) addressed environmental issues through design of CLSC. For this purpose, a bi-objective non-linear integer problem was formulated. The proposed model optimised the flow of products and parts in CLSC network and number of trucks in forward chain. An interactive multi-objective technique was used as solution methodology and was coded in LINGO 11. The trade-off between operational and environmental performance was also discussed. Das and Posinasetti (Citation2015) studied CLSC and planned to build the model with sustainable objectives. For sustainable concerns, the author addressed environmental issues related to the supply chain. For this purpose, a multi-objective approach was proposed with the objectives to optimise profit, spent energy and emission in product distribution. The model was solved by using two approaches, namely, goal programming and a bi-objective Pareto optimal approach.
Kaya and Urek (Citation2016) presented a network model for investigating a CLSC, which captured the collection of discarded products after use along with new product distribution. First, a MILP facility location for inventory and pricing was developed. The aim of the model was to take decision related to facility location, inventory amounts, pricing of products and collection of used products. The proposed methodologyused to solve the model was simulated annealing variable neighbourhood search (SAVNS) algorithm, Tabu search variable neighbourhood search (TSVNS) algorithm and Genetic Algorithm variable neighbourhood search (GAVNS) algorithm. To solve that model, CPLEX 12.2 with GAMS 23.6 software was used and meta-heuristic algorithm was coded in C. Finally TSVNS performed better than GAVNS and SAVNS in terms of profit obtained. Ashby (Citation2018) investigated the use of CLSC for addressing environmental sustainability in clothing industries of the UK through natural resource-based view framework.
2.2. Uncertainty in supply chain
With respect to CLSC, few authors considered uncertainty in quantity as well as quality of returned products (Jindal, Sangwan, and Saxena Citation2015; Zare and Lotfi Citation2015; Pedram et al. Citation2017; Zeballos, Méndez, and Barbosa-Povoa Citation2018; Li et al. Citation2018; Gooran, Rafiei, and Rabani Citation2018). Zeballos, Méndez, and Barbosa-Povoa (Citation2018) developed a multi-item, multi-tier and multi-time horizon CLSC under uncertain environment. Further, the uncertainty was considered in quantity and quality of returned items using CVaR concept to maximise profit. Formulation of model tries to integrate two decisions (i.e. product development and CLSC design) simultaneously for two types of customers (i.e. new and secondary market). A two-stage stochastic MILP was suggested to solve the proposed model. To test the applicability of the proposed model, a case study of consumer goods from Europe is given which shows the effectiveness of the proposed solution. Jerbia et al. (Citation2018) studied a CLSC network design for a structure having multiple recovery options. At first, a MILP model was proposed with deterministic parameters. To study the impact of variations on the structure of network and company profit, a sensitivity analysis was carried out on input parameters (cost, revenues, customer return rates and proportions of return at each recovery points). Also, to account for higher uncertainties, a stochastic model was built based on the previous version of the model. A scenario-based uncertainty approach was considered for different input cost, return rates, quality of returns and revenues.
Li et al. (Citation2018) developed a multi-time horizon, multi-tier, multi-item reverse logistic network for recovery process. Uncertainty was considered in quality and quantity of returned product and handled using fuzzy triangular numbers. Also, fuzzy mathematical programming was suggested to solve the proposed model. Validation of the model was shown through a real-world case of manufacturing in the electronic industry. The results suggested that to have a successful recovery process, the customers must be legally enforced to return the products after their end life. Pedram et al. (Citation2017) came up with an integrated CLSC network for forward and reverse supply chain. They considered issues such as demand uncertainty, returned products and condition of the product in terms of quality. A MILP model was formulated for multi-tier, multi-product with the aim of profit maximisation. The applicability of the model was tested in a tire industry of Iran. Jeihoonian, Zanjani, and Gendreau (Citation2017) proposed a CLSC design considering the quality of returned as an uncertain parameter. Also, the scenario reduction technique was used to find the most pertinent scenario of interest. For this, they formulated L-shaped algorithm with surrogate constraints.
Demand is another aspect which is often affected due to many random causes and is most probable to have uncertain scenario. Singh, Jain, and Mishra (Citation2013) developed a Supply Chain Network Design for minimising the total supply chain cost using a two-stage stochastic programming approach under capacity constraint and uncertain demand scenario. For this purpose, a MILP model was proposed to find optimal decision regarding location and allocation of various facilities. The model was coded and solved using LINGO 8. Zeballos et al. (Citation2014) developed a multi-time horizon, multi-item CLSC network considering uncertainty in supply and customer demand. A MILP model was proposed to deal with multi-stage stochastic programming problem. The main objective was to minimise the expected cost associated with storage, facilities, transportation, purchasing and emissions. A scenario reduction algorithm was used to deal with an uncertain parameter. The problem formulation was coded in GAMS and solved through CPLEX Solver. Üster and Hwang (Citation2016) proposed an integrated CLSC under demand uncertainty and return of the products. Uncertainty was incorporated through modelling a two-stage stochastic MILP model. Objective function consists of fixed cost of operation, investment for expansion of facilities, transportation cost between facilities, operation cost such as collection, recycling, inspection and remanufacturing, etc. Bender decomposition method was suggested as a solution methodology for the proposed methodology.
Dai and Zheng (Citation2015) studied a multi-time horizon, multi-item, multi-tier CLSC under uncertain environment. A MILP model was formulated with the objective to maximise profit and minimise computational time. The problem was solved using Monte Carlo simulation-based embedded hybrid Genetic Algorithm (GA) where fuzzy and the chance-constrained algorithm were utilised to handle uncertainty parameters. The proposed hybrid genetic algorithm was made to find out the composition of the CLSC network and the effect of uncertainty in capacities, disposed rates and demands were studied through sensitivity analysis. Pasandideh, Niaki, and Asadi (Citation2015) attempted to optimise multi-item, multi-time horizon, multi-tier SC network model under uncertain scenario. The author addressed variable cost and fixed cost as two important parameters in their network. For this purpose, first a single-objective stochastic MILP model was prepared. Then, a deterministic bi-objective MILP model was developed in order to minimise total network cost. Well-known NSGA-II was utilised to solve the problem by using GAMS software to obtain Pareto solutions. To validate the result, NRGA was used by using modified priority-based encoding. T test and Simple Additive Weight (SAW) method were used to decide which algorithm performs better. In this work, only demand uncertainty is considered as the demand is the most volatile factor in paper industry, and this factor often decides the capacity of other operations in paper manufacturing and distribution. Other uncertainties like quality and quantity of returns in paper industries are not predominant.
2.3. Research motivation
Paper is one of the highest consumable items in India. Some probable reasons for this could be rapidly growing urbanisation, intense paper use in academics, increased literacy rates and increased economic growth in recent times. According to a report by Associated Chambers of Commerce Industry of India, the annual demand of paper in India will reach to 25 million tons by 2019–2020, and it was the fastest-growing market in terms of consumptions with Compound Annual Growth Rate (CAGR) of 10.6% in 2017–2018. There are 12 major players in the Indian market and approximately 600 paper mills exist in India. According to a report by CARE Ratings 2018, the per capita consumption of paper by an Indian individual is little above 13 kg which is much lower than the world average of 57 kg and 200 kg of North America. But with the second highest population in the world, India consumes paper in huge amount. This huge consumption does not only create a burden on forest which was approximately 19.3% of the total land in India but also decrease the forest areas at the rate of 0.6% per year as reported by van Beukering and Duraiappah (Citation1998). Thus, to reduce the excess burden on forest, there is a need for systematic recovery of paper through CLSC along with creating awareness about the effective use of paper. Moreover, today’s consumers have become aware of environmental concerns and simultaneously prefer the brand with eco-labelling. For this, the sustainability incorporation through various means has become a mandatory assignment for the organisations to remain in competition under the present day’s scenario. This work attains both concerns through designing a CLSC for effective recovery of paper and incorporate environment sustainability through an innovative approach. Also, only one paper, i.e. Pati, Vrat, and Kumar (Citation2008), was found which was limited to only recycling in case of Indian paper industry. Further, our work considers not only recycling but also other two Rs, i.e. reuse and remanufacturing. Further, the proposed work also explores the secondary market for gaining economic benefits while addressing environmental sustainability at the same time.
3. Problem statement
PQR Pvt. Ltd. is a paper industry situated at Raipur in Chhattisgarh state of India which produces printing & writing paper. It was founded in the last decade of the twentieth century, and at present, it is a well-established manufacturer of the above-said paper. In this work, the identity of the company is not revealed to maintain any loss of confidential data. The manufacturing plant has well-equipped latest technology, and the company infrastructure operations and services are consistently reviewed for the satisfaction of customer expectation. The company provides good quality of paper and also ensures high service level. However, due to strict legislation imposed by government agencies, it requires to adopt closed-loop network of paper distribution. This would not only help the company in terms of economic gain but also help to achieve economic sustainability and helps in gaining incentives from the government. Thus, the company aims to design CLSC network for distribution of paper. The company wishes to include 3 Rs (reduce, reuse and recycle) policy and achieves the goals of sustainability. Also, due to the recent advancement in cutting-edge technology, the lives of the product have shortened and newer products are often demanded. Such instances not only result in economic loss but also become a severe issue for the environment. Thus, these products need proper recovery systems in the form of recycling and reuse through the effective reverse logistics set-up. Reverse logistics often start with the collection of discarded products. Product returns can be collected by company-owned collection centre through various means of quality check according to organisation policies. From collection centre, usable products could be sent to refurbished centre to sell in the secondary market. However, leftover products are sent to recycling centre for end-life treatment either to the disposal sites or in the form of material recovery. However, with the extension of the network, company might face the problem of uncertainty for the flexibility of the supply chain. As a result, CLSC network is often motivated for the maximisation of economic gain and minimisation of environmental impacts under uncertain scenarios. Our task was to accomplish these goals for the PQR Pvt. Ltd.
4 Model formulation and description
4.1. Model formulation
To handle the problem of PQR Pvt. Ltd., a CLSC network (as shown in ) has been proposed that can be helpful for the company. CLSC consists of both forward chain and backward chain Bhattacharya et al. (Citation2018). Forward chain is similar to classic supply chain. It consist of a set of vendors, paper manufacturers, wholesale distributor and market (i.e. customer). The forward supply chain starts with the raw material procurement from vendors. Manufacturing plants are well equipped with the required updated technology and manufacture products. From there, the product moves to the wholesale distributor where a large amount of products are kept and then finally moves to the market for consumption by customers. Collection centre collects the returned product from customers, and sorting is also performed at the collection centre itself. This sorting helps to segregate the paper which can be either refurbished for secondary market use or sent for recycling and disposal activities. Refurbishing of paper could be used to make cards and for decoration purpose. Further, high-quality recycled paper could be sent to the vendor for meeting the demand of the next period, while other low-grade paper is to be disposed in disposal sites.
With a view to accomplish the goals of PQR Pvt. Ltd., the objective of the model is to maximise SC surplus and minimise the total number of trucks in the forward and backward chain as to achieve environmental sustainability. Further, it would take care of strategic decision regarding opening and closing of facilities. The model is aimed to perform well under uncertain environment for multi-time horizon. Moreover, the model formulation is done by considering the following assumptions:
All operations in CLSC are to be performed under capacity limitation, and cost parameters are known.
The locations of all the facilities are known in advance.
The model is designed for single product only.
It is assumed that the total associated transportation cost is summation of transportation cost and processing cost from the place of transport except for vendor and manufacturing plant.
Various cost parameters considered in the proposed model remain the same in all periods.
The consumption limit of the secondary market is unlimited.
4.2. Model description
enlists the notation used in the model formulation, while mentions decision variables.
Table 1. Notations used in mathematical model formulations
Table 2. Decision variables
4.3. Objective function
The objective function of the proposed model is to maximise supply chain surplus and minimise carbon content emission by reducing the number of trucks involved in the distribution network under the uncertain scenario.
4.3.1. First objective
The first objective is to maximise supply chain surplus which is defined as the difference of revenue generated and total supply chain cost.
Max F1 = Revenue generated – Total supply chain cost (raw material cost + fixed cost of facility + production cost + associated transportation cost)
Revenue generated: It is the summation of sales in primary market, secondary market and revenue generated through recycling. However, in the case of consecutive periods, some of the demand gets fulfilled from through quantity being refurbished in the previous period. Further, as a part of corporate social responsibility, the paper was safely disposed in the case organisation disposal sites and not to be sold for economic gains. Hence, the revenue from the disposal was not considered.
(1)
(1)
Total supply chain cost: It is the summation of fixed cost of facilities, raw material cost, production cost and associated transportation cost.
Fixed cost of facilities: Fixed cost is the summation of all costs involved before production of the first unit. Fixed cost, capacities and location of various facilities are expected to be known in advance. The corresponding binary variables account for the opening and closing of the facilities.
(2)
(2)
Raw material cost: It is the multiplication of unit ton cost of raw material and total tons of raw material quantity required.
(3)
(3)
Production cost: It is the multiplication of unit ton cost of production and total tons of quantity produced.
(4)
(4)
Associated transportation cost: It is the multiplication of unit ton cost of shipping and processing at concerned facilities and total tons of quantity to be transported between concerned echelons. Further, inventory carrying cost at the concerned facility was included in the processing cost.
(5)
(5)
From EquationEquations (1)(1)
(1) –(Equation5
(5)
(5) ), the first objective could be written as,
4.3.2. Second objective: to minimise the number of trucks in the forward and backward chain
4.4. Constraint equations
The objectives mentioned above are to be optimised under the following constraints:
4.4.1. Flow balance constraints
Constraint (8) ensures that the quantity shipped from the manufacturing plant cannot exceed what it has received from vendors. Constraint (9) ensures that the quantity shipped from the wholesale distributor warehouse cannot exceed what it has received from manufacturing plants. Constraint (10) ensures that market demand must be met through quantity received from retailer distributor warehouse.
Constraint (11) shows the amount of the returned product quantity collected at each collection/sorting centre, while constraint (12) is the quantity shipped to refurbished centre. Constraint (13) ensures flow between refurbished centre and secondary market.
Constraint (14) accounts for quantity of product being sent to the disposal site, while constraint (15) calculates quantity supplied to the vendor from the recycling centre.
4.4.2. Capacity constraints
Constraints (16), (17) and (18) limit the capacity of vendor, manufacturing plant and wholesale distributor warehouse to their set-specified capacity.
Constraints (19) and (20) account for the quantity shipped from collection/sorting centre and ensure that it cannot exceed their capacity. In similar way, constraints (21), (22) and (23) ensure that quantity shipped from recycling centre, refurbished centre and disposal sites cannot exceed their respective capacities.
4.4.3. Transportation capacity constraints
Constraints (24) to (27) represent the capacity of trucks at vendor, manufacturing plant, wholesale distributor warehouse and market.
Constraints (28), (29), (30), (31) and (32) represent the capacity of trucks at collection/sorting centre, refurbished centre, recycling centre, disposal sites and vendors, respectively.
Integer and non-negativity constraints are being imposed by constraint (33) on the corresponding decision variables.
Further, in the case of investment, Return on Investment (ROI) is one of the good indicators to justify any investment. The formula for calculating ROI is as follows:
Thus, for our case, the formula for ROI becomes:
5. Existing cost details in the industry under consideration
The cost parameters were discussed with the top officials of the PQR organisation. A committee was formed which include three senior managers, three operational mangers, four logistic managers, one professor and one masters student who were responsible for finalising the cost parameters. The various parameters and cost associated with the concerned PQR Pvt. Ltd. were given in . All the costs associated with it are in millions of Indian rupees.
Table 3. Various parameters of the given problem
Table 4. Unit ton (raw material + transportation) cost (in million Rs. (₹)) from vendor to manufacturing plants
Table 5. Unit ton (production + transportation) cost (in million Rs. (₹)) for raw material sent to wholesale distributor warehouse
Table 6. Unit ton associated transportation cost (in million Rs. (₹)) from whole distributor warehouse (J) to market (K) & fixed cost (in million Rs. (₹)) of warehouses along with demand for all three periods
Table 7. Unit ton associated transportation cost (in million Rs. (₹)) from market (K) to collection/sorting centre (L)
Table 8. Unit ton associated transportation cost (in million Rs. (₹)) from collection/sorting centre (L) to refurbished centre (R) & fixed cost (in million Rs. (₹)) of collection/sorting centre
Table 9. Unit ton associated transportation cost (in million Rs. (₹)) from refurbished centre (R) to secondary market (T) & fixed cost (in million Rs. (₹)) of refurbished centre
Table 10. Unit ton (in million Rs. (₹)) associated transportation cost from collection/sorting centre (L) to recycling centre (F)
Table 11. Unit ton associated transportation cost (in million Rs. (₹)) from collection centre (L) to disposal site (Z) & fixed cost (in million Rs. (₹)) of disposal sites
Table 12. Unit ton associated transportation cost (in million Rs. (₹)) from recycling centre (F) to vendor (H)
6. Different cases with their results, interpretation and solution methodology
Different cases have been examined to test the applicability of the proposed model. In the first two cases, each objective is dealt with separately, and their effect on other objective is observed. This is done in accordance with giving flexibility to decision-maker of organisation to choose optimal policy for his/her organisation. This would help to gain useful insight in selecting suitable trade-off between economic and environmental objectives. However, due to real-world scenarios, the model is bound to face uncertain scenario. For this purpose, ±20% uncertainty is considered in case 3 , which is a much safer assumption to handle uncertain scenario (Yadav, Tripathi, and Singh Citation2019). The details about all three cases are discussed below:
Case 1 involves maximisation of single objective, i.e. supply chain surplus without considering minimisation of number of trucks for a single-product multi-period time horizon. Three time periods have been considered for this scenario, and strategic decision regarding opening and closing of facilities is also considered. For consecutive next period, there has been a 5–20% increase in demand. This case has been coded in MATLAB 2014a. For coding in MATLAB, function like linprog was used which is used to solve linear programming (LP) problems.
Solution approach through linprog: linprog is a programme which is coded to solve LP problems having a structure as:
Subjected to:
where is the linear objective vector, while
is the left-hand side (LHS) of inequality constraint matrix and
is the right-hand side (RHS) of the inequality constraint matrix. Similarly,
is the LHS of equality constraint matrix and
is the RHS of equality constraint matrix.
is lower bound for the variables, while
is upper bound for the variables. Using this, the proposed model was solved. However, MS-Excel was also utilised to compare the results which gave the same results. But for larger data set problem, MS-Excel would fail to provide an optimal solution due to exceeding the limit of variables. shows the results obtained for the first case.
Table 13. Scenario of case 1 for different periods
The optimal configuration for the product flow and strategic decision regarding opening and closing of facilities for period 1 are presented in . Similar results were obtained for other periods. The dotted blank space shows the closed facilities. The number on the arrowed head shows the optimal ton of product flow between the echelons. In forward SCN, manufacturing plant 1 and retailer distributor warehouses 2 and 4 were closed, while in reverse network, collection/sorting centre 1, refurbished centre 2 and disposal sites 1 were closed. The existing supply chain surplus was 15,435.17 million Indian rupees, while the number of truck being used was 302 trucks. For comparison purpose, this was also considered as period 1 parameters of existing FSC. Further, the projected parameters in existing FSC for consecutive periods are mentioned in . The SC surplus of the proposed CLSC is higher because of serving the secondary market as well as getting some portion of raw material through recycling in CLSC. But, the number of trucks in the proposed CLSC is higher. This is because the existing system is of forward supply chain only but later considered CLSC which consists of both forward and reverse supply chain. The ROI for this case was found to be 0.2546. So it can be said that the new investment in CLSC would be recovered in four time periods.
Figure 2. Optimal product flow and network configuration between echelons in forward supply chain for case 1
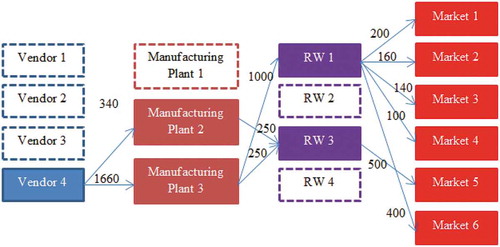
Figure 3. Optimal product flow and network configuration between echelons in reverse supply chain for case 1
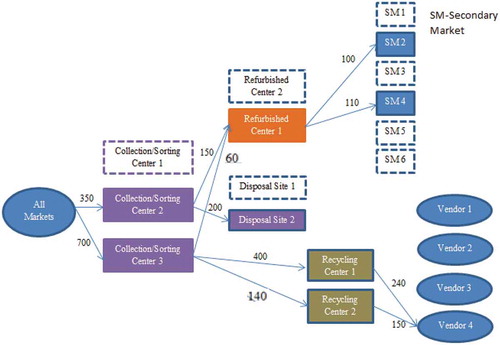
Table 14. Scenario of case 2 for different periods
Table 15. Comparison of existing FSC, case 1 and case 2
Case 2 involves minimisation of number of trucks for a single-product multi-period time horizon. Three time periods have been considered for this scenario, and strategic decision regarding opening and closing of facilities have also been considered. For consecutive next period, there has been a 5–20% increase in demand. This case has also been coded in MATLAB 2014a.
For coding, the intlinprog syntax is being used which is used to solve linear optimisation problem having flexibility to keep variable as integer, and it requires objective function, equalities and inequalities constraint similar to linprog as explained in case 1. However, ceil function is also used where integer value is not possible as the demand could not be integer value at all time. The available capacity of the truck was 20 tons. So each known quantity, i.e. demand, fixed cost of facilities, was divided by 20 initially to get the required integer flow quantity and then multiplied by 20 to get the exact ton of flow quantities. The results obtained are presented in .
The optimal configuration for the product flow and strategic decision regarding opening and closing of facilities are presented in . The dotted blank space shows the closed facilities. The number on the arrowed head shows the optimal tons of product flow between the echelons. For this case, manufacturing plant 1 and retailer warehouses 2 and 4 were closed, while in reverse network, collection centre 1, refurbished centre 2 and disposal sites 1 were closed as similar to that of case 1. The ROI for this case was found to be 0.2274.
Figure 4. Optimal product flow and network configuration between echelons in forward supply chain for case 2
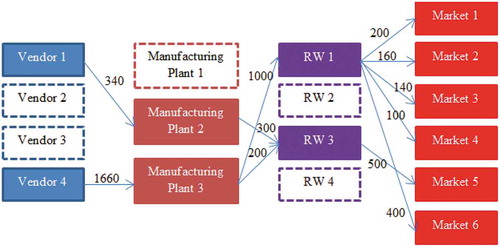
Figure 5. Optimal product flow and network configuration between echelons in reverse supply chain for case 2
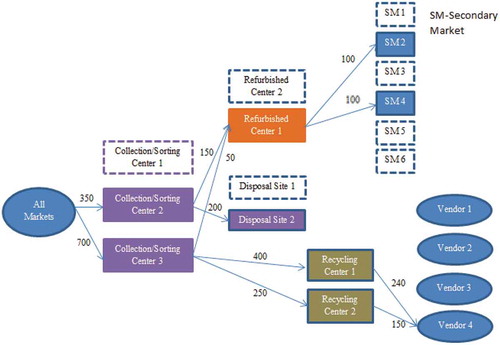
Comparison of case 1 and case 2
The comparison for all three periods in existing FSC and the above two cases is shown in which reveals that reducing the number of trucks results in environmental sustainability through less release of carbon in the environment. It was observed that the model has optimised both supply chain surplus and reduced carbon content separately. It was found that while prioritising first objective results in more economic gain but releases more carbon in the environment, while prioritising second objective results in lesser carbon but increase in supply chain cost. However, the average net profit was found to be only 1.58% in case 1 over case 2; thus, a slight increase in supply chain cost (or decrease in profit) can bring better environmental sustainability. This trend is clearly depicted in . Further, it is a general notion and not the mandatory argument among industrial practitioners that the operation of CLSC is justifiable only if profit in CLSC is 30% more than that of FSC. Thus, the obtained results reveal that CLSC operation is justifiable from the second period.
Figure 6. Comparison of SC surplus and number of trucks for existing FSC and proposed CLSC (cases 1 and 2)
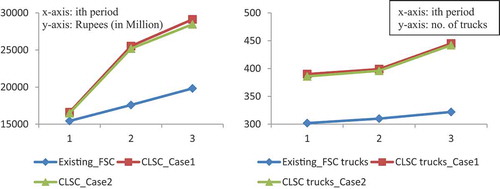
Case 3 involves the introduction of uncertainty to check how does model behave in an uncertain environment because most of the CLSC fail due to inability of handling uncertainty. Here, uncertainty is introduced at demand points. For this purpose, 20% uncertainty is considered at each demand point through the application of Monte Carlo simulation (performed in MATLAB). Several instances were performed and their averages were taken for each demand period. Also, similar approach as discussed in case 1 and case 2 was used to solve the above problem. Three time periods have been considered for this scenario, and strategic decision regarding opening and closing of facilities is also considered. For consecutive next period, there has been 5–20% increase in demand under uncertain scenario. The results obtained are presented in .
Table 16. Scenario of case 3 for different periods
The optimal configuration for the product flow and strategic decision regarding opening and closing of facilities are presented under uncertain environment as shown in . The dotted blank space shows the closed facilities. The number on the arrowed head shows the optimal tons of product flow between the echelons. In forward SCN, manufacturing plant 1 and retailer distributor warehouses 2 and 4 were closed, while in reverse network, collection centre 1, refurbished centre 2 and disposal sites 1 were closed as similar that of previous two cases which show the ability of model to handle the uncertain environment. However, this case shows lower average profitability as compared to the first and second cases due to uncertain demand. However, the profit is still significant as compared to both cases which prove the proposed model is well capable in handling uncertain demand scenario. Further, the comparison of existing FSC and proposed CLSC under case 3 circumstances is depicted in .
Figure 7. Optimal product flow and network configuration between echelons in forward supply chain for case 3
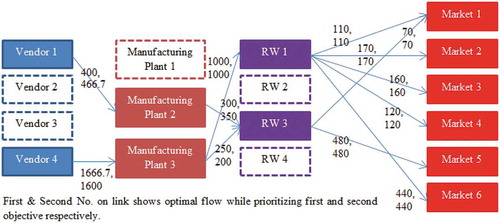
Figure 8. Optimal product flow and network configuration between echelons in reverse supply chain for case 3
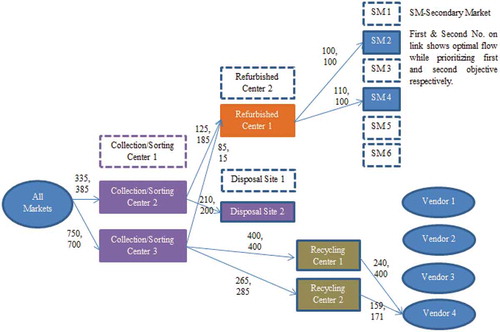
Figure 9. Comparison of SC surplus and no. of trucks for existing FSC and CLSC under uncertain environment for case 3
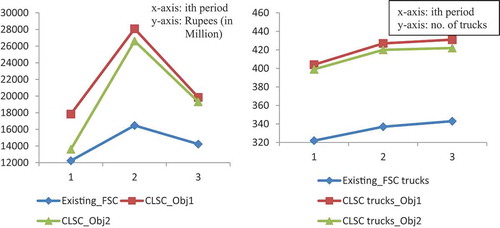
6.1. Managerial implications
With the emergence of circular economy, the organisation needs to focus on the implementation of CLSC to remain in the competition. Thus, it is relevant to say that CLSC has become a customary solution for the present-day manufacturing organisation needs. Further, CLSC has the potential to introduce sustainability through the use of 3 Rs, i.e. reuse, remanufacturing and recycle. The organisations could use CLSC to incorporate sustainability and simultaneously comply with the regulations laid by government agencies. The proposed model is also a unique approach to introduce sustainability through two ways, namely, first by reducing the demand of next period by refurbishing some of the product collected from the customer and second by reducing the raw material requirement at vendor side by using some of the recycled and usable product from the recycling centre. The model is also well capable of handling uncertainty up to a certain limit. Further, it was learnt from the comparison graph that minor investment can be made to achieve better sustainability so in between two objectives, the second objective (i.e. minimisation of carbon content) should be given more emphasis. It was seen in our model that, moreover, the proposed model could help the case organisation in gaining eco-labelling and consumer support for complying with government regulations. Further, such initiatives are supported by various government schemes, and thus, it is a strong probability to gain government subsidies either in taxation or CLSC implementation. The reputation of organisation is also expected to enhance which further help in gaining more market share and thus boost the economic growth of the organisation.
7. Conclusion and future scope
A CLSC network is analysed in this study. For this purpose, a MILP model is introduced to deal with the design and planning problem of multi-echelon and multi-period CLSC for a paper industry in the Indian context. The proposed MILP model consists of four layers (i.e. vendor, manufacturing plants, wholesale distributor warehouse and customer) in the forward chain and five layers (i.e. collection centre, refurbished centre, secondary market, recycling centre and disposal site) in the backward chain with considering sustainability under uncertain environment. Two objectives (i.e. maximising supply chain surplus and minimising carbon content through reducing the number of trucks) were addressed to achieve sustainable goals for the concerned paper industry. The sustainability is being introduced by two ways by reducing the demand of next period by refurbishing some of the products collected from the customers and reducing the raw material requirement at supplier by using some of the recycled and usable products from the recycling centre. It was found that with little increase in supply chain cost (or decrease in profit) can be used to achieve better environmental sustainability. Also, the effect of uncertain demand was studied which shows a reduction in profit compared to certain demand. But the model was well capable of handling such instances and still produces significant profit.
However, our model could also be solved using augmented epsilon method and Lpnorm. The researcher may try these techniques and compare results or may perform sensitivity analysis having different values of epsilon in augmented epsilon method. Goal programming (GP) is another option that could be utilised to solve such problems. In GP, the designer can set goals for each objective that he or she wishes to attain. Lexicographic method could also be used if the importance of the objective is predetermined and known. For future research in this area, some heuristic and meta-heuristics approach may be proposed to handle large-size problems. The proposed model could also be designed in a way so that it can tackle multi-product multi-time horizon problem with intra-echelon flow because generally most of the organisations deal with multi-products or services and often need intra-flow due to application of various business strategies. Also, it is recommended to test the model with other types of uncertainties at supply side and the process side by considering operational activities such as inventory, choice of inventory, mode of transport and product remanufacturing. Furthermore, the model that we have presented could be improved by several other extensions such as risk mitigation in supply chain and budget constraints of the organisations.
Disclosure statement
No potential conflict of interest was reported by the authors.
Additional information
Notes on contributors
Kamlesh Pant
Kamlesh Pant holds a master degree in Industrial Engineering and Management from National Institute of Technology Raipur, India. He received gold medal for excellence performance in his M.Tech degree. His areas of research include close loop supply chain, optimization techniques, multi criteria decision making and industrial engineering.
Vinay Surendra Yadav
Vinay Surendra Yadav is a research scholar in the department of mechanical engineering at National Institute of Technology (NIT) Raipur, India. He also holds a master degree in Industrial Engineering and Management from NIT Raipur, India. He received gold medal for excellence performance in his M.Tech degree. His areas of research include supply chain management, optimization techniques, omnichannel, blockchain and multi criteria decision making. He has published more than 10 papers in international journals and conferences.
A. R. Singh
Dr. A. R. Singh is working as an assistant professor in the department of mechanical engineering at National Institute of Technology Raipur, India. He also holds a PhD degree in mechanical engineering from Motilal Nehru National Institute of Technology (MNNIT), Allahabad, India. He completed his Master of Technology degree in CAD-CAM from MNNIT, Allahabad while Bachelor of Engineering degree in mechanical engineering from U.P.T.U, India. His areas of specialization are operation research, supply chain management, optimization techniques, multi criteria decision making, blockhain, lean six sigma and education management. He has published more than 50 papers in international journals and conferences.
References
- Akçalı, E., and S. Cetinkaya. 2011. “Quantitative Models for Inventory and Production Planning in Closed-loop Supply Chains.” International Journal of Production Research 49 (8): 2373–2407. doi:10.1080/00207541003692021.
- Amin, S. H., and G. Zhang. 2013. “A Multi-objective Facility Location Model for Closed-loop Supply Chain Network under Uncertain Demand and Return.” Applied Mathematical Modelling 37 (6): 4165–4176. doi:10.1016/j.apm.2012.09.039.
- Ashby, A. 2018. “Developing Closed Loop Supply Chains for Environmental Sustainability: Insights from a UK Clothing Case Study.” Journal of Manufacturing Technology Management 29 (4): 699–722. doi:10.1108/JMTM-12-2016-0175.
- Bhattacharya, R., A. Kaur, and R. K. Amit. 2018. “Price Optimization of Multi-stage Remanufacturing in a Closed Loop Supply Chain.” Journal of Cleaner Production 186: 943–962. doi:10.1016/j.jclepro.2018.02.222.
- Chopra, S., and P. Meindl. 2007. “Supply Chain Management. Strategy, Planning & Operation.” In Das Summa Summarum Des Management, 265–275. Gabler.
- Coenen, J., R. E. Van der Heijden, and A. C. van Riel. 2018. “Understanding Approaches to Complexity and Uncertainty in Closed-loop Supply Chain Management: Past Findings and Future Directions.” Journal of Cleaner Production 201: 1–13. doi:10.1016/j.jclepro.2018.07.216.
- Dai, Z., and X. Zheng. 2015. “Design of Close-loop Supply Chain Network under Uncertainty Using Hybrid Genetic Algorithm: A Fuzzy and Chance-constrained Programming Model.” Computers & Industrial Engineering 88: 444–457. doi:10.1016/j.cie.2015.08.004.
- Das, C., and S. Jharkharia. 2018. “Low Carbon Supply Chain: A State-of-the-art Literature Review.” Journal of Manufacturing Technology Management 29 (2): 398–428.
- Das, K., and N. R. Posinasetti. 2015. “Addressing Environmental Concerns in Closed Loop Supply Chain Design and Planning.” International Journal of Production Economics 163: 34–47. doi:10.1016/j.ijpe.2015.02.012.
- Garg, K., D. Kannan, A. Diabat, and P. C. Jha. 2015. “A Multi-criteria Optimization Approach to Manage Environmental Issues in Closed Loop Supply Chain Network Design.” Journal of Cleaner Production 100: 297–314. doi:10.1016/j.jclepro.2015.02.075.
- Gooran, A., H. Rafiei, and M. Rabani. 2018. “Modeling Risk and Uncertainty in Designing Reverse Logistics Problem.” Decision Science Letters 7 (1): 13–24. doi:10.5267/j.dsl.2017.5.001.
- Govindan, K., M. Fattahi, and E. Keyvanshokooh. 2017. “Supply Chain Network Design under Uncertainty: A Comprehensive Review and Future Research Directions.” European Journal of Operational Research 263 (1): 108–141. doi:10.1016/j.ejor.2017.04.009.
- Govindan, K., H. Soleimani, and D. Kannan. 2015. “Reverse Logistics and Closed-loop Supply Chain: A Comprehensive Review to Explore the Future.” European Journal of Operational Research 240 (3): 603–626. doi:10.1016/j.ejor.2014.07.012.
- Guide, V. D. R., Jr, and L. N. Van Wassenhove. 2009. “OR FORUM—The Evolution of Closed-loop Supply Chain Research.” Operations Research 57 (1): 10–18. doi:10.1287/opre.1080.0628.
- Jain, N., and A. R. Singh. 2020. “Sustainable Supplier Selection under Must-be Criteria through Fuzzy Inference System.” Journal of Cleaner Production 248: 119275. doi:10.1016/j.jclepro.2019.119275.
- Jeihoonian, M., M. K. Zanjani, and M. Gendreau. 2017. “Closed-loop Supply Chain Network Design under Uncertain Quality Status: Case of Durable Products.” International Journal of Production Economics 183: 470–486. doi:10.1016/j.ijpe.2016.07.023.
- Jena, S. K., and S. P. Sarmah. 2016. “Future Aspect of Acquisition Management in Closed-loop Supply Chain.” International Journal of Sustainable Engineering 9 (4): 266–276. doi:10.1080/19397038.2016.1181120.
- Jerbia, R., M. K. Boujelben, M. A. Sehli, and Z. Jemai. 2018. “A Stochastic Closed-loop Supply Chain Network Design Problem with Multiple Recovery Options.” Computers & Industrial Engineering 118: 23–32. doi:10.1016/j.cie.2018.02.011.
- Jindal, A., K. S. Sangwan, and S. Saxena. 2015. “Network Design and Optimization for Multi-product, Multi-time, Multi-echelon Closed-loop Supply Chain under Uncertainty.” Procedia Cirp 29: 656–661. doi:10.1016/j.procir.2015.01.024.
- Kaya, O., and B. Urek. 2016. “A Mixed Integer Nonlinear Programming Model and Heuristic Solutions for Location, Inventory and Pricing Decisions in a Closed Loop Supply Chain.” Computers & Operations Research 65: 93–103. doi:10.1016/j.cor.2015.07.005.
- Kumar, S., and T. Yamaoka. 2007. “System Dynamics Study of the Japanese Automotive Industry Closed Loop Supply Chain.” Journal of Manufacturing Technology Management 18 (2): 115–138. doi:10.1108/17410380710722854.
- Li, Y., D. Kannan, P. C. Jha, K. Garg, J. Darbari, and N. Agarwal. 2018. “Design of a Multi Echelon Product Recovery Embeded Reverse Logistics Network for Multi Products and Multi Periods.” In Annals of Operations Research, 1–22. doi: 10.1007/s10479-018-2776-4
- Melo, M. T., S. Nickel, and F. Saldanha-Da-Gama. 2009. “Facility Location and Supply Chain management – A Review.” European Journal of Operational Research 196 (2): 401–412. doi:10.1016/j.ejor.2008.05.007.
- Memari, A., A. R. A. Rahim, R. Ahmad, and A. Hassan. 2016. “A Literature Review on Green Supply Chain Modelling for Optimising CO2 Emission.” International Journal of Operational Research 26 (4): 509–525. doi:10.1504/IJOR.2016.077725.
- Özceylan, E., and T. Paksoy. 2013. “A Mixed Integer Programming Model for a Closed-loop Supply-chain Network.” International Journal of Production Research 51 (3): 718–734. doi:10.1080/00207543.2012.661090.
- Pant, K., A. R. Singh, U. Pandey, and R. Purohit. 2018. “A Multi Echelon Mixed Integer Linear Programming Model of a Close Loop Supply Chain Network Design.” Materials Today: Proceedings 5 (2): 4838–4846.
- Pasandideh, S. H. R., S. T. A. Niaki, and K. Asadi. 2015. “Bi-objective Optimization of a Multi-product Multi-period Three-echelon Supply Chain Problem under Uncertain Environments: NSGA-II and NRGA.” Information Sciences 292: 57–74. doi:10.1016/j.ins.2014.08.068.
- Pati, R. K., P. Vrat, and P. Kumar. 2008. “A Goal Programming Model for Paper Recycling System.” Omega 36 (3): 405–417. doi:10.1016/j.omega.2006.04.014.
- Pedram, A., N. B. Yusoff, O. E. Udoncy, A. B. Mahat, P. Pedram, and A. Babalola. 2017. “Integrated Forward and Reverse Supply Chain: A Tire Case Study.” Waste Management 60: 460–470. doi:10.1016/j.wasman.2016.06.029.
- Pishvaee, M. S., M. Rabbani, and S. A. Torabi. 2011. “A Robust Optimization Approach to Closed-loop Supply Chain Network Design under Uncertainty.” Applied Mathematical Modelling 35 (2): 637–649. doi:10.1016/j.apm.2010.07.013.
- Ramezani, M., M. Bashiri, and R. Tavakkoli-Moghaddam. 2013. “A Robust Design for a Closed-loop Supply Chain Network under an Uncertain Environment.” The International Journal of Advanced Manufacturing Technology 66 (5–8): 825–843. doi:10.1007/s00170-012-4369-8.
- Singh, A. R., R. Jain, and P. K. Mishra. 2013. “Capacities-based Supply Chain Network Design considering Demand Uncertainty Using Two-stage Stochastic Programming.” The International Journal of Advanced Manufacturing Technology 69 (1–4): 555–562. doi:10.1007/s00170-013-5054-2.
- Singh, S. R., and N. Saxena (2014). “The Value of Product Life-cycle for Deteriorating Items in a Closed Loop under the Reverse Logistics Operations.” Proceedings of the Second International Conference on Soft Computing for Problem Solving (SocProS 2012), December 28–30, 2012, 383–395. New Delhi: Springer.
- Stindt, D., and R. Sahamie. 2014. “Review of Research on Closed Loop Supply Chain Management in the Process Industry.” Flexible Services and Manufacturing Journal 26 (1–2): 268–293. doi:10.1007/s10696-012-9137-4.
- Talaei, M., B. F. Moghaddam, M. S. Pishvaee, A. Bozorgi-Amiri, and S. Gholamnejad. 2016. “A Robust Fuzzy Optimization Model for Carbon-efficient Closed-loop Supply Chain Network Design Problem: A Numerical Illustration in Electronics Industry.” Journal of Cleaner Production 113: 662–673. doi:10.1016/j.jclepro.2015.10.074.
- Tiwari, A., P. C. Chang, M. K. Tiwari, and R. Kandhway. 2016. “A Hybrid Territory Defined Evolutionary Algorithm Approach for Closed Loop Green Supply Chain Network Design.” Computers & Industrial Engineering 99: 432–447. doi:10.1016/j.cie.2016.05.018.
- Üster, H., and S. O. Hwang. 2016. “Closed-Loop Supply Chain Network Design under Demand and Return Uncertainty.” Transportation Science 51 (4): 1063–1085. doi:10.1287/trsc.2015.0663.
- van Beukering, P., and A. Duraiappah. 1998. “The Economic and Environmental Impact of Wastepaper Trade and Recycling in India: A Material Balance Approach.” Journal of Industrial Ecology 2 (2): 23–42. doi:10.1162/jiec.1998.2.2.23.
- Yadav, V. S., S. Tripathi, and A. R. Singh. 2019. “Bi-objective Optimization for Sustainable Supply Chain Network Design in Omnichannel.” Journal of Manufacturing Technology Management 30 (6): 972–986. doi:10.1108/JMTM-06-2017-0118.
- Zare, F., and M. M. Lotfi. 2015. “A Possibilistic Mixed-integer Linear Programme for Dynamic Closed-loop Supply Chain Network Design under Uncertainty.” International Journal of Industrial and Systems Engineering 21 (1): 119–140. doi:10.1504/IJISE.2015.070878.
- Zeballos, L. J., C. A. Méndez, and A. P. Barbosa-Povoa. 2018. “Integrating Decisions of Product and Closed-loop Supply Chain Design under Uncertain Return Flows.” Computers & Chemical Engineering 112: 211–238. doi:10.1016/j.compchemeng.2018.02.011.
- Zeballos, L. J., C. A. Méndez, A. P. Barbosa-Povoa, and A. Q. Novais. 2014. “Multi-period Design and Planning of Closed-loop Supply Chains with Uncertain Supply and Demand.” Computers & Chemical Engineering 66: 151–164. doi:10.1016/j.compchemeng.2014.02.027.
- Zhang, Q., N. Shah, J. Wassick, R. Helling, and P. Van Egerschot. 2014. “Sustainable Supply Chain Optimisation: An Industrial Case Study.” Computers & Industrial Engineering 74: 68–83. doi:10.1016/j.cie.2014.05.002.
- Zohal, M., and H. Soleimani. 2016. “Developing an Ant Colony Approach for Green Closed-loop Supply Chain Network Design: A Case Study in Gold Industry.” Journal of Cleaner Production 133: 314–337. doi:10.1016/j.jclepro.2016.05.091.