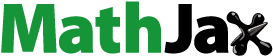
ABSTRACT
In this article, an integrated two-level supply chain model is described in two different scenarios by treating the demand rate as a triangular fuzzy number. In the first scenario, the retailer offers a price discount for customers on the backorders; it encourages the customer to wait for the product. In the second scenario, the retailer does not provide any discount; instead, an investment is made to reduce lost sales during the stock-out. In both scenarios, the shortages are allowed, and it is partially back-ordered. Two different cases are discussed within these scenarios with two different production functions related to the process quality and production rate. The signed distance method is employed to handle the fuzzified parameter. This research aims to minimize the total expected cost of the supply chain model under each scenario with energy consumption and greenhouse gas emissions during the production process. Moreover, to investigate how changes in the production rate affect the quality of products produced and the entire model’s total cost under the effects of backorder price discounts and lost sales reduction. An iterative algorithm is followed to acquire numerical results. To demonstrate the model, some numerical instances, sensitivity analysis and managerial insights are provided.
1 Introduction
Over the years, industries and manufacturing companies have been releasing various pollutants into the environment through land, air and water. It is estimated that about 50 per cent of all pollution is caused by industrial and manufacturing activities. It reveals that industries also play an essential role in delivering harmful and hazardous pollutants to the environment. Furthermore, diseases, loss of life and environmental degradation are the effects of pollution. This pollution has been proven to be extremely harmful to the environment in several forms. Moreover, in industry, energy is used in many ways, i.e. most businesses or organizations use energy during the production phase for several processes. In general, electrical energy, steam energy, and cooling energy are the energy used by companies. So there are costs involved in operating and purchasing these systems. Therefore, it seems necessary to consider the need for energy. It requires both electricity and workforce during the production process, so we incorporate the cost of energy use during the production and rework of defective materials. Greenhouse gas (GHG) absorb energy and warm the earth. Human activities have been mainly responsible for increasing greenhouse gases in the atmosphere over the past 150 years.
In any industry, the production rate is not always balanced, and it keeps changing concerning the situations. Also, if the product’s demand is high among the consumers, the company will increase production rate. Similarly, as the demand for a product decreases, so does the rate of production. Therefore, it is good to consider the variable production, which is one of the realistic factors. Suppose there is any problem with the production machinery during the production phase. In that case, the machine shift from the in-control position to the out-of-control position, so the resulting product will be defective, leading to the imperfect production process.
It is difficult for a company to predict its demand rate before marketing, so the demand for goods in the market may be low or high. Based on this, it is evident that the market demand for a product cannot be constant; that is, the demand rate is unclear. In this stage, the requirement for the product is said to be an uncertain demand. The demand uncertainty occurs when a business or an industry cannot accurately predict consumer demand for its products or services. The fuzzy logic techniques effectively solve complex, ill-defined problems characterized by environmental uncertainty and ambiguity of information. It allows for handling uncertain and imprecise knowledge and provides a robust framework for reasoning. Therefore, it has been confirmed that fuzzy logic is compelling in overcoming such uncertainty, and it describes a phenomenon in which a mathematical model or input data is unknown. Thus, the solution to these sorts of challenges can be found by considering an uncertainty parameter with a fuzzy number. In our model, the product’s demand rate holds the uncertainty, and to treat this, we consider a triangular fuzzy number.
Consequently, in this model, we will look at how the industry affects the environment and how to reduce overall costs by mitigating these impacts.
2 Literature review
This subsection describes the detailed review analysis of the existing literature works.
2.1 Energy consumption and GHG emission
Over time, industries emit more and more greenhouse gases, especially as the production process increases, resulting in more greenhouse gas emissions. Plambeck (Citation2012) has described the experience of the world’s largest business and how it can reduce GHG emissions in the supply chains of start-ups and offer suggestions for expanding the efficiency of new zero-emission supply chains. By considering GHG emissions from manufacturing processes and various carbon trading systems, Jaber et al. (Citation2013) have developed a two-stage supply chain model with a collaborative framework, proposing possible combinations between these projects. Bazan et al. (Citation2015) explained two models, typically considering the energy used in the manufacturing process from a single seller to a single buyer under consignment stock policy. Ugarte et al.(Citation2016) presented a supplier-retailer supply chain model by incorporating the cost for GHG emissions during the production process and analysed the profit functions of decentralised and centralised models with the sense of emissions trading schemes. Sarkar et al. (Citation2018) have developed a supply chain manufacturing model with suppliers for the automobile parts manufacturing industry to improve production volume with multiple objectives, mainly by reducing energy costs while considering renewable energy. With better energy policy and energy consumption, Safarzadeh and Rasti-Barzoki (Citation2019) discussed share price decisions to increase the profitability of supply-chain players. Karthick and Uthayakumar (Citation2021) developed a multi-item inventory model with CS policy to reduce the carbon emission through the logarithmic investment.
2.2 Imperfect manufacturing process
In the literature, many researchers had described imperfect production models. Imperfect production is caused due to mechanical errors in the manufacturing process. Rosenblatt and Lee (Citation1986) examined the effects of imperfect manufacturing processes on deteriorating goods during the optimum production cycle. Cheng (Citation1991) proposed an EOQ model with demand-oriented unit manufacturing cost under the imperfect manufacturing processes. Salameh and Jaber (Citation2000) have illustrated a production inventory model by considering the manufacturing process in perfect and imperfect situations. Hayek and Salameh (Citation2001) have examined the impact of defective quality products on a limited production scale and predicted that faulty products to rework at a constant rate. Liao et al. (Citation2009) developed comprehensive maintenance and improvement plans with an EPQ model for an incomplete process, including a deteriorating production system with an increased risk ratio. Sarkar et al. (Citation2011) described an EPQ model for continuous and unique random demand for goods and examines the percentage decrease in overall output with an increase in production run time when the manufacturing process is considered imperfect.
2.3 Sustainable and variable production
Khouja and Mehrez (Citation1994) have illustrated an imperfect production model by considering the production rate as a variable with the quality process. Sarkar et al. (Citation2018) have established an imperfect supply chain model under varying production process with the incorporation of the unit manufacturing cost as a function of the production rate, and analysed the three various production functions correlated with system quality and production rate. Marchi et al. (Citation2019) have developed a two-stage supply chain model that considers two integrated principles: classical and vendor-managed inventory policies, including inventory maintenance costs, GHG emissions, material and process efficiency, and transport operations. Herbon (Citation2020) developed an approximated solution to the constrained integrated supply model by considering the variable production rate.
2.4 Expected shortages and effects of backorder price discount
Shortages can occur when there is not enough inventory. Simultaneously, some customers may switch to other enterprise products, and to overcome this problem, some researchers have proven through their model that they can retain customers by offering price discounts on backorder products. For practical purposes, Gallego and Moon (Citation1993) were first to develop an inventory model, in which the lead time demand doesn’t follow any specific distribution. Subsequently, considering (Gallego and Moon (Citation1993)) model, an inventory model has been developed by Lin (Citation2008) to address the demand for a lead time that does not follow any specific distribution with a backorder price discount. Sarkar et al. (Citation2015) illustrated the concept of an inventory model with a price discount plan, taking into account the initial investment to enhance the quality of the product. Ganesh Kumar and Uthayakumar (Citation2019) have considered a two-level supply chain model with multi-item, variable backorder under trade credit policy.
2.5 Lost sale reduction
In many cases, some customers will not wait for the goods they need in the absence of inventory. In this situation, the manufacturer or seller may lose customers. So some researchers thought lost sale reduction would help such situations. Ouyang and Chang (Citation2001) illustrated a model to analyse the effects of lost sale reduction with the lead time demand to follow normal and free distribution approach. Hwang et al. (Citation2013) developed the economic lot-sizing problem with lost sales and bounded inventory. Soni et al. (Citation2018) have developed an inventory model with lost sale reduction, quality improvement under the fuzzy environment. ElHafsi et al. (Citation2021) examined optimal production and inventory control of multi-class mixed backorder and lost sales demand class models.
2.6 Fuzzy demand
Most researchers consider the demand rate of the product to be uncertain, for instance, Ouyang and Yao (Citation2002), Dutta et al. (Citation2007), Björk (Citation2009), Mula et al. (Citation2010) considers the customers demand to be a fuzzy number. Moreover, Taleizadeh et al. (Citation2013) illustrated the supply chain model by considering the demands as a fuzzy rough variable. Malik and Sarkar (Citation2019) developed an inventory model based on the coordinating system with fuzzy demand and the defuzzification process is made by the signed distance method. Noh and Kim (Citation2019) derived a coordinating supply chain model between a single vendor and multi-buyer by considering the demand as a triangular fuzzy number. Karthick and Uthayakumar (Citation2020) investigated the imperfect production model with a triangular fuzzy demand.
2.7 Research gap and contribution
Contributions of various study articles from the existing literature are given in . From examining the above subsections, there has been very little research on back-order price discount and lost sales, and no inventory model has been found under the ambiguous/fuzzy context, especially since there is no model to compare these two (i.e., back-order price discount and lost sales) together. And, in particular, it is important to consider the fact that energy consumption and carbon emissions studies are limited in the literature. Of these, to our knowledge, none of the supply chain models has been reported in the literature on the imperfect inventory model with fuzzy demand, energy consumption, GHG emissions under two different scenarios, such as providing a price discount to the customers on the first scenario and in the second scenario, an investment is made in reducing lost sales without offering any discounts.
Table 1. A comparison of the present model with related existing models
The rest of the paper is given as follows: some basic definitions are given in section 3. In section 4 problem definition, notations and assumptions are given to develop the model. Section 5 contains the mathematical model of both retailer and manufacturer. In section 6–7, an integrated cost function is developed under two scenarios, one is while providing backorder price discounts to the customers and another is while retailer plans to make an investment to reduce lost sale respectively, in which two different cases are considered to relate the production rate and process quality under these scenarios. Moreover, in this paper, the demand rate is considered a triangular fuzzy number and the defuzzification process is done using the signed distance method. Two numerical examples are considered for each scenario to validate this model. Graphical representations are given to illustrate the model. In section 8, sensitivity analysis and managerial implications are given. Finally, the conclusion is given in section 9.
3 Preliminaries
Definition 3.1 (Fuzzy number) A fuzzy number is a fuzzy subset of which is both normal and convex. In addition, the membership function must be piece wise continuous. The membership function of a fuzzy number
is usually represented as
where, is continuous from the right, strictly increasing for
and there exist
< m such that
for
and
is continuous from the left, strictly decreasing for
and there exist
such that
for
and
are called the left and right reference functions respectively.
Definition 3.2 ( cut of a fuzzy set (Björk Citation2009)) The set
where
is called the
cut of
is a nonempty bounded closed interval contained in the set of real numbers and it is denoted by
Both
and
are continuous functions of
Let
and
are the left and right limits of
respectively, are usually known as the left and right α cuts of
Definition 3.3 (Triangular fuzzy number (Karthick and Uthayakumar Citation2020)) The fuzzy number is a triangular fuzzy number (see, ) if it is fully determined by
of crisp numbers such that
with membership function
where, and
are the lower limit, mode and upper limit of the fuzzy number. The interval
is called the support of the fuzzy number which gives the range of all values that are at least marginally possible or plausible.
is known as the core or the most plausible value. For the triangular fuzzy number
the left and right α cuts of
are respectively given by
and
Definition 3.4 (Signed distance method (Karthick and Uthayakumar Citation2020)) For any
is named as the signed distance from t to 0. If
then the distance from t to 0 is
if
the distance from t to 0 is
Therefore,
is known as the signed distance from t to 0 For the fuzzy set
the following expression can be obtained as
The signed distance of the interval
measured from the origin 0 is given by
For the fuzzy number the proposed defuzzification methods
(the distance from
to 0) is written as
4 Problem definition, notations and assumptions
4.1 Problem definition
The primary purpose of this article is to examine experimental parameters in two scenarios of a model – (1) when the retailer offers discounts to the customers on the backorders, (2) to minimize the lost sales without offering any discounts and these two scenarios are described by considering uncertain demand, energy consumption for manufacturing and reworking process, greenhouse gas emissions under imperfect production in a fuzzy environment. In fact, many industries speed up their production and increase its volume to deliver products to their customers faster. If there are any defects in the general mechanism during the manufacturing process, it causes the production of defective items, leading to shortages and a lost sale. To reduce the shortage/lost sale, the retailer takes the shortages as a backorder and offers a discount to the customers in the first scenario. Moreover, some customers may accept the backorders, and some may not accept that there may be an occurrence of lost sale in such cases. In the second scenario, if the retailer decided to make an investment for reducing the lost sale may be helpful to attain a minimum total cost without offering any discount. Due to imperfect production, the retailer is unable to deliver the products on time, and as a result, cannot meet the demand of the customers. Therefore, the demand for the product is likely to decrease, but it is impossible to calculate it accurately, which leads to uncertainty. Therefore, in this model, the demand is considered as a triangular fuzzy number. Moreover, under these two scenarios, the lead time demand does not follow any specific distribution but mean, and standard deviation are known.
In this paper, we develop a mathematical model using the notations and assumptions listed below.
4.3 Assumptions
1. A single-manufacturer single-retailer inventory model is considered with a single type of item.
2. Retailer follows a continuous review inventory system with partial backordering.
3. Manufacturer receives the order quantity of size per year from retailer.
4. The reorder point r = Expected demand during lead time + Safety stock, i.e.,
5. The demand during lead time is assumed to be stochastic and it doesn’t follows any specific distribution but mean and variance are known.
6. The production rate of non-defective products is greater than the demand rate.
7. A warrenty cost for each defective item is paid by the manufacturer.
8. The lead time is considered to be a function of order quantity and production rate i.e.,
where is the fixed delay due to the setup, production and transportation time.
5 Mathematical model
In this paper, a two-level supply chain model is considered between the single manufacturer and the single retailer, and it is not possible to predict whether the demand for goods is in equilibrium during the lead time between the two players. Therefore, we assume the p.d.f. of demand on the lead time belongs to the class of distribution function F with mean
and variance
. Here, the distribution of lead time X is unknown, so the definite value of
cannot be determined. Thereby, we consider the following proposition of a min-max distribution-free approach to find the value of
approximately (Gallego and Moon (Citation1993).
Proposition 5.1 For any ,
Therefore, the expected shortage is calculated by substituting the value of (by assumption (8)) in Equationequation (5
(5)
(5) )
Using assumption (4), we substitute instead of
we get
5.1 Retailer’s model
The inventory on the retailer’s side is reviewed on a continuous basis. Once the inventory reaches the level of the reorder and the retailer places the order quantity to the manufacturer. The ordering cost seems to be
. The expected inventory area of the retailer is given by
The expected shortage is calculated as
The transportation cost is considered in two manners, one with fixed transportation cost and another in variable transportation cost i.e.,
. The cost function of the retailer involves the cost of ordering, holding, shortage, and transportation. In this cost function, the shortages are allowed and it is fully backordered.
In reality, if the shortages are high, then it is not easy to take the full shortages as a backorder. Therefore, we assumed that the shortages were partially backordered and the rest to be lost sale.
5.2 Manufacturer’s model
The retailer’s order quantity is delivered by the manufacturer in n shipments. Therefore, the cost associated with the manufacturer is derived in the following: the cost for setting up the machinery (setup cost) is derived as . The expected average inventory of the vendor is given by
. Defective materials are produced due to certain problems during the manufacture of the items. Therefore, the cost for reworking a defectively produced items is given by
, where
and the cost for producing the products is considered as a function i.e.,
. The energy usage for production process is given by
, where
and the energy usage for reworking the produced items takes more energy than the regular production process i.e.,
, where
. Therefore, the specific energy consumption for both producing and reworking the products is given by
(see for instance (Marchi et al. Citation2019)). The greenhouse gas emission cost is considered due to the emission of gases during the production process is given by (Noh and Kim Citation2019) as The cost function of the vendor involves a cost for setup, holding, reworking the defective items, inspection, production, warranty, energy consumption for producing the new products, reworking defective items, and GHG emissions.
During the manufacturing of the product, if there is a malfunction in the process, the products produced will be defective. As a consequence, the quality of the goods/products has been decreased and production has been affected. As a result, two distinct functions related to the production rate and the quality of the process are considered. If the machine operation ceases, i.e. the machine is inoperative, defective products will not be produced. If the machine operates, there will be a chance that the defective items will appear. Therefore, the mean time to failure (MTTF) is assumed to be independent of the production rate in practical issues is inappropriate. It is perfectly suitable to assume that the MTTF function is strictly decreasing the production rate. A strictly increasing function of P is considered from (Khouja and Mehrez Citation1994), so that the MTTF
becomes a strictly decreasing function of P. Two distinct cases with two distinct functions were implemented to describe MTTF:
Case 1. (The quality function
is linear in P)
Case 2. (The quality function
is quadratic in P)
where and
are the non-negative real numbers that will provide the good approximation for
as well as
.
6 Scenario 1: Integrated methodology with a discount on backorders
The price discount on backorder is provided by the retailer to encourage the customers to wait for their late delivery and to prevent from the loss on the shortages and the backorder ratio is proportional to the backorder price discount , i.e.,
The expected inventory area of the retailer is given by
and the expected shortage is calculated as
Then the expected total cost of the retailer while providing the backorder discount seems to be,
Hence, the integrated cost is obtained by adding cost function of retailer (11) and manufacturer (10).
subject to
Therefore, this objective function is divided into two sub cases with respect to the outcome of defective items during the production process.
6.1 Case 1. While the quality function 
is linear in the production rate
By substituting , the integrated cost function (12) becomes
subject to
Fuzzification
Several researchers have considered the demand rate as constant or dependent on certain variables such as selling price, stock level, etc. Moreover, in some situation, it is not easy to predict the exact demand. Therefore, in this model, the demand rate is considered as the non-negative triangular fuzzy number, i.e.,
The fuzzified cost function is given in Appendix E.
Defuzzification
We can not obtain the results of the proposed model by means of a fuzzified integrated cost function. Therefore, it is necessary to convert the fuzzy number into crisp values, which can be achieved through the defuzzification process.
subject to
, a positive integer.
Here, the process of defuzzification can be performed by numerous methods, here we have used a signed distance method for simplicity, i.e., (by definition 3.4)
subject to .
Solution procedure
In this subsection, we derive the optimal values of and prove the convexity of the integrated cost function
with respect to the decision variables (i.e.,
). Before determining optimal values, the given integrated cost function (17) is non-linear, so first, we ignore the constraints and take first-order partial derivatives of (17) with respect to the decision variables
, which are given below.
On equating (18), (19), (20) and (21) to zero, we obtain the values of , k,
and P. To achieve the global minimum of the entire supply chain model, the second-order partial derivative of
is taken with respect to
and P.
where,
Proposition 6.1 For fixed values of ,
, P and n, the objective function
(17) is convex in k, there exists a unique value for the safety factor
that minimizes the cost function
. For fixed values of
, k, P and n, the objective function
(17) is convex in
, there exists a unique value for the backorder price discount
that minimizes the cost function
.
Proof. Appendix A.
Proposition 6.2 For fixed values of , k,
and P,
(17) is a convex function on n.
Proof. Appendix B.
6.2 Case 2. While the quality function 
is quadratic in the production rate
By substituting , the integrated cost function (12) becomes
subject to
The following fuzzification and defuzzification are done similar to the subsection 6.1. And the fuzzified cost function of this particular case is given in Appendix E.
Defuzzification
To defuzzify the cost function (45), we substitute , therefore we obtain the defuzzified cost as
subject to .
Solution procedure
The optimal values are obtained by taking first order partial derivatives of (23) with respect to the decision variables and P, by ignoring the constraints, which are given below.
On equating (24), (25), (26) and (27) to zero, we obtain the values of , k,
, and P. To achieve the global minimum of the entire supply chain model, the second-order partial derivative of
is taken with respect to
, k,
, and P.
where,
Proposition 6.3 For fixed values of ,
, P and n, the objective function
(23) is convex in k, there exists a unique value for the safety factor
that minimizes the cost function
. For fixed values of
, k, P and n, the objective function
(23) is convex in
, there exists a unique value for the backorder price discount
that minimizes the cost function
.
Proof. Appendix A.
Proposition 6.4 For fixed values of , k,
and P,
(23) is a convex function on n.
Proof. Appendix D.
6.3 Algorithm
Step 1 Set where,
(for Case 1, 2).
Step 2 For each do the following steps.
Step 2(i) First, initialize the values for kj = 0, πjx = π0 and Pj .
Step 2(ii) Using the values of and compute
by solving (18) and (24).
Step 2(iii) Using the values of and compute
by solving (19) and (25).
Step 2(iv) Using the values of and compute
by solving (20) and (26).
Step 2(v) Repeat the steps until the values of
,
,
(
), occurs no change and denote the values as
,
,
.
Step 2(vi) Using the values of compute
by solving (21) and (27).
Step 3 Compute using the values of
,
,
and
.
Step 4 Set n = n + 1, repeat the Steps from 2 to 3.
Step 5 =
and we obtain
as the optimal solution.
6.4 Numerical examples
Example 6.1 Let us consider the following numerical data to illustrate the above model.
For retailer:
For manufacturer:AV= 4000, shows the optimal values of the decision variables. The graphic representations in and shows how the effect of the order quantity
and the rate of production P affects the joint total cost (
and
) respectively.
Example 6.2 Let us consider the following numerical data to illustrate the above model.
Table 2. Optimal values of example 6.1 for case 1 and case 2 (scenario 1)
Figure 2. Scenario: 1. The effects of order quantity and production rate on the combined total cost of case 1 and case 2
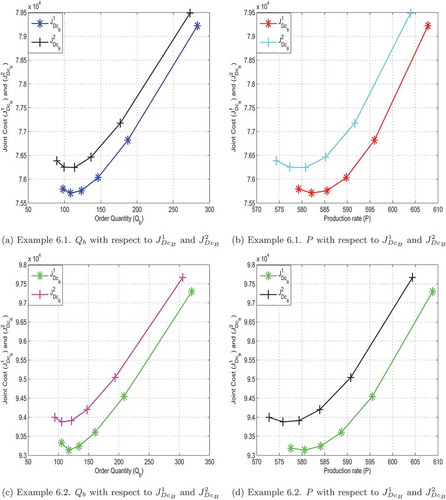
For retailer:
For manufacturer: shows the optimal values of the decision variables. The graphic representations in and shows how the effect of the order quantity
and the rate of production P affects the joint total cost (
and
) respectively. Energy usage and carbon emission is determined using the optimal values, which are listed in .
Table 3. Optimal values of example 6.2 for case 1 and case 2 (scenario 1)
Table 4. Scenario 1. Specific energy usage and carbon emission
7 Scenario 2. Integrated methodology with reducing a lost sales
In many cases, the back-order system may not fully help the retailer, and may sometimes lose sales, so some authors have done their part to reduce lost sales in some manner (see, for instance, (Ouyang and Chang Citation2001), Hwang et al. (Citation2013) and ElHafsi et al. (Citation2021)).
In this section, the retailer doesn’t offer any price discount to the customers instead that retailer made an investment for reducing the lost sale, for convenience The investment
is a logarithmic function for reducing the lost sale. i.e.,
where . The cost function of the buyer,
The integrated cost function is developed by adding cost function of retailer (28) and manufacturer (10), we obtain
subject to
7.1 Case 1. While the quality function 
is linear in the production rate
By substituting , the integrated cost function (29) becomes
subject to
The fuzzified cost function of this case is given in Appendix F.
Defuzzification
Inorder to defuzzify the cost function, we substitute , then (46) turns into the following equation
subject to
Solution procedure
In order to obtain the optimal values of the decision variables, we take first order partial derivative of (31) with respect to the and P, by ignoring the constraints and equating to zero.
To achieve the global minimum of the entire supply chain model, the second-order partial derivative of is taken with respect to
and P.
where,
Proposition 7.1 For fixed values of , ρ, P and n, the objective function
(31) is convex in k, there exists a unique value for the safety factor
that minimizes the cost function
. For fixed values of
, k, P and n, the objective function
(31) is convex in ρ, there exists a unique value for the lost sale
that minimizes the cost function
.
Proof. Appendix C.
Proposition 7.2 For fixed values of , k,
and P,
(31) is a convex function on n.
Proof. Appendix B.
7.2 Case 2. While the quality function 
is quadratic in the production rate
By substituting , the integrated cost function (12) becomes
subject to
The fuzzified cost function of this case is given in Appendix F.
Defuzzification
We follow the same procedure for the defuzzification process, by substituting on (47), we obtain the defuzzified cost as
subject to
Solution procedure
To obtain the optimal values of the decision variables, we take first order partial derivative of (37) with respect to the and P, by ignoring the constraints and equating to zero.
To achieve the global minimum of the entire supply chain model, the second-order partial derivative of is taken with respect to
and P.
where,
Proposition 7.3 For fixed values of , ρ, P and n, the objective function
(37) is convex in k, there exists a unique value for the safety factor
that minimizes the cost function
. For fixed values of
, k, P and n, the objective function
(37) is convex in ρ, there exists a unique value for the lost sale
that minimizes the cost function
.
Proof. Appendix C.
Proposition 7.4 For fixed values of , k, ρ and P,
(37) is a convex function on n.
Proof. Appendix D.
7.3 Algorithm
Step 1 Set where,
(for cases 1, 2).
Step 2 For each do the following steps.
Step 2(i) First, initialize the values for .
Step 2(ii) Using the values of and compute
by solving (32) and (38).
Step 2(iii) Using the values of and compute
by solving (33) and (39).
Step 2(iv) Using the values of and compute
by solving (34) and (40).
Step 2(v) Repeat the steps until the values of
,
,
(
), occurs no change and denote the values as
,
,
.
Step 2(vi) Using the values of and compute
solving (35) and (41).
Step 3 Compute using the values of
,
,
and
.
Step 4 Set , repeat the Steps from 2 to 3.
Step 5 =
and we obtain
as the optimal solution.
7.4 Numerical examples
Example 7.1 Let us consider the following numerical data to illustrate the above model.
For retailer:
For manufacturer:
The optimal values of the decision variables are shown in using the above data. The graphic representations in and shows how the effect of the order quantity and the production rate P affects the joint total cost (
and
) respectively.
Example 7.2 Let us consider the following numerical data to illustrate the above model.
For retailer:
. For manufacturer:
The optimal values of the decision variables are shown in using the above data. The graphic representations in and shows how the effect of the order quantity
and the rate of production P affects the joint total cost (
and
) respectively. shows the energy usage and carbon emission determined using the optimal values.
Table 5. Optimal values of example 7.1 for case 1 and case 2 (scenario 2)
Table 6. Optimal values of example 7.2 for case 1 and case 2 (scenario 2)
Table 7. Scenario 2. specific energy usage and carbon emission
8 Sensitivity analysis and managerial implications
In this section, sensitivity analysis is performed for the core parameters of the proposed model. The parameters which are considered for this analysis are and R. The sensitivity results are provided in . (4a, 4b, 4 c, 4d, 4e, 4 f, 4 g) and (5a, 5b, 5 c, 5d, 5e, 5 f, 5 g) illustrates sensitivity graph of case 1 and case 2 in both scenarios respectively.
is found to be more sensitive compared with other parameters.
and
are found to be slightly sensitive than
and R.
Table 8. Sensitivity analysis
Figure 4. Sensitivity analysis of and R with respect to the joint cost for Scenario – 1 and 2 (Case-1)
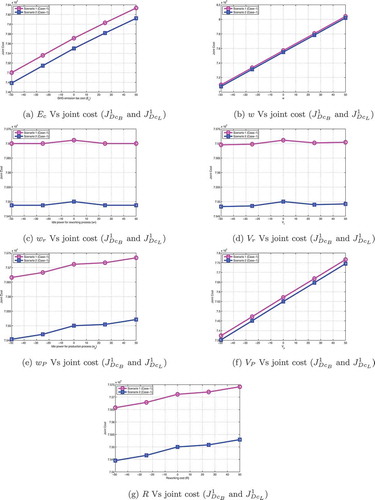
Figure 5. Sensitivity analysis of and R with respect to the joint cost for Scenario – 1 and 2 (Case-2)
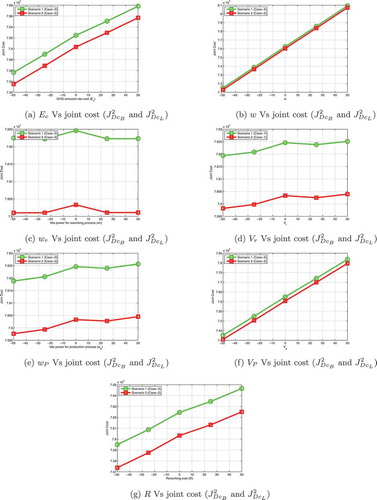
From this article, managers can explore a variety of ways to create better deals and limit the production of products without harming the environment. By looking at the high range of overall (GHG) emissions, manufacturing companies can optimise their current resources and make full use of all available resources and determine how much of each product they can afford to stay within the GHG emissions range. Higher production levels affect environmental performance, such as energy consumption and greenhouse gas emissions. When looking at the specific energy use from both scenarios, it is almost identical. Also, carbon emissions appear to be slightly higher in the second scenario than in the first scenario. Defective materials are exposed in the event of a malfunction in the engine during rapid production, so this model is capable of adapting production to suit the environment. In addition, managers can take advantage of this model to determine whether discounting the cost of goods to the customer reduces the total cost or reduces the total cost by reducing lost sales despite offering no discounts.
9 Conclusion
This article is designed to reduce the total cost in two different scenarios, including the need for energy during perfect and imperfect production, greenhouse gas emissions, and when the demand for the product is fuzzy (ambiguous) under the integrated green supply chain model. In order to relate process quality and production rate, two different cases are explored in each situation. This research aims to analyse which scenario is appropriate to obtain the minimum total cost in the fuzzy environment. The retailer’s demand is considered to be a triangular fuzzy number because the demand for the product may be ambiguous under certain circumstances. The reworking process is considered if there are any defective items produced during the production. In this regard, we have compared the single supply chain model in two scenarios, whereby investing to reduce lost sales enables supply chain partners to obtain a minimum total cost than providing discounts on backorder.
The proposed model’s extension can be implemented in several ways by treating the demand rate with different fuzzy numbers such as the trapezoidal, pentagonal and hexagonal fuzzy number. Considering multiple retailers with multiple items would be a reasonable extension of this model. If the greenhouse emissions exceed the limit, the penalty cost of expelling more gases from industries would be another beneficial extension of this model. The practical study of inspection process under controllable lead time would be another extension. Moreover, this model can be extended by recycling unwanted and used materials to create a circular economy model. Furthermore, this model can be extended by considering the carbon emission function depending on the production rate.
Acknowledgments
The work of the authors are supported by UGC - SAP (DSA - I), Department of Mathematics, The Gandhigram Rural Institute (Deemed to be University) Gandhigram, Dindigul District, Tamil Nadu, India. Pincode: 624 302.
Disclosure statement
No potential conflict of interest was reported by the author(s).
Additional information
Notes on contributors
B. Karthick
B. Karthick received his Bachelor of Science and Master of Science degrees in Mathematics in 2016 and 2018, respectively, from The Gandhigram Rural Institute (Deemed to be University), Gandhigram, Tamilnadu, India. He is currently a Full-Time PhD Research Scholar in the Department of Mathematics, The Gandhigram Rural Institute (Deemed to be University), Gandhigram, Tamilnadu, India. His current research interests include operations research, inventory control, fuzzy optimization and supply chain management.
R. Uthayakumar
R. Uthayakumar obtained his Master of Science in Mathematics in 1989 from The American College, Madurai, Tamilnadu, India. He completed his Master of Philosophy in Mathematics at Madurai Kamaraj University, Madurai, Tamilnadu, India. He is currently a Professor in the Department of Mathematics, The Gandhigram Rural Institute (Deemed to be University), Gandhigram, Tamilnadu, India. He has published about 220 articles in national and international journals. His research interests include fractal geometry, optimization techniques, inventory control, fuzzy decision making and supply chain management.
References
- Bazan, E., M. Y. Jaber, and S. Zanoni. 2015. “Supply Chain Models with Greenhouse Gases Emissions, Energy Usage and Different Coordination Decisions.” Applied Mathematical Modelling 39 (17): 5131–5151. doi:10.1016/j.apm.2015.03.044.
- Björk, K. M. 2009. “An Analytical Solution to a Fuzzy Economic Order Quantity Problem.” International Journal of Approximate Reasoning 50 (3): 485–493. doi:10.1016/j.ijar.2008.10.001.
- Cheng, T. C. E. 1991. “An Economic Order Quantity Model with Demand-dependent Unit Production Cost and Imperfect Production Processes.” IIE transactions 23 (1): 23–28. doi:10.1080/07408179108963838.
- Dutta, P., D. Chakraborty, and A. R. Roy. 2007. “An Inventory Model for Single-period Products with Reordering Opportunities under Fuzzy Demand.” Computers & Mathematics with Applications 53 (10): 1502–1517. doi:10.1016/j.camwa.2006.04.029.
- ElHafsi, M., J. Fang, and E. Hamouda. 2021. “Optimal Production and Inventory Control of Multi-class Mixed Backorder and Lost Sales Demand Class Models.” European Journal of Operational Research 291 (1): 147–161. doi:10.1016/j.ejor.2020.09.009.
- Gallego, G., and I. Moon. 1993. “The Distribution Free Newsboy Problem: Review and Extensions.” Journal of the Operational Research Society 44 (8): 825–834. doi:10.1057/jors.1993.141.
- Ganesh Kumar, M., and R. Uthayakumar. 2019. “Multi-item Inventory Model with Variable Backorder and Price Discount under Trade Credit Policy in Stochastic Demand.” International Journal of Production Research 57 (1): 298–320. doi:10.1080/00207543.2018.1480839.
- Hayek, P. A., and M. K. Salameh. 2001. “Production Lot Sizing with the Reworking of Imperfect Quality Items Produced.” Production Planning & Control 12 (6): 584–590. doi:10.1080/095372801750397707.
- Herbon, A. 2020. “An Approximated Solution to the Constrained Integrated Manufacturer-buyer Supply Problem.” Operations Research Perspectives 7: 100140. doi:10.1016/j.orp.2020.100140.
- Hwang, H. C., W. Van Den Heuvel, and A. P. Wagelmans. 2013. “The Economic Lot-sizing Problem with Lost Sales and Bounded Inventory.” IIE Transactions 45 (8): 912–924. doi:10.1080/0740817X.2012.724187.
- Jaber, M. Y., C. H. Glock, and A. M. El Saadany. 2013. “Supply Chain Coordination with Emissions Reduction Incentives.” International Journal of Production Research 51 (1): 69–82. doi:10.1080/00207543.2011.651656.
- Karthick, B., and R. Uthayakumar. 2020. “Optimizing an Imperfect Production Model with Varying Setup Cost, Price Discount, and Lead Time under Fuzzy Demand.” Process Integration and Optimization for Sustainability 1–17. doi:10.1007/s41660-020-00133-8.
- Karthick, B., and R. Uthayakumar. 2021. “A Multi-Item Sustainable Manufacturing Model with Discrete Setup Cost and Carbon Emission Reduction under Deterministic and Trapezoidal Fuzzy Demand.” Process Integration and Optimization for Sustainability 1–39. doi:10.1007/s41660-021-00159-6.
- Khouja, M., and A. Mehrez. 1994. “Economic Production Lot Size Model with Variable Production Rate and Imperfect Quality.” Journal of the Operational Research Society 45 (12): 1405–1417. doi:10.1057/jors.1994.217.
- Liao, G. L., Y. H. Chen, and S. H. Sheu. 2009. “Optimal Economic Production Quantity Policy for Imperfect Process with Imperfect Repair and Maintenance.” European Journal of Operational Research 195 (2): 348–357. doi:10.1016/j.ejor.2008.01.004.
- Lin, Y. J. 2008. “Minimax Distribution Free Procedure with Backorder Price Discount.” International Journal of Production Economics 111 (1): 118–128. doi:10.1016/j.ijpe.2006.11.016.
- Malik, A. I., and B. Sarkar. 2019. “Coordinating Supply-Chain Management under Stochastic Fuzzy Environment and Lead-Time Reduction.” Mathematics 7 (5): 480. doi:10.3390/math7050480.
- Marchi, B., S. Zanoni, L. E. Zavanella, and M. Y. Jaber. 2019. “Supply Chain Models with Greenhouse Gases Emissions, Energy Usage, Imperfect Process under Different Coordination Decisions.” International Journal of Production Economics 211: 145–153. doi:10.1016/j.ijpe.2019.01.017.
- Mula, J., D. Peidro, and R. Poler. 2010. “The Effectiveness of a Fuzzy Mathematical Programming Approach for Supply Chain Production Planning with Fuzzy Demand.” International Journal of Production Economics 128 (1): 136–143. doi:10.1016/j.ijpe.2010.06.007.
- Noh, J., and J. S. Kim. 2019. “Cooperative Green Supply Chain Management with Greenhouse Gas Emissions and Fuzzy Demand.” Journal of Cleaner Production 208: 1421–1435. doi:10.1016/j.jclepro.2018.10.124.
- Ouyang, L. Y., and H. C. Chang. 2001. “The Effects of Investing in Lost Sales Reduction on the Stochastic Inventory Models.” Journal of Information & Optimization Sciences 22 (2): 357–368. doi:10.1080/02522667.2001.10699497.
- Ouyang, L. Y., and J. S. Yao. 2002. “A Minimax Distribution Free Procedure for Mixed Inventory Model Involving Variable Lead Time with Fuzzy Demand.” Computers & Operations Research 29 (5): 471–487. doi:10.1016/S0305-0548(00)00085-X.
- Plambeck, E. L. 2012. “Reducing Greenhouse Gas Emissions through Operations and Supply Chain Management.” Energy Economics 34: S64–S74. doi:10.1016/j.eneco.2012.08.031.
- Rosenblatt, M. J., and H. L. Lee. 1986. “Economic Production Cycles with Imperfect Production Processes.” IIE transactions 18 (1): 48–55. doi:10.1080/07408178608975329.
- Safarzadeh, S., and M. Rasti-Barzoki. 2019. “A Game Theoretic Approach for Assessing Residential Energy-efficiency Program considering Rebound, Consumer Behavior, and Government Policies.” Applied Energy 233: 44–61. doi:10.1016/j.apenergy.2018.10.032.
- Salameh, M. K., and M. Y. Jaber. 2000. “Economic Production Quantity Model for Items with Imperfect Quality.” International Journal of Production Economics 64 (1–3): 59–64. doi:10.1016/S0925-5273(99)00044-4.
- Sarkar, B., A. Majumder, M. Sarkar, N. Kim, and M. Ullah. 2018. “Effects of Variable Production Rate on Quality of Products in a Single-vendor Multi-buyer Supply Chain Management.” The International Journal of Advanced Manufacturing Technology 99 (1–4): 567–581. doi:10.1007/s00170-018-2527-3.
- Sarkar, B., B. Mandal, and S. Sarkar. 2015. “Quality Improvement and Backorder Price Discount under Controllable Lead Time in an Inventory Model.” Journal of Manufacturing Systems 35: 26–36. doi:10.1016/j.jmsy.2014.11.012.
- Sarkar, B., M. Omair, and S. B. Choi. 2018. “A Multi-objective Optimization of Energy, Economic, and Carbon Emission in A Production Model under Sustainable Supply Chain Management.” Applied Sciences 8 (10): 1744. doi:10.3390/app8101744.
- Sarkar, B., S. S. Sana, and K. Chaudhuri. 2011. “An Economic Production Quantity Model with Stochastic Demand in an Imperfect Production System.” International Journal of Services and Operations Management 9 (3): 259–283. doi:10.1504/IJSOM.2011.041100.
- Soni, H. N., B. Sarkar, A. S. Mahapatra, and S. K. Mazumder. 2018. “Lost Sales Reduction and Quality Improvement with Variable Lead Time and Fuzzy Costs in an Imperfect Production System.” RAIRO-Operations Research 52 (3): 819–837. doi:10.1051/ro/2016075.
- Taleizadeh, A. A., H. M. Wee, and F. Jolai. 2013. “Revisiting a Fuzzy Rough Economic Order Quantity Model for Deteriorating Items considering Quantity Discount and Prepayment.” Mathematical and Computer Modelling 57 (5–6): 1466–1479. doi:10.1016/j.mcm.2012.12.008.
- Ugarte, G. M., J. S. Golden, and K. J. Dooley. 2016. “Lean versus Green: The Impact of Lean Logistics on Greenhouse Gas Emissions in Consumer Goods Supply Chains.” Journal of Purchasing and Supply Management 22 (2): 98–109. doi:10.1016/j.pursup.2015.09.002.
Appendix A
On taking second-order partial derivatives of (17) and (23) with respect to k and , we get
where, This shows that
and
is convex on k and
. Hence, this completes the proof of Proposition 6.1 and 6.3.
Appendix B
If we take the first and second-order partial derivatives of (17) and (31) with respect to n, we get
where,
Therefore, for fixed values of , k,
, ρ, P, the total cost
and
is convex on n. Hence, this completes the proof of Proposition 6.2 and 7.2.
Appendix C
On taking second-order partial derivatives of (31) and (37) with respect to k and ρ, we get
where, This shows that
and
is convex on k and
. Hence, this completes the proof of Proposition 7.1 and 7.3.
Appendix D
If we take the first and second-order partial derivatives of (23) and (37) with respect to n, we get
where,
Therefore, for fixed values of , k,
, ρ, P. This shows that
and
is convex in n. Hence, this completes the proof of Proposition 6.4 and 7.4.
Appendix E
For Scenario 1
Case 1
On substituting (14) in (13), we get
subject to .
Case 2
By substituting instead of D in the cost function (22), then we obtain the following fuzzified cost function.
subject to .
Appendix F
For Scenario 2
Case 1
To fuzzify the integrated cost function, we substitute where,
, then (30) becomes
subject to
Case 2
To fuzzify the integrated cost function, we substitute , then (36) becomes
subject to