ABSTRACT
Due to recent technological advancements, automation and autonomous solutions are gaining increasing popularity. Yet, a lack of in-depth investigations is noticed on the potential environmental benefits and economic repercussions of implementing autonomous systems. The present study aims to fill part of this gap by quantifying the environmental and economic sustainability of a robotic lawn mower, in comparison with human-operated counterparts. Combining life cycle assessment and life cycle costing methodologies, and by defining adequate functional units, building simulation models, and collecting life cycle inventory data, a systematic comparative study between autonomous and conventional lawn mowers is performed on their environmental and economic impacts. Through this multi-indicator analysis, environmental and economic trade-offs between the autonomous and conventional mowing solutions are quantitatively discussed for key relevant usage scenarios, from mowing an average residential yard to maintaining larger fields like a football stadium or a schoolyard. Concretely, sensitivity analyses on key parameters influencing the performance of the autonomous mower have been conducted to evaluate the environmental and economic benefits of an augmented robotic mower. While optimising the path planning of the current robotic mower would lead to the most substantial savings, improvements on the battery performance, cutting width, and speed of the autonomous solution appear as other promising areas for future work.
1. INTRODUCTION
1.1. CONTEXT AND MOTIVATIONS
Automation and autonomous solutions are increasingly considered as promising and timely solutions to enhance the safety, reliability, and productivity of human-operated tasks. Automation is a set of human-defined functions performed by a robot or piece of equipment. Autonomy is a state in which a robot or piece of equipment operates independently, without explicit instructions from a human. For the Society of Automotive Engineers (SAE International Citation2016), there are five levels of autonomy from Level 0 for the human driver doing everything (all manned vehicles), to Level 4 being an automated system that can perform all driving tasks (no local supervision, remote supervision or artificial intelligence) under all conditions that a human driver could perform, through intermediate levels (manned back-up, or in-field supervision of unmanned vehicles). A large number of industries are implementing state-of-the-art automated systems, such as: automotive industry, agriculture industry, aerospace industry, defence industry, mining industry, energy industry, or food industry (Productivity Inc Citation2019). A review of existing autonomous systems in several industries has emphasised that the sustainability and related environmental impact of such autonomous systems are barely studied and quantified in comparison to conventional human-operated systems, except in the automotive industry with an increased focus on connected and autonomous vehicles. The potential promising benefits of automation – which still need to be validated quantitatively with sound and transparent studies – include: (i) a faster return on investment, a priori due to lower operating costs, reduced lead times, and increased output; and (ii), a smaller environmental footprint, by streamlining equipment and processes, reducing scrap and using less energy. Despite promises of increased efficiency (Kurilova-Palisaitiene et al. Citation2017; Bahri and Ouled Amor Citation2019), it is not clear whether the paradigm shift towards autonomous systems will change ‘how we decide when our self-interest (e.g., comfort) is pitted against the collective interest (e.g., environment)’ (De Melo, Marsella, and Gratch Citation2019). In fact, Nouzil et al. (Citation2017) reviewed the sustainable impacts of automation on society and qualitatively discussed how automation could affect our society, by considering four dimensions: ecology, economics, politics, and culture. When it comes to the design and development of autonomous technical solutions, it has been noticed that most studies do not comprehensively consider the societal, environmental, and economic impacts of automation. Particularly, a lack of life cycle analysis on automation processes and autonomous systems has been observed. Further research is therefore needed to study the environmental effect of automation technologies through life cycle assessment to better understand their ecological footprint.
1.2. RESEARCH APPROACH, OBJECTIVES AND EXPECTED CONTRIBUTIONS
In this line, this research initiates the measurement of the environmental impacts of such new automated technologies in other sectors than the automotive industry, by wondering how automation could be used to build more sustainable machines in the gardening industry. Through the case study on a robotic lawn mower, put in comparison with human-operated mowers, the questions that this study sets out to answer are: (i) whether the automation of lawn service is making sense environmentally and economically, and (ii) under which conditions it could provide further sustainable benefits. Therefore, the present research work focuses on the contributions of autonomous systems to sustainability, by questioning here the relevance, implication, and applicability for lawn mowing equipment. After a literature survey and critical analysis of existing autonomous solutions, including a review of state-of-the-art examples (section 2), an original and in-depth case study is conducted to assess and compare the sustainability performance of autonomous mowers with their human-driven counterparts, as well as to discuss the conditions (e.g., enhanced design, augmented features, appropriate use modes) for further economic profitability and environmental savings. To do so, comparative life cycle assessment (LCA) and life cycle costing (LCC) are deployed on autonomous solutions and their human-operated counterparts (all details being presented in section 3). Interestingly, the impact of different usage scenarios for the autonomous solution is compared to conventional lawn mowing activities (sub-sections 4.1 and 4.2). Beyond the technical aspect of this study and interpretation of the results that might interest original equipment manufacturers, consumers or policy-makers, insights from this applied study also aim to bring new elements of discussion to the proper comparative environmental assessment between autonomous systems and traditional ones. As such, the present piece of research brings new relevant data related to the use phase of autonomous lawn mower, with their current limitations and areas of improvements, as well as sheds fresh lights on the life cycle assessment of autonomous solutions to help design and develop more-sustainable products (sub-section 4.3).
2. LITERATURE SURVEY
To identify and analyse relevant prior works on this topic, a screening of the literature available on autonomous systems through the light of sustainability has been conducted using a combination of the following keywords in the main database for research papers:
Keywords: autonomy, autonomous, automation, systems, equipment, sustainability, environmental, impact, life cycle, assessment, evaluation, performance.
Database: Google Scholar, Scopus, Science Direct, for academic literature; and Google search, for grey literature including industrial and technical reports.
A search of this literature revealed that recent studies have begun to explore quantitatively the potential energy and greenhouse gas emission impacts of connected and automated vehicles (CAVs) (Gawron et al. Citation2018; Kopelias et al. Citation2020). Gawron et al. (Citation2018) reported the results of a comparative life cycle assessment (LCA) between a CAV and a conventional internal combustion engine vehicle. The functional unit defined for conducting this comparative LCA was as follows: ‘a passenger car with a service life of 160,000 miles traveled over 12 years’. This study particularly explored the trade-offs between the increased environmental impacts from adding autonomous vehicle equipment and the expected gains in driving efficiency. Indeed, to be operational, these CAVs are required to be equipped with multiple cameras, sonar, radar, LiDAR, a GPS navigation system, a computer, and support structures. Their findings showed that the sensing and computing subsystems in CAVs could increase a vehicle’s energy use and greenhouse gas emissions (GHG) from 3 to 20 percent due to increases in power consumption, weight, and aerodynamic drag. Yet, when potential operational benefits of such autonomous vehicles are included (including, e.g., eco-driving, platooning, and intersection connectivity), the net result can be up to a 9% reduction in energy use and GHG emissions in the base case scenario (Gawron et al. Citation2018). Kopelias et al. (Citation2020) provided a review of the studies estimating the potential environmental impacts induced by the implementation of these CAVs in the market and in road traffic. Eleven factors affecting the environmental performance of the CAVs were identified and categorised based on whether they are related to the vehicle, the road network, or the user.
While most research on the sustainable performance of autonomous systems has been carried out on CAVs, the use of autonomous solutions in other sectors has not been investigated in any systematic way. Dusik et al. (Citation2019) stated that ‘the uptake of automation is typically supported through implicit public policies that are not subject to formal environmental assessments. In the absence of rigorous studies, it is often suggested that new technologies will help to solve the most urgent sustainability challenges.’ It becomes thus of the utmost importance to conduct scientifically sound studies that compare the impact of implementing autonomous systems from a sustainability standpoint. In their working paper, they explored the potential environmental impacts associated with the deployment of several key automation technologies over the next decade, including advanced industrial robotics and autonomous transports. As a first assessment, these automation technologies are qualitatively evaluated through best and worst-case scenarios (from significant positive impact to significant adverse impact), and according to four complementary indicators (greenhouse gases (GHG) emissions, non-GHG emissions, resource use, and ecosystem use). The authors mentioned their working paper is just a first step that can serve as a primary relevant basis to foster in-depth analyses and invited researchers for more refined studies in this area of sustainable automation (Dusik et al. Citation2019).
Indeed, further empirical investigations are needed into the environmental impacts of other growing and promising autonomous technologies, such as autonomous tractors in the agricultural industry and intelligent robotics in smart home hubs (e.g., self-cleaning vacuums and autonomous mowers). In order to contribute to filling the gap on the lack of quantitative research addressing the potential environmental sustainability of autonomous systems, especially in the agriculture or gardening industry, a real-world case study is performed here on a robotic autonomous mower system. This study aims to quantitatively assess the sustainable performance of this autonomous solution, in terms of environmental saving and economic profit, of different usage scenarios compared to conventional lawn mowing activities. It is hoped that this research will generate fresh insights related to the sustainability impacts of implementing autonomous systems for agricultural and gardening activities, in comparison to the traditional use of human-driven systems.
3. MATERIALS AND METHODS
3.1. PRESENTATION OF THE SYSTEM: ROBOTIC LAWN MOWER
The farming and gardening industry is an interesting application field for this study as it appears particularly relevant for the uptake and deployment of autonomous systems, considering the following elements. First, it is a labour-intensive industry, with constrained time windows for the operations that need to be performed. Second, one can argue the implementation of such autonomous equipment can be easier and further operational in an off-road land area compared to the deployment of on-road autonomous vehicles, facing a lot of issues related to reliability and safety. The autonomous lawn mowing solution analysed in this study is a relatively small mower that constantly maintains a lawn according to a user-defined schedule and a mowing zone that is dictated by a buried boundary wire. The movement or walk of this autonomous mower is currently dictated by ‘bouncing’ off the boundary wire at a random angle. It has the capability to find its own charging station (also included in the scope of the analysis), routinely charges itself, is completely weatherproof, and could thus operate on a 24 hour/7 day basis. The robotic mower consideration here, in its current version, is already available on the European market (mainly in Germany, France, and the UK) at a price of 2665. USD And as autonomous mowers become more capable of cutting larger areas, it is estimated this market will grow. For instance, this autonomous solution is expected to enter the US market in 2020–2021.
3.2. METHODOLOGY: COMPARATIVE LIFE CYCLE ASSESSMENT (LCA) AND COSTING (LCC)
3.2.1. Goal and scope definition, functional unit, and system boundaries
Throughout this case study, life cycle assessment (LCA) and life cycle costing (LCC) methodologies are deployed to evaluate the environmental impacts and total cost of ownership induced by mowing and maintaining a given field area, using either: a conventional riding mower (electric- and gasoline-powered one), a conventional pushing mower (electric- and gasoline-powered one), an autonomous electric mower (with current and realistic improved features), or a fleet of autonomous mowers (with current and realistic improved features), according to the size of the field to mow. The application of LCA methodology (ISO 14,040–44, International Organization for Standardization Citation2006b) is acknowledged to be a sound approach when it comes to comparing the environmental impact of product alternatives. LCC is the equivalent of LCA for the economic side. Combining LCA and LCC is particularly relevant for a more comprehensive sustainability analysis as it allows trade-off analysis between product alternatives (Guinee et al. Citation2011; Kwak and Kim Citation2013; Saidani et al. Citation2019a). The primary phase of an LCA, namely the goal and scope definition (ISO 14,040, International Organization for Standardization Citation2006a), includes the identification of the product system boundaries (which processes are to be included in the assessment) and the functional unit (that quantifies the functions or services delivered by the product system, to which all impacts will be scaled).
First, to make a sound comparison between these autonomous lawn mowers and conventional pushing or riding mowers, defining an appropriate functional unit (FU) is of the utmost importance. A well-defined FU enables scientifically sound (consistent and unbiased) comparison between different product systems and scenarios. FU is defined as a ‘quantified performance of a product system for use as a reference unit’ (ISO 14,040, International Organization for Standardization Citation2006a) and the ISO standard 14,044 (International Organization for Standardization Citation2006b) recommends that the FU should be clearly defined and measurable. Although no further guideline exists in the ISO standards to define a FU, numerous authors have proposed elements to structure FUs (Cooper Citation2003; Collado-Ruiz and Ostad-Ahmad-Ghorabi Citation2010). To reduce the variability and uncertainty around the choice of a FU, Cluzel, Leroy, and Yannou (Citation2013) have proposed a more structured and unified framework, in accordance with the Joint Research Center (EC-European Commission Citation2010). On this basis, they identified that five key elements have to be included in the definition of a sound FU: (i) verb (functional analysis); (ii) what (form of the output); (iii) how much (magnitude); (iv) how well (performance); (v) for how long (duration, time horizon of the analysis). Also, the functional unit has to be tied directly to the goals of the analysis and to the audience that the LCA is addressing (Caffrey and Veal Citation2013). The main objective of the present study is to evaluate the life cycle environmental impact and economic performance of autonomous mowers compared to human-operated ones. Furthermore, the challenge is how to compare adequately the sustainable performance of a human-operated system with an autonomous one having the same primary function, but having non-negligible differences in terms of features and constraints, plus a certain degree of uncertainty related their usage scenarios.
The performance and constraints of the autonomous solution compared to the conventional one has thus to be investigated in order to help defining an appropriate FU. According to technical experts, three specific constraints have to be considered for the autonomous mower: (i) a boundary wire constraint (i.e. how large of an area can be covered by the actual 350 metres of boundary wires); (ii) a battery constraint (how large of an area can the robotic mower cover given its 90 min work time and 33 cm/s speed); and, (iii) a time-window mowing constraint (how large of an area can robotic cover before a grass grow from a minimum to a maximum acceptable height, which is important information for larger area such as football field or golf course). These constraints were used to quantify the workable area of robotic and to come up with realistic and feasible scenarios by deploying whether one robotic mower or a fleet of robotic mowers to accomplish the task(s) described in the FU. With this background, in order to compare these mowers on a technically equivalent basis, an example of a proper functional unit for the residential garden scenario (see use case #1.1 described in the next sub-section) is : ‘Maintaining the lawn of a 0.25 acre (1000 m2) garden (average US residential grass surface) under a height of 2.5 inches (6.35 cm) (recommended height for a cool-season grass), 26 weeks a year (mowing season), for 10 years (lifespan of the mowers) in the US’.
Eventually, according to the International Organization for Standardization (Citation2006b) guidelines, the system boundaries have to be determined according to the functional unit and the available data. This is particularly important to ensure consistency in the comparative analysis between several product systems (Simões et al., Citation2016). The system delimitations of this study, the indicators used, and the systems being compared, are depicted in , and summarised in .
Table 1. Life cycle inventory
3.2.2. Relevant use cases for the robotic mower and scenario-based LCA/LCC
The frames of the scenarios are also set up in the ‘goal and scope definition’ of an LCA (Pesonen et al. Citation2000). Cluzel et al. (Citation2014) made a comprehensive review of scenario development and use in LCA. A scenario is defined here as ‘a description of a possible future situation relevant for specific LCA applications, based on specific assumptions about the future, and (when relevant) also including the presentation of the development from the present to the future’. On this basis, the analysis of explorative ‘what-if’ scenarios in LCA is suitable to ‘gain operational information and to compare two or more alternatives, where the researcher can set defined hypotheses on the basis of existing data’ (Pesonen et al. Citation2000). For example, scenario analysis has been applied to a prospective LCA of transport systems, to assess the potential evolution of diverse technologies (Spielmann et al. Citation2004).
Currently, the first generations of autonomous mowers are mostly utilised in the European market. Residential properties in Europe have indeed been assumed to be appropriate for autonomous vehicle implementation because their average size is much smaller than a residential property in the United States. For instance, in Spain, the average lawn size is 1,507 square feet (0.035 acre), and in the United States (US) the average lawn size is 10,871 square feet (0.25 acre) (Home Advisor Citation2019). As mowers become more capable of cutting larger areas, the market will grow in the US. With this background, two scenarios are proposed as common use-cases of the robotic mower, each with its own defined functional unit.
Scenario 1 aims to capture the average residential lawn for the untapped US market (expected to enter the US market in 2021), while scenario 2 aims to capture large commercial lawns. The scope and purpose of comparative use case #1 are actually for the automated solution to replace or supplement the traditional walk-behind or riding lawnmower for a residential garden. As opposed to scenario 2, only a single robotic mower is considered in scenario 1. In comparison, the scope of scenario 2 is for a fleet of autonomous mowers to replace or supplant several riding lawnmowers for use in a large field for commercial or professional purposes. The FUs, system boundaries, and indicators used for these comparative LCA and LCC are listed in . The specifics are then defined for each sub-scenario in the results section.
3.3. SIMULATIONS TO ESTIMATE THE MOWING TIME: RANDOM VS. OPTIMISED PATH PLANNING
With the FUs and use cases defined, it is now of the utmost importance to calculate the time required by the autonomous mower to cover these different sizes of yard (i.e., to achieve the tasks described in the FUs). A good estimate of the electricity consumption of the robotic mower is indeed crucial to properly evaluate the environmental impact allocated to the use phase.
This first generation of autonomous mower uses a random-walk navigation system, meaning the mower turns a random angle between set bounds when it hits the boundary wired, buried in the yard, and delimitating the mowing area. Random-walk path planning is a common and practical solution for such mowers but presents limitations with larger fields, creating a potential limiting factor for commercial markets, due to overlaps (i.e., zones covered more than one time) induced by this random navigation mode. Mathematical models have been formulated to approximate the time performance of a random walk versus optimised path planning. Particularly, Matlab simulations have been performed to assess the performance of the current generation of random-walk robotic, i.e. to quantify the time it takes to cover a certain percentage of the 0.25-, 0.5-, 1.32- and 4-acre fields of the use cases described in the previous sub-section.
For example, as illustrated in , given the default mowing speed of 33 cm/s and the 31 cm cutting blade of the robotic mower, simulations were first running for a 0.25-acre rectangular field with the following dimensions: 30 metres by 33.3 metres. mapped out the visual results for this 0.25-acre field random-walk simulation, following to the percentage of area covered. By these graphics, one can argue that 90% or 95% completion is not acceptable for maintaining properly a professional field, or even a residential garden depending on the owner’s requirements. While it has been estimated it requires 14 hours to cover 99% of a homogeneous rectangular yard, the original equipment manufacturer recommends using the robotic mower 20 hours to ensure sufficient coverage of more complex yard shapes.
The heat maps in highlights the overlap induced by this random walk. To reach 99% completion, it has been counted that 99.7% of the points were covered more than one time, and 81.9% of the points more than five times, which can already highlight a large room for improvement here for the autonomous solution. In the following sub-sections, potential environmental and economic savings associated with an improved autonomous mower are quantified.
The graphs in are showing the use time of the random-walk robotic mower as a function of covered area for a 0.25- and 0.5-acre field. It is observed that using random walk alone, the time required to achieve a high percentage of covered area, from 90% to almost 100% completion, increases exponentially. Note that using optimal path planning, it would take 2.5 hours a week of actual mowing time to mow a 0.25-acre field, including a first 1 h30 of mowing, 1h20 of charging time, and a final 1h of mowing time. With one battery load (charging time of 80 minutes), the autonomous mower can mow up to 90 minutes. In comparison, the human time to mow a 0.25-acre garden is, on average, around 1 hour, using a push mower. And according to an industry benchmark on private lawn care services, it takes around 2.5 hours to mow a 4-acre field using a riding mower.
3.4. LIFE CYCLE INVENTORY (LCI): SUPPORTING DATA AND ASSUMPTIONS
Life cycle inventory (LCI) aims to identify and compile inputs (e.g., materials and energy) and outputs (e.g., emissions) used to perform the environmental impact assessment (see life cycle impact assessment (LCIA) in the following sub-section). The purpose of the study here is to assess and compare the environmental and economic performances of an autonomous robotic mower with the conventional types of human-operated lawn mowers, as listed in . As such, particular attention is paid to the use phase and the production of both the fuel and electricity that are used to run respectively the gasoline- and electric-powered lawn mowers. In addition to a high level of modelling details for the use and maintenance phases, solid and complete bills of materials are used to evaluate the environmental impact attributed to upstream materials extraction and manufacturing stages.
The manufacturing impact of the machine is composed of two parts – materials impact and processing impact. For the manufacturing impact of the autonomous mowers, the actual bill of materials provided by the original equipment manufacturer (OEM) is used for the robotic mower (note that a simplified and approximated version is given in , for confidential purposes). For the human-operated pushing and riding mowers (electric- or gasoline-powered ones), generic but well-detailed bill of materials are used, combining information given by the OEM with date available in the literature (Lan and Liu Citation2010; Charif Citation2013). The materials impacts – through ReCiPe (H) Midpoints and GWP – are calculated using data from the ecoinvent database (see detailed in the next sub-section). Note that impacts from the infrastructure needed to support the manufacturing facilities of lawn mowers are beyond the scope of this study.
Table 2. Life cycle inventory of the lawn mowers being compared
As illustrated in , a well-to-wheels approach (Silva et al., Citation2006; Woo et al., Citation2017) is used to assess the impact related to the energy consumption of the different mowing systems. The production of fuel includes extraction, refining, and transportation to market activities. For electric equipment, the usage impact refers to the impact of the generation and transmission of electricity. Regarding the emissions and costs induced during the use phase, the gasoline fuel E10 ($2.6/gal), the average electricity mix, and the average electricity price ($0.12/kWh) at the scale of the United States in 2019 are considered (EPA-Environmental Protection Agency Citation2019a). It has been estimated that the gasoline-powered riding mower consumes 0.5 gallon per hour, while the push mower consumes 0.25 litre per hour of mowing. Regarding the actual electricity consumption to recharge the lithium-ion battery (Stanford Citation2010; Battery University Citation2019), the charging losses and efficiencies considered, as illustrated in , are the following: li-ion battery charge efficiency: 90%; electric motor efficiency: 60%; overall energy outlet to wheel efficiency per charging: 54%.
Under proper maintenance, the lifetime of push and ride-on is estimated to 10 years (Lan and Liu Citation2010; Charif Citation2013; Home Guides Citation2019). Manufacturers also estimate the predicted lifespan of robotic lawn mowers to 10 years (Hunker Citation2019). After discussion with a technical expert of the robotic solution, it is fair to assume that one robotic mower, with good maintenance, could perform and ensure the tasks required by the functional unit for scenario #1, while a fleet of robotic mowers would be required for larger fields (e.g., use case #2.2). Regarding the maintenance of the robotic solution, two items might need to be replaced over the lifetime of the robotic mower: (i) the lithium-ion battery, for which two rules are used to figure out when the battery should be replaced, according to the technical performance of lithium-ion batteries discussed in the literature (Stanford Citation2010; Battery University Citation2019; Popular Mechanics Citation2019a, Citation2019b): whether (1) after a 5-year usage period, or (2) after 1,000 charge cycles, if reached before the 5-year time frame; (ii) the cutting blades for which a replacement is recommended every 500 hours of mowing. Appropriate maintenance operations and part replacement for the conventional mowers over their lifetimes are also taken into consideration (Popular Mechanics Citation2019c; Today’s Mower Citation2019). The tune-ups (including filters, oils, and spare parts replacement) for the conventional mowing systems are summarised in . Prices provided by the OEM, as well as average prices found on the main retailer of gardening equipment (Home Advisor Citation2019; The Lawn Mower Guy Citation2020) have been used for the LCC.
Note that the end-of-life stage is not considered within the scope of our analysis, due to a lack of data and information regarding the fate of such equipment at their end-of-life. Also, one can argue the end-of-life stage would not be a significant criterion of differentiation for these mowing systems. Yet, for further studies, to include the impact from the disposal, recycling, or reuse of the mowers, it could be assumed that the majority of the metals parts (e.g., steel, aluminium) are recycled, while the remaining materials (e.g., plastics) are either incinerated or sent to landfill according to estimated disposal scenarios of durable goods in the United States. In the present analysis, actual impact or impact credits from potential reuse of parts or recycling of materials are not included.
3.5. LIFE CYCLE IMPACT ASSESSMENT (LCIA)
The SimaPro software (version 8.5), developed by PRé (Product Ecology Consultants), was used to model the different mowing systems and to conduct the comparative LCA. Within SimaPro 8.5, the database ecoinvent 3.4 (Citation2017), the ReCiPe Midpoint (H), and the GWP100a indicators have been used to perform the environmental evaluation (see ). Detailed descriptions of the ReCiPe metric and GWP100a metric can be found in Goedkoop et al. (Citation2008) and Solomon et al. (Citation2007), respectively. Particularity, more than ten relevant indicators – representing the impact on climate change, human health, ecosystem quality, and resources depletion – are computed, reported, and discussed hereafter. This completes the initial study performed for the use case #1.1 where the global warming potential indicator (GWP, expressed in kg of CO2 eq.) was the only indicator considered for the environmental footprint (Saidani et al. Citation2020a). For the economic side, the total cost of ownership (TCO, expressed in $) is the indicator used to compare the mowing systems. Finally, several sensitivity analyses are carried out in order to assess the influence (on the environmental and economic performance) of potential design improvements (e.g., cutting blade width, speed, battery duration) for the autonomous solution.
4. RESULTS AND INTERPRETATIONS
4.1. SCENARIO #1: RESIDENTIAL GARDEN
The overall objectives of this scenario are to (i) compare the current environmental and economic performance of one robotic with conventional lawn care solutions to mow a 0.25- or 0.5-acre residential garden, as detailed in the previous section; (ii) evaluate the environmental and economic benefits of an augmented and more intelligent robotic allowing a reduction of its usage time through, e.g., a higher speed, a larger cutting blade, an optimal path planning, or an improved battery pack.
The use case #1.1 of this study compares the robotic mower with gasoline and electric walk-behind mowers in a residential context, specifically for a 0.25-acre lawn, i.e. the average US lawn size. This use case #1.1 is further split into three situations: (i) the situation () one considers a homeowner who decides to maintain the lawn personally, and the situations two and three consider lawncare being outsourced to either (ii) an independent worker (), or (iii) a private lawn care (PLC) company (), respectively. The compared products for this use case #1.1 are following: 1 generic gasoline push (walk-behind) mower; 1 generic electric push (walk-behind) mower; 1 autonomous robotic mower, with current features; 1 autonomous robotic mower, with improved features, enabling a reduction in its use time (as illustrated through the X-axis of ); 1 autonomous robotic mower, with ideal path planning. The relevance of these use cases resulted from discussions with the OEM on the targeted market segments for the new autonomous solution.
Note that for space and clarity consideration, as the approach is very similar for each scenario and associated use cases, we are detailing in the main body of this manuscript the complete results (with illustrative graphs and figures) of the lifecycle impact assessment solely for the first scenario and its use case #1.1. The detailed results for use case #1.2 are available in Appendix A, to mainly focus on the interpretation and comparison of these different scenarios in the present manuscript.
According to the comparative LCA results mapped out in , it appears that owning an autonomous robotic mower is a less carbon-intensive alternative to gasoline push mower: 23% reduction of carbon dioxide emissions for the current autonomous version, and up to 70% for an improved robotic mower with optimal path planning. In absolute terms, the emissions of half a metric ton of carbon dioxide could be avoided thanks to the replacement of one gasoline pushing mower by one robotic mower under optimal path planning. This value represents non-negligible as it represents around 10% of the annual emissions of an average US car (EPA-Environmental Protection Agency Citation2019b).
However, looking at the total cost of ownership over the 10-year lifetime of such equipment, the actual higher buying of autonomous mower could be a hindrance to their adoption. Interestingly, according to , it could take up to eight years for the owner of the autonomous mower to intrinsically get a return of investment (using the TCO indicator) compared to the purchase and use of conventional lawn mowing systems. As such, from the OEM perspective, extending the expected useful life of their autonomous solution would be a promising line for future improvement in the next generations of robotic mowers, as further discussed in sub-section 4.3.
In the meantime, as illustrated through situations two and three (see , , ), when integrating how much one is valuing their time or the price of outsourcing their mowing, owning an autonomous mower could become a competitive alternative. Note that for situations two and three, the transportation impact attributed to the commute of an independent worker or of a private lawn care company has been included, assuming, per visit, a 3-mile travel distance using a passenger car for the second situation (), and a 6-mile travel distance using a light commercial vehicle (to carry the mowing equipment) for the third situation ().
Figure 9. a (upper left). Comparative LCA – GWP indicator – Use case #1.1 Situation 2. b (upper right). Comparative LCC – TCO indicator – Use case #1.1 Situation 2. c (lower left). Comparative LCA – GWP indicator – Use case #1.1 Situation 3. d (lower right). Comparative LCC – TCO indicator – Use case #1.1 Situation 3
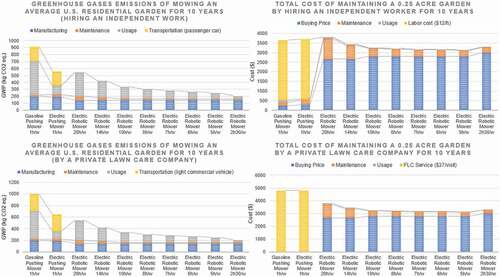
To complete this single environmental indicator analysis, further environmental analyses have been conducted following the ReCiPe (H) methodology with midpoint indicators, as illustrated in , , and . Importantly, although it is essential to be able to contribute in mitigating the greenhouse gas emissions through quantitative studies, we must ensure at the same time that there are no negative impact transfers, especially here with the impact related to the manufacturing and use of batteries for electrical solutions (Saidani et al. Citation2020a).
Figure 11. a (top). Comparative LCA – ReCiPe (H) midpoint indicators – Impacts on human health. b (middle). Comparative LCA – ReCiPe (H) midpoint indicators – Ecosystem impacts.c (bottom). Comparative LCA – ReCiPe (H) midpoint indicators – Resources depletion
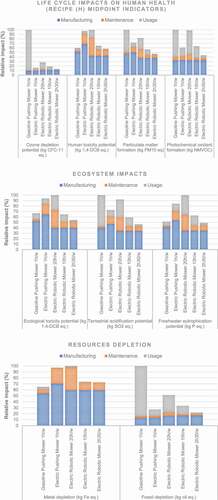
Here are the three takeaways provided by this complementary multi-indicators analysis. First, overall, the electrical solutions (conventional and autonomous), batteries and their replacements included, perform better than the generic gasoline solution. Second, except for the following indicators: human toxicity, ecotoxicity, freshwater eutrophication, and metal depletion (mainly because of (i) lithium-ion batteries and their replacements, (ii) the average US electrical mix). But in absolute terms, this impact surplus is not huge or outrageous compared to the fairly large benefits on global warming potential, ozone depletion, and fossil depletion indicators. Third, the positive and promising point for the autonomous solution is that its optimised version (i.e., optimal path planning) version is better than conventional electric and gasoline solutions on all indicators, except one (metal depletion).
The use case #1.2 of this study differs from the previous one by comparing the robotic mower with a gasoline riding mower and an electric riding mower, to mow a larger residential garden. The three situations for this scenario remain the same as the ones for use case #1.1: situation one considers a homeowner who decides to personally maintain the lawn, and situation two and three consider lawncare being outsourced to an independent worker or a private lawn care company. The detailed results for this use case #1.2 are available in Appendix A (see Figure A1, Figure A2, Figure A3, and Figure A4).
In all, the main interpretations of these comparative LCA and LCC results for scenario #1 is that: (i) the robotic mower appears to be a non-negligible greener alternative to both gasoline push/riding mower; (ii) the environmental savings could be significantly higher by improving the autonomous mower efficiency; and, (iii) when integrating hidden cost (labour cost and/or time saved for the user) the robotic mower could be an even cheaper alternative than human-operated mowing service.
4.2. SCENARIO #2: PROFESSIONAL MOWING ACTIVITY
The use case #2.1 of this study compares the performance of the robotic mower with a gasoline ride-on mower, in a commercial use context, to mow a 1.32-acres football field. The required mowing time for the autonomous mower under random-walk path planning is still determined by the Matlab simulation model (described in sub-section 3.3). Note that through complementary sensitivity analyses conducted in the next sub-section, this time value would change according to the change of speed and blade width. After an initial calculation, it has been discovered that it would take for the robotic mower under random path planning 139 hours per week to cover a 1.32 acres field, leaving 29 hours of spare time for the field to be used (by the football players, for training and match purposes).
Thus, this use case compares a total of four different systems, namely: one robotic mower under random path planning (when the field is used less than 29 hours a week), two robotic mowers under random path planning (to enable more than 29 hours of spare time for the field to be used), one robotic mower under ideal path planning, and one gasoline riding mower ideal path planning. As illustrated through , the robotic mower with ideal path planning performs the best from a carbon emission standpoint, while the gasoline riding mower appears to be the least environmentally friendly solution for such usage. Note that by improving the path planning of the autonomous mowing system, the carbon footprint of the robotic mower can be reduced by more than 75% in this situation.
Comparing the LCC results of the different systems (see ), the robotic mower under ideal path planning still performs the best from the economic perspective. Yet, in this situation, owning the current version of the autonomous mower (under random-walk path planning) does not appear to be a cost-effective solution compared to the conventional gasoline riding mower. In fact, due to the lack of efficiency of the robotic, the maintenance cost of the robotic under random path planning is significantly higher than that of the ideal path planning. Promising areas of improvement for the autonomous solution include, as further quantified in sub-section 4.3, increasing the cutting width of the blades and the lifespan of the battery, while increasing the efficiency of the path planning algorithm.
The use case #2.2 of this study compares the performance of the robotic mower with conventional mowing solutions to maintain a 4-acre schoolyard, namely: one generic gasoline riding mower, one generic electric riding mower used by the owner or dedicated employee of the school, and by hiring a private lawn care company bringing its gasoline riding mower. As mentioned in , note that a fleet of four robotic mowers, operating at a random-walk path planning, are required the cover the full schoolyard on a weekly basis.
Comparing the LCA results for the different mowing systems (see ), the electric solutions – whether the electric riding mower, the robotic mower with optimal path planning, or the fleet of the current generation of four robotic mowers – outperform the gasoline solution in terms of carbon dioxide mitigation. From an economic perspective for the school owner (see ), the robotic mower under ideal path planning is the most cost-effective solution, followed by the gasoline riding mower, the electric riding mower, and the fleet of four robotic mowers with random-walk path planning.
After comparing the autonomous mowing solution with human-operated counterparts though these two use cases, it is clear that a robotic with ideal path planning is the most attractive option from an environmental and economic standpoint (under the assumption that adding such a feature would not increase its cost or overall ecological footprint), while the current version of the robotic mower has still a large potential of improvement. Particularly, the present state of a robotic fleet would be an inferior option to the existing ride-on mowers and therefore needs to improve on its efficiency by employing better path planning algorithm, and potentially having a larger blade width and an enhanced powertrain, to cover a larger area and decreasing its use time.
4.3. SENSITIVITY ANALYSIS: IMPACT OF DESIGN IMPROVEMENTS ON THE SUSTAINABILITY PERFORMANCE
Based on the results reported in the previous sub-sections, sensitivity analyses are carried out to identify more specifically the most promising improvement directions for the next generation(s) of robotic mowers. For each case, new calculations are done with an improvement of a single parameter (namely, battery lifespan, blade lifespan, blade width, speed) by 5% and 10%. The basic assumption for this analysis is that the bill of material remains unchanged for the autonomous solution. It is indeed considered here that a 10% improvement on the four features or parameters aforementioned would not impact the manufacturing impacts significantly. Note also that the conventional human-operated mowing systems remain unchanged as a comparative baseline.
This sub-section particularly exposes the results of the sensitivity analysis for the case #2.1 (i.e., mowing a 1.32-acre football field) in , which is the intermediate use case in terms of acreage covered by the robotic mower, but note that similar trends have been found running sensitivity analyses for the other use cases. Out of the four design parameters tested, improvements of the blade width and the speed provide the most significant environmental and economic benefits, followed by an enhanced battery lifetime. Augmenting the time-efficiency of the current generation of robotic mower is actually key to substantially reduce the energy consumption and associated CO2 emissions (depending on the energy mix).
Combining this sensitivity analysis with the previous findings, the main directions of improvement for the robotic mower to be more competitive, in front of conventional mowing systems, are the following (by order of importance): enabling an optimal path planning (or at least, reducing the number of overlaps), wider cutting blades, higher speed, and more durable and/or performant lithium-ion batteries. Promising and concrete lines of future research to augment the sustainability of the autonomous robotic mowing solutions are further discussed in the next section.
5. Conclusion and perspectives
Comparing the sustainability performance of autonomous mowing equipment with their human-operated counterparts is not straightforward. While having a similar overall function, it has been found that there are non-negligible differences – in terms of features (e.g., random vs. optimal path planning), constraints (e.g., boundary wire, battery duration) or freedom of operation (e.g., a possible non-stop 24-hour time window for the autonomous system). Having to consider these specificities when defining the functional unit and system boundaries is a key challenge for a sound comparative environmental and economic assessment. While few studies have been assessing the environmental and economic performance of autonomous systems, except in the automotive industry, this piece of research brings a new contribution in that regard for the gardening (residential or commercial) sector. Particularly, through LCA and LCC, environmental and economic trade-offs between the autonomous and conventional mowing solutions have been highlighted, as summarised in , according to relevant usage scenarios, from mowing his own garden to maintaining larger fields like a football stadium or a schoolyard. This study also sheds interesting lights on potential design improvements for the next generations of autonomous lawn mowing systems. In fact, sensitivity analyses – on key parameters influencing the performance of the autonomous mower – have been conducted to evaluate the environmental and economic benefits of an augmented robotic, allowing a reduction of use time, through appropriate incremental or radical design improvement.
Table 3. Ranking of solutions for each use case from an economic and environmental perspective
In addition to the contribution to the scientific literature on the quantification of the sustainability performance of autonomous systems in comparison with traditional ones in the gardening sector, the findings reported here could support decision-makers in the industry with tangible elements of improvement (e.g., for original equipment manufacturers of autonomous mowers), as well as policy-makers (e.g., to foster the adoption of greener mowing solutions. It is indeed the role of life cycle engineering researchers and designers to help quantify and inform on the real impact of these new and advanced autonomous systems, in order to figure out how to exploit the full potential of such systems in terms of sustainability performance.
On the one hand, to be a cost-effective alternative in the long run, as well as to be a competitive solution for larger fields, the following areas of improvement for the next generation of autonomous mowers are essential. First, the main recommendation from our analysis is to improve the path planning of robotic. Indeed, in its current version, it has no topological understanding of the yard that it is operating in; thus, it has no path-planning capabilities. This lack of spatial awareness is actually a major design shortcoming and should be a focus for improvement in the next generation of the autonomous mower. Some OEMs are already working in this direction and are trying to implement optimal path-planning capabilities, using high-precision navigation systems, allowing an accuracy of 2 to 3 centimetres to track and control the mower. In the meantime, a short-term solution could be to do so by adding in the possibility for the user to self-program a path flowing the topology of the field to mow. Taking inspirations from current path planning for autonomous cleaners could also be relevant to study the time-efficiency of several navigation systems to cover a field, including e.g., a spiral walk, wall following (boundary wire following here, in the case of lawn mowing systems), or S-shape path planning, combined with a smartphone pairing function (Liu, Lin, and Zhu Citation2008; Hasan and Reza Citation2014). Second, the width of the cutting blades, the speed, and the battery performance of the robotic mower are other key directions of improvement to augment its sustainability.
On the other hand, while the market is currently dominated by gasoline-powered mowing systems (Banks and McConnell Citation2015; Technavio Citation2017), the present findings showed that the electric solutions – either the robotic mower or the electric push/ride-on mowers – are more environmentally friendly than the gasoline push and ride-on mowers. Yet, the initial buying prices for these electric alternatives are higher than the well-established gasoline mowers. As such, such a study can help policy-makers quantify and set up an appropriate financial incentive (Miller Citation2018) to support the adoption of the electric mowing systems (of course, where it makes sense environmentally according to the energy mix used in a given country or region). Notably, the willingness to pay for the autonomous solution could also be further investigated, as owners might not take into consideration the total cost of ownership over a 10-year period when buying products. Importantly, the price evolution of the robotic, in case of mass production, increased market share, or improved features should be further discussed as well. Also, one has to bear in mind that the ongoing research and development to increase the performance of lithium-ion batteries (Sangwan and Jindal Citation2013; International Energy Agency Citation2017; Rigamonti et al. Citation2017; Akram and Abdul-Kader Citation2021), as well as the shift from fossil-based energy to renewable energy (Lu et al. Citation2010; Caldeira and Brown Citation2019; UNEP-United Nations Environment Programme Citation2019; Visual Capitalist Citation2019), are factors that would influence positively the environmental footprint of electricity-powered solutions.
Eventually, one limitation of the present study is that the end-of-life fate and impact of the different mowers are not considered in the comparative life cycle assessment, as explained in sub-section 3.4. When more reliable data would be available, e.g., related to the end-of-life pathways of robotic mowers or potential recycling options for lithium-ion batteries, it would be interesting to update this study and analyse how it impacts the overall life cycle performance. Meanwhile, designing the new generation of robotic mower in a circular economy perspective (including, e.g., design for easy-disassembly, business models facilitating take-back scheme and remanufacturing) appears as a promising and timely line for future research (Saidani et al. Citation2019b, Citation2020b). In this case, the system boundaries of the LCA and LCC would have to be extended to two or more successive purchases from the same product line of mowers. Notably, it might be relevant to consider possible product-as-a-service scenarios (Kjaer et al., Citation2019) for the robotic mower, in order to facilitate the traceability, optimised maintenance operations during the use phase, and recovery at its end-of-life for the manufacturer. In this case, it could be particularly interesting to evaluate and discuss the economic profitability of such product-service systems for the producers, as well as the environmental implications of such a shift of business model. Last but not least, further works are encouraged to scale up comparative LCA and LCC to wider and more complex systems (e.g., autonomous tractors working in fleet and collaboration with the farmer (Walter et al. Citation2017) at a scale of a whole crop product system). More industrial case studies, integrating as well social indicators, would be essential to ensure that the shift towards more autonomous systems is truly sustainable not only from a profitability point of view, but also for the planet and the people.
Acknowledgments
This material is partially based upon the work supported by Deere and Company. Any opinions, findings, and conclusions or recommendations expressed in this publication are those of the authors and do not necessarily reflect the views of the sponsor.
Disclosure statement
No potential conflict of interest was reported by the author(s).
Additional information
Funding
Notes on contributors
Michael Saidani
Michael Saidani is currently a postdoctoral researcher at the University of Illinois at Urbana-Champaign, within the Industrial and Enterprise Systems Engineering Department, under the supervision of Prof. Harrison Kim. He received his PhD in Industrial Engineering from CentraleSupélec, at the Paris-Saclay University, under the supervision of Prof. Bernard Yannou. His research work aims to advance the sustainable and circular design, usage, and end-of-life of complex product systems.
Zhonghao Pan is a graduate student in Industrial System Engineering at the University of Illinois at Urbana-Champaign. He is conducting applied research in sustainable engineering and computer science within the Department of Industrial and Enterprise Systems Engineering under the supervision of Prof. Harrison Kim.
Harrison Kim is an award-winning educator, researcher, and designer in complex systems design and sustainability – engineering design, manufacturing, analytics, and systems optimisation. He is a Professor in the Department of Industrial and Enterprise Systems Engineering at the University of Illinois at Urbana-Champaign and the director of the Enterprise Systems Optimization Laboratory. He is also affiliated with the Computational Science and Engineering Program and the Beckman Institute at the University of Illinois.
Jason Wattonville is the enterprise manager within the Product Sustainability Department at John Deere. He received a BS in Agricultural Engineering from Iowa State University. He has more than 20 years’ work experience at Deere and Company, leading projects in design engineering, business development, innovative process for cotton harvesting, and now in sustainable agricultural practices.
Andrew Greenlee is a senior staff engineer with the Product Sustainability Department at the John Deere Moline Technology & Innovation Center. He receives a BS in Agricultural Engineering from the University of Illinois at Urbana-Champaign, and a MS in Mechanical Engineering from Iowa State University. He has more than 15 years’ work experience at Deere and Company.
Troy Shannon is a senior engineer with the Product Sustainability Department at the John Deere Moline Technology & Innovation Center. He receives a BS from the University of Wisconsin.
Bernard Yannou is a professor of Design Engineering and director of the Industrial Engineering Research Department at CentraleSupélec. His research interests are design automation, new product development, value and user centred design, artificial intelligence and computers in design, system thinking and engineering, design under uncertainty, innovation engineering, sustainable design, industrial ecology, and multidisciplinary design optimisation.
Yann Leroy is an assistant professor in Design Engineering and Sustainability at CentraleSupélec, Université Paris-Saclay. His areas of expertise include eco-design of products, lifecycle assessment, and circular economy. He is an active member of the Sustainable Design Special Interest Group of the Design Society.
François Cluzel is an assistant professor at the Industrial Engineering Research Department at CentraleSupélec, member of Université Paris-Saclay. He holds a PhD in Industrial Engineering from Ecole Centrale Paris and Engineering degree in Mechanics from Supméca Paris.
References
- Akram, M. N., and W. Abdul-Kader. 2021. “Electric Vehicle Battery State Changes and Reverse Logistics Considerations.” International Journal of Sustainable Engineering 1–14. press. doi:https://doi.org/10.1080/19397038.2020.1856968.
- Bahri, N., and W. Ouled Amor. 2019. “Intelligent Power Supply Management of an Autonomous Hybrid Energy Generator.” International Journal of Sustainable Engineering 12 (5): 312–332. doi:https://doi.org/10.1080/19397038.2019.1581852.
- Banks, J. L., and R. McConnell. 2015. “US National Emissions from Lawn and Garden Equipment”. International Emissions Inventory Conference, April 2015, San Diego, USA.
- Battery University. 2019. “Comparing the Battery with Other Power Sources”. www.batteryuniversity.com/learn/article/comparing_the_battery_with_other_power_sources Accessed May 1, 2020.
- Caffrey, K. R., and M. W. Veal. 2013. “Conducting an Agricultural Life Cycle Assessment: Challenges and Perspectives.” The Scientific World Journal, Article ID 472431 2013: 13. doi:https://doi.org/10.1155/2013/472431.
- Caldeira, K., and P. T. Brown. 2019. “Reduced Emissions through Climate Damage to the Economy.” Proceedings of the National Academy of Sciences 116 (3): 714–716. doi:https://doi.org/10.1073/pnas.1819605116.
- Charif, M. 2013. “Comparative Life Cycle Assessment of Biodiesel- and Battery-Powered Ride-on Mowers”. University of British Columbia, Report, A SEEDS Project, December 2013.
- Cluzel, F., B. Yannou, D. Millet, and Y. Leroy. 2014. “Exploitation Scenarios in Industrial System LCA.” The International Journal of Life Cycle Assessment 19 (1): 231–245. doi:https://doi.org/10.1007/s11367-013-0631-z.
- Cluzel, F., Y. Leroy, and B. Yannou. 2013. “Toward a Structured Functional Unit Definition Framework to Limit LCA Results Variability”. 6th International Conference on Life Cycle Management; 2013; Paper no. 484; Gothenburg, Sweden.
- Collado-Ruiz, D., and H. Ostad-Ahmad-Ghorabi. 2010. “Fuon Theory: Standardizing Functional Units for Product Design. Resources.” Resources, Conservation and Recycling 54 (10): 683–691. doi:https://doi.org/10.1016/j.resconrec.2009.11.009.
- Cooper, J. 2003. 2003. “Specifying Functional Units and Reference Flows for Comparable Alternatives.” Int J Life Cycle Assess, 8 (6): 337–349.
- De Melo, C. M., S. Marsella, and J. Gratch. 2019. “Human Cooperation When Acting through Autonomous Machines.” Proceedings of the National Academy of Sciences 116 (9): 3482–3487. doi:https://doi.org/10.1073/pnas.1817656116.
- Dusik, J., R. Therivel, B. Sadler, T. Fischer, and I. Saric. 2019. “Strategic Environmental and Social Assessment of Automation, Scoping Working Paper”. https://www.iisd.org/blog/automation-environment Accessed May 1, 2020.
- EC-European Commission, International Reference Life Cycle Data System. 2010. Handbook First. European Commission, Joint Research Centre, Institute for Environment and Sustainability, Springer, Berlin.
- Ecoinvent 3.4. 2017. Ecoinvent Centre. www.ecoinvent.org/database/database.html Accessed May 1, 2020. Accessed 1, 2020. Accessed May 1 May 1, 2020. Accessed May 1, 2020.
- EPA-Environmental Protection Agency. 2019a. “Energy Power Profiler”. www.epa.gov/energy/power-profiler Accessed May 1, 2020.
- EPA-Environmental Protection Agency. 2019b. “Emissions from Passenger Vehicle in the U.S”. 2019. Retrieved from: www.epa.gov/greenvehicles/greenhouse-gas-emissions-typical-passenger-vehicle
- Gaines, L. L., and J. B. Dunn. 2014. Lithium-Ion Battery Environmental Impacts, Editor(s): Gianfranco Pistoia, Lithium-Ion Batteries, 483–508. Amsterdam: Elsevier.
- Gawron, J. H., G. A. Keoleian, R. D. De Kleine, T. J. Wallington, and H. Kim. 2018. “Life Cycle Assessment of Connected and Automated Vehicles: Sensing and Computing Subsystem and Vehicle Level Effects.” Environmental Science & Technology 52 (5): 3249–3256. doi:https://doi.org/10.1021/acs.est.7b04576.
- Goedkoop, M. J., R. Heijungs, M. Huijbregts, A. De Schryver, J. V. Z. R. Struijs, and R. Van Zelm. 2008.. A Life Cycle Impact Assessment Method Which Comprises Harmonised Category Indicators at the Midpoint and the Endpoint Level. The Hague, Ministry of VROM. Report
- Guinee, J. B., R. Heijungs, G. Huppes, A. Zamagni, P. Masoni, R. Buonamici, T. Ekvall et al. 2011. “Life Cycle Assessment: Past, Present, and Future.” †. Environmental Science & Technology 45 (1): 90–96. DOI:https://doi.org/10.1021/es101316v.
- Hasan, K. M., and K. J. Reza. 2014. “Path Planning Algorithm Development for Autonomous Vacuum Cleaner Robots”. In 2014 International Conference on Informatics, Electronics & Vision (ICIEV), Dhaka, Bangladesh, 1–6, IEEE.
- Home Advisor. 2019. www.homeadvisor.com/cost/lawn-and-garden/maintain-a-lawn Accessed May 1, 2020.
- Home Guides. 2019. www.homeguides.sfgate.com/long-should-lawnmower-last-88324.html Accessed 1 May 2020.
- Hunker. 2019. www.hunker.com/13405219/life-expectancy-of-a-lawn-mower Accessed 1 May 2020.
- International Energy Agency. 2017. Energy Technology Perspectives. Paris: International Energy Agency.
- International Organization for Standardization. 2006a. “ISO 14040: 2006 Environmental Management - Life Cycle Assessment - Principles and Framework”. In: ISO 14000 International Standards Compendium. Genève, Switzerland.
- International Organization for Standardization. 2006b. “ISO 14044:2006 Environmental Management - Life Cycle Assessment - Requirements and Guidelines”. In: ISO 14000 International Standards Compendium. Genève, Switzerland.
- Kjaer, L. L., D. C. A Pigosso, M. Niero, N. M. Bech, and T. C. McAloone. 2019. “Product/service‐systems for a circular economy: The route to decoupling economic growth from resource consumption?.” Journal of Industrial Ecology 23 (1): 22–35.
- Kopelias, P., E. Demiridi, K. Vogiatzis, A. Skabardonis, and V. Zafiropoulou. 2020. “Connected & Autonomous vehicles – Environmental Impacts – A Review.” Science of the Total Environment 712: 135237. doi:https://doi.org/10.1016/j.scitotenv.2019.135237.
- Kurilova-Palisaitiene, J., E. Permin, T. Mannheim, K. Buhse, M. Lorenz, R. Schmitt, B. Corves et al. 2017. “Industrial Energy Efficiency Potentials: An Assessment of Three Different Robot Concepts.” International Journal of Sustainable Engineering 10 (3): 185–196. DOI:https://doi.org/10.1080/19397038.2017.1284280.
- Kwak, M., and H. Kim. 2013. “Economic and Environmental Impacts of Product Service Lifetime: A Life-cycle Perspective.” In Product-Service Integration for Sustainable Solutions, edited by Meier H., 177–189. Berlin, Heidelberg: Springer.
- Lan, X., and Y. Liu. 2010. “Life Cycle Assessment of Lawnmowers - Two Mowers’ Case Studies”. Master’s Thesis in Environmental Measurements and Assessments. Department of Energy and Environment, Chalmers University of Technology, Göteborg, Sweden.
- Liu, Y., X. Lin, and S. Zhu. 2008. “Combined Coverage Path Planning for Autonomous Cleaning Robots in Unstructured Environments”. 2008 7th World Congress on Intelligent Control and Automation, Chongqing, China, 8271–8276.
- Lu, S., N. B. Schroeder, H. M. Kim, and U. V. Shanbhag. 2010. “Hybrid Power/energy Generation through Multidisciplinary and Multilevel Design Optimization with Complementarity Constraints.” Journal of Mechanical Design 132 (10): 10. doi:https://doi.org/10.1115/1.4002292.
- Miller, M. D. 2018. “Rethinking Electric Vehicle Incentives.” Colo. Nat. Resources, Energy & Envtl. L. Rev 29: 2.
- Nouzil, I. A. Raza, and S. Pervaiz. 2017. “Social Aspects of Automation: Some Critical Insights.” IOP Conf. Ser.: Mater. Sci. Eng, 244 (1): 012020. IOP Publishing.
- Pesonen, H. L., T. Ekvall, G. Fleischer, G. Huppes, C. Jahn, Z. S. Klos, G. Rebitzer et al. 2000. “Framework for Scenario Development in LCA.” The International Journal of Life Cycle Assessment 5 (1): 21. DOI:https://doi.org/10.1007/BF02978555.
- Popular Mechanics. 2019a. www.popularmechanics.com/technology/gadgets/how-to/a7432/why-your-gadgets-batteries-degrade-over-time-6705747 Accessed 1 May 2020
- Popular Mechanics. 2019b. www.popularmechanics.com/technology/gadgets/a15731/best-way-to-keep-li-ion-batteries-charged Accessed 1 May 2020
- Popular Mechanics. 2019c. www.popularmechanics.com/home/lawn-garden/how-to/a6701/is-it-time-to-tune-up-your-mower Accessed 1 May 2020
- Productivity Inc. 2019. “Benefits of Automation”. https://www.productivity.com/benefits-of-automation Accessed May 1, 2020.
- Rigamonti, L., A. Falbo, L. Zampori, and S. Sala. 2017. “Supporting a Transition Towards Sustainable Circular Economy: Sensitivity Analysis for the Interpretation of LCA for the Recovery of Electric and Electronic Waste.” The International Journal of Life Cycle Assessment 22 (8): 1278–1287. DOI:https://doi.org/10.1007/s11367-016-1231-5.
- SAE International. 2016. “Taxonomy and Definitions for Terms Related to On-Road Motor Vehicle Automated Driving Systems”. https://www.sae.org/standards/content/j3016_201401/ Accessed May 1, 2020.
- Saidani, M., B. Yannou, Y. Leroy, and F. Cluzel. 2020b. “Dismantling, Remanufacturing and Recovering Heavy Vehicles in a Circular economy—Technico-economic and Organisational Lessons Learnt from an Industrial Pilot Study.” Resources, Conservation and Recycling 156: 104684. doi:https://doi.org/10.1016/j.resconrec.2020.104684.
- Saidani, M., B. Yannou, Y. Leroy, F. Cluzel, and A. Kendall. 2019b. “A Taxonomy of Circular Economy Indicators.” Journal of Cleaner Production 207: 542–559. doi:https://doi.org/10.1016/j.jclepro.2018.10.014.
- Saidani, M., E. Pan, H. Kim, A. Greenlee, J. Wattonville, B. Yannou, Y. Leroy, et al. 2020a. “Assessing the Environmental and Economic Sustainability of Autonomous Systems: A Case Study in the Agricultural Industry”. Procedia CIRP 90: 209–214. https://doi.org/10.1016/j.procir.2020.02.123.
- Saidani, M., H. Kim, B. Yannou, Y. Leroy, and F. Cluzel. 2019a. “Framing Product Circularity Performance for Optimized Green Profit”. In ASME 2019 International Design Engineering Technical Conferences and Computers and Information in Engineering Conference. Anaheim, California, USA: American Society of Mechanical Engineers Digital Collection.
- Sangwan, K. S., and A. Jindal. 2013. “An Integrated Fuzzy Multi-criteria Evaluation of Lithium-ion Battery Recycling Processes.” International Journal of Sustainable Engineering 6 (4): 359–371. doi:https://doi.org/10.1080/19397038.2012.735717.
- Silva, C. M., G. A. Gonçalves, T. L. Farias, and J. M. C. Mendes-Lopes. 2006. “A tank-to-wheel analysis tool for energy and emissions studies in road vehicles.” Science of the Total Environment 367(1): 441–447.
- Simões, C. L., R. Figueirêdo De Sá, C. J. Ribeiro, P. Bernardo, A. J. Pontes, and C. A. Bernardo. 2016. “Environmental and economic performance of a car component: Assessing new materials, processes and designs”. Journal of Cleaner Production 118: 105–117.
- Solomon, S.M. Manning, M. Marquis, and D. Qin. 2007. “Climate Change 2007: The Physical Science Basis. Contribution of Working Group I to the Fourth Assessment Report of the Intergovernmental Panel on Climate Change”. Cambridge University Press, Cambridge.
- Spielmann, M., R. Scholz, O. Tietje, and P. De Haan. 2004. “Scenario Modeling in Prospective LCA of Transport Systems.” Application of Formative Scenario Analysis. Int J Life Cycle Assess 10: 325–335.
- Stanford. 2010. “Batteries Efficiencies”. www.large.stanford.edu/courses/2010/ph240/sun1 Accessed May 1, 2020.
- Sullivan, J. L., and L. Gaines. 2012. “Status of Life Cycle Inventories for Batteries.” Energy Conversion and Management 58: 134–148. doi:https://doi.org/10.1016/j.enconman.2012.01.001.
- Technavio. 2017. “Electric Lawn Mowers Market to Register the Highest Growth through 2021”. Report.
- The Lawn Mower Guy. 2020. “Prices and Services”. www.thelawnmowerguy.net/Prices-and-services.html Accessed May 1, 2020.
- Today’s Mower. 2019. “Electric Riding Mower Costs”. www.todaysmower.com/is-owning-a-ryobi-rm480e-electric-riding-mower-cost-effective Accessed May 1, 2020
- UNEP-United Nations Environment Programme. 2019. “Emissions Gap Report 2019”. Executive summary. UNEP, Nairobi.
- Visual Capitalist. 2019. “Global Transition to Green Energy”. www.visualcapitalist.com/global-transition-to-green-energy Accessed May 1, 2020.
- Walter, A., R. Finger, R. Huber, and N. Buchmann. 2017. “Smart Farming Is Key to Developing Sustainable Agriculture.” Proc. Natl. Acad. Sci. USA 114: 6148–6150.
- Woo, J. R., H. Choi, and J Ahn. 2017. “Well-to-wheel analysis of greenhouse gas emissions for electric vehicles based on electricity generation mix: A global perspective”. Transportation Research Part D: Transport and Environment51: 340–350.
Appendix A.
Details of the results for the use case #1.2
Figure A3. a. (upper left). Comparative LCA – GWP indicator – Use case #1.2 Situation 2. b. (upper right). Comparative LCC – TCO indicator – Use case #1.2 Situation 2. c. (lower left). Comparative LCA – GWP indicator – Use case #1.2 Situation 3. d. (lower right). Comparative LCC – TCO indicator – Use case #1.2 Situation 3
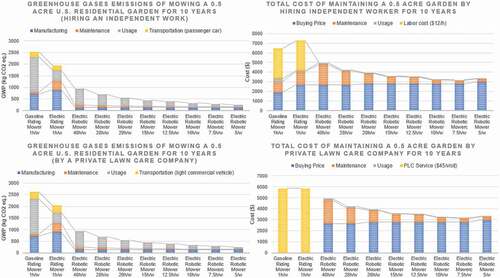