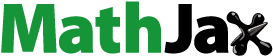
ABSTRACT
Remanufacturing of end-of-life products is one of the most preferred disposition alternatives to address environmental and economic concerns. In this paper, a hybrid decision-making framework is proposed to address the strategic planning issues of manufacturing-remanufacturing closed loop systems. The framework consists of qualitative and quantitative parts. The AHP-based qualitative part can be used to determine the configuration of the closed-loop network. The framework takes into consideration the product complexity and other characteristics as well as the supply chain network structure. The model considers the concerns of a manufacturer who offers a variety of complex modular products. The second part of the framework provides a quantitative optimisation model of technology implementation. A case study from the washing machine industry sector is used to illustrate the application of the proposed decision-making framework.
1. Introduction
Product recovery and its reverse logistics activities have evolved over the years as the global economic, environmental and legal circumstances developed. Initially, reverse logistics activities to recover manufactured products started as a waste reduction effort. In 1994, recycling of paper and glass in Europe was estimated to generate 27.7 million tonnes and 7 million tonnes respectively (Fleischmann et al. Citation1997). The accumulation of electronic and electrical waste in landfills prompted a wider interest in reverse logistics. The growing concerns about limited raw materials resources, hazardous materials in used products and other environmental concerns resulted in legal pressure on manufacturers to take-back their products. Manufacturers were also motivated to implement reverse logistics initiatives to reap some economic benefits. Moreover, globalisation and shorter product lifecycles forced manufacturing firms to consider reverse logistics in their business strategies to be more competitive and profitable.
Global manufacturers’ efforts to maximise recovered value from used products has led to the emergence of smart remanufacturing and demanufacturing systems with high levels of automation, flexibility and adaptability (Tolio et al. Citation2017). These smart systems include automated disassembly, active disassembly, robotic sorting, embedded sensors, flexible and reconfigurable automation (Tolio et al. Citation2017). The application of Industry 4.0 principles in the remanufacturing segment is still in the early stage compared to their application in the manufacturing segment (Butzer et al. Citation2016). Challenges that manufacturers have to tackle, and the technologies that can be used to enable efficient remanufacturing were discussed in several recent papers (Butzer et al. Citation2016; Yang et al. Citation2018; Kurilova-Palisaitiene, Sundin, and Poksinska Citation2018).
The extent of technology deployed to perform the necessary activities throughout the product cradle-to-cradle lifecycle needs to be determined considering the environmental, economic and legislative constraints. A higher level of technology would lead to higher infrastructure cost; however, it would also lead to cost and time saving in the respective operational areas such as sorting, disassembly, and remanufacturing. For this reason, a carefully designed closed loop system is necessary to achieve an optimum trade-off between cost and long-term performance. The decision-making process should also consider the nature of manufacturer relationships with other stakeholders such as suppliers, retailers, distribution dealers and customers.
In order to maximise the value recovered from used products, efficient product recovery systems have to be in place. Product recovery can be achieved through reverse logistics. Reverse logistics can be investigated separately or combined with forward materials flow (Fleischmann et al. Citation1997). One of the main advantages of well-organised and efficient product recovery systems is the mitigation of risks associated with uncertainties in returned products’ quantity, quality and timing of return. Time delays can be caused by extended storage of used products by customers or processing delays of returned products in the collection stage or subsequent stages in the backward flow of products (i.e. reverse logistics).
The performance of product recovery systems depends on many criteria such as product design, materials, manufacturing processes, customer behaviour, and supply chain network. Because of the complexity involved in considering all these criteria and their subcriteria, a flexible and customisable decision-making approach is needed to address the concerns of different industries. Effective decisions can be made by considering the industry sector in general and specific manufacturer’s circumstances. Efficient reverse logistics systems play an important role in the product recovery process.
The characteristics of the product and its associated manufacturing systems and supply chain structure can put some industries ahead of others in terms of the speed and extent of remanufacturing. For example, an overview of reverse logistics strategies showed that manufacturer’s facilitated remanufacturing, refurbishing and recycling were common in automobile, copy machine and printer toner industries (Kumar and Putnam Citation2008). Design for Remanufacturing (DfR) was studied by a number of researchers (Yang et al. Citation2017; Chakraborty, Mondal, and Mukherjee Citation2017). However, the actual implementation of product remanufacturing depends on the overall product design, supply chains, manufacturing and product recovery systems. For this reason, a decision-making framework that takes into consideration the product and manufacturing characteristics as well as the supply and distribution networks is needed.
In this paper, the closed loop supply chain is investigated from the perspective of a manufacturer intending to integrate the product manufacturing and remanufacturing activities using new technologies to increase efficiency and eliminate waste. The literature provides a limited coverage for closed loop systems where product variety and complexity need to be considered. In the present study, a hybrid decision-making framework that consist of an AHP-based decision-making model and a mathematical optimisation model is proposed to enhance and optimise the overall performance of the closed loop system. The proposed decision-making framework aims at assisting manufacturers determine the optimum selection of advanced technologies to maximise the profit of the manufacturing-remanufacturing system.
2. Literature review
Manufacturing-remanufacturing closed loop systems are extensions of reverse logistics (RL) and closed-loop supply chain systems (CLSC) that aim at increasing their contributions towards circular economy through more manufacturer involvement and implementation of advanced manufacturing systems. Rogers and Tibben-Lembke (Citation2001)defined reverse logistics as ‘the process of planning, implementing, and controlling the efficient, cost effective flow of raw materials, in-process inventory, finished goods and related information from the point of consumption to the point of origin for the purpose of recapturing value or proper disposal’. This definition is considered to be the most widely accepted definition of reverse logistics (Agrawal, Singh, and Murtaza Citation2015). Remanufacturing is the best form of value recovery; however, lack of technologies and closed-loop planning resulted in high costs and reduced interests in remanufacturing.
To improve their efficiency, reverse logistics networks can be studied as separate networks of reverse flow or as integrated forward and reverse distribution networks (Fleischmann et al. Citation1997). The integration of forward and reverse logistics results in closed-loop supply chains (CLSC) management which can be defined as ‘the design, control and operation of a system to maximize value creation over the entire life cycle of a product with dynamic recovery of value from different types and volumes of returns over time’ (Guide and Van Wassenhove Citation2009). In contrast to reverse logistics, closed loop supply chains (CLSC) represent an evolution in the efforts of maximising value recovery from used products by including the circular movement of products in the initial design of the supply chain structure to reduce the risks caused by uncertainties in returned product volume and quality. Therefore, the design of closed loop supply chains (CLSC) is an important element in the transition from a linear economy to circular economy. Decision-making for RL and CLSC networks requires both quantitative and qualitative analysis.
A review of the recent literature in the application of multi-criteria decision making (MCDM) and other methods to study reverse logistics (RL), closed loop supply chains (CLSC) and remanufacturing systems is presented to examine the extent of coverage and identify potential areas of contribution to manufacturing-remanufacturing closed loop systems. Prakash and Barua (Citation2015) developed a multi-criteria decision making (MCDM) method using an integrated analytic hierarchy process (AHP) and the technique for order performance by similarity to ideal solution (TOPSIS) approach. The method was used to rank solutions to overcome barriers to reverse logistics adoption in electronics industry. Other studies have also focused on reverse logistics using hybrid MCDM methods. A fuzzy AHP-TOPSIS method was used by Sirisawat and Kiatcharoenpol (Citation2018) to rank solutions to reverse logistics barriers in the electronics industry. Remanufacturing was not included in the study as a disposition alterative for the recovered products. A hybrid MCDM method was also developed for risk analysis and prioritisation in reverse logistics (Senthil, Murugananthan, and Ramesh Citation2018). The approach used in the study comprises of AHP, TOPSIS and the Preference Ranking Organization Method for Enrichment Evaluation (PROMETHEE) methods. A case of plastics recycling was used to illustrate the hybrid method. A hybrid fuzzy ANP and optimisation approach was proposed by Tosarkani and Amin (Citation2018) to study reverse logistics in the electronics industry. The hybrid approach was used for the selection of the third-party provider based on multiple objectives.
A hybrid mixed integer linear programming (MILP)-analytic hierarchy process (AHP) method was developed and applied to a reverse logistics network design problem (Galvez et al. Citation2015). The method was used to evaluate possible scenarios for a biogas plant logistics network with economic and environmental considerations. Recovery options in the process industry (beverages, packaging and construction chemicals) were investigated using case studies and expert analysis approach (Sellitto Citation2018).
A multi-criteria decision making (MCDM) approach was used for the selection of disposition alternatives assigned to returned products at the sorting-inspection stage (Agrawal, Singh, and Murtaza Citation2016). The study utilised the Graph Theory and Matrix Approach (GTMA) to evaluate four disposition alternatives based on their attributes such as market conditions, environmental impact and product value. Other MCDM studies have focused on third-party provider selection (Tavana et al. Citation2016; Mavi, Goh, and Zarbakhshnia Citation2017). A summary of some papers in the literature, shown in , indicates increasing interests in remanufacturing as new Industry 4.0 technologies and circular economy business models gain more momentum.
Table 1. Summary of papers on decision-making for remanufacturing and reverse logistics
Some recent studies have focused on product remanufacturability. For example, a fuzzy MCDM model was used to assess product remanufacturability based on product design, materials and manufacturing processes (Roy, Vimal, and Jayakrishna Citation2019). The model didn’t include supply chain characteristics in the assessment process. In addition to product remanufacturing characteristics, the success of remanufacturing relies also on the associated supply chains. A remanufacturing system with reverse logistics was investigated using simulation modelling to analyse the economic and environmental performance (Dev et al. Citation2020). The study considered real-time information sharing using Industry 4.0 principles for a refrigerator manufacturing company. Hybrid decision-making approaches were also used for studying remanufacturing supply chains. For example, a hybrid decision-making methodology was used to rank solutions to mitigate remanufacturing supply chain risks (Ansari, Kant, and Shankar Citation2020). The approach consists of stepwise weight assessment ratio analysis (SWARA) and complex proportional assessment of alternatives (COPRAS) methods. In addition to conceptual frameworks of decision-making, quantitative optimisation models were also developed to increase the profitability and efficiency of remanufacturing systems. Zhang and Chen (Citation2021) developed a mathematical optimisation model for production planning of manufacturing-remanufacturing systems. Both new and remanufactured products were assumed to have the same price in the market. Mathematical optimisation was also used to determine the optimal production schedule of manufacturing-remanufacturing systems (Aminipour, Bahroun, and Hariga Citation2021). The manufacturing and remanufacturing unit costs were assumed to be equal. Moreover, the modular structure of products was not included in the analysis. Modular design of products is very common in modern manufacturing systems. Modular design is also one of the criteria for better product remanufacturability (Lindkvist Haziri and Sundin Citation2020).
Mathematical optimisation was used in reverse logistics and closed-loop supply chains with a focus on location-allocation problems (Ilgin and Gupta Citation2010). The use of mathematical optimisation for production planning in remanufacturing supply chains has received less attention in the literature. Subulan and Tasan (Citation2013) used a fuzzy mixed integer programming model to optimise the production quantities of new and remanufactured products. In another paper, production planning was considered for a closed-loop supply chain with multiple products (Shi, Zhang, and Sha Citation2011). However, modular design of products was not considered in both studies. In order to fill the gap in the literature, a hybrid decision-making framework that combines multi-criteria decision making and mathematical optimisation is proposed in this paper. An introduction to the framework and the organisation of this paper is given in Section 3. The decision-making framework contributes to the existing literature in multiple ways:
The framework assists decision makers in formulating a network configuration that embraces remanufacturing as the preferred disposition alternative to enhance the transition to a circular economy system.
The framework considers product characteristics and supply chain structure using qualitative and quantitative analysis methods.
The framework considers a family of products with modular structures, the optimal selection of technologies to be implemented and quantities of new and remanufactured products.
3. Hybrid decision-making and optimisation framework
In light of the extended capacities enabled by modern manufacturing systems, this study considers an integrated plant in which manufacturing and remanufacturing activities are executed. The saved cost of establishing separate remanufacturing facilities with all the necessary support systems is a very important incentive for manufacturers to handle remanufacturing of returned products. Any extra cost incurred in implementing remanufacturing initiatives will eventually add to the price paid by customers making the remanufactured products less attractive and reducing their market demands.
Remanufacturing returned products by third-party remanufacturers is not the ideal approach because they lack the marketing capability of the original manufacturer as well as the ability to coordinate with distribution dealers and parts suppliers. Moreover, third-party remanufacturers would have separate reverse logistics networks making it difficult to benefit from the existing forward logistics infrastructure and communications systems. presents a summary of the differences between remanufacturing by original manufacturers and third-party remanufacturers.
Table 2. Comparison between the product manufacturer and third-party remanufacturers with respect to remanufacturing operations
The hybrid decision-making and optimisation framework proposed can be used by the manufacturer for product recovery and remanufacturing system planning. The application of the framework is illustrated for the case of household washing machines. The framework consists of a conceptual AHP model to determine the optimal configuration of the closed loop network and a mathematical optimisation model to optimise the level of technology implementation. The optimal network configuration obtained in the first part is used as an input element in the second mathematical optimisation part as illustrated in .
4. The analytic hierarchy process (AHP) model
The analytic hierarchy process (AHP) method developed by Saaty (Citation2016) is used in the decision-making framework because it allows the decision makers to impartially consider a set of sub-criteria and alternatives and convert subjective judgements into objective rankings of alternatives. To formulate the process, the goal, criteria, sub-criteria and alternatives are arranged in hierarchical interconnected levels. The sub-criteria and alternatives are pairwise compared to determine their priorities and calculate alternative rankings. The comparisons are made using the fundamental scale given in .
Table 3. The fundamental AHP pairwise comparison scale
Once the pairwise comparison matrices are formed, the consistency index (CI) of each n x n matrix is computed as
CI = (λmax – n)/(n – 1)
where λ max is the largest eigenvalue of the comparison matrix.
The consistency of judgements is measured by calculating the consistency ratio (CR) given by
CR = CI/RI
where RI is the random consistency index corresponding to the comparison matrix size as shown in (Saaty Citation2016).
Table 4. Random consistency index (RI)
If the value of CR is sufficiently small (about 10% or less), the consistency of the judgements is considered acceptable. The priority vector w for a pairwise comparison matrix A is found by solving the equation
Aw = λ max w
The overall rank of each alternative is then calculated by multiplying each alternative’s local priority by the priority of the corresponding sub-criterion and summing up the adjusted alternative’s priorities.
4.1. Configuration decisions and alternatives
While it is a preferred option for the original manufacturer to handle all processes in the manufacturing closed-loop system, it is usually necessary to delegate some of the tasks to other shareholders because of economic and cost saving reasons as well as better capability of those stakeholders to handle certain tasks. The closed loop system configuration needs to address the operation at the collection stage, inspection and disassembly stage and processing stage.
The decision-making process can be divided into a set of decisions. The decisions should provide the answers to the following questions
Who should perform the collection of returned products?
Who should inspect, sort and disassemble the returned products?
Who should process the returned products (remanufacturing, refurbishing and recycling options)?
The configuration decisions and their corresponding alternatives are shown in .
Table 5. The model decisions and their corresponding alternatives
In the AHP model, two phases of decision-making are identified. The first phase is concerned with product, processes and materials characteristics. The second phase consists of the supply and distribution network. This phase involves a higher level of coordination and collaboration between the manufacturer and other stakeholders.
When it comes to product design and manufacturing processes considered in the first phase, there are many ways that the decisions made can affect the overall remanufacturing and product recovery performance. For example, electronic products tend to fail unpredictably and therefore represent a challenge to remanufacture. Instead, manufacturers can disassemble and recycle the parts in the returned products. Because of their small materials content and relatively low cost of manufacturing, the economic, environmental and social advantages of remanufacturing are also limited compared to products with large materials content and high cost of manufacturing.
The decision-making process involves multi-criteria making the AHP approach suitable to assess the alternatives described. The criteria can be customised to suit different situations by adding or removing some of the criteria and/or alternatives. For each one of the above defined decision alternatives, an AHP ranking can be established to determine the optimum alternative. The overall network configuration would be composed of the set of optimum alternatives determined.
4.2. Criteria and sub-criteria of the AHP model
The model consists of two phases with two criteria that require input from different internal and external stakeholders. The first phase is concerned with the product design, materials requirements and process requirements (PMP) criterion and how they relate to the ease of recovery and remanufacturing feasibility as opposed to other disposition alternatives. In this phase a set of alternatives is compared from the perspective of the PMP criteria. The second phase consists of examining current supply and distribution structure (SDS) criterion and how they can be used to determine the preferred alternatives. Based on the preferred alternatives, a closed loop supply chain is configured to fulfill the objective of sustainable waste minimisation through appropriate disposition of recovered products.
4.2.1. Phase I: product, Materials and Process (PMP) Assessment
In this phase, a comprehensive assessment of the product, materials and processes is conducted to identify opportunities and limitations from product recovery perspective. A simple example is the plastic water bottle which has a simple design and is made of a single material. The manufacturing process is relatively inexpensive, and the bottle thickness is usually designed for a single use. In this case, remanufacturing may not be the ideal disposition alternative. Instead, material recycling is the only disposition channel as other disposition channels such as repair and reuse are not applicable. Therefore, collection of used bottles can be left for third-party collectors.
As the complexity of the product and the resources consumed in its design and manufacturing increase, remanufacturing becomes a more attractive alternative. Increasing product complexity tends to increase the potential benefits of product recovery and remanufacturing because of the higher cost of producing new products. It can also induce some technical and financial challenges regarding how the product can be acquired, inspected, disassembled, processed and remanufactured. Complexity increases with higher number of parts, product variety and manufacturing system requirements (ElMaraghy et al. Citation2012).
The size of the product affects the cost of product handling and transportation. Bulky products make remanufacturing a more preferred option and initiate interests in more manufacturer’s involvement in the product recovery and remanufacturing activities. The level of technology refers to electronic and computer systems in product design and manufacturing. Products with fast-changing technologies are more challenging to be remanufactured because the product value depreciates quickly with time. The expected volume of demand and returned products can be used to determine the level of investment at different stages in the closed-loop system. Lower volumes will likely lead to decisions that have lower cost of implementation.
If the product consists of several components as in the case of modular products, each component should be assessed separately. High levels of complexity, as in the case of automotive industry, may dictate breaking down the product into parts or modules that can be economically and practically managed for optimal product recovery. provides a description of the sub-criteria of Phase I.
Table 6. Phase I sub-criteria and the affected configuration decisions
4.2.2. Phase II: supply and distribution structure
The nature of the relationship between customers, manufacturers, suppliers and distribution dealers has an important role in determining the feasibility and extent of product remanufacturing. A high level of value recovery and remanufacturing requires a strong relationship between all supply chain parties. It also depends on the available supply infrastructure such as warehousing and inventory control systems.
A stronger manufacturer-supplier relationship increases the feasibility of supplier-managed processing of modules recovered from returned products. Products that are serviced and maintained by the manufacturers or their agents are more likely to be remanufactured. Therefore, the level of after-sales service is one of the sub-criteria needed to compare alternatives. The types of markets in which the products are sold and used can affect the demands for remanufactured products, the quality of the returned products and relationship with customers after sale.
The cost of transporting the returned products can be a deterring factor in the network activities if the distances travelled are very long. The transportation carbon footprint depends also on the geographic locations of facilities. Higher carbon footprint reduces the environmental benefits. This sub-criterion can affect all configuration decisions. A description of the sub-criteria in Phase II is given in .
Table 7. Phase II sub-criteria and the affected configuration decisions
The general AHP structure for the network configuration decision-making is illustrated in for Decision 1. The framework is applied for the case of washing machines in the next section to illustrate its use to make decisions in product recovery and remanufacturing of a product with significant levels of complexity and variety.
5. Implementation of the AHP model
The proposed framework is used for the case of washing machine’s industry, an industry that offer a variety of brands with a considerable level of complexity and production volumes. The potential benefits of implementing product recovery and remanufacturing programmesfor washing machines have attracted the attention of some researchers (Marconi et al. Citation2019; Lieder et al. Citation2017; Gnoni et al. Citation2017; Sousa-Zomer et al. Citation2017). Once the optimal configuration of the closed-loop network is determined, the mathematical optimisation model is used to optimise the technology implementation (Sections 6 and 7).
5.1. Pairwise comparison of sub-criteria
Pairwise comparisons of the sub-criteria in Phase I and II are performed using judgements based on washing machine industry practice as shown in to determine the priority of each sub-criterion. The comparisons were based on the relative importance of the sub-criteria and how they might affect the rankings of the alternatives of the decisions to be made. Since the judgements made to compare the sub-criteria are subjective and this may result in some inconsistencies in the comparison, the consistency of the comparison matrices is evaluated by calculating the consistency ratio CR for each matrix. A consistency ratio of 10% or less indicates that the judgements are consistent.
Table 8. Phase I sub-criteria pairwise comparison
Table 9. Phase II sub-criteria pairwise comparison
The pairwise comparisons of the sub-criteria in are used for the three decisions as the relative importance of the sub-criteria is deemed to be equally relevant to the three decisions to be analysed. The outcome of the hierarchical analysis for the three decisions will constitute the overall ranking of alternatives needed to determine the network configuration.
5.2. Decision 1: collection
In Phase I for Decision 1, the collection alternatives will be pairwise compared with respect to the five sub-criteria identified. This comparison will result in five comparison matrices corresponding to the five sub-criteria in Phase I. For the Complexity sub-criterion (PMP-1), collection by the manufacturer is more preferred than collection by the distribution dealer because the manufacturer has a better knowledge of the technical aspects of the washing machines than the distribution dealers. As the complexity of the product increases, it becomes more strongly preferred that the manufacturer handles the collection and initial assessment. Since the washing machines have a moderate complexity due to a limited number of critical parts, the manufacturer is considered moderately to strongly more preferred (a level of 4 on Saaty’s comparison scale from 1 to 9) than the distribution dealer. The distribution dealers are more preferred than third-party collection agents who would require more training efforts and resources to acquire the returned products. The complete pairwise comparison matrices for the alternatives in Decision 1 are given in for Phase I.
Table 10. Phase I pairwise comparison matrices for alternatives in Decision 1
In Phase II, the alternatives are pairwise compared to determine the more feasible alternative. The comparison of the alternatives is shown in . The relationship between the manufacturer and suppliers will have no influence on the collection decision. Therefore, there are only four matrices in this phase for collection. In the washing machine market, the manufacturer will have a limited number of distribution dealers who are in most cases retailers selling different lines of products from multiple manufacturers. The established logistics and information channels with the distribution dealers makes collection by the dealers a more feasible alternative than the manufacturer and the third-party collection agent. The after-sales services are usually managed by the manufacturer authorised technical agents and that makes the manufacturer more suitable for collection than the distribution dealer and the third-party collection agent because they lack access to service history. However, collection by the distribution dealer is more feasible than the other two alternatives from the diverse consumer market and wide geographic product distribution network perspectives.
Table 11. Phase II pairwise comparison matrices for alternatives in Decision 1
5.3. Decision 2: inspection, sorting and disassembly
In Decision 2, the inspection, sorting and disassembly alternatives are compared with respect to sub-criteria in Phase I and II. As the washing machine complexity increases due to market and government regulation related reasons, inspection and subsequent sorting and disassembly of the returned products require a higher level of technical knowledge. Moreover, the manufacturer may decide not to share the advanced technology features of the product with other parties. Therefore, from the complexity and technology perspectives it would be more preferred that the manufacturer performs these tasks rather than delegating them to the distribution dealer or a third-party agent.
With the relatively high prices of new washing machines, the potential benefits from the recovered products and modules may justify the capital investment in a manufacturer-run inspection, sorting and disassembly facilities. Thus, the manufacturer is a more preferred alternative than the distribution dealer and the third-party agent. Additionally, the bulky size of the product may require a more expensive materials handling system and therefore it is more preferred to have a centralised facility to receive returned products from multiple distribution dealers to improve the economy of scale. The alternative pairwise comparison matrices for Phase I is shown in .
Table 12. Phase I pairwise comparison matrices for alternatives in Decision 2
The alternatives pairwise comparison matrices for Phase II are given in . Considering the relationship between the manufacturer and distribution dealers in the washing machine market, the distribution dealers who carry products from different, usually competing, manufacturers are not likely to be a suitable alternative for handling the inspection and disassembly of the returned products. Thus, it is more feasible to have these tasks done by the manufacturer. The manufacturer is also more capable of dealing with the variations in the condition of the returned products that come from diverse markets and patterns of use. Additionally, the manufacturer can use after-sales maintenance records to get better inspection results. However, the global geographic regions in which the products are used can make it more feasible for the distribution dealers to inspect the returned products because they are familiar with the local geographic region they serve.
Table 13. Phase II pairwise comparison matrices for alternatives in Decision 2
5.4. Decision 3: processing
The pairwise comparison matrices for Decision 3 are given in for Phase I and II respectively. Once the returned products are disassembled the complex and critical modules should ideally be processed by their respective suppliers because they manage the facilities in which the modules were originally manufactured. The suppliers are also more capable of efficiently processing large modules and ensuring that their quality and technology levels are adequate. In order to sell the remanufactured products at a lower price, the modules should be processed by the manufacturer rather than the supplier or a third-party agent as this reduces transportation costs particularly when the volumes to be processed are large.
Table 14. Phase I pairwise comparison matrices for alternatives in Decision 3
Table 15. Phase II pairwise comparison matrices for alternatives in Decision 3
The existing work relationship between the manufacturer and the suppliers can be extended to cover the processing of the returned modules. However, the after-sales services that are provided by a local service agent and the wide geographical distribution of washing machines make assigning the processing of modules to local third-party agents a more feasible alternative.
5.5. Results of the AHP model
The analysis was performed for five cases with different weights assigned to criteria in Phase I and II as shown in to evaluate the effect on the results. A summary of global priorities and rankings of alternatives is given in for case 3. For the first decision, the collection of returned products by the distribution dealer is the preferred alternative. The distribution dealers would be responsible for motivating the customers to register their products for after-sales services managed by the manufacturer’s authorised agents and to return their products to the dealer at the end of the service term. Once the products are returned, the distribution dealers arrange for shipping them to the assigned inspection, sorting and disassembly facilities managed by the manufacturer. After sorting and disassembly, the returned modules are sent to the suppliers for processing. Modules and parts that can be remanufactured are processed by the suppliers in the disassembly location and sent directly to the integrated manufacturing-remanufacturing plant. Any items that can’t be processed for value recovery are sent to disposal sites.
Table 16. Weights assigned to phase I and II for the five cases of the analysis
Table 17. Summary of global priorities and rankings for alternatives of the decisions (phase I and II have equal weights)
The elimination of third-party collection will protect the manufacturer market share against the used product market. Therefore, the market demand for remanufactured products is likely to increase. In order to evaluate the sensitivity of the results to the weights given to phase 1 and 2, the results for the cases in are shown in for decisions 1, 2 and 3 respectively. For decision 1, the results show that collection by the distribution dealer was the preferred alternative for cases 1, 2 and 3. However, as the weight of phase 1 (product, materials and processes) increases collection by the manufacturer gains higher score and becomes the highest ranking alternative in cases 4 and 5. For the inspection and disassembly activities, the direct control by manufacturer is significantly more preferred than the other two alternatives in all cases. The strong preference of manufacturer-controlled inspection and disassembly can be attributed to the technical expertise needed for effective execution of tasks. Moreover, the distribution dealer and third-party agents may lack the necessary technology and infrastructure required for the activities. For the third decision (processing), the results in indicate that the supplier alternative has the highest ranking in all cases. However, the third-party processor alterative ranking approaches the highest ranking as the weight of phase 2 criterion increases (cases 1 and 2). On the other end, the manufacturer alternative ranking approaches the highest ranking as the weight of phase 1 criterion increases (cases 4 and 5). A network configuration based on the outcome of the framework for case 3 is illustrated in .
6. Mathematical optimisation of technology selection
Once the network configuration is determined, the second step in the framework is to use mathematical optimisation to determine the optimal technology selection. The objective of the mathematical model is to select the optimum number of technologies that would maximise the manufacturer net profit. With the large number of new technologies that can be used in inspection, disassembly, remanufacturing and other tasks for products and modules, the mathematical optimisation model can determine the optimal number of technologies to be used, and the new and remanufactured product quantities. The model considers the case of a family of products and each product consists of a number of modules. The modular structure of each product variant is given by a product-module index Xij which has a value of 1 if product i has module j or 0 if product i doesn’t have module j. The sets, parameters and decision variables of the model are given in .
Table 18. Sets and parameters used for the mathematical model
Table 19. Decision variables for the mathematical model
The flow of materials is determined from the network configuration as shown in . It is assumed that each module is purchased from a single supplier. The new products are shipped to a collection dealer who also acquires the returned products from the customers and sends them to the disassembly and inspection facility. The disassembly and inspection facility is located at the manufacturing-remanufacturing facility site. The module supplier buys back the recycled modules to reduce the raw materials requirements for new modules. The refurbished modules are sold to the spare part market. The remanufactured modules are used for the production of remanufactured products. Remaining remanufactured modules are sold as spare parts.
The mathematical model can be formulated as
Objective function
Subject to
Capacity Constraints
The objective function in EquationEquation (1)(1)
(1) maximises the net profit. The equation accounts for revenues from the sale of new, remanufactured products, recycled, refurbished and remaining remanufactured modules. The expenses include the costs of manufacturing and remanufacturing products, costs of acquisition, disassembly and inspection of returned products. The expenses associated with modules include the costs of purchasing new module, remanufacturing, refurbishing and disposal of returned modules. The product transportation costs are the transportation cost of new products to the distribution dealer and the transportation cost of returned products to the disassembly and inspection facility. The module transportation costs are the transportation costs of new, recycled and refurbished modules and the cost of module transportation to the disposal site. The equation also includes the fixed costs of collection, disassembly and inspection, manufacturing-remanufacturing and refurbishing facilities. The fixed costs are overhead cost and payments to cover the capital cost of equipment and other operation and maintenance costs. The implementation of new technologies would incur additional capital and operation cost while reducing the unit processing costs of products and modules. The model considers the technologies for the product disassembly and inspection, module refurbishing and product and module remanufacturing.
EquationEquations (2)(2)
(2) and (Equation3
(3)
(3) ) ensures that the quantities of new and returned modules equal to the quantities needed for new products and retrieved from returned products respectively. The quantity of returned modules equals the sum of remanufactured, refurbished, refurbished and discarded modules as represented in EquationEquation (4)
(4)
(4) . EquationEquation (5)
(5)
(5) states that the quantity of returned products can not exceed the quantity of new products. EquationEquations (6)
(6)
(6) , (Equation7
(7)
(7) ) and (Equation8
(8)
(8) ) represent the proportions of remanufactured, refurbished and recycled modules. The market demands for new products are to be met by the new and remanufactured products as given by EquationEquation (9)
(9)
(9) . The capital investment limit for technology implementation is represented by EquationEquation (10)
(10)
(10) . EquationEquations (11)
(11)
(11) , (Equation12
(12)
(12) ), (Equation13
(13)
(13) ) and (Equation14
(14)
(14) ) ensure that the operation capacities are not exceeded at the collection centre, manufacturing-remanufacturing plant, disassembly and inspection, and refurbishing facilities respectively. The nonnegativity constraint for all product and module quantities is expressed in EquationEquation (15)
(15)
(15) . EquationEquations (16)
(16)
(16) and (Equation17
(17)
(17) ) define binary variables that determine whether a given technology is selected for the processing of products and modules respectively.
7. Implementation of the mathematical optimisation model
To illustrate the application of the mathematical model, a case of a manufacturer who produces seven models of washing machines with 14 modules is considered. The washing machine family of products was adapted from ElMaraghy and AlGeddawy (Citation2012). The parameters used for the case study are consistent with other studies in the literature (Jeihoonian et al. Citation2017; Alumur et al. Citation2012; Annamalai et al. Citation2012).
7.1. Model data
The product-module index values are shown in (ElMaraghy and AlGeddawy Citation2012). The disposal rate of modules is assumed to be 5%. The product and module parameters are given in respectively. The transportation cost of new products from the manufacturer to the distribution dealer and the transportation cost of returned products from the distribution dealer to the disassembly and inspection facility are both taken as $1 per unit. The transportation cost of modules is taken as a flat rate of $0.3 per unit. The fixed cost of collection, disassembly and inspection, refurbishing and manufacturing-remanufacturing facilities are taken as $1.2 million, $2 million, $8 million and $2 million, respectively. The fixed costs of facilities increase with the implementation of technologies that reduce the processing cost per unit. The total cost of technology implementation is limited by a capital investment cost $500,000.
Table 20. Product-module index values Xij (adapted from ElMaraghy and AlGeddawy Citation2012)
Table 21. Values of product parameters
Table 22. Values of module parameters
7.2. Results and discussion
The market demand for remanufactured products is one of the important factors to consider in planning product recovery and remanufacturing. To compare the results, the model was solved for multiple scenarios based on the minimum percentage of remanufactured products for each variant (r). The scenarios considered and the corresponding set of selected technologies are shown in . In scenario 1, there is no minimum remanufactured product requirement. Scenario 2 represents the case in which the minimum remanufactured product market demand for each variant (r) is 5%. Scenarios 3 to 6 represent cases of higher remanufactured product requirements to be supplied to the market. The results show that a higher net profit is expected for lower percentage requirements of remanufactured products. The low remanufactured product percentage for each product variant allows the manufacturer to increase the remanufacturing of variants that are more profitable to remanufacture. Scenario 1 leads to the highest net profit. However, some product variants are supplied to the market as new products only while others are produced as remanufactured products.
Table 23. Results of mathematical optimisation for six scenarios
The sets technologies selected for different scenarios have also varied to suit the quantities of recovered and remanufactured products for each scenario. The net profit and total remanufactured product percentage for each scenario are shown graphically in . The results show that for low remanufactured product variant percentage requirements, the net profit gets lower as the percentage requirement increases. The total remanufactured product percentage decreases for scenarios 1, 2 and 3. At higher remanufactured product percentage requirements, the net profit gets lower while the total remanufactured product percentage increases (scenarios 4, 5 ad 6). As the percentage requirement increases, the manufacturer ability to remanufacture the more profitable variants decreases. As the percentage requirement continues to increase, the manufacturer is forced to produce more remanufactured products, and this leads to higher total remanufactured product percentage and lower net profits.
8. Conclusions
A manufacturer driven product recovery and remanufacturing system is an emerging trend in many industries. The proposed hybrid decision-making framework provides a basis for determining the preferred network configuration and optimising technology selection using an AHP methodology and a mathematical optimisation model. In order to deal with complexity involved in the decision-making process, the preferred configuration is composed of the outcome of the preferred alternatives for a set of three decisions that address the collection of returned products, disassembly and inspection as well as processing of returned modules. The results of the AHP model part show that disassembly and inspection by the manufacturer is a strongly preferred alternative for all cases indicating the importance of this operation for the success of the integrated manufacturing-remanufacturing system. The rankings of alternatives vary depending on the weights of product-related criteria and logistics-related criteria. As the weight of product, materials and process criteria increases, collection by the manufacturer gains higher score (for weights of 0.75 and 0.9, the manufacturer becomes the preferred alternative for collection). The framework can be expanded and customised to suit various needs of the decision makers by adding and/or modifying the decisions, alternatives and sub-criteria.
Based on the network configuration obtained using the preferred alternatives, the mathematical optimisation model was formulated to select the set of technologies. The results show that the required level of remanufactured product variants leads to different sets of selected technologies. At lower levels of remanufactured product variant requirements, the net profit was higher as the manufacturer can have the ability to remanufacture the more profitable product variants. The technology selection varies depending on remanufactured product requirements. This justifies the investment in a reconfigurable system with high technology implementation to increase responsiveness. As a future research extension, other multi-criteria decision-making (MCDM) methods can be employed to enrich the decision-making outcomes as well as solving larger instances of the network using heuristics methods.
Disclosure statement
No potential conflict of interest was reported by the author(s).
Additional information
Notes on contributors
Saleh Bagalagel
Saleh Bagalagel has earned his PhD in Industrial and Manufacturing Systems Engineering in 2021 at the University of Windsor, Canada. His research interests are in the areas of decision-making and optimisation models of integrated manufacturing and closed-loop supply chain systems, development of sustainable manufacturing systems using Circular Economy principles and the application of smart technologies in product recovery and remanufacturing systems. He also holds MSc degree in Mechanical Engineering and MEng degree in Industrial Engineering.
Waguih ElMaraghy
Dr. Waguih ElMaraghy is a Professor of Engineering and Director of the Intelligent Manufacturing Systems (IMS) Centre at the University of Windsor, Canada. He is an international expert in engineering design, manufacturing, industrial productivity, and competitiveness. He has a number of Patents and over 300 publications and Industry reports. He is a Fellow of the following societies: The Society of Manufacturing Engineers (SME), the Canadian Society for Mechanical Engineering (CSME), the American Society of Mechanical Engineers (ASME), and the International Academy of Production Engineering (CIRP). Dr. ElMaraghy is a Fellow of the Canadian Academy for Engineering (CAE) and a Fellow of Engineers Canada (EC).
References
- Agrawal, S., R. K. Singh, and Q. Murtaza. 2015. “A Literature Review and Perspectives in Reverse Logistics.” Resources, Conservation and Recycling 97: 76–92. doi:https://doi.org/10.1016/j.resconrec.2015.02.009.
- Agrawal, S., R. K. Singh, and Q. Murtaza. 2016. “Disposition Decisions in Reverse Logistics: Graph Theory and Matrix Approach.” Journal of Cleaner Production 137: 93–104. doi:https://doi.org/10.1016/j.jclepro.2016.07.045.
- Alumur, S A, Nickel, S, Saldanha-da-Gama, F, and Verter V. 2012. Multi-period reverse logistics network design. European Journal of Operational Research, 220(1): 67–78. https://doi.org/10.1016/j.ejor.2011.12.045
- Aminipour, A., Z. Bahroun, and M. Hariga. 2021. “Cyclic Manufacturing and Remanufacturing in a Closed-loop Supply Chain.” Sustainable Production and Consumption 25: 43–59. doi:https://doi.org/10.1016/j.spc.2020.08.002.
- Annamalai K, Naiju C, Karthik S, and Prashanth M Mohan. 2012. Early Cost Estimate of Product during Design Stage Using Design for Manufacturing and Assembly (DFMA) Principles. AMR 622-623: 540-544.
- Ansari, Z. N., R. Kant, and R. Shankar. 2020. “Evaluation and Ranking of Solutions to Mitigate Sustainable Remanufacturing Supply Chain Risks: A Hybrid Fuzzy SWARA-fuzzy COPRAS Framework Approach.” International Journal of Sustainable Engineering 13 (6): 473–494. doi:https://doi.org/10.1080/19397038.2020.1758973.
- Butzer, S., D. Kemp, R. Steinhilper, and S. Schötz, 2016. “Identification of Approaches for Remanufacturing 4.0.” in: Presented at the 2016 IEEE European Technology and Engineering Management Summit (E-TEMS), IEEE, Frankfurt am Main, Germany, pp. 1–6. doi:https://doi.org/10.1109/E-TEMS.2016.7912603
- Chakraborty, K., S. Mondal, and K. Mukherjee. 2017. “Analysis of Product Design Characteristics for Remanufacturing Using Fuzzy AHP and Axiomatic Design.” Journal of Engineering Design 28 (5): 338–368. doi:https://doi.org/10.1080/09544828.2017.1316014.
- Dev, N K, Shankar, R, and Qaiser F Hasan. 2020. Industry 4.0 and circular economy: Operational excellence for sustainable reverse supply chain performance. Resources, Conservation and Recycling, 153: 104583. DOI: https://doi.org/10.1016/j.resconrec.2019.104583
- ElMaraghy, H., and T. AlGeddawy. 2012. “New Dependency Model and Biological Analogy for Integrating Product Design for Variety with Market Requirements.” Journal of Engineering Design 23 (10–11): 722–745. doi:https://doi.org/10.1080/09544828.2012.709607.
- ElMaraghy, W., H. ElMaraghy, T. Tomiyama, and L. Monostori. 2012. “Complexity in Engineering Design and Manufacturing.” CIRP Annals 61 (2): 793–814. doi:https://doi.org/10.1016/j.cirp.2012.05.001.
- Fleischmann, M., J. M. Bloemhof-Ruwaard, R. Dekker, E. Van der Laan, J. A. Van Nunen, and L. N. Van Wassenhove. 1997. “Quantitative Models for Reverse Logistics: A Review.” European Journal of Operational Research 103 (1): 1–17. doi:https://doi.org/10.1016/S0377-2217(97)00230-0.
- Galvez, D., A. Rakotondranaivo, L. Morel, M. Camargo, and M. Fick. 2015. “Reverse Logistics Network Design for a Biogas Plant: An Approach Based on MILP Optimization and Analytical Hierarchical Process (AHP).” Journal of Manufacturing Systems 37: 616–623. doi:https://doi.org/10.1016/j.jmsy.2014.12.005.
- Gnoni, M. G., G. Mossa, G. Mummolo, F. Tornese, and R. Verriello. 2017. “Supporting Circular Economy through Use-Based Business Models: The Washing Machines Case.” Procedia CIRP 64: 49–54. doi:https://doi.org/10.1016/j.procir.2017.03.018.
- Guide, V. D. R., and L. N. Van Wassenhove. 2009. “OR FORUM—The Evolution of Closed-Loop Supply Chain Research.” Operations Research 57 (1): 10–18. doi:https://doi.org/10.1287/opre.1080.0628.
- Ilgin, M. A., and S. M. Gupta. 2010. “Environmentally Conscious Manufacturing and Product Recovery (ECMPRO): A Review of the State of the Art.” Journal of Environmental Management 91: 563–591. doi:https://doi.org/10.1016/j.jenvman.2009.09.037.
- Jeihoonian. M, Kazemi Zanjani. M, and Gendreau. M. 2017. Closed-loop supply chain network design under uncertain quality status: Case of durable products. International Journal of Production Economics, 183: 470–486. doi: https://doi.org/10.1016/j.ijpe.2016.07.023
- Kumar, S., and V. Putnam. 2008. “Cradle to Cradle: Reverse Logistics Strategies and Opportunities across Three Industry Sectors.” International Journal of Production Economics 115 (2): 305–315. doi:https://doi.org/10.1016/j.ijpe.2007.11.015.
- Kurilova-Palisaitiene, J., E. Sundin, and B. Poksinska. 2018. “Remanufacturing Challenges and Possible Lean Improvements.” Journal of Cleaner Production 172: 3225–3236. doi:https://doi.org/10.1016/j.jclepro.2017.11.023.
- Lieder, M., F. M. A. Asif, A. Rashid, A. Mihelič, and S. Kotnik. 2017. “Towards Circular Economy Implementation in Manufacturing Systems Using a Multi-method Simulation Approach to Link Design and Business Strategy.” The International Journal of Advanced Manufacturing Technology 93 (5–8): 1953–1970. doi:https://doi.org/10.1007/s00170-017-0610-9.
- Lindkvist Haziri L, and Sundin E. 2020. Supporting design for remanufacturing - A framework for implementing information feedback from remanufacturing to product design. Jnl Remanufactur 10(1): 57–76. DOI: https://doi.org/10.1007/s13243-019-00074-7
- Marconi, M., M. Germani, M. Mandolini, and C. Favi. 2019. “Applying Data Mining Technique to Disassembly Sequence Planning: A Method to Assess Effective Disassembly Time of Industrial Products.” International Journal of Production Research 57 (2): 599–623. doi:https://doi.org/10.1080/00207543.2018.1472404.
- Mavi, R. K., M. Goh, and N. Zarbakhshnia. 2017. “Sustainable Third-party Reverse Logistic Provider Selection with Fuzzy SWARA and Fuzzy MOORA in Plastic Industry.” The International Journal of Advanced Manufacturing Technology 91 (5–8): 2401–2418. doi:https://doi.org/10.1007/s00170-016-9880-x.
- Prakash, C., and M. K. Barua. 2015. “Integration of AHP-TOPSIS Method for Prioritizing the Solutions of Reverse Logistics Adoption to Overcome Its Barriers under Fuzzy Environment.” Journal of Manufacturing Systems 37: 599–615. doi:https://doi.org/10.1016/j.jmsy.2015.03.001.
- Rogers, D. S., and R. Tibben-Lembke. 2001. “An Examination of Reverse Logistics Practices.” Journal of Business Logistics 22 (2): 129–148. doi:https://doi.org/10.1002/j.2158-1592.2001.tb00007.x.
- Roy, R., K. E. K. Vimal, and K. Jayakrishna. 2019. “Development of a Framework Model to Explore the Remanufacturing Feasibility of Automotive Components.” In Research into Design for a Connected World, edited by A. Chakrabarti, 381–390. Singapore: Springer Singapore. doi:https://doi.org/10.1007/978-981-13-5974-3_33.
- Saaty, T. L. 2016. “The Analytic Hierarchy and Analytic Network Processes for the Measurement of Intangible Criteria and for Decision-Making.” In Multiple Criteria Decision Analysis, edited by S. Greco, M. Ehrgott, and J. R. Figueira, 363–419. New York: Springer New York. doi:https://doi.org/10.1007/978-1-4939-3094-4_10.
- Sellitto, M. A. 2018. “Reverse Logistics Activities in Three Companies of the Process Industry.” Journal of Cleaner Production 187: 923–931. doi:https://doi.org/10.1016/j.jclepro.2018.03.262.
- Senthil, S., K. Murugananthan, and A. Ramesh. 2018. “Analysis and Prioritisation of Risks in a Reverse Logistics Network Using Hybrid Multi-criteria Decision Making Methods.” Journal of Cleaner Production 179: 716–730. doi:https://doi.org/10.1016/j.jclepro.2017.12.095.
- Shi, J., G. Zhang, and J. Sha. 2011. “Optimal Production Planning for a Multi-product Closed Loop System with Uncertain Demand and Return.” Computers & Operations Research 38 (3): 641–650. doi:https://doi.org/10.1016/j.cor.2010.08.008.
- Sirisawat, P., and T. Kiatcharoenpol. 2018. “Fuzzy AHP-TOPSIS Approaches to Prioritizing Solutions for Reverse Logistics Barriers.” Computers & Industrial Engineering 117: 303–318. doi:https://doi.org/10.1016/j.cie.2018.01.015.
- Sousa-Zomer, T. T., L. Magalhães, E. Zancul, and P. A. Cauchick-Miguel. 2017. “Lifecycle Management of Product-service Systems: A Preliminary Investigation of A White Goods Manufacturer.” Procedia CIRP 64: 31–36. doi:https://doi.org/10.1016/j.procir.2017.03.041.
- Subulan, K., and A. S. Tasan. 2013. “Taguchi Method for Analyzing the Tactical Planning Model in a Closed-loop Supply Chain considering Remanufacturing Option.” The International Journal of Advanced Manufacturing Technology 66 (1–4): 251–269. doi:https://doi.org/10.1007/s00170-012-4322-x.
- Tavana, M., M. Zareinejad, F. J. Santos-Arteaga, and M. A. Kaviani. 2016. “A Conceptual Analytic Network Model for Evaluating and Selecting Third-party Reverse Logistics Providers.” The International Journal of Advanced Manufacturing Technology 86 (5–8): 1705–1721. doi:https://doi.org/10.1007/s00170-015-8208-6.
- Tolio, T., A. Bernard, M. Colledani, S. Kara, G. Seliger, J. Duflou, O. Battaia, and S. Takata. 2017. “Design, Management and Control of Demanufacturing and Remanufacturing Systems.” CIRP Annals 66 (2): 585–609. doi:https://doi.org/10.1016/j.cirp.2017.05.001.
- Tosarkani, B. M., and S. H. Amin. 2018. “A Multi-objective Model to Configure an Electronic Reverse Logistics Network and Third-party Selection.” Journal of Cleaner Production 198: 662–682. doi:https://doi.org/10.1016/j.jclepro.2018.07.056.
- Yang, S. S., N. Nasr, S. K. Ong, and A. Y. C. Nee. 2017. “Designing Automotive Products for Remanufacturing from Material Selection Perspective.” Journal of Cleaner Production 153: 570–579. doi:https://doi.org/10.1016/j.jclepro.2015.08.121.
- Yang, S., A. M. R., J. Kaminski, and H. Pepin. 2018. “Opportunities for Industry 4.0 To Support Remanufacturing.” Applied Sciences 8 (7): 1177. doi:https://doi.org/10.3390/app8071177.
- Zhang, Y., and W. Chen. 2021. “Optimal Production and Financing Portfolio Strategies for a Capital-constrained Closed-loop Supply Chain with OEM Remanufacturing.” Journal of Cleaner Production 279: 123467. doi:https://doi.org/10.1016/j.jclepro.2020.123467.